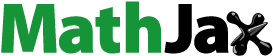
ABSTRACT
Cultivated land system confronts with a series of problems hindering its socioeconomic and ecological sustainability. Sustainable intensification of cultivated land use (SICLU) is considered as an efficient method to achieve three aspects of sustainable goals in society, economy and ecology simultaneously. Farmers, the main executor of all agricultural strategies, are the core of SICLU research. However, there is a small quantity of researches on SICLU from the perspective of farmers’ social psychology. Therefore, this article measured the level of SICLU at the farmer scale using super-efficiency SBM model, and relying on structural equation modeling and an innovative research framework, explored the association mechanism among farmers’ cognition, intention and behavior of SICLU. Finally, the results showed that (1) most of samples are at a medium or higher level of SICLU. (2) all samples’ cognition are significantly positively related to their behavior and their behavior significantly negatively affect their intention. (3) correlation characteristics among them are slightly different under each level. The proposed research outline not only expand the scope of SI research to the perspective of social psychology in breadth, but in depth take targeted actions to protect agricultural environment, make cultivated land system sustainable and alleviate food crisis.
1. Introduction
Over the past decades, several food crises have occurred all around the world and they have also sparked a host of problems at the same time (FAO, Citation2019; IPCC, Citation2020; UN, Citation2015). In order to maximize agricultural outputs, thousands of chemical products, such as fertilizers and pesticides, have been poured into the cultivated land (FAOSTAT, Citation2018; Rahman & Zhang, Citation2018; Xiao et al., Citation2021), and which threatened the sustainability of cultivated land ecosystem (Lyu et al., Citation2022; McLaughlin & Mineau, Citation1995; Wu et al., Citation2021) and adversely exacerbated global food security (Jalilov et al., Citation2022; Siebrecht & Schmid, Citation2020). Cultivated land is one of the most fundamental elements for food production (Ichinose et al., Citation2020) and it is also the main source of livelihood for local farmers (Aravindakshan et al., Citation2021). Therefore, we should acknowledge that maintaining the sustainability of cultivated land ecosystems is a prerequisite to responding to food crises (FAO, Citation2022).
Sustainable Intensification (SI) emphasizes relatively higher outputs with fewer inputs, going along with no deterioration or improvement in surrounding environmental conditions (Burke et al., Citation2022; Petersen & Snapp, Citation2015). In response to global food security and agriculture environmental aggravation, SI has been proposed by the United Nations as an effective mode to increase food supply, conserve biodiversity and enhance ecosystem resilience (Kurgata et al., Citation2018; Petersen & Snapp, Citation2015). SI research is constantly advancing globally. A large number of theoretical and empirical investigations (Lyu et al., Citation2021; Mullan et al., Citation2022; Niu et al., Citation2021) on SI have been conducted, Jules et al. (Citation2018) provided a comprehensive review of the progress about SI in agricultural system at the global scale with seven SI subsystems; Jain et al. (Citation2020) assessed the impact of SI practices on food production in the South Africa and the results showed SI practices positively affected food production; As a result of contrasting global SI development planning with actual processes, Cassman and Grassini (Citation2020) found that there was a mismatch between them and, in the follow-up process for promoting SI, a priority implementation framework should be firstly formulated.
SI is considered one of the most effective methods to solve the serious problems brought by global agriculture. Although the literature about it is rich in content and abundant in quantity, the amount of SI research based on the perspective of cultivated land use is quite limited. Sustainable Intensification of Cultivated Land Use (SICLU) is a concrete application of SI in cultivated land systems (Peng et al., Citation2020). There is a close connection among cultivated land use, food security, social stability, and farmers’ livelihoods. SICLU is a practicable alternative to coordinate social, economic and ecologically sustainable development and to optimize agricultural management models (Niu et al., Citation2018). So, it is extremely urgent to explore the SI in cultivated land use.
Farmers are the pivot during agriculture activities, and also the most basic and important micro socio-economic entity and independent decision-making unit in cultivated land use (Galeana-Pizaña et al., Citation2021). The Global Food Policy Report once pointed out that small farmers have become the main contributors to global food security and nutrition. Farmers’ behaviour of cultivated land use is the result of rational choice and a combination of management scale, management methods, resource utilization and investments, which can directly affect the quality of rural economic development and the direction of land use transformation (Lyu et al., Citation2022). Especially, with the deepening of research on SI, the social benefits of SI have also been more widely reflected in relevant studies (Loos et al., Citation2014; Petersen & Snapp, Citation2015), involving adjusting dietary structure, promoting distribution equity, narrowing the wealth gap, and ensuring social equity. Therefore, from the perspective of farmers, making clear the correlation mechanism and impact path between farmers’ cognition, intention, and behaviour can be conducive to solving practical problems about the activities of cultivated land use. The relationship among the three is sophisticated and constantly changing with research objectives altering. At present, although most of the literature explores the interrelationship just between intention and behaviour based on the Theory of Planned Behaviour (TPB) theory (Ataei et al., Citation2021; Elahi et al., Citation2022; Maleksaeidi & Keshavarz, Citation2019; Tama et al., Citation2021), there is a paucity of studies focusing on the interaction and correlation among the cognition, intention and behaviour. However, substantial evidence has shown that farmers’ cognition has a direct impact on their environmental protection behaviour and sustainability intention. Zhang et al. (Citation2021), regarding farmers’ waste management investment, detected that farmers’ environmental responsibility and policy cognition positively influenced their intention and behaviour, and that policy cognition promoted investment behaviour to manage waste in rural production. Guo et al. (Citation2021) found that farmers’ pro-environmental behaviour and intention were inconsistent, and the higher the farmers’ cognition, the less likely they were to do. The farmers’ cognition, intention, and behaviour of cultivated land use are directly associated with the realization of SI. Exploring the mechanism among the three towards SI is particularly important for optimizing cultivated land use patterns, and maintaining food security and ecological sustainability.
China, a large country majoring in agriculture and having a huge population, feeds about 22% of the world’s population occupying only 10% of the world’s cultivated land (Chen et al., Citation2021), as a result cultivated land is undertaking more enormous pressures on production and the productivity of cultivated land is also constantly declining (Xu et al., Citation2023; Zhao et al., Citation2018). In addition, increasing agricultural pollution has a strong impact on sustainable development (Wang et al., Citation2023). According to the National Bureau of Statistics in China, the consumption of fertilizer was 41.46 million tons in 2000, and ratcheted up to 52.51 million tons by 2020. For the sake of reconciling regional and global food damaged with cultivated land sustainability, there is an urgent need to achieve sustainable cultivated land use under the premise of moderate intensification (Niu et al., Citation2022; Petersen & Snapp, Citation2015; Song & Xin, Citation2021; Wu & Xu, Citation2019). SICLU research is important and urgent for China. However, the research on SI in China started relatively late and is still in the exploratory and initial stages. The overall number of literature is relatively small and the research content is single. In theoretical research, Chinese scholars often focused on distinguishing and clarifying the concepts of SICLU and some similar connotations. In addition, some literature reviewed existing international research summarized theoretical exploration system and concluded the experience of international empirical research, which provided a theoretical basis for the study of SICLU in China (Lyu et al., Citation2020; Peng et al., Citation2020; Zuo et al., Citation2018). The empirical research in terms of SICLU in China is relatively limited (Lu et al., Citation2015; Xie et al., Citation2021). Although there were comprehensive explorations that combined with themes such as rural land transfer, rural land ownership confirmation, and farmers’ livelihood transformation, the quantity was very small and almost concentrated on the micro-scale of farmers. Most empirical explorations conducted at the provincial, regional, and national levels were mainly based on level measurement and comprehensive evaluation.
This study is based on the important significance of SI and the special position of farmers in cultivated land use. It extended the traditional TPB and innovatively constructed a research framework for the association mechanism of farmers’ cognition, intention, and behaviour towards SICLU. The super-efficiency SBM model was employed to measure the level of SICLU and the structural equation modelling (SEM) quantified the related path coefficient and made the association among them clearer, which would provide a reference for guiding farmers to transform their mode of cultivated land use towards SI and ensure regional and global food security and ecological security. The possible academic contributions of this paper include: (1) International academic community has had a large amount of empirical research around SI from the perspective of small-scale farmers. However, the literature, focusing on cultivated land use, building the theoretical framework of farmers’ cognition, behaviour and willingness, and then conducting the correlation study between farmers’ cognition, intention, and behaviour towards SI, is relatively limited. The content of this paper is consistent with the connotation of China’s cultivated land protection strategy and global sustainable development goals (SDGs). In practice, it helps to alleviate the global food crisis and promote the transformation of cultivated land use patterns. In theory, it can expand and enrich research on SI in China and all over the world. (2) We extend traditional TPB to the discussion of three aspects cognition, intention, and behaviour. Based on the connotation and significance of SICLU, we innovatively construct a research framework for the correlation mechanism among the three at the household level, extend the application of TPB, explore the social and psychological characteristics of farmers in SICLU, and analyse the implementation path of SICLU at a micro-scale.
2. Materials and methods
2.1. Theoretical analysis
SICLU is a mode to manage cultivated land, which emphasizes exploiting efficiently cultivated land in a certain area, increasing production, lowering or not expanding negative environmental impacts, ameliorating ecosystem services, strengthening the resilience of cultivated land use system, and achieving multilateral sustainable development. The notion of SICLU integrates intensive management, high productivity, resource conservation, non-degradation of the ecological environment, and social sustainability. It plays a positive role in the current food crisis, agricultural non-point source pollution, the green development of cultivated land as well as the sustainable use of resources (Xie et al., Citation2021). The SICLU process is actually a process of sustainable utilization of resources and is also a process to obtain more economical, social and ecological productions (Kotu et al., Citation2022; Smith et al., Citation2017).
The Theory of Planned Behaviour (TPB) was proposed by Ajzen on the basis of the Theory of Reasoned Action (TRA), and was one of the most effective theories to explain the relationship among individual cognition, intention and behaviour (Ajzen, Citation1991; Li et al., Citation2021). In addition, according to cognitive economics (Bourgine, Citation2004), the bounded rationality of individuals manifests as the inability to maximize utility in many decisions, but can directly take action on behavioural goals with the help of other information. At the same time, when this cognitive system is distributed among social groups, it constitutes social distributive cognition, and group decision-making is also affected by individual cognitive differences. According to TPB, intention is a mediating variable that can explain and predict behaviour, but it does not necessarily translate into behaviour. This theory is widely applied in the exploration of social psychology problems (Beck & Ajzen, Citation1991; Duan & Jiang, Citation2008; Liao, Citation2021). Senger et al. (Citation2017) interviewed 101 farmers in the northwest region of the state of Rio Grande do Sul/Brazil about their intention to diversify their agricultural production, and the results showed that their attitude, subjective norm and perceived behavioural control are positively correlated with the intention. The result of Ataei et al. (Citation2021) demonstrated that in TPB, moral norms, attitude, and self-identity accounted for 52.2 percent of the variance in the intention to use green pesticides, which revealed that TPB could potentially predict intention. The traditional TPB does not mention the correlation between cognition and individual intention and behaviour, but cognition is a key factor in promoting the formation or change of willingness (Cao et al., Citation2022). A lot of literature showed that cognition has a direct impact on individual behaviour (Irwin et al., Citation2022; Mosavian et al., Citation2023), and clarifying the correlation between cognition, intention and behaviour is of great significance for exploring the logic behind individual behaviour (Zhao et al., Citation2018). Therefore, in order to promote and achieve SICLU in the region, this study innovatively constructs a theoretical analysis framework for the correlation between farmers’ cognition, intention, and behaviour based on traditional TPB and proposes theoretical hypotheses.
Firstly, make clear the conception of farmers’ cognition, intention and behaviour in the process of SICLU. The SICLU cognitions are farmers’ current knowledge, mainly including the cognition of the environmental impacts of chemical products such as fertilizers, pesticides and herbicides, the knowledge of land policies and relevant laws and so on. Intention stands for what farmers want to do to achieve SICLU in the future but are not doing presently, including the intention of reducing the usage of chemical inputs such as fertilizers and mulch films, transferring land and participating in green farming techniques training. Behaviour refers to the acts taken by farmers to achieve SICLU, involving adjustment of the input-output ratio and cultivated land management scale.
According to the content explained by the risk aversion theory (Freudenreich & Musshoff, Citation2022; Wu et al., Citation2023), cognitive economics (Bourgine, Citation2004) and social cognitive theory (Kreft et al., Citation2021; Yazdanpanah et al., Citation2015), without considering the complex relationship between individual cognition and decision-making and collective cognition and decision-making, only understanding the role of cognition from the perspective of individual farmers, the cognition of farmers who are the bounded rational economic individuals directly affects their behaviour in utilizing cultivated land and promotes or changes the formation, transformation, and implementation of their intentions. Based on existing concepts and awareness of interests, farmers would be driven by short-term maximization of benefits and minimization of risks (Carnegie et al., Citation2020), change the intention to reduce investment and learn new skills, and then comprehensively regulate the direction of cultivated land use by adjusting management scale, input-output ratio, and other behaviours (Anderson et al., Citation2020). The cognition of environmental protection among farmers is further reflected in the behaviour of adjusting the input-output ratio by influencing the intention to reduce implementation, or may also directly affect the input-output behaviour of farmers. The clearer the cognition of permanent basic cultivated land and land management laws are, the more farmers’ intention to transfer land and the behaviour to adjust the management scale will be influenced. The farmers getting more profound cognition of relevant laws and policies will be more likely to have the intention to transfer land, and finally lead to the behaviour of changing the scale of land management. Farmers’ cognition directly influences the adjustment of the management scale (). Based on the above analysis, two hypotheses are proposed ():
Hypothesis 1: There is a positive correlation between the cognition and intention of farmers for SICLU
Hypothesis 2: There is a positive correlation between the cognition and behavior of farmers for SICLU
Figure 1. Theoretical analysis framework of the association of farmers’ cognition, intention and behaviour towards SICLU.

Hypothesis 3: There is a correlation between the behavior and intention of farmers for SICLU
2.2. Data and study area
Qufu, the research area, in Shandong province that is a big agricultural province in China, belongs to the plain agricultural area where small-scale management is the typical agricultural model. This is a very common cultivated land use model in the northern plain area of China, which can represent Shandong Province as a whole and has reference value for most northern agricultural areas in China. Taking Shandong province as a case, exploring the SICLU can help ensure food security for the 1.4 billion population and achieve SICLU in China. Qufu city is located in the southwest of Shandong Province and the east-central part of Jining city, with a total area of 815 km2. There is an average annual precipitation of 698 mm and a warm temperate monsoon climate in Qufu, with dry spring and autumn, rainy summer, dry-cooling and less-snowy winter. The average annual runoff is 180.44 million m3, and the total annual available water resources are 230 million m3, indicating relatively sufficient water resources. The cultivated land area is 47.1 thousand hm2, accounting for 57.52% of the total land area of the city. The quality of cultivated land is mainly first-grade, and the soil is mostly composed of brown soil. Grain and rice, corn and wheat are the main food crops in Qufu. In 2019, the gross output value of agriculture in Qufu was 5.245 billion RMB, of which the cultivation output value is about 2.61 billion RMB, with 63,900 hectares of grain area and 436,300 tons of grain production (Lyu et al., Citation2022).
Based on the comprehensive development of rural agriculture and socio-economic conditions, three agricultural towns, namely Zhongshimenshan Town, Wucun Town, and Xizou Town, were selected as the research areas in Qufu. To ensure the randomness of the samples, we randomly selected four agricultural villages in each town, and randomly selected several farmers from each village to conduct a questionnaire survey. The respondents to the survey are general farmers, which can reflect the common situation of cultivated land use and farmers’ characteristics in the village. Each questionnaire takes an average of 1–1.5 h. Our questionnaire is not only designed for the study of SICLU, but also supports research on the livelihoods of farmers and households. Therefore, there is a situation that there is no cultivated land in the sample of farmers we surveyed, so it is necessary to exclude these samples and carry out subsequent research. We distributed a total of 380 questionnaires and 359 are valid. The final number of questionnaires that can be used for the study of SICLU is 333.
The data is sourced from a survey questionnaire conducted by the author’s research group in Qufu in 2020. The survey questionnaire includes 8 parts: basic information about farmers, family composition and livelihood situation, cultivated land transfer situation, specific information about cultivated land use activities, farmers’ environmental cognition and intention to reduce the use of chemical agents, cognition and intention of cultivated land scale management, cognition and intention of land transfer, and life expectations and social security. To ensure a complete agricultural growth cycle, the information on farmers’ land use activities is emphasized as the situation in 2019 during the interview process. The questionnaire involves a lot of content and serves as a data source for this study and other studies in the research group. This study only used a small part of it. The language used in the questionnaire and the interview process are both Chinese, and the questionnaire consists of approximately 15 pages of A4 paper. The survey was conducted in a combination of structured and semi-structured interviews. We obtained data by asking regarding the questionnaire content, and all farmers used a unified questionnaire. The interviewers are all doctoral and master’s students majoring in land resource management and possess professional knowledge related to cultivated land use. In addition, we conducted multiple trainings and pre-survey before conducting on-site research to ensure the consistency of the questioning content and the accuracy of data acquisition. After the questionnaire was completed, we browsed the whole content of it on the spot. For answers with significant deviations, we revised the results based on their answers to other questions or confirmed with the farmers again. Therefore, the reliability of the data on farmers’ cognition, intention and behaviour obtained through the questionnaire is relatively high.
2.3. Research methods
2.3.1. Super efficiency slack based model
Model establishment SICLU is not a simple matter of considering inputs and outputs, but classifying specifically outputs as desirable outputs and undesirable outputs. We defined that the outputs can promote the level of SICLU as desirable outputs, and the outputs inhibit the level of SICLU as undesirable outputs. The specific indicators selection has been shown in . Compared with the traditional DEM model, the Super Efficiency Slack Based Model (super-efficiency SBM) integrates inputs, desirable outputs and undesirable outputs, which takes negative impacts into account and has been used in various academic fields (Tone, Citation2001; Tone, Citation2002). Zhao et al. (Citation2022) evaluated China’s green economic growth levels based on the super-efficiency SBM model. They took the capital, labour, energy, land and water resources as input indicators, took regional GDP as desirable output, and industrial wastewater emissions, industrial surplus dioxide emissions and industrial soot emissions were seen as undesirable output indicators; Khan et al. (Citation2021) used it to carry out the efficiency evaluation of sustainable rural development in the Yellow River Basin of China, and simultaneously integrated desirable and undesirable indicators, like farmers’ livelihoods, the current state of rural development, environmental assets, energy use and environmental pollution. We attempted to apply the super-efficiency SBM model at the farmer scale and estimate the sustainable intensification degree of cultivated land use (SIDCLU) to characterize the level of SICLU, which is calculated as follows:
(1)
(1)
(2)
(2) where φ refers to the SIDCLU;
,
and
represent the indicator values of input, desirable output and undesirable output respectively; p,
and
represent the number of input, desirable output and undesirable output respectively;
,
and
refer to the slack variables of input, desirable output and undesirable output respectively;
refers to the weight vector; X, Yg and Yu refer to input, desirable output and undesirable output matrix respectively.
Table 1. Indicator selection of SIDCLU.
Variable selection Based on the definition of SICLU, we take input, desirable output and undesirable output as the primary indicators to select variables (), and measure the SIDCLU of 333 samples.
Input factors consist of labours, seeds, irrigations, machinery, fertilizers, herbicides, pesticides and mulch film, of which the labours input is the sum of each link of the farming process, with one farmer performing eight hours of agricultural work per day counting as one unit of labour input; seeds input refers to the cost of purchasing seeds; cultivated lands input is the total operating area. We use mu to describe the land area, and 1 ha is equal to 15 mu; because data on irrigation water consumption cannot be directly available, the cost of irrigation electricity consumption is used to represent irrigations input; machinery input for larger-scale farmers is the cost of machinery, while smallholders realizing mechanized farming through hiring use the cost of hiring as machinery input; the fertilizers, herbicide, pesticide and mulch films inputs are directly reflected by usage amounts.
The desired outputs are measured from three aspects: economy, society and environment. The economic output is reckoned as the cultivated land produce value. Regional surplus food production is the difference between the total cultivated land production and the household consumption, which is evaluated as the social output and reflects the role of guaranteeing regional and national food security. The environmental output involves the total carbon sink which is a dynamic process to fix CO2 in the air through photosynthesis (Chen et al., Citation2016; Tian & Zhang, Citation2013). According to the current situation of the study area, we only consider carbon sequestration of the cultivated land product, including wheat, corn, peanuts, vegetables and fruits. The measuring equation for carbon sink is:
(3)
(3) where Cc refers to the total carbon sink of the cultivated land, Cci represents the carbon sink of the ith crop, m is the number of crop species, and Ci, Yi, wi and Hi refer to carbon content rate, productions, water coefficient and economic coefficient (Ke et al., Citation2021) of the ith crop, respectively.
The undesirable outputs take carbon emissions and agricultural non-point source pollution into consideration. Carbon emission encompasses the usage of chemical fertilizers, pesticides, mulch films, agricultural diesel and irrigation in the process of cultivated land use, and is calculated as in Equation (4) (Ma et al., Citation2018). As for agricultural non-point source pollution, the calculating elements contain the residues of pesticides, chemical fertilizers and mulch films and the estimated equation is (5):
(4)
(4)
(5)
(5) Where Tc is the carbon emission, Wa is the agricultural non-point source pollution; Tj and αj are the carbon emission and emission coefficient of the jth emission source, respectively; Wi and βi are the consumptions and residual coefficient of ith non-point source pollutant, respectively. Carbon emission coefficient and residual coefficients of non-point source pollutants refer to existing studies (Zhang et al., Citation2016; Zhu, Citation2020).
2.3.2. Structural equation modeling
Structural Equation Modelling (SEM) is one of the models to explore the underlying bonds or traits that are implicit in psychological and social phenomena and hard to directly observe or investigate. The research subjects of SEM may be a theoretical idea, a research hypothesis, or an objective reality that cannot yet be precisely and directly measured by existing methods (Zhang, Citation1993). SEM has been widely used recently in multiple disciplines. Tiglao et al. (Citation2020) conducted a pilot survey on university students’ cognition of the quality of public transportation services in Manila to improve paratransit services using SEM; Castro-Jiménez et al. (Citation2020) carried out a study for 430 adolescents in Spain to clarify the association between adolescents’ health status and personal habits based on SEM. Zhou et al. (Citation2022) employed it to reveal farmers’ adaptation processes and mechanisms of heavy metal pollution in cultivated land relying on 278 mining farmers in Hubei Province from cognition, intention, characteristics and adaptive behaviour. For the sake of investigating the association of farmers’ cognition, intention and behaviour towards SICLU, we applied SEM to reckon farmers’ cognition, intention and behaviour and explore the relationship among them by virtue of path analysis among latent variables.
Model establishment Measurement model:
(6)
(6)
(7)
(7) Structure equation:
(8)
(8) where X and Y are the exogenous and endogenous explicit variables respectively; ξ is the exogenous latent variable; η is the endogenous latent variable; Aχ is the load matrix of X on ξ; Ay is the load matrix of Y on η; δ and ϵ are the measurement errors of X and Y respectively; B and T are the path coefficients; B is the relationship between endogenous latent variables; T is the degree of influence of exogenous latent variables on endogenous latent variables; β is the residual vector.
Variable description On account of the theoretical analysis framework on cognition, intention and behaviour of SICLU, three latent variables were set up. There are 6, 3 and 5 observed variables opted under the cognition latent variable, intention latent variable and behaviour latent variable, respectively ().
Table 2. Variable description and descriptive statistics.
Reliability and validity test The KMO and Bartlett’s test and Cronbach’s alpha coefficient are applied to check the validity and reliability of the data. shows that the KMO value is greater than 0.6 and Bartlett’s Test value is 0, indicating that the validity is good. As shown in , all samples’ Cronbach α is 0.634 and the Cronbach α values of each latent variable are greater than 0.600, meaning the data reliability is qualified.
Table 3. KMO and Bartlett test.
Table 4. Variable reliability index value.
3. Results
3.1. Assessment of SICLU
3.1.1. SICLU characteristics
The distribution of farmers’ SIDCLU is relatively even as a whole, with the majority located in the upper middle (). According to distribution characteristics, the SIDCLUs are divided into four intervals: (0, 0.3], (0.3, 0.6], (0.6, 0.9] and (0.9, 1.2], corresponding to four levels of unsustainable intensification (USICLU), low (LSICLU), medium (MSICLU) and high sustainable intensification of cultivated land use (HSICLU), respectively (). The number of farmers at the USICLU level is the lowest, with 37 households, accounting for 11.11% of the total. The peasant quantity at the LSICLU level is the largest, accounting for 34.83% of the total. The number at the MSICLU and HSICLU levels are both 90, respectively occupying 27.03% of the total. The samples at the MSICLU level and above are more than 50%, elucidating that the SI implementation potential of the majority of farmers is quite high ().
Figure 3. Input-output intensity characteristics.
Note: LC: Labour (day/person/mu), SE: Seed (RMB/mu), IR: Irrigation (RMB /mu), ME: Mechanical (RMB /mu), CF: Chemical fertilizer (kg/mu), HE: Herbicide (kg/mu), PE: Pesticides (kg/mu), FI: Plastic film (kg/mu), CV: Cultivated land value (RMB /mu), CY: Cultivated land productions (kg/mu), CW: Cultivated land surplus (kg/mu), CS: Carbon sink (kg/mu), CE: Carbon emission (kg/mu), AP: Agricultural non-point source pollution (kg/mu).

Table 5. Characteristics of SIDCLU under different management scales.
3.1.2. Influence exploration
Both management scale regulation and input-output ratio adjustment are behaviours affecting the SICLU level. Contrasting the behaviours characteristic with SICLU levels provides theoretical support for the subsequent investigation of the association among cognition, intention and behaviour, and the promotion of SICLU.
The mean of farmers’ SIDCLU are 0.617, 0.673, 0.756, 0.876 and 0.991 under the five management scales of 0–5 mu, 5–10 mu, 10–30 mu, 30–50 mu and 50–400 mu, respectively (). The management scale is positively correlated to SICLU level. Specifically, at 0–5 mu, samples at the LSICLU level are the most with 73 farmers and much more than other levels, accounting for 41.01% of the total. At 5–10 mu, the number of farmers at the LSICLU level is still the biggest with 33 farmers, accounting for 38.37%, and the proportion at the MSICLU level increases, accounting for 34.88%. At 10–30 mu, the maximum appears at the MSICLU level, accounting for 38.00%, and the secondary is at the HSICLU level, accounting for 32.00%. Both at 30–50 mu and 50–400 mu, the proportion of farmer quantity at the HSICLU level is highest, accounting for 50% and 84.62%, respectively.
The farmers’ input-output characteristics are different significantly under each level but they consist of the notion of SICLU (). Under the USICLU, there are the highest input intensity, the lowest desired output intensity, and the higher undesirable output intensity. The input intensity of labour, seeds, fertilizers, herbicides and mulch films are the highest, the desired output intensity of cultivated land productions, output value, surplus and carbon sink are the lowest, and the undesired output intensity like agricultural non-point source pollution are the highest. Under the LSICLU, the average input intensity of machinery, herbicides and pesticides are the highest, the desired output intensity of cultivated land productions, value, surplus and carbon sink are less, and the per mu undesired outputs are higher. At the MSICLU, input intensity is lower, desired output intensity is higher and undesirable output intensity is lower. The inputs intensity of labour, fertilizers and mulch films are the lowest, the other input intensity are relatively lower, the desired output intensity is higher, and all factors of undesired outputs intensity are the lowest. At the HSICLU, there are the lowest average input intensity, the highest desired output intensity and the lowest undesirable output intensity. The input intensity is the lowest, except labour, machinery, fertilizers and mulch film inputs, all the desired output intensities are the highest, and all undesired output intensities are relatively lower.
To sum up, there is a relevance between SICLU characteristics and farmers’ behaviours, such as management scale adjustment and input-output ratio modification.
3.2. The association among cognition, intention and behaviour
3.2.1. For all samples
Description of model fitness 333 samples are imported into the SEM for exploring the association among cognition, intention, and behaviour towards SICLU in Amos 22.0 software. After modified, all fitting index values of the model are within the ideal range and the overall fitness is satisfactory ().
Table 6. Fitting index value of SEM of 333 samples.
Results analysis The observed variables have a significant effect on latent variables at the 1% significance level in all three measurement models. The samples’ cognition significantly positively affects behaviour, and behaviour significantly negatively influences intention (). The path coefficient of cognition → intention is 0.170 and there is no significant relevance between cognition and intention, failing to prove hypothesis 1. The path coefficient of cognition → behaviour is 0.255, which implies a significant positive correlation at the 1% level and verifies hypothesis 2, that is, the higher the farmers’ cognition , the higher the possibility for them to carry out SI behaviour. The path coefficient of behaviour → intention is −0.211, which shows a significantly negative correlation at the 5% level and verifies hypothesis 3, that is, the more the SI behaviour is executed, the lower the intention of farmers to carry out SI behaviour in the future will be.
3.2.2. For different SICLU levels
Description of model fitness under different SICLU levels The samples’ cognition, intention and behaviour are analysed based on SEM at each SICLU level and the results are shown in . All the fitting index values have reached the ideal situation at any level, except GFI value which is 0.820 in the USICLU model and is lower than 0.9. In general, the model is still meaningful.
Table 7. Samples’ SEM fitting index value under each levels.
Results analysis The effect of farmers’ cognition on intention and behaviour is positively significant at the USICLU level while the influence of behaviour on intention is not significant, verifying hypotheses 1 and 2. The path coefficient of farmers’ cognition to behaviour is 0.715 with a significantly positive effect at the 5% level, and the path coefficient of farmers’ cognition to intention is 0.594 with a significantly positive effect at the 10% level. On the contrary, samples’ behaviour have a non-significant effect on the intention with a path coefficient of −0.389 ();
Table 8. Path coefficient of cognition, intention and behaviour under each levels.
At the LSICLU level, both the positive effect of farmers’ cognition on behaviour and the negative effect of behaviour on intention are significant, in accordance with hypotheses 2 and 3. The path coefficient of cognition to behaviour is 0.419, which means a significant positive effect at the 1% level. The influence of farmers’ behaviour on intention is significantly negative at the 10% level with a path coefficient of −0.137. However, the path coefficient of farmers’ cognition to intention is 0.167 and is not significant ().
Both the positive effect of farmers’ cognition on behaviour and the negative effect of behaviour on intention are significant under the MSICLU level, proving hypotheses 2 and 3. When the significance level is at 5%, the path coefficients of farmers’ cognition to behaviour (0.594) and behaviour on intention (−0.688) are significant. Yet the path coefficient of farmers’ cognition to intention is −0.014 with a non-significant negative effect (); Under the HSICLU level, only hypothesis 2 is confirmed and the positive effect of farmers’ cognition to behaviour is significant. The path coefficient of farmers’ cognition to behaviour is 0.397, holding a significantly positive impact under the 5% confidence level. Neither the path coefficient of farmers’ cognition to intention (−0.154) nor the path coefficient of behaviour to intention (−0.015) is significant ().
4. Discussion
4.1. The interaction between farmers’ cognition and behaviour towards SICLU
The result showed that the SICLU cognition for all samples has a significantly positive effect on behaviour, and the cognition at each level has a significant positive effect on behaviour, which is consistent with Liao (Citation2021) and Wei et al. (Citation2021) and substantiates the hypothesis 2 at the same time. This result indicates that as long as farmers understand the benefits that SICLU can bring, they will play their characteristics of rational economic man and positively transform cognition into their behaviour. The most significant impact of cognition on behaviour is among farmers in LSICLU. From , we found that the significance of cognition on behaviour gradually decreases with the level of SICLU increasing. Because the simples with higher SIDCLU have a larger operation scale, they would attach increasing importance to economic benefits. Compared to cognition, economic benefits may have a more significant impact on the behaviour of larger farmers, leading to the emergence of this result.
4.2. The interaction between farmers’ cognition and intention towards SICLU
The impact of cognition on intention is not significant for all samples and the positive effect of farmers’ cognition to intention is relatively weak at each level, which is contrary to the findings of Wei et al. (Citation2021) and Li et al. (Citation2020) and demolishes the hypothesis 1. We consider this phenomenon may closely coincide with farmers’ Economic Man attributes and their farming experience. Although farmers possess a high level of cognition for the negative environmental impacts of fertilizers and knowledge of land policies, their intention to reduce application and change farming practices for the sake of protecting the environment can be susceptibly driven by economic interests, which finally leads to the mismatch between intention and cognition. In addition, it was learned during the field research that huge quantities of farmers are more willing to rely on their own experience and are not inclined to convert their intrinsic farming practices even with the cognition of environmental pollution.
4.3. The interaction between farmers’ intention and behaviour towards SICLU
A significantly negative correlation between behaviour and intention occurs in the whole sample. There is no significant association between behaviour and intention at the USICLU and HSICLU levels, while under the LSICLU and MSICLU levels there is a significantly negative effect of behaviour to intention. The fact is that the contradiction between behaviour and intention is consistent with the findings of Xu et al. (Citation2021) and Sun et al. (Citation2020), and also verifies hypothesis 3. This manifests that the future intention to conduct SICLU practices is quite low for farmers who have implemented it. To explore the reason behind the phenomenon, we divide it into two cases to discuss in depth. In the first case, farmers with a small operation size, do not implement SI measures typically, possess lower SIDCLU, and most of them are elderly people who live alone and farm basically on experience. As a result of the small size of land and high economic costs of mechanized application, the majority of smallholders tend to farm through labour. And they set up the willingness to reduce chemical application on account of limitations by their own physiological conditions. However, after synthesizing a cluster of causes, like the drive of short-term economic benefits and farming experience, smallholders will not easily change their farming styles and implement reduction behaviour, which eventually leads to the contradiction between intention and behaviour. In another case, farmers with a large management scale launch SICLU behaviour and have higher SIDCLU. Since most arable land is acquired by transferring in, farmers only have short-term usufruct. As a result, farmers with large-scale operations do not show solicitude for the health of the soil and the sustainable use of land resources while they just consider how to achieve maximum economic returns in the shortest possible time. To raise crop production and economic outputs, the majority of large-scale farmers don’t have the intention to reduce the application of chemical inputs. Thus, the HSICLU level is only high under the unilateral domination of economic interests, but not optimal by the social, economic and ecological benefits.
4.4. Policy recommendations for enhancing SICLU
Due to the obvious benefits that SICLU will not bring to farmers in the short term, and recording to the risk aversion theory, social cognition theory and the individual attribute of economic man, they may be more willing to maintain their original farming habits, and the possibility of transitioning to SI mode is relatively low. The enhancing recommendations of SICLU need to start with policymakers and supervisors of policy implementation who can be regarded as a medium to achieve changes in farmers’ cognition, willingness and behaviour. Therefore, combining the research findings and discussions, the following suggestions are come up with (1) the farmers’ cognition at each level had a significantly positive influence on behaviour. To achieve the comprehensive promotion of SICLU, it is fundamental for the government or village collective to optimize the allocation of rural education resources, popularize the knowledge of environmental protection and land policy, and ensure the smooth promotion of SICLU starting from farmers’ cognition; (2) reducing the amount of farmers under USICLU is of great significance for improving the overall level. At this level where most peasants manage small-scale cultivated land, the positive influence of cognition on intention is significant. So, pointing at the peasants with small operation scale, carrying out targeted publicity on agricultural land transfer and green agriculture is considerably decisive through igniting their cognition to change behaviour; (3) farmers under the LSICLU and MSICLU levels always are driven by economic interests, which makes their behaviour negatively influence on intention. Agriculture sectors should offer or increase economic compensation, such as grain subsidies and allowance for the use of organic fertilizers, bio-pesticides and other environmentally friendly agents, to ensure farmers’ agricultural income, reduce economic concerns and achieve further improvement of SICLU. (4) The expansion of farmers’ cultivated land area can promote the formation of scale management, which can lead to a decrease in input per unit of cultivated land. This behaviour is positively correlated with the level of SICLU, so policymakers should propose financial incentive policies to promote land transfer for small farmers and reduce the amount of small farmers, which can increase the diversity of farmers’ livelihoods and raise household economic income.
4.5. Limitations and prospects
In the survey process, it is difficult to ensure the accuracy of farmers’ cognitive data. To avoid significant deviations as much as possible, we obtain data by directly asking according to the questionnaire. Cognition is expressed directly by farmers, and the reliability of the results is high. However, it is undeniable that farmers may be subject to external interference during the interview process, which may affect their responses, and this situation is inevitable. Therefore, in the subsequent research, we need to control the external environment and improve questioning methods and questionnaire proofreading methods to further ensure the accuracy of data. In addition, we will conduct follow-up surveys on farmers based on the correlation between their cognition and behaviour, and reversely verify their cognition by observing their behaviour.
Shandong province is a typical plain agricultural region in China. Taking it as the research area to explore the SICLU can help ensure food security for China’s 1.4 billion population and promote SICLU, which is of great significance for achieving global sustainable development goals. Due to the differences in socio-economic development, individual characteristics of farmers, natural conditions, customs and other factors, the generalizability of the research results at a global scale is greatly limited, but the research paradigm and approach are generalizable. The research results may trigger the thinking of relevant scholars and provide new ideas for future research about SI, cultivated land use or the application of TPB. For example, farmers who have already carried out SICLU practices have a smaller intention of SICLU in the future, which is contrary to theoretical analysis. This unusual result may have enlightening effects on relevant scholars. In addition, we will conduct further research on other agricultural regions in China, and Japan which has similar cultivated land use patterns with China, and in Belarus which has significant differences in cultivated land use patterns with China. Through conducting comparative studies domestically and internationally, we are looking forward to clarifying the differences in the interactions of farmers’ cognitive, intention and behaviour under different social, cultural, economic, and natural conditions, exploring the path to promote the SICLU, summarizing cultivated land use models, and prompting global sustainable development goals.
At present, this study only focuses on the correlation between farmers’ cognition, intention, and behaviour towards SICLU. What specific factors play an important role in the mutual feedback relationship among the three? How do the influencing factors affect the mutual feedback network? What is the degree of these factors? Clarifying these issues helps to identify the points for promoting SICLU from the perspective of farmers, and further realize the SICLU from the perspective of farmers’ cognition, willingness, and behaviour. This study has not yet addressed these issues, and we will continue to consider ways to translate these considerations into practice at the farmer level and achieve sustainable development at the economic, ecological, and social levels.
5. Conclusion
This study applied the concept of SI to the exploration of cultivated land use activities and employed the super-efficiency SBM that existent researches barely use to gauge the SIDCLU at the scale of farmers. In addition, the traditional TPB theory that only encapsulated the interaction between cognition and behaviour has been extended to explore the association among farmers’ cognition, intention and behaviour. Based on the new theory, this study originally proposed a novel theoretical analysis framework of SICLU. This study highlighted that the proportion of farmers at the USICLU, LSICLU, MSICLU and HSICLU levels are 27.03%, 27.03%, 34.83% and 11.11%, respectively. Overall, farmers’ SICLU level is quite well with the number of farmers at medium and above accounting for 54.06%. The mean of SIDCLU for farmers at five management scales of 0–5 mu, 5–10 mu, 10–30 mu, 30–50 mu and 50–400 mu are 0.617, 0.673, 0.756, 0.876 and 0.991, respectively, and there is a positive correlation between the management scale and SIDCLU. In addition, there is also a strong match between the input-output characteristics in the process of cultivated land utilization and SIDCLU. Specifically, the lower the input intensity is, the more the desired output intensity is, the less the undesirable output intensity is, and the higher the level of SI is.
For the association among cognition, intention and behaviour of SICLU in all samples, this paper showed that cognition has a significantly positive influence on behaviour and behaviour has a significantly negative influence on intention. Yet between cognition and intention, they don’t exist obvious relevance. At the USICLU level, farmers’ cognition significantly positively affects behaviour and intention; at the LSICLU and MSICLU levels, behaviour is affected positively by cognition but it negatively influences intention, markedly; at the HSICLU level, cognition significantly positively impacts behaviour. The non-significant relationship between cognition and intention is mainly led by economic interests and farmers’ farming experience, while the negative relationship between behaviour and intention is driven by factors such as operation scale and economic interests.
The content of this paper is in line with the connotation of China’s cultivated land protection strategy and global sustainable development goals. In practice, it helps to alleviate the global food crisis and promote the transformation of cultivated land use patterns. In theory, it can expand and enrich research on SI in China and all over the world. Additionally, we extend the traditional theory of planned behaviour (TPB) to the discussion of three aspects among cognition, willingness, and behaviour. Based on the connotation and significance of SICLU, we innovatively construct a research framework for the correlation mechanism among the three at the household level, extend the application of TPB, explore the social and psychological characteristics of farmers in SICLU, and analyse the implementation path of SICLU at a micro-scale.
Disclosure statement
No potential conflict of interest was reported by the author(s).
Data availability statement
Data will be made available on reasonable request.
Additional information
Funding
References
- Ajzen, I. (1991). The theory of planned behavior. Research in Nursing & Health, 14(2), 137–144. https://doi.org/10.1016/0749-5978(91)90020-T
- Anderson, R., Bayer, P. E., & Edwards, D. (2020). Climate change and the need for agricultural adaptation. Current Opinion in Plant Biology, 56, 197–202. https://doi.org/10.1016/j.pbi.2019.12.006
- Aravindakshan, S., Krupnik, T. J., Amjath-Babu, T. S., Speelman, S., Tur-Cardona, J., Tittonell, P., & Groot, J. C. J. (2021). Quantifying farmers’ preferences for cropping systems intensification: A choice experiment approach applied in coastal Bangladesh's risk prone farming systems. Agricultural Systems, 189, 103069. https://doi.org/10.1016/j.agsy.2021.103069
- Ataei, P., Gholamrezai, S., Movahedi, R., & Aliabadi, V. (2021). An analysis of farmers’ intention to use green pesticides: The application of the extended theory of planned behavior and health belief model. Journal of Rural Studies, 81, 374–384. https://doi.org/10.1016/j.jrurstud.2020.11.003
- Beck, L., & Ajzen, I. (1991). Predicting dishonest actions using the theory of planned behavior. Journal of Research in Personality, 25(3), 285–301. https://doi.org/10.1016/0092-6566(91)90021-H
- Bourgine, P. (2004). Cognitive economics: An interdisciplinary approach. Springer Science & Business Media.
- Burke, W. J., Snapp, S. S., Peter, B. G., & Jayne, T. S. (2022). Sustainable intensification in jeopardy: Transdisciplinary evidence from Malawi. Science of The Total Environment, 837, 155758. https://doi.org/10.1016/j.scitotenv.2022.155758
- Cao, H., Li, F., Zhao, K., Qian, C., & Xiang, T. (2022). From value perception to behavioural intention: Study of Chinese smallholders’ pro-environmental agricultural practices. Journal of Environmental Management, 315, 115179.
- Carnegie, M., Cornish, P. S., Htwe, K. K., & Htwe, N. N. (2020). Gender, decision-making and farm practice change: An action learning intervention in Myanmar. Journal of Rural Studies, 78, 503–515. https://doi.org/10.1016/j.jrurstud.2020.01.002
- Cassman, K. G., & Grassini, P. (2020). A global perspective on sustainable intensification research. Nature Sustainability, 3(4), 262–268. https://doi.org/10.1038/s41893-020-0507-8
- Castro-Jiménez, R. A., Pozo, F. J. F. D., Moral, G. J., & Fruet-Cardozo, J. V. (2020). Analysis of health habits, vices and interpersonal relationships of Spanish adolescents, using SEM statistical model. Heliyon, 6, e04699. https://doi.org/10.1016/j.heliyon.2020.e04699
- Chen, L., Hao, J. M., Wang, F., Yin, Y. Y., Gao, Y., Duan, W. K., & Yang, J. (2016). Carbon sequestration function of cultivated land use system based on the carbon cycle for the Huang-Huai-Hai Plain. Resources Science, 38, 1039–1053. In Chinese. https://doi.org/10.18402/resci.2016.06.04.
- Chen, Y. F., Miao, J. F., & Zhu, Z. T. (2021). Measuring green total factor productivity of China's agricultural sector: A three-stage SBM-DEA model with non-point source pollution and CO2 emissions. Journal of Cleaner Production, 318, 128543. https://doi.org/10.1016/j.jclepro.2021.128543
- Duan, W. T., & Jiang, G. R. (2008). A review of the theory of planned behavior. Advances in Psychological Science, 315–320.
- Elahi, E., Khalid, Z., & Zhang, Z. X. (2022). Understanding farmers’ intention and willingness to install renewable energy technology: A solution to reduce the environmental emissions of agriculture. Applied Energy, 309, 118459. https://doi.org/10.1016/j.apenergy.2021.118459
- FAO, IFAD, UNICEF, WFP, WHO. (2019). The state of food security and nutrition in the world 2019. Safeguarding against economic slowdowns and downturns. FAO. https://doi.org/10.18356/63e608ce-en.
- FAO, IFAD, UNICEF, WFP, WHO. (2022). The state of food security and nutrition in the world 2022: Repurposing food and agricultural policies to make healthy diets more affordable, the state of food security and nutrition in the world (SOFI). FAO, IFAD, UNICEF, WFP, WHO. https://doi.org/10.4060/cc0639en.
- FAOSTAT. (2018). Food and agriculture organization of the United Nations Rome, Italy. http://faostat.fao.org.
- Finucane, M. L., Alhakami, A., Slovic, P., & Johnson, S. M. (2000). The affect heuristic in judgments of risks and benefits. Journal of Behavioral Decision Making, 13(1), 1–17. https://doi.org/10.1002/(SICI)1099-0771(200001/03)13:1%3c1::AID-BDM333%3e3.0.CO;2-S
- Freudenreich, H., & Musshoff, O. (2022). Experience of losses and aversion to uncertainty - experimental evidence from farmers in Mexico. Ecological Economics, 195, 107379. https://doi.org/10.1016/j.ecolecon.2022.107379
- Galeana-Pizaña, J. M., Couturier, S., Figueroa, D., & Jiménez, A. D. (2021). Is rural food security primarily associated with smallholder agriculture or with commercial agriculture?: An approach to the case of Mexico using structural equation modeling. Agricultural Systems, 190, 103091. https://doi.org/10.1016/j.agsy.2021.103091
- Guo, Q. H., Li, H., Li, S. P., & Nan, L. (2021). Analysis on the response mechanism and determinants of farmer investment in rural waste governance. Resources and Environment in the Yangtze Basin, 30, 212–224. https://doi.org/10.11870/cjlyzyyhj202101020
- Ichinose, Y., Higuchi, H. H., Kubo, R., Nishigaki, T., Kilasara, M., Shinjo, H., & Funakawa, S. (2020). Adaptation of farmland management strategies to maintain livelihood by the Chagga people in the Kilimanjaro highlands. Agricultural Systems, 181, 102829. https://doi.org/10.1016/j.agsy.2020.102829
- IPCC. (2020). Climate change and land: An IPCC special report on climate change, desertification, land degradation, sustainable land management, food security, and greenhouse gas fluxes in terrestrial ecosystems.
- Irwin, R., Dhubháin, A., & Short, I. (2022). Irish dairy and drystock farmers’ attitudes and perceptions to planting trees and adopting agroforestry practices on their land. Environmental Challenges, 9, 100636. https://doi.org/10.1016/j.envc.2022.100636
- Jain, M., Solomon, D., Capnerhurst, H., Arnold, A., Elliott, A., Kinzer, A. T., Knauss, C., Peters, M., Rolf, B., Weil, A., & Weinstein, C. (2020). How much can sustainable intensification increase yields across South Asia? A systematic review of the evidence. Environmental Research Letters, 15(8), 083004. https://doi.org/10.1088/1748-9326/ab8b10
- Jalilov, S.-M., Rahman, W., Palash, S., Jahan, H., Mainuddin, M., & Ward, F. A. (2022). Exploring strategies to control the cost of food security: Evidence from Bangladesh. Agricultural Systems, 196, 103351. https://doi.org/10.1016/j.agsy.2021.103351
- Jules, P., Benton, T. G., Pervez, B. Z., Bharucha, Z. P., Dicks, L. V., Flora, C. B., Godfray, H. C. J., Goulson, D., Hartley, S., Lampkin, N., Morris, C., Pierzynski, G., Prasad, P. V. V., Reganold, J., Rockström, J., Smith, P., Thorne, P., & Wratten, S. (2018). Global assessment of agricultural system redesign for sustainable intensification. Nature Sustainability, 1(8), 441–446. https://doi.org/10.1038/s41893-018-0114-0
- Ke, N., Lu, X. H., Kuang, B., Han, J., et al. (2021). Regional differences and influencing factors of green and low-carbon utilization of cultivated land under the carbon neutrality target in China. China Land Science, 35, 67–76. https://doi.org/10.11994/zgtdkx.20210809.164152
- Khan, S. U., Cui, Y., Khan, A. A., Ali, M. A. S., Khan, A., Xia, X., Liu, G., & Zhao, M. (2021). Tracking sustainable development efficiency with human-environmental system relationship: An application of DPSIR and super efficiency SBM model. Science of The Total Environment, 783, 146959. https://doi.org/10.1016/j.scitotenv.2021.146959
- Kotu, B. H., Oyinbo, O., Hoeschle-Zeledon, I., Nurudeen, A. R., Kizito, F., & Boyubie, B. (2022). Smallholder farmers’ preferences for sustainable intensification attributes in maize production: Evidence from Ghana. World Development, 152, 105789. https://doi.org/10.1016/j.worlddev.2021.105789
- Kreft, C., Huber, R., Wuepper, D., & Finger, R. (2021). The role of non-cognitive skills in farmers' adoption of climate change mitigation measures. Ecological Economics, 189, 107169. https://doi.org/10.1016/j.ecolecon.2021.107169
- Kurgata, B. K., Ngenohc, E., Bett, H. K., Stöber, S., Mwonga, S., Lotze-Campen, H., & Rosenstock, T. S. (2018). Drivers of sustainable intensification in Kenyan rural and peri-urban vegetable production. International Journal of Agricultural Sustainability, 16(4-5), 385–398. https://doi.org/10.1080/14735903.2018.1499842
- Li, M. Y., Wang, J. J., & Zhao, P. J. (2020). Factors affecting the willingness of agricultural green production from the perspective of farmers’ perceptions. Science of The Total Environment, 738, 140289. https://doi.org/10.1016/j.scitotenv.2020.140289
- Li, Y., Wang, Y., & Luo, J. C. (2021). Farmland cognition, farmland certification and farmland transfer—Based on the survey of farmers from six provincial regions of western China. Research on Economics and Management, 42, 120–132. https://doi.org/10.13502/j.cnki.issn1000-7636.2021.01.008
- Liao, B. (2021). Family livelihood capital, the recognition and the behavior of paying for the governance of rural living environment for farmers: Taking 873 farmers in Jiangxi Province for example. Journal of Agro-Forestry Economics and Management, 20, 598–609. https://doi.org/10.16195/j.cnki.cn36-1328/f.2021.05.62
- Loos, J., Abson, D. J., Chappell, M. J., Hanspach, J., Mikulcak, F., Tichit, M., & Fischer, J. (2014). Putting meaning back into “sustainable intensification”. Frontiers in Ecology and the Environment, 12(6), 356–361. https://doi.org/10.1890/130157
- Lu, Y. L., Chadwick, D., Norse, D., Powlson, D., & Shi, W. (2015). Sustainable intensification of China's agriculture: The key role of nutrient management and climate change mitigation and adaptation. Agriculture, Ecosystems & Environment, 209, 1–4. https://doi.org/10.1016/j.agee.2015.05.012
- Lyu, X., Niu, S. D., Gu, G. Z., & Peng, W.-l. (2020). Conceptual cognition and research framework on sustainable intensification of cultivated land use in China from the perspective of the ‘New agriculture, countryside and peasants’. Journal of Natural Resources, 35(9), 2029–2043. https://doi.org/10.31497/zrzyxb.20200901
- Lyu, X., Peng, W. L., Niu, S. D., Qu, Y., & Xin, Z. (2022). Evaluation of sustainable intensification of cultivated land use according to farming households’ livelihood types. Ecological Indicators, 138, 108848. https://doi.org/10.1016/j.ecolind.2022.108848
- Lyu, X., Peng, W. L., Yu, W., Xin, Z., Niu, S., & Qu, Y. (2021). Sustainable intensification to coordinate agricultural efficiency and environmental protection: A systematic review based on metrological visualization. Journal of Land Use Science, 16(3), 313–338. https://doi.org/10.1080/1747423X.2021.1922524
- Ma, X. J., Dong, B. Y., Yu, Y. B., Wang, C. X., & Yang, Q. (2018). Measurement of carbon emissions from energy consumption in three Northeastern provinces and its driving factors. China Environmental Science, 38, 3170–3179.
- Maleksaeidi, H., & Keshavarz, M. (2019). What influences farmers' intentions to conserve on-farm biodiversity? An application of the theory of planned behavior in fars province, Iran. Global Ecology and Conservation, 20, e00698. https://doi.org/10.1016/j.gecco.2019.e00698
- McLaughlin, A., & Mineau, P. (1995). The impact of agricultural practices on biodiversity. Agriculture, Ecosystems & Environment, 55(3), 201–212. https://doi.org/10.1016/0167-8809(95)00609-V
- Mosavian, S. H., Rostami, F., & Tatar, M. (2023). Modeling farmers’ intention to water protection behavior: A new extended version of the protection motivation theory. Journal of Environmental Psychology, 90, 102036. https://doi.org/10.1016/j.jenvp.2023.102036
- Mullan, S., Wiltshire, P., Cross, K., Main, D. C., Still, K., Crawley, M., & Dowsey, A. W. (2022). National farm assurance scheme demonstrates welfare outcome improvements for sustainable intensification of dairy production. International Journal of Agricultural Sustainability, 20(4), 558–575. https://doi.org/10.1080/14735903.2021.1957348
- Niu, S. D., Lyu, X., & Gu, G. Z. (2022). A new framework of green transition of cultivated land-use for the coordination among the water- land-food-carbon nexus in China. Land, 11(6), 933. https://doi.org/10.3390/land11060933
- Niu, S. D., Lyu, X., Gu, G. Z., Zhou, X., & Peng, W. (2021). Sustainable intensification of cultivated land use and its influencing factors at the farming household scale: A case study of Shandong Province, China. Chinese Geographical Science, 31(1), 109–125. https://doi.org/10.1007/s11769-021-1178-8
- Niu, S. D., Lyu, X., & Shi, Y. Y. (2018). Spatial-temporal pattern of sustainable intensification of agricultural land-use in Shandong Province, China. Chinese Journal of Applied Ecology, 29, 607–616. https://doi.org/10.13287/j.1001-9332.201802.020
- Peng, W. L., Lyu, X., Xin, Z. F., & Niu, S. D. (2020). International experience of sustainable intensification and its implications for the protection of cultivated land in China. China Land Science, 34, 18–25. https://doi.org/10.11994/zgtdkx.20200313.171447
- Petersen, B., & Snapp, S. (2015). What is sustainable intensification? Views from experts. Land Use Policy, 46, 1–10. https://doi.org/10.1016/j.landusepol.2015.02.002
- Rahman, K. M. A., & Zhang, D. F. (2018). Effects of fertilizer broadcasting on the excessive use of inorganic fertilizers and environmental sustainability. Sustainability, 10(3), 759. https://doi.org/10.1016/j.landusepol.2015.02.002
- Savari, M., & Gharechaee, H. (2020). Application of the extended theory of planned behavior to predict Iranian farmers’ intention for safe use of chemical fertilizers. Journal of Cleaner Production, 263, 121512. https://doi.org/10.1016/j.jclepro.2020.121512
- Senger, I., Borges, J. A. R., & Machado, J. A. D. (2017). Using the theory of planned behavior to understand the intention of small farmers in diversifying their agricultural production. Journal of Rural Studies, 49, 32–40. https://doi.org/10.1016/j.jrurstud.2016.10.006
- Siebrecht, N., & Schmid, H. (2020). Implementation of the concept of sustainable intensification to a real farm – Was its development over 17 years a sustainable intensification? International Journal of Agricultural Sustainability, 18(2), 151–171. https://doi.org/10.1080/14735903.2020.1743073
- Smith, A., Snapp, S., Chikowo, R., Thorne, P., Bekunda, M., & Glover, J. (2017). Measuring sustainable intensification in smallholder agroecosystems: A review. Global Food Security, 12, 127–138. https://doi.org/10.1016/j.gfs.2016.11.002
- Song, H. F., & Xin, L. J. (2021). Differentiation characteristics and influencing factors of cultivated land use intensity in China. Transactions of the Chinese Society of Agricultural Engineering, 37, 212–222 + 303. https://doi.org/10.11975/j.issn.1002-6819.2021.16.027
- Sun, Q. L., Fang, K. X., & Liu, T. P. (2020). Impact of social norms and public supervision on the willingness and behavior of farming households to participate in rural living environment improvement: Empirical analysis based on generalized continuous ratio model. Resources Science, 42, 2354–2369. https://doi.org/10.18402/resci.2020.12.08
- Tama, R. A. Z., Ying, L., Yu, M., & Hoque, H. H. (2021). Assessing farmers’ intention towards conservation agriculture by using the extended theory of planned behavior. Journal of Environmental Management, 280, 111654. https://doi.org/10.1016/j.jenvman.2020.111654
- Tian, Y., & Zhang, J. B. (2013). Regional differentiation research on net carbon effect of agricultural production in China. Journal of Natural Resources, 28, 1298–1309. https://doi.org/10.11849/zrzyxb.2013.08.003
- Tiglao, N. C. C., De Veyra, J. M., Tolentino, N. J. Y., & Tacderas, M. A. Y. (2020). The perception of service quality among paratransit users in metro Manila using structural equations modelling (SEM) approach. Research in Transportation Economics, 83, 100955. https://doi.org/10.1016/j.retrec.2020.100955
- Tone, K. (2001). A slacks-based measure of efficiency in data envelopment analysis. European Journal of Operational Research, 130(3), 498–509. https://doi.org/10.1016/S0377-2217(99)00407-5
- Tone, K. (2002). A slacks-based measure of super-efficiency in data envelopment analysis. European Journal of Operational Research, 143(1), 32–41. https://doi.org/10.1016/S0377-2217(01)00324-1
- UN. (2015). Transforming our world: the 2030 agenda for sustainable development. General Assembly 70th Session. https://sustainabledevelopment.un.org/post2015/transformingourworld/publication.
- Wang, H. Z., Ren, H., Zhang, L. H., Zhao, Y., Liu, Y., He, Q., Li, G., Han, K., Zhang, J., Zhao, B., Ren, B., & Liu, P. (2023). A sustainable approach to narrowing the summer maize yield gap experienced by smallholders in the North China Plain. Agricultural Systems, 204, 103541. https://doi.org/10.1016/j.agsy.2022.103541
- Wang, Y. D., Liang, J. P., Yang, J., Ma, X., Li, X., Wu, J., Yang, G., Ren, G., & Feng, Y. (2019). Analysis of the environmental behavior of farmers for non-point source pollution control and management: An integration of the theory of planned behavior and the protection motivation theory. Journal of Environmental Management, 237, 15–23. https://doi.org/10.1016/j.jenvman.2019.02.070
- Wei, X., Khachatryan, H., & Zhu, H. G. (2021). Poyang lake wetlands restoration in China: An analysis of farmers’ perceptions and willingness to participate. Journal of Cleaner Production, 284, 125001. https://doi.org/10.1016/j.jclepro.2020.125001
- Wu, H. X., Hao, H. T., Lei, H. Z., Ge, Y., Shi, H., & Song, Y. (2021). Farm size, risk aversion and overuse of fertilizer: The heterogeneity of large-scale and small-scale wheat farmers in Northern China. Land, 10(2), 111. https://doi.org/10.3390/land10020111
- Wu, H. X., Song, Y., Yu, L. S., & Ge, Y. (2023). Uncertainty aversion and farmers’ innovative seed adoption: Evidence from a field experiment in rural China. Journal of Integrative Agriculture, 22(6), 1928–1944. https://doi.org/10.1016/j.jia.2023.04.004
- Wu, Y. Z., & Xu, Z. Y. (2019). Study on the transformation of cropland protection under the background of rehabilitation system. Resources Science, 41, 9–22. https://doi.org/10.18402/resci.2019.01.02
- Xiao, L., Liu, J. Y., & Ge, J. W. (2021). Dynamic game in agriculture and industry cross-sectoral water pollution governance in developing countries. Agricultural Water Management, 243, 106417. https://doi.org/10.1016/j.agwat.2020.106417
- Xie, H. L., Huang, Y. Q., Choi, Y., & Shi, J. (2021). Evaluating the sustainable intensification of cultivated land use based on emergy analysis. Technological Forecasting and Social Change, 165, 120449. https://doi.org/10.1016/j.techfore.2020.120449
- Xu, J. B., Wang, Y., & Li, C. X. (2021). Reasons behind the paradox between farmers’ willingness and their behaviors of applying organic fertilizer: A case study of Heilongjiang Province. Research of Agricultural Modernization, 42, 474–485. https://doi.org/10.13872/j.1000-0275.2021.0062
- Xu, Z., He, P., Yin, X. Y., Huang, Q., Ding, W., Xu, X., & Struik, P. C. (2023). Can the advisory system nutrient expert® balance productivity, profitability and sustainability for rice production systems in China? Agricultural Systems, 205, 103575. https://doi.org/10.1016/j.agsy.2022.103575
- Yazdanpanah, M., Feyzabad, F. R., Forouzani, M., Mohammadzadeh A, S., & Burton, R. J. F. (2015). Predicting farmers’ water conservation goals and behavior in Iran: A test of social cognitive theory. Land Use Policy, 47, 401–407. https://doi.org/10.1016/j.landusepol.2015.04.022
- Zhang, D., Hu, W. L., Liu, H. B., Du, L. F., Xu, Y, Cheng, Z. H., Sun, S. Y., & Wang, H. Y. (2016). Characteristics of residual mulching film and residual coefficient of typical crops in North China. Transactions of the Chinese Society of Agricultural Engineering, 32, 1–5. https://doi.org/10.11975/j.issn.1002-6819.2016.03.001
- Zhang, J. P. (1993). Structural equation modelling: A new kind of statistical and research method. Acta Psychologica Sinica, 25, 93–10.
- Zhang, J. Q., Yan, T. W., & Zhang, T. C. (2021). Analysis on the response mechanism and decision-making factors of farmers’ investment in rural waste treatment. Resources and Environment in the Yangtze Basin, 30, 2521–2532. https://doi.org/10.11870/cjlyzyyhj202110020
- Zhao, L., Wu, D. T., Wang, Z. H., Qu, L. P., & Yu, W. (2018). Spatio-temporal pattern and influencing factors of the allocation of rural basic education resources in China. Economic Geography, 38, 39–49. https://doi.org/10.15957/j.cnki.jjdl.2018.11.005
- Zhao, X., Ma, X. W., & Shang, Y. P. (2022). Green economic growth and its inherent driving factors in Chinese cities: Based on the Metafrontier-global-SBM super-efficiency DEA model. Gondwana Research, 106, 315–328. https://doi.org/10.1016/j.gr.2022.01.013
- Zhou, H., Chen, Y., Liu, Y. Z., Wang, Q., & Liang, Y. (2022). Farmers’ adaptation to heavy metal pollution in farmland in mining areas: The effects of farmers’ perceptions, knowledge and characteristics. Journal of Cleaner Production, 365, 132678. https://doi.org/10.1016/j.jclepro.2022.132678
- Zhu, L. Y. (2020). Study on annual yield and resource utilization of wheat and maize under different planting systems and sub-soil plastic film mulching in Hebei Plain. Baoding: Master Dissertation of Hebei Agricultural University, 56. https://doi.org/10.27109/d.cnki.ghbnu.2020.000196.
- Zuo, L., Zhang, Z., Carlson, K. M., MacDonald, G. K., Brauman, K. A., Liu, Y., Zhang, W., Zhang, H., Wu, W., Zhao, X., Wang, X., Liu, B., Yi, L., Wen, Q., Liu, F., Xu, J., Hu, S., Sun, F., Gerber, J. S., & West, P. C. (2018). Progress towards sustainable intensification in China challenged by land-use change. Nature Sustainability, 1(6), 304–313. https://doi.org/10.1038/s41893-018-0076-2