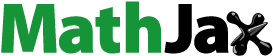
ABSTRACT
The slow pace of the adoption of the latest innovations in agricultural technology innovations impedes sustainable farming practices and sustainable agriculture in developing countries. This study investigates the potential reasons that stop smallholder farming households from adopting genetically engineered seeds (Bt cotton and improved wheat seeds), herbicides resulting from cutting-edge technologies, and no-tillage farming. Utilizing original farm household-level data from 275 smallholder farming households in Pakistan, we employ multivariate probit models. The results show that the adoption of innovative technologies is not an isolated, separate process but a concoction of available technologies and cropping patterns. The estimates of the multivariate probit models show that farm machinery index, off-farm income sources, and farmers’ education facilitate technology adoption. The observations and estimates indicate that a lack of agricultural extension service contacts is present, which slows down the farmers’ adoption of agricultural technological inputs. Therefore, promoting the role of agricultural extension services (qualitatively and quantitatively) is likely to play a role in multiple technology adoption. Furthermore, the significant effect of off-farm employment shows that the lack of financial resources is another factor slowing the adoption of innovative technologies, which depends on liquidity for necessary expenditures.
1. Introduction
Innovation has been an integral part of agriculture since its creation and innovation in agriculture has played a crucial role in economic development globally (Alston & Pardey, Citation2021). As it is defined by Edmund Phelps, a Nobel Prize winner in economics, innovation is any ‘new method or new product that becomes a new practice somewhere in the world’ (Ridley, Citation2020). Public policies are important for the creation of new innovations in agriculture and their adoption, which seems to fail in many cases (Alston & Pardey, Citation2021). In spite of these failures, innovation can accelerate the transition toward sustainable food systems (Herrero et al., Citation2020). The innovation's adoption does not inclusively consist of the adoption of new materials or methods. It could be the adoption of new institutions, such as the water market (Jaghdani & Brümmer, Citation2016; Wheeler et al., Citation2009) or the adoption of new agricultural systems such as pesticide-free practices (Möhring & Finger, Citation2022).
The acceleration in the diffusion and adoption of new innovations in agricultural technologies increases farm productivity and can enhance sustainable development globally. However, in developing countries, the low acceleration of the adoption of the latest innovations in agricultural technologies impedes sustainable farming practices and sustainable agricultural development in general. Hence, the low adoption levels hamper overall economic growth and agricultural transformation. Despite dogged perseverance in fostering rural development in developing countries in recent years, low adoption levels still persist. The issue of lagged adoption of agricultural innovation has been of interest to economists for a long time (Alston & Pardey, Citation2021), and there are different methods available that try to model the adoption or diffusion of new innovations in agriculture (de Oca Munguia et al., Citation2021) and different theories in economics to explain them (Gallardo & Sauer, Citation2018). The recent empirical evidence shows the significant snags that hamper low adoption levels. For example, findings from Mendola (Citation2007), Kassie et al. (Citation2011), Simtowe et al. (Citation2016) and Bilal and Barkmann (Citation2019) all display that there is less contact with extension service providers, that farmers’ associations are lacking membership, that there’s fewer means of generating off-farm incomes, and greater distances between farms from primary agricultural input/output markets. These issues, among others, hamper technology adoption. Furthermore, the role of new analogue or digital technologies cannot be neglected. For instance, Silvestri et al. (Citation2021) assessed the impact of radio and short message service (SMS) in expanding smallholder participation in legume-based sustainable agricultural intensification (SAI) practices and technologies in Tanzania. The results show that both awareness and adoption are increased if SMS supports radio campaigns. Nevertheless, radio alone was found to be the most cost-effective approach. Furthermore, Zhong et al. (Citation2023) have shown that internet usage can significantly promote the joint adoption of sustainable agricultural practices (SAPs).
A growing body of literature indicates the fundamental role of technology adoption in boosting farm productivity and sustainable development at large. For example, the adoption of crop protection products from multinational brands (CMBs); modern seed technologies; Bt (Bacillus thuringiensis) cotton; integrated pest management (IPM) techniques; soil and water conversation methods; natural resource management technologies; and no-tillage farming show a positive association with farm productivity and sustainable development at large (Abdulai & Huffman, Citation2014; Asfaw et al., Citation2012; Barbera et al., Citation2012; Bilal et al., Citation2022a; Noltze et al., Citation2013).
Some meta-studies have summarized the convergences and divergences in the literature on the adoption of agricultural technologies. For instance, in a meta-analysis of 96 adoption studies of farm-level soil conservation practices in developed countries, Wauters and Mathijs (Citation2014) found inconsistencies in the effectiveness of the classic factors that influence the adoption of innovation. In their meta-analysis, Ruzzante et al. (Citation2021) have concluded that there are few universal effective factors of agricultural adoption. They found education, land size, access to credit, land tenure, contact with extension agents, and membership in farmers’ organizations to all positively influence the adoption of most of the technological innovations under study.
Dessart et al. (Citation2019) reviewed the studies on the behavioural factors that influence farmers’ decisions to adopt environmentally sustainable practices for the period after 1999. They address how extraversion, openness to new experiences, risk-seeking, morals and environmental concern, as well as lifestyle farming objectives are associated with a higher adoption of sustainable practices. In contrast, being resistant to change and moved by economic objectives increases the reluctancy of farmers to adopt sustainable practices. They conclude that farmers’ behaviours can be indirectly segmented according to sociodemographic and geographic characteristics can lead to necessary policies. In their review of the publications between 2000 and 2021 on the adoption of sustainable agriculture innovations, Rosário et al. (Citation2022) found that the use of behavioural models that select sociopsychological constructs has increased. They suggest building a trans- and multidisciplinary model for future research.
In another review of the adoption studies on developing countries’ agriculture, Takahashi et al. (Citation2020) have concluded that there are profitable technologies that are not diffused widely due to weak extension systems. Furthermore, they found there to be a lack of analysis on the profitability of new innovations within adoption studies to date, and also noted how many diffusion studies focus only on a single technology, such as an improved seed variety, improved planting method, or the use of compost. In two studies, de Oca Munguia et al. (Citation2021) and de Oca Munguia and Llewellyn (Citation2020) reviewed different conceptual frameworks surrounding adoption, showing how a diversity of prospective exists among adoption studies. de Oca Munguia and Llewellyn (Citation2020) specifically address how there is a clear tendency for variables related to the technologies or practices to be underrepresented in adoption studies compared to behavioural or socioeconomic variables. Furthermore, they argue that the topic of multiple technology adoption can be considered a gap in the adoption research literature both conceptually and practically (de Oca Munguia et al., Citation2021).
Most previous studies discuss the determinants of single-input adoption or sustainable farming practices (e.g. CMBs, modern seed technologies, Bt cotton, IPM, soil and water conversation methods, natural resource management technologies and no-tillage farming). However, the empirical evidence may be tainted due to methodological challenges related to single-input adoption decisions, endogeneity and farmers’ adoption decisions for multiple modern inputs, which may produce biased estimates (Abay et al., Citation2018). The introduction of SAI has increased the importance of multiple technology adoption. SAI refers to a combination of agricultural processes that maintains a level of production or increases it while environmental outcomes are also enhanced. For example, farmers who adopt different SAI practices could increase their yield per hectare by using new seed varieties and employing different agroeconomic-agroecological practices (Pretty, Citation2018).
Irrespective of the empirical evidence regarding the determinants of single-input adoption decisions of agricultural technology products in developing countries, adopting multiple modern inputs remains an empirical puzzle. The adoption of multiple modern inputs presumably complements each other and favours sustainable development. For example, multinational crop protection products and mechanized farming provide higher farm revenues (Bilal et al., Citation2022a). Similarly, improved seed varieties complement chemical fertilizers and provide better yields (Nyangena & Juma, Citation2014). Likewise, an efficient soil conservation method, such as no-tillage, helps to avoid ploughing/aggressive mechanized farming (Barbera et al., Citation2012). Hence, Brookes et al. (Citation2017) and Danne et al. (Citation2019) highlight the pros and cons of the joint adoption of no-tillage systems with modern herbicides (e.g. glyphosate formulations). Tadjiev et al. (Citation2023) have found that no-tillage farming practices among smallholders in Kyrgyzstan to be accompanied with higher herbicide costs and higher labour intensity. Considering the adoption of other technologies in SAI practices, Kotu et al. (Citation2017) have studied multiple technology adoption of cereal-legume intercropping, cereal-legume rotation, organic fertilizers, soil and water conservation practices, chemical fertilizers and improved seeds in Ghana. They found that access to markets, capital and information/knowledge would increase the multiple adoptions in the context of SAI. Furthermore, a higher technology adoption is accompanied by higher productivity. In another study on vegetable production in Tanzania, Ochieng et al. (Citation2022) found that improved varieties, fertilizers and pest management practices are complementarities, as manure and mineral fertilizers show trade-offs between them.
Adopting genetically engineered seeds such as Bt cotton and high-quality wheat seeds, herbicides resulting from cutting-edge technology, and no-tillage farming are regarded as sustainable agriculture innovations (Lee, Citation2005). Abay et al. (Citation2018) studied input complementarity between chemical fertilizer and improved seeds, while Tadjiev et al. (Citation2023) observed how no-tillage adoption supports higher herbicide costs. Considering the nuances deduced from Pretty (Citation2018), the relevant stakeholders pay more attention to the adaptation process of the SAI model as the introduction of SAI has increased the importance of multiple technology adoption. Based on a careful review of the existing literature, we noticed that the determinants affecting the adaptation processes of multiple sustainable agricultural innovations have not been widely explored. Hence, it is of high interest to contribute to the present knowledge on this issue. Furthermore, we have noticed in particular how understanding an explicit decision to adopt Bt cotton, high-quality wheat seeds, herbicides resulting from cutting-edge technology, and no-tillage farming is lacking. Therefore, this study envisages the complementary (positive correlation) or trade-offs (negative correlation) between the adoption of multiple sustainable agricultural innovations. We intend to contribute to the existing literature by providing empirical evidence regarding the most promising determinants of the adoption of complementary inputs/farming practices by smallholders with a particular focus on policy implications. In this study, we mainly investigate the potential reasons stopping smallholder farming households from adopting genetically engineered seeds (Bt cotton and high-quality wheat seeds), herbicides resulting from cutting-edge technology, and no-tillage farming in the Punjab Province of Pakistan.
The present study is confined exclusively to smallholders because the significant features of South Asian agriculture are smallholders and their smallholdings (Wiggins & Keats, Citation2013). The farm size in major agricultural countries of South Asia ranges from 0.5 to 3.0 ha on average. The average farm size is 0.5 ha in Bangladesh, 0.8 ha for Nepal and Sri Lanka and 1.4 ha in India. The average farm size in Pakistan is relatively higher than in neighbouring countries due to a high concentration of significant land holdings (Thapa & Gaiha, Citation2014). According to the agricultural census 2010 in PakistanFootnote1, farms with an area of less than 5 acres (farm size ≤ 2.02 ha) constitute small farms, farms with an area more than 5 acres and less than 25 acres (2.02 ha < farm size ≤10.11 ha) comprised the category of average farms, and the large farms’ size is 25 acres or more (farm size > 10.11 ha). The total cultivated area of the Punjab Province is 10,941,000 ha, which are unevenly distributed among farmers. Only 23% (2,516,430 ha) of the area is cultivated by small farms and farmers operating these farms are thus considered smallholders, while 37% (4,048,170 ha) of the cultivated area is operated by average farmers, and 40% (4,376,400 ha) by large farmers (considering the definition of Pakistan Bureau of Statistics). Most noteworthy, the average and large farms account for 77% of the total cultivated area (Government of Punjab, Citation2019). In this study, we have utilized primary data from 275 smallholder farming households in Pakistan, which was acquired by a field survey during 2017. In order to analyze our research questions, we employed a multivariate probit model to test the possible complementary or trade-offs among different technology adoption practices.
This paper is structured as follows: an overview of smallholder constraints in the adoption of multiple agricultural innovations, extension institutions and policies in Pakistan is given in section 2 while the following section 3 details the study site and sampling techniques. The theoretical framework is provided in section 4 and the empirical strategy in section 5, while section 6 covers the results and section 7 contains a discussion. Section 8 concludes this analysis with policy implications and prospects for future research in developing countries.
2. Smallholder constraints in the adoption of multiple agricultural innovations in Pakistan and extension policies
The adoption of multiple innovations in Pakistan is the focus of this study. Pakistani farmers face substantial problems in terms of socio-economic constraints that affect their adoption behaviour. Among the plausible reasons are that they have larger household sizes, small landholding sizes, high illiteracy rates, a lack of farm machinery, less interest in technology adoption, a lack of communication between farmers, and less contact with agricultural extension services (Jalal-Ud-Din, Citation2011). Initial adoption of low-quality inputs is widespread in Pakistan, and it retards agricultural development (Ahmad Khan et al., Citation2013; Hashmi, Citation2016). The aggressive and inappropriate use of low-quality herbicides can endanger ecosystem services, including soil microorganisms, birds and other non-targeted organisms (Aktar et al., Citation2009). Smallholdings in the agricultural heartland of Pakistan are approximately 60% of the total agricultural holdings. The smallholders operating these farms use less multiple agricultural innovations due to socio-economic constraints (Malik et al., Citation2016; Thapa & Gaiha, Citation2014). Aslam (Citation2016) highlights how less adoption of technological innovations is the major constraint for Pakistan's lower average yields compared to average yields obtained internationally. Access to agricultural credit supports the adoption of technological innovations; however, deprived socio-economic factors play a key role in farmers’ lesser access to agricultural credit (Bilal et al., Citation2015). In addition, women farmers in Pakistan face more severe problems in obtaining agricultural credit (Muhammad et al., Citation2020).
Since 2002 Pakistani farmers have been cultivating genetically modified (e.g. Bt cotton), pest-resistant cultivars of cotton seeds (Abdullah, Citation2010; James, Citation2012). Also, in the cotton-wheat zone of Punjab in Pakistan, there exists a diversity of wheat seed of varying quality, and high-quality wheat seeds such as sehar-06 and Inqilab-91 varieties that are the most popular varieties in Punjab (Battese et al., Citation2017). Likewise, farmers adopt herbicides to resist weed growth (Ali et al., Citation2013); however, the diversity of herbicides of varying quality goes from cutting-edge technology imported from OECDFootnote2 listed countries based upon their successful registration in the source countries to locally manufactured sub-standard herbicides (Bilal & Barkmann, Citation2019). The sub-standard herbicides may have low-quality formulations, unpredictably varying concentrations of active agents, and a lack of product information and sufficient labelling (Ahmad Khan et al., Citation2013; Hashmi, Citation2016).
As was mentioned before, less contact with agricultural extension services is an important constraint on the adoption of multiple innovations in Pakistan. The importance of agricultural extension services has been of interest among researchers and is reflected in the recent and past literature regarding agricultural policy reforms (Ragasa & Mazunda, Citation2018; Rogers, Citation1962). However, Khan and Imam (Citation1985) imply firmly that the agricultural reform outcomes mainly benefit large farms and traditionally large farmers in developing countries, such as Pakistan. They assert that the ignorance of small farmers likely increases agricultural inefficiencies. Reform policies that are not coupled with small farming households are constrained and inefficient since the smaller farmers are deprived of certain socio-economic attributes (e.g. illiteracy, less awareness of modern innovations, and accessibility to modern innovations due to financial constraints). Hence, Elahi et al. (Citation2018) suggest that the lack of agricultural advisory services and barriers that restrict small farmers from obtaining agricultural credit are the main reasons behind less agricultural productivity. Likewise, for developing countries such as Pakistan, the adoption of innovation/modern farming practices is generally slower, and farmers’ interests vary according to the feasibility of technologies (Ashraf et al., Citation2015).
Government institutions, which are mainly in the form of agricultural extension services, contribute substantially to technology adoption. In disseminating modern technologies and modern ways of farming, the government of Pakistan much relies on agricultural extension services. However, Baloch and Thapa (Citation2019) and Bilal et al. (Citation2015) highlight the main hurdles in the effective outreach and in the implementation of agricultural policies because of institutional constraints that include:
lack of knowledge capital and fewer extension workers
the focus of extension services mainly on large farmers
ignorance of smallholders
financial constraints
lack of communication between agriculture departments
Considering the latest digital developments, farmers’ awareness of modern digital technologies and electronic media play an essential role in innovation adoption. It expedites famers’ existing knowledge of modern farming technologies and keeps them aware of the latest information (Abbas et al., Citation2003). Among the medium of information transfer to farmers, the information and communication technology (ICT) is now considered a vital tool for the farming community. However, the effectiveness of ICT on disseminating information to farmers’ demands in-service training and capacity building programmes for extension service providers (Aldosari et al., Citation2019).
3. Study sites and sample selection
We administrated a smallholder farming household survey in the Punjab Province of Pakistan, focusing on smallholders because of their substantive majority (64%). The Punjab Province is vital concerning agriculture as it accounts for 58% of the total cultivated area of Pakistan. In 2022, the total population of Pakistan was 224.78 million, of which 141.96 was rural, while the population of Punjab was 110 million, of which 70 million was rural (Government of Pakistan, Citation2022). Additionally, rural Punjab's literacy rate is just 57%, out of which 67% of males are literate and 48% of females. The shares of Punjab in the total production of major crops in Pakistan are as follows: cotton 76%, rice 64%, sugarcane 64% and wheat 75% (Government of Punjab, Citation2019).
The Punjab Province has nine divisions (a division is the highest administrative unit), with each division consisting of some districts. Data used for the study was drawn from the three vital agricultural divisions of the Punjab Province, resulting in three randomly selected districts. Among these, the Rahimyar Khan District in the Bahawalpur Division, the Vehari District in the Multan Division and the Pakpattan District in the Sahiwal Division constitute the cotton-wheat zone of Pakistan (see ).
Figure 1. Map of Pakistan pointing to the study area; source: own elaboration. (This map is developed using shapefiles from https://geodata.lib.utexas.edu and ggplot2 package in R statistical software; Wickham, Citation2016.)

Administratively, each division is further delineated into districts, tehsils (below the district administrative unit), and union councils (the lowest administrative unit with a formal government comprising several villages). Using a multi-stage random sample, households from 18 villages in the cotton-wheat zone of the Punjab Province were surveyed from September to December 2017. The final sample size yields N = 275 smallholders who were selected for face-to-face interviews. We use a structured questionnaire and collected information about the household's socio-demographics, food security, the adoption status of genetically engineered seeds (Bt cotton and high-quality wheat seeds), herbicides resulting from cutting-edge technology, and no-tillage farming.
presents the percentage shares of important farming indicators of the survey area from the entire Punjab Province. The table shows a substantial share of the study area under cotton crops (72%) in comparison to the whole province. Additionally, displays that 28% of the province's total population is living in the study area, which is a bit higher for the rural population alone (33% of the province's rural population lives in the study area). Furthermore, the literacy ratio of the rural and urban population of the survey area is substantially low and similar to the entire Punjab Province.
Table 1. Salient indicators of the survey area.
4. Theoretical framework
In this section, we present a theoretical framework for the adoption of multiple technologies which is built on previous studies on farmers’ adoption decisions. A theoretical framework is necessary to model the adoption process numerically. Many frameworks have been developed during the last decades to model innovation adoption in agriculture. We have employed the utility maximization theoretical framework that was first employed by Rahm and Huffman (Citation1984) for the empirical analysis of adoption and further developed for adoption modelling of farmers by Adesina and Zinnah (Citation1993) and Batz et al. (Citation1999). As was formulated by Ruzzante et al. (Citation2021), the economic constraints paradigm suggests that farmers’ main goal is utility maximization subject to their available uneven resource endowments, and this leads to observed patterns of innovation adoption. This paradigm is widely used by economists to analyze technology adoption. We have used the recent adaptations of the utility maximization model by Möhring and Finger (Citation2022), which allows us to model individual farmers’ decisions on multi-technology adoption based on the utility maximization theoretical framework.
We assumed the jth smallholder farming household (j = 1, … , N), which was determined based on whether or not they opted for the given technologies. Following the conceptual framework defined by Möhring and Finger (Citation2022) for farmers’ adoption of a single technology, let denotes the random profit of farmer j, where
denotes a farmer's adoption decision of kth modern inputs technology/farming practice (with
reflection adoption of one of the technologies).
denotes the vector of the farm and farmers’ characteristics and
reflects the uncertainty affiliated to the adopted technology. We can define the utility maximizing problem of a farmer due to its adoption as:
(1)
(1) where
is a von Neumann-Morgenstern utility function of the farmer and
denotes a farmer's expectations of the technology effects on his production yields, income and costs. A utility maximizing farmer would then choose to adopt the new production technology, ceteris paribus, if:
(2)
(2) here, let
represent the utility level of the smallholder farming household from traditional farming practices and let
represent the utility level of the adoption of kth modern inputs technology/farming practice. The smallholder farming household decided to adopt the kth technology if:
(3)
(3) where
represents the expected benefit a smallholder farming household earns from adopting kth technology, and it is a latent variable determined by observed and unobserved characteristics of the farming households, which can be presented as (Cappellari & Jenkins, Citation2003):
(4)
(4) where
represents the observed characteristics of a farm and farmer;
represents unobserved characteristics; k denotes the type of technology available and
denotes the vector of the parameter to be estimated.
5. Empirical strategy
Based on our theoretical framework, we employed multivariate probit models as they require capturing the unobserved factors and the extent of association among adoption decisions to avoid biased and inefficient estimates (Abay et al., Citation2018). According to Kassie et al. (Citation2009) and Mesfin et al. (Citation2016), the significant features of this model are:
allowing for potential correlation between unobserved factors
simultaneously modelling the effect of observed factors on each specific adopted technology/farming practice
Therefore, by applying the multivariate probit models, in addition to finding the influential factors, we can envisage the complementary (positive correlation) and trade-off (negative correlation) between the adoption of genetically engineered seeds such as Bt cotton (referred to as B) and high-quality wheat seeds (referred to as W), herbicides resulting from cutting-edge technology (referred to as H), and no-tillage farming (referred to as T).
Using the indicator function, the unobserved preferences in Equation (4) translate to the observed binary outcome equation for each choice as follows:
(5)
(5)
In the multivariate probit model, the error terms jointly follow a multivariate normal distribution (MVN) with zero conditional mean and variance, which is normalized to unity. With this condition, the symmetric covariance matrix can be given by:
(6)
(6)
The off-diagonal elements in the covariance matrix represent the unobserved correlation between the stochastic components of the different types of innovative technologies (Teklewold et al., Citation2013).
6. Results
The descriptive summary of the demographic and socio-economic variables of the smallholder farming households that was acquired from the survey is presented in . We follow other empirical studies and economic theory to have necessary covariates for this study (Bilal et al., Citation2022a; Bilal & Barkmann, Citation2019; de Janvry et al., Citation1991).
Table 2. Descriptive statistics.
Most notably, farm machinery index is remarkably low across the sampled farmers (14%) but a substantive majority (79%) practice laser levelling. Likewise, almost half (46%) of the total farms also generate income other than farming. The average amount of contact made by agricultural extension agents with farmers is only less than one visit/month (0.80), however, almost a quarter seek agricultural information via radio (26%), and a few were members of a local farmers’ association (10%). The variable of access to credit does not necessary mean that respondents actually received a credit. It only shows that if the respondents need credit, they can easily fulfil requirements to obtain the agri-credit.Footnote3 shows the visual picture of the causal relationship between the six selected factors and innovation adoption by farm operators.
Figure 2. Confidence interval plots of demographic and structural factor of the farm management of smallholder farming households concerning adoption. Source: Field survey results.

The average area under wheat cultivation (0.93 ha) by farm operators compared to the mean area under cotton cultivation (0.76 ha) is almost the same. Interestingly, the average of cotton and wheat area significantly differs between exclusive non-adopters’ farms ((b)/(d)). However, there is a moderate causal relationship between the area under cotton cultivation and technology adoption on average. The farm machinery index and off-farm income sources also tends to be higher for adopters’ farms ((c)/(e)). Nevertheless, on average there is a more consistent causal pattern between farm machinery index and technology adoption. The same trend can be seen for number of years of education and visits by agricultural extension services ((a)/(f)). Likewise, there is a more persistent causal trend between visits by agricultural extension services and technology adoption on average. For all farm variables, ANOVA with the post hoc Scheffé test indicates that adopters’ farms differ from non-adopters’ farms.
shows a descriptive analysis of adoption levels in the sample. The results of show that, in our sample, less than 16% are exclusive non-adopters and approximately 25% of farm operators are exclusive adopters. Approximately 59% of adopters are between these two extremes of adoption and non-adoption. A simple comparison of demographic and socio-economic variables based on adoption decisions presented in might be tainted due to a failure of capturing the unobserved factors and the extent of association among adoption decisions. Therefore, we employ a multivariate probit model to test different hypothesis of the possible causal effects of demographic and socio-economic variables on adoption choices.
Table 3. Smallholder farming households’ distribution with respect to adoption decisions.
In we model the multivariate probit outcomes of whether smallholder farming households adopt/practice no-tillage farming, Bt cotton, high-quality wheat seeds and herbicides resulting from cutting-edge technology as functions of demographic and socio-economic variables of smallholder farming households as presented in .
Table 4. Estimates of the multivariate probit models variable.
We also performed independent probit models for the four different adopted technologies as a means of a robustness check of the estimated multivariate probit model results. Tables A1, A2, A3 and A4 in the appendix present the results of the probit models.
Compared to the multivariate probit outcomes of whether smallholder farming households adopt/practice no-tillage farming, Bt cotton, high-quality wheat seeds, and/or herbicides resulting from cutting-edge technology as functions of demographic and socio-economic variables of smallholder farming households, the independent probit models for the four different adopted technologies showed structurally similar effects in terms of the significance and signs of the coefficients. However, following Pregibon (Citation1980), to further rationalize the included variables, we employed a model specification test to test the null hypothesis that predictions squared have no explanatory power. In addition, we performed the Hosmer–Lemeshow goodness-of-fit test (Hosmer & Lemeshow, Citation1980) to measure the discrimination and calibration measures of how well the model estimated probabilities agreeing with the observed outcomes (Fagerland & Hosmer, Citation2012). The results are presented in the last rows of Tables A1, A2, A3 and A4 in the appendix.
Most importantly, also presents the correlation between the error terms of the adoption decisions. The correlation coefficients among smallholder farming households’ adoption decisions/farming practices were statistically different from zero in all six of the combinations and all combinations were positive, indicating complementarity among the adoption decisions significantly. However, the magnitude of the correlation coefficients varied respectively. The Likelihood ratio test which tests the hypothesis of zero elements of the covariance matrix (see Equation (6)) can be defined as ρTB = ρTW = ρTH = ρBW = ρBH = ρWH = 0. This test strongly rejected the zero elements of the covariance matrix with χ2 (6) equal to 99 at a 1% level of significance. This indicates that our assumption that the adoption of Bt cotton and high-quality wheat seeds, herbicides resulting from cutting-edge technologies, and no-tillage farming is not mutually independent is held. Therefore, we were justified in employing multivariate probit models to yield unbiased and efficient estimates required to capture unobserved factors and the extent of association among adoption decisions (Abay et al., Citation2018).
We calculated the marginal effect estimates of the coefficients presented in the third column of for meaningful economic interpretations and this indicated that a significant impact of area was sowed with wheat (ha) and cotton (ha) was exclusively pronounced for all adoption decisions. Interestingly, the marginal effects estimates of the said covariates differ depending on the nature of adoption. For example, having land sowed with wheat negatively influences the probability of adoption/farming practices of no-tillage, Bt cotton and herbicides resulting from cutting-edge technology by −20%, −29% and −12% per hectare, respectively. However, area sowed under wheat (ha) positively +9% promotes the adoption of high-quality wheat seeds. In contrast, the area sowed under cotton positively influences the probability of adoption/farming practices of no-tillage, Bt cotton, high-quality wheat seeds and herbicides resulting from cutting-edge technology strongly +31%, +61%, +19% and +22% per hectare, respectively. This shows that farmers with a tendency to plant more cotton are more motivated to apply more innovative technologies compared to wheat cultivation.
Off-farm income activities positively influence the probability of adopting Bt cotton, high-quality wheat seeds and herbicides resulting from cutting-edge technology by 13%, 16% and 12% per hectare, respectively, while no-tillage is insignificant. Similarly, farmers’ education tends to promote the adoption of Bt cotton and herbicides resulting from cutting-edge technology significantly +2% and +2%, while no significant impact of farmers’ education was observed for adopting/farming practices of no-tillage and high-quality wheat seeds.
Similarly, higher farm machinery index tends to promote the adoption of high-quality wheat seeds and herbicides resulting from cutting-edge technology strongly +17% and +18%, while no significant impact of farm machinery index was observed for adopting/farming practices of no-tillage and Bt cotton.
The number of visits of an agricultural extension agent is insignificant for adopting high-quality wheat seeds, weakly significant (at the 10% level) for the adoption of Bt cotton and highly significant for the adoption of herbicides. However, it could not be eliminated from the equation system. One additional visit of an agricultural extension agent increases the probability of adopting Bt cotton and herbicides resulting from cutting-edge technology +5% and +10%, respectively. Similarly, the probability of the farm distance to the input market −1% was significantly pronounced for the adoption of Bt cotton only.
The traditional literature regarding no-tillage adoption decisions does not consider the farm distance from the input and output market as a determinant of the adoption (D’Emden et al., Citation2008). For a subsequent analysis, we excluded the distance variables from the main model presented above for the no-tillage equation only. Consequently, based on a non-significant likelihood ratio test LR χ2 (3) = 5.79 at higher than 10%, the exclusion of these variables in the main model presented above was supported.
The application of laser levelling has a marginally significant effect on the application of no-tillage, Bt cotton and herbicides resulting from cutting-edge technology which can be a necessary complementary input for the application of these technologies. Interestingly, the number of milch animals positively influenced the probability of adopting high-quality wheat seeds and endured insignificant for the rest of the adoption decisions. Years of education only has a positive effect on Bt cotton and herbicides resulting from cutting-edge technology. This could be due to the simpler nature of no-tillage and high-quality wheat seeds.
7. Discussion and policy implications
Adopting genetically engineered seeds such as Bt cotton or high-quality wheat seeds, herbicides resulting from cutting-edge technologies and no-tillage farming are regarded as sustainable agriculture technologies. As Lee (Citation2005) highlights, improved crop varieties, no-tillage and the complementary use of inorganic chemicals, among others, illustrate sustainable agriculture. Pretty (Citation2018) proposes that policies in favour of SAI need to be handled delicately and that relevant stakeholders should pay more attention to the adaptation process of the SAI model, as the introduction of SAI has increased the importance of multiple technology adoption. Technological innovations have the potential to benefit small farms in low and middle income countries and developing relevant policies for farmers to apply these technologies is essential (Diao et al., Citation2023). As a result, any information on likely adopters can help responsible organizations target certain farmers or guide the resources to certain effective policies (Dorfman, Citation1996). Still there are factors that are not captured, or the unobserved heterogeneity of households and soil can be affected by technology adoption choices (Abay et al., Citation2018).
This study firstly shows that adoption of innovative technologies is not an isolated, separate process but rather an interconnected decision process between farmers and their selection options of different technologies and cropping patterns. The likelihood ratio test shows that we cannot look to technology adoption and barriers to apply innovation in isolation but in a coordinated way. The fact that all eligible causal factors have a different correlation when employing different levels of technologies. shows different associations and even possible contrasting patterns of the causal effects of the factors on the adoption of technologies can be recognized. This fact is reflected in . The contrasting results of the negative effect of planting wheat on the adoption of no-tillage, Bt cotton seed and herbicides of cutting age technology shows that the diversification between wheat and cotton will be a barrier toward multiple innovative technology adoption. Therefore, diversification between wheat and cotton and the adoption of advanced technologies are not mutually exclusive goals in this case. However, the planting of cotton should be promoted for policies geared towards the adoption of more innovative technologies.
By considering the different means of transferring information on new technologies to the farmers, we have three factors: years of education, number of agri-extension visits and membership in a farmers’ union. The insignificance of a farmers’ union is a sign of a low-level of possible technology information transferring from these institutions. It is evident that years of education would positively affect the application of Bt cotton and cutting-age herbicides. This means more knowledgeable farmers are interested in these technologies and cotton production. Looking to , we find that the number of visits of extension officers is generally low (less than one on average). The effectiveness of this factor on herbicide application is a sign that these limited visits may only be guided to one technology. The effectiveness of training on the application of innovations by farmers has already been tested by many studies (e.g. Barrett et al., Citation2022). Therefore, more visits by extension officers with diversified programmes in technology training could be a possible policy to follow.
Farm machinery index affects the adoption of cutting-edge herbicides and high-quality wheat seeds. Bilal et al. (Citation2022b) observed a significant complementary effect between the adoption of multinational crop care products and mechanized farming. Their study presents the broader societal impacts of the adoption of multinational crop care products on smallholders’ food security. No-tillage technology is free from machinery and Bt cotton seed is probably more labour intensive for smallholders.
Laser level affects no-tillage and Bt cotton application positively and significantly. This is a sign that this input, which is used by 79% of farmers in the sample, is an integrated innovation in this production system. This also shows that the adoption of some innovations and technologies can further promote the application of other innovations. Giordano et al. (Citation2017) highlight the agronomic advantages (e.g. water conservation) of the laser levelling of fields and no-tillage. Therefore, a synergic effect of promoting one innovation to promote others can be a strategy for responsible agencies.
One special observation in this study was a significant positive effect of the number of milch animals on high-quality wheat seeds. This reflects the presence of diversified agri-food systems (Puech & Stark, Citation2023), as wheat is considered a joint supply crop since wheat straw can also be used as livestock fodder (Shrivastava et al., Citation2014). Moreover, considering the average size of household and small farm size, we can conclude that labour abundancy is available in the region, and it supports small farming households practising diversification. In addition, in their scoping review, Nabuuma et al. (Citation2022) discuss the positive effects of improved quality seeds on dietary quality, nutrition status and smallholder farming households’ resilience.
Finally, our results show that there's no effect of access to credit but a positive effect of off-farm income on all technology adoptions except no-tillage. Tadjiev et al. (Citation2023) suggest that no-tillage adoption supports higher herbicide costs but reduces machinery costs for the resource constrained farmers. No-tillage paved the way for targeting resource constrained smallholders in developing countries (Montt & Luu, Citation2020). As no-tillage is not an intensively financially dependent technology, we can conclude that limited financial resources are a restriction to other technology adoption. The insignificance of access to credit by definition doesn't necessarily show whether enough financial resources are available to a farmer as the off-farm income has significant effects on the adoption of innovations except no-tillage farming. As other studies show (e.g. Marenya & Barrett, Citation2009), limited access to credit is a restriction for technology adoption by smallholders.
8. Conclusion
This paper examined the determinants of adopting vital agricultural technological inputs simultaneously. Mainly, this study investigated the potential reasons stopping smallholder farming households from adopting genetically engineered seeds (Bt cotton and improved wheat seeds), herbicides resulting from cutting-edge technologies, and no-tillage farming. We employed multivariate probit models to utilize original farm household-level data from 275 smallholder farming households in Pakistan. We found that the adoption selection is an interconnected decision that takes the affecting factors and cropping patterns into consideration. We recognize that planting cotton motivates farmers to adopt higher levels of different technologies. The estimates of the multivariate probit models show that a lack of or inappropriate agricultural extension services contacts was one of the barriers to farmers adopting technological agricultural inputs. The estimates also indicate that higher farm machinery index and farmers’ education facilitate certain technology adoption. Therefore, promoting the role of agricultural extension services (qualitatively and quantitatively) and providing more financial resources through credit access and off-farm income sources are likely to play a role in multiple technology adoption.
Based on the results, we suggest a few crucial policy implications: (1) the number of milch animals positively influences the probability of adopting high-quality wheat seeds, and this reflects the presence of diversified agri-food systems. In their scoping review, Nabuuma et al. (Citation2022) discuss the positive effects of improved quality seeds on dietary quality, nutrition status and smallholder farming households’ resilience, hence, promoting diversification as a policy instrument amidst high food insecurity in developing countries, such as Pakistan. (2) No-tillage adoption is not dependent on heavily mechanized farming; it paved the way for targeting resource constrained smallholders in developing countries, hence, it could be an important policy tool for achieving further agronomic advantages (e.g. water conservation and mitigating soil erosion). (3) Since a lack of or inappropriate agricultural extension services contacts was one of the barriers to farmers adopting technological agricultural inputs, more visits by extension officers with diversified programmes in technology training could be a possible policy to follow. In sum, a synergic effect of promoting one innovation that has the potential to promote the adoption of other innovations can be a strategy for responsible agencies.
We acknowledge that the research presented in this study has some limitations. This research was confined solely to smallholder farming households, who are characterized by their landholdings being equal to or less than 5 acres [∼2.02 ha]. The inclusion of medium-sized farming households (landholdings equal to or less than 25 acres [∼10.11 ha] but more than 5 acers [∼2.02 ha]) and large-scale farmers (landholdings of more than 25 acres [∼10.11 ha]) would be interesting for further investigating the adoption underpinnings. Further research could be conducted by considering access to digital technologies and the role of social media channels on extension activities for adopting multiple technologies, as well as the adoption of simple digital technologies. More types of farm production could also be included in future analyzes.
Acknowledgement
This research was conducted during the presence of the two authors at the School of Business & Economics, Westminster International University in Tashkent in Uzbekistan and Leibniz Institute of Agricultural Development in Transition Economies (IAMO) in Germany. We thank Prof. Dr. Bernhard Brümmer (University of Göttingen) and Prof. Dr. Jan Barkmann (Darmstadt University of Applied Sciences) for their valuable suggestions and guidance in the construction of the field survey. The authors also like to thank four anonymous reviewers for very helpful comments on an earlier draft of this paper. Thanks also go to Dr. Abdusame Tadjiev (IAMO) for his intellectual support during the revision process of this paper. The authors are grateful to Mary Wales for her proofreading support. Farmers in the sample were interviewed after they provided informed consent orally and volunteered to participate in the survey.
Disclosure statement
No potential conflict of interest was reported by the author(s).
Additional information
Funding
Notes on contributors
Muhammad Bilal
Muhammad Bilal is currently a Senior Lecturer at the School of Business & Economics, Westminster International University in Tashkent, Uzbekistan. He got Ph.D. from Georg-August-University Göttingen, Germany. His research interests include different aspects of food systems, sustainable development goals, innovation in agricultural technologies, smallholders, and multinational corporations in the agriculture sector.
Tinoush Jamali Jaghdani
Tinoush Jamali Jaghdani is a Research Associate at the Leibniz Institute of Agricultural Development in Transition Economies (IAMO) in Germany since October 2016. He received his PhD in agricultural economics (Faculty of Agriculture) with a minor in applied statistics (Faculty of Mathematics) from the University of Göttingen (Germany) in 2012. He acquired his B.Sc. (2001) and M.Sc. (2007) in agricultural economics from Iran and Germany respectively. His research focus has been on water economics, food price volatility, market power, trade duration and food supply chain governance.
Notes
1 Agricultural Census 2010 in Pakistan; https://www.pbs.gov.pk/publication/agricultural-census-2010-pakistan-report
2 Organization for Economic Cooperation & Development
3 The correlation coefficient between ‘access to credit’ and ‘off farm income’ is −0.0479 and is insignificant.
References
- Abay, K. A., Berhane, G., Taffesse, A. S., Abay, K., & Koru, B. (2018). Estimating input complementarities with unobserved heterogeneity: Evidence from Ethiopia. Journal of Agricultural Economics, 69(2), 495–517. https://doi.org/10.1111/1477-9552.12244
- Abbas, M., Sheikh, D., Muhammad, S., & Ashfaq, M. (2003). Role of electronic media in the adoption of agricultural technologies by farmers in the central Punjab-Pakistan. International Journal of Agriculture and Biology, 5(1), 417–419. https://www.researchgate.net/publication/237304797_Role_of_Electronic_Media_in_the_Adoption_of_Agricultural_Technologies_by_Farmers_in_the_Central_Punjab-Pakistan
- Abdulai, A., & Huffman, W. (2014). The adoption and impact of soil and water conservation technology: An endogenous switching regression application. Land Economics, 90(1), 26–43. https://doi.org/10.3368/le.90.1.26
- Abdullah, A. (2010). An analysis of Bt cotton cultivation in Punjab, Pakistan using the agriculture decision support system (ADSS). AgBioForum, 13(3), 274–287. https://mospace.umsystem.edu/xmlui/handle/10355/8975
- Adesina, A. A., & Zinnah, M. M. (1993). Technology characteristics, farmers’ perceptions and adoption decisions: A Tobit model application in Sierra Leone. Agricultural Economics, 9(4), 297–311. https://doi.org/10.1111/j.1574-0862.1993.tb00276.x
- Ahmad Khan, F. Z., Sagheer, M., Hasan, M. u., Tahira Gul, H., Hassan, F., Manzoor, S. A., & Wahid, A. (2013). Agricultural dynamics in Pakistan: Current issues and solutions. Russian Journal of Agricultural and Socio-Economic Sciences, 20(8), 20–26. https://doi.org/10.18551/rjoas.2013-08.03
- Aktar, W., Sengupta, D., & Chowdhury, A. (2009). Impact of pesticides use in agriculture: Their benefits and hazards. Interdisciplinary Toxicology, 2(1), 1. https://doi.org/10.2478/v10102-009-0001-7
- Aldosari, F., Al Shunaifi, M. S., Ullah, M. A., Muddassir, M., & Noor, M. A. (2019). Farmers’ perceptions regarding the use of information and communication technology (ICT) in Khyber Pakhtunkhwa, Northern Pakistan. Journal of the Saudi Society of Agricultural Sciences, 18(2), 211–217. https://doi.org/10.1016/j.jssas.2017.05.004
- Ali, H., Shoukat, A., Ahmad, S., Sarwar, N., Arooj, M., Mahmood, A., & Shahzad, A. N. (2013). Impact of integrated weed management on flat-sown cotton (Gossypium hirsutum L.). Journal of Animal and Plant Sciences, 23(4), 1185–1192. https://thejaps.org.pk/Volume/2013/23-4/abstracts/36.php
- Alston, J. M., & Pardey, P. G. (2021). Chapter 75 - The economics of agricultural innovation. In C. B. Barrett, & D. R. Just (Eds.), Handbook of agricultural economics (Vol. 5, pp. 3895–3980). Elsevier B.V. https://doi.org/10.1016/bs.hesagr.2021.10.001
- Asfaw, S., Kassie, M., Simtowe, F., & Lipper, L. (2012). Poverty reduction effects of agricultural technology adoption: A micro-evidence from rural Tanzania. Journal of Development Studies, 48(9), 1288–1305. https://doi.org/10.1080/00220388.2012.671475
- Ashraf, S., Khan, G. A., Ali, S., & Iftikhar, M. (2015). Socio-economic determinants of the awareness and adoption of citrus production practices in Pakistan. Ciência Rural, 45(9), 1701–1706. https://doi.org/10.1590/0103-8478cr20131227
- Aslam, M. (2016). Agricultural productivity current scenario, constraints and future prospects in Pakistan. Sarhad Journal of Agriculture, 32(4), 289–303. https://doi.org/10.17582/journal.sja/2016.32.4.289.303
- Baloch, M. A., & Thapa, G. B. (2019). Review of the agricultural extension modes and services with the focus to Balochistan, Pakistan. Journal of the Saudi Society of Agricultural Sciences, 18(2), 188–194. https://doi.org/10.1016/j.jssas.2017.05.001
- Barbera, V., Poma, I., Gristina, L., Novara, A., & Egli, M. (2012). Long-term cropping systems and tillage management effects on soil organic carbon stock and steady state level of C sequestration rates in a semiarid environment. Land Degradation & Development, 23(1), 82–91. https://doi.org/10.1002/ldr.1055
- Barrett, C. B., Islam, A., Malek, A., Pakrashi, D., & Ruthbah, U. (2022). Experimental evidence on adoption and impact of the system of rice intensification. American Journal of Agricultural Economics, 104(1), 4–32. https://doi.org/10.1111/ajae.12245
- Battese, G. E., Nazli, H., & Smale, M. (2017). Factors influencing the productivity and efficiency of wheat farmers in Punjab, Pakistan. Journal of Agribusiness in Developing and Emerging Economies, 7(2), 82–98. https://doi.org/10.1108/JADEE-12-2013-0042
- Batz, F. J., Peters, K. J., & Janssen, W. (1999). The influence of technology characteristics on the rate and speed of adoption. Agricultural Economics, 21(2), 121–130. https://doi.org/10.1111/j.1574-0862.1999.tb00588.x
- Bilal, M., & Barkmann, J. (2019). Farm and farmer capital foster adoption of improved quality agrochemical inputs in the cotton-wheat zone of the Punjab, Pakistan. Journal of Agriculture and Rural Development in the Tropics and Subtropics (JARTS), 120(2), 229–240. https://doi.org/10.17170/KOBRA-20191217881
- Bilal, M., Barkmann, J., & Brümmer, B. (2022a). The role of crop protection products of multinational brands for agricultural sustainability in the cotton-growing zone in Pakistan. Journal of Agriculture and Rural Development in the Tropics and Subtropics, 123(1), 1–11. https://doi.org/10.17170/KOBRA-202201195568
- Bilal, M., Brümmer, B., & Barkmann, J. (2022b). A discussion on the outcomes of adopted agricultural technological products and specific sustainable development goals: Evidence from Pakistan. Cogent Economics & Finance, 10(1), 1–21. https://doi.org/10.1080/23322039.2022.2132644
- Bilal, M., Shakoor, U., Nasir, A., & Raza, Q. (2015). Revisiting snags for obtaining agricultural credit in Pakistan: The case of small farmers. International Journal of Scientific Footprints, 3(4), 41–51.
- Brookes, G., Taheripour, F., & Tyner, W. E. (2017). The contribution of glyphosate to agriculture and potential impact of restrictions on use at the global level. GM Crops & Food, 8(4), 216–228. https://doi.org/10.1080/21645698.2017.1390637
- Cappellari, L., & Jenkins, S. P. (2003). Multivariate probit regression using simulated maximum likelihood. The STATA Journal, 3(3), 278–294. https://doi.org/10.1177/1536867X0300300305
- Danne, M., Musshoff, O., & Schulte, M. (2019). Analysing the importance of glyphosate as part of agricultural strategies: A discrete choice experiment. Land Use Policy, 86, 189–207. https://doi.org/10.1016/j.landusepol.2019.04.023
- de Janvry, A., Fafchamps, M., & Sadoulet, E. (1991). Peasant household behaviour with missing markets: Some paradoxes explained. The Economic Journal, 101(409), 1400. https://doi.org/10.2307/2234892
- D’Emden, F. H., Llewellyn, R. S., & Burton, M. P. (2008). Factors influencing adoption of conservation tillage in Australian cropping regions*. Australian Journal of Agricultural and Resource Economics, 52(2), 169–182. https://doi.org/10.1111/j.1467-8489.2008.00409.x
- de Oca Munguia, O. M., & Llewellyn, R. (2020). The adopters versus the technology: Which matters more when predicting or explaining adoption? Applied Economic Perspectives and Policy, 42(1), 80–91. https://doi.org/10.1002/aepp.13007
- de Oca Munguia, O. M., Pannell, D. J., & Llewellyn, R. (2021). Understanding the adoption of innovations in agriculture: A review of selected conceptual models. Agronomy, 11(1), 139. https://doi.org/10.3390/agronomy11010139
- Dessart, F. J., Barreiro-Hurlé, J., & Van Bavel, R. (2019). Behavioural factors affecting the adoption of sustainable farming practices: A policy-oriented review. European Review of Agricultural Economics, 46(3), 417–471. https://doi.org/10.1093/erae/jbz019
- Diao, X., Reardon, T., Kennedy, A., DeFries, R. S., Koo, J., Minten, B., Takeshima, H., & Thornton, P. (2023). The future of small farms: Innovations for inclusive transformation. In J. von Braun, , K. Afsana, L. O. Fresco, & M. H. A. Hassan (Eds.), Science and innovations for food systems transformation (pp. 191–205). Springer International Publishing. https://doi.org/10.1007/978-3-031-15703-5_10
- Dorfman, J. H. (1996). Modeling multiple adoption decisions in a joint framework. American Journal of Agricultural Economics, 78(3), 547–557. https://doi.org/10.2307/1243273
- Elahi, E., Abid, M., Zhang, L., ul Haq, S., & Sahito, J. G. M. (2018). Agricultural advisory and financial services; farm level access, outreach and impact in a mixed cropping district of Punjab, Pakistan. Land Use Policy, 71, 249–260. https://doi.org/10.1016/j.landusepol.2017.12.006
- Fagerland, M. W., & Hosmer, D. W. (2012). A generalized Hosmer–Lemeshow goodness-of-fit test for multinomial logistic regression models. The Stata Journal, 12(3), 447–453. https://doi.org/10.1177/1536867X1201200307
- Gallardo, R. K., & Sauer, J. (2018). Adoption of labor-saving technologies in agriculture. Annual Review of Resource Economics, 10(1), 185–206. https://doi.org/10.1146/annurev-resource-100517-023018
- Giordano, M., Turral, H., Scheierling, S. M., Tréguer, D. O., & Mccornick, P. G. (2017). Beyond “More Crop per Drop”: evolving thinking on agricultural water productivity. In IWMI Research Report 169. International Water Management Institute (IWMI). https://doi.org/10.5337/2017.202
- Government of Pakistan. (2017). Punjab Population 2017. Pakistan Bureau of Statistics (PBS). https://www.pbs.gov.pk/sites/default/files/population/2017/punjab_tehsil.pdf
- Government of Pakistan. (2022). Pakistan Economic Survey 2021-22. Ministry of Finance. https://www.finance.gov.pk/survey_2022.html
- Government of Punjab. (2019). Bureau of Statistics. Government of Punjab. https://www.bos.gop.pk/developmentstat
- Hashmi, M. S. (2016). Agrochemical and agricultural sustainability: A case study of Pakistan. Journal of Environmental and Agricultural Sciences, 9, 1–9. http://www.agropublishers.com/files/JEAS 9 1.PDF. http://jeas.agropublishers.com/
- Herrero, M., Thornton, P. K., Mason-D’Croz, D., Palmer, J., Benton, T. G., Bodirsky, B. L., Bogard, J. R., Hall, A., Lee, B., Nyborg, K., Pradhan, P., Bonnett, G. D., Bryan, B. A., Campbell, B. M., Christensen, S., Clark, M., Cook, M. T., de Boer, I. J. M., Downs, C., … West, P. C. (2020). Innovation can accelerate the transition towards a sustainable food system. Nature Food, 1(5), 266–272. https://doi.org/10.1038/s43016-020-0074-1
- Hosmer, D. W., & Lemeshow, S. (1980). Goodness of fit tests for the multiple logistic regression model. Communications in Statistics - Theory and Methods, 9(10), 1043–1069. https://doi.org/10.1080/03610928008827941
- Jaghdani, T. J., & Brümmer, B. (2016). Determinants of water purchases by pistachio producers in an informal groundwater market: A case study from Iran. Water Policy, 18(3), 599–618. https://doi.org/10.2166/wp.2015.093
- Jalal-Ud-Din, M. (2011). The socio-economic problems of small farmers in adopting new agricultural technology: A case study of three villages in district Mardan. Sarhad Journal of Agriculture, 27(2), 299–304. https://www.aup.edu.pk/sj_pdf/23-THE SOCIO-ECONOMIC PROBLEMS OF SMALL FARMERS IN.pdf
- James, C. (2012). Global Status of Commercialized Biotech/GM Crops: 2012. In ISAAA Brief No. 44. ISAAA. International Service for the Acquisition of Agri-biotech Applications (ISAAA). https://ifs.org.uk/publications/15065
- Kassie, M., Shiferaw, B., & Muricho, G. (2011). Agricultural technology, crop income, and poverty alleviation in Uganda. World Development, 39(10), 1784–1795. https://doi.org/10.1016/j.worlddev.2011.04.023
- Kassie, M., Zikhali, P., Manjur, K., & Edwards, S. (2009). Adoption of sustainable agriculture practices: Evidence from a semi-arid region of Ethiopia. Natural Resources Forum, 33(3), 189–198. https://doi.org/10.1111/j.1477-8947.2009.01224.x
- Khan, M. H., & Imam, S. F. (1985). Public policy and agricultural transformation in Pakistan. The Pakistan Development Review, 24(3/4), 305–332. https://www.jstor.org/stable/41258714
- Kotu, B. H., Alene, A., Manyong, V., Hoeschle-Zeledon, I., & Larbi, A. (2017). Adoption and impacts of sustainable intensification practices in Ghana. International Journal of Agricultural Sustainability, 15(5), 539–554. https://doi.org/10.1080/14735903.2017.1369619
- Lee, D. (2005). Agricultural sustainability and technology adoption: Issues and policies for developing countries. American Journal of Agricultural Economics, 87(5), 1325–1334. https://doi.org/10.1111/j.1467-8276.2005.00826.x
- Malik, S. J., Sheikh, A. T., & Jilani, A. H. (2016). Inclusive agricultural growth in Pakistan-understanding some basic constraints. Pakistan Development Review, 55(4), 889–903. https://doi.org/10.30541/v55i4I-IIpp.889-903
- Marenya, P. P., & Barrett, C. B. (2009). State-conditional fertilizer yield response on Western Kenyan farms. American Journal of Agricultural Economics, 91(4), 991–1006. https://doi.org/10.1111/j.1467-8276.2009.01313.x
- Mendola, M. (2007). Agricultural technology adoption and poverty reduction: A propensity-score matching analysis for rural Bangladesh. Food Policy, 32(3), 372–393. https://doi.org/10.1016/j.foodpol.2006.07.003
- Mesfin, H. M., Ahmed, M. H., & Abady, S. (2016). Determinants of multiple groundnut technology adoption in eastern Ethiopia. Review of Agricultural and Applied Economics, 19(02), 51–60. https://doi.org/10.15414/raae/2016.19.02.51-60
- Möhring, N., & Finger, R. (2022). Pesticide-free but not organic: Adoption of a large-scale wheat production standard in Switzerland. Food Policy, 106, 102188. https://doi.org/10.1016/j.foodpol.2021.102188
- Montt, G., & Luu, T. (2020). Does conservation agriculture change labour requirements? Evidence of Sustainable Intensification in Sub-Saharan Africa. Journal of Agricultural Economics, 71(2), 556–580. https://doi.org/10.1111/1477-9552.12353
- Muhammad, F., Hassan, M. A., & Mehmood, T. (2020). An overview of the existing problems faced by Pakistani women in agriculture: Conclusion and recommendation. Ecofeminism and Climate Change, 1(2), 97–104. https://doi.org/10.1108/EFCC-04-2020-0010
- Nabuuma, D., Reimers, C., Hoang, K. T., Stomph, T. J., Swaans, K., & Raneri, J. E. (2022). Impact of seed system interventions on food and nutrition security in low- and middle-income countries: A scoping review. Global Food Security, 33, 100638. https://doi.org/10.1016/j.gfs.2022.100638
- Noltze, M., Schwarze, S., & Qaim, M. (2013). Impacts of natural resource management technologies on agricultural yield and household income: The system of rice intensification in Timor Leste. Ecological Economics, 85, 59–68. https://doi.org/10.1016/j.ecolecon.2012.10.009
- Nyangena, W., & Juma, O. M. (2014). Impact of improved farm technologies on yields: The case of improved maize varieties and inorganic fertilizer in Kenya. In RFF Working Paper Series, dp-14-02-efd. Resources for the Future. https://www.efdinitiative.org/publications/impact-improved-farm-technologies-yields-case-improved-maize-varieties-and-inorganic
- Ochieng, J., Afari-Sefa, V., Muthoni, F., Kansiime, M., Hoeschle-Zeledon, I., Bekunda, M., & Thomas, D. (2022). Adoption of sustainable agricultural technologies for vegetable production in rural Tanzania: Trade-offs, complementarities and diffusion. International Journal of Agricultural Sustainability, 20(4), 478–496. https://doi.org/10.1080/14735903.2021.1943235
- Pregibon, D. (1980). Goodness of link tests for generalized linear models. Journal of the Royal Statistical Society Series C: Applied Statistics, 29(1), 15–24. https://doi.org/10.2307/2346405
- Pretty, J. (2018). Intensification for redesigned and sustainable agricultural systems. Science, 362(6417), 1–7. https://doi.org/10.1126/science.aav0294
- Puech, T., & Stark, F. (2023). Diversification of an integrated crop-livestock system: Agroecological and food production assessment at farm scale. Agriculture, Ecosystems & Environment, 344, 108300. https://doi.org/10.1016/j.agee.2022.108300
- Ragasa, C., & Mazunda, J. (2018). The impact of agricultural extension services in the context of a heavily subsidized input system: The case of Malawi. World Development, 105, 25–47. https://doi.org/10.1016/j.worlddev.2017.12.004
- Rahm, M. R., & Huffman, W. E. (1984). The adoption of reduced tillage: The role of human capital and other variables. American Journal of Agricultural Economics, 66(4), 405–413. https://doi.org/10.2307/1240918
- Ridley, M. (2020). How Innovation Works (Vol. 1, 1st ed.). 4th Estate. https://www.4thestate.co.uk/book/how-innovation-works-9780008334819/
- Rogers, E. M. (1962). Diffusion of innovations (Vol. 1, 1st ed.). Free Press of Glencoe.
- Rosário, J., Madureira, L., Marques, C., & Silva, R. (2022). Understanding farmers’ adoption of sustainable agriculture innovations: A systematic literature review. Agronomy, 12(11), 2879. https://doi.org/10.3390/agronomy12112879
- Ruzzante, S., Labarta, R., & Bilton, A. (2021). Adoption of agricultural technology in the developing world: A meta-analysis of the empirical literature. World Development, 146, 105599. https://doi.org/10.1016/j.worlddev.2021.105599
- Shrivastava, B., Jain, K. K., Kalra, A., & Kuhad, R. C. (2014). Bioprocessing of wheat straw into nutritionally rich and digested cattle feed. Scientific Reports, 4(1), 1–9. https://doi.org/10.1038/srep06360
- Silvestri, S., Richard, M., Edward, B., Dharmesh, G., & Dannie, R. (2021). Going digital in agriculture: How radio and SMS can scale-up smallholder participation in legume-based sustainable agricultural intensification practices and technologies in Tanzania. International Journal of Agricultural Sustainability, 19(5–6), 583–594. https://doi.org/10.1080/14735903.2020.1750796
- Simtowe, F., Asfaw, S., & Abate, T. (2016). Determinants of agricultural technology adoption under partial population awareness: The case of pigeonpea in Malawi. Agricultural and Food Economics, 4(1), 1–21. https://doi.org/10.1186/s40100-016-0051-z
- Tadjiev, A., Djanibekov, N., & Herzfeld, T. (2023). Does zero tillage save or increase production costs? Evidence from smallholders in Kyrgyzstan. International Journal of Agricultural Sustainability, 21(1), 1–16. https://doi.org/10.1080/14735903.2023.2270191
- Takahashi, K., Muraoka, R., & Otsuka, K. (2020). Technology adoption, impact, and extension in developing countries’ agriculture: A review of the recent literature. Agricultural Economics, 51(1), 31–45. https://doi.org/10.1111/agec.12539
- Teklewold, H., Kassie, M., & Shiferaw, B. (2013). Adoption of multiple sustainable agricultural practices in rural Ethiopia. Journal of Agricultural Economics, 64(3), 597–623. https://doi.org/10.1111/1477-9552.12011
- Thapa, G., & Gaiha, R. (2014). Smallholder farming in Asia and the pacific: Challenges and opportunities. In P. B. R. Hazell, & A. Rahman (Eds.), New directions for smallholder agriculture (1st ed., pp. 69–114). Oxford University Press. https://doi.org/10.1093/ACPROF:OSO/9780199689347.003.0004
- Wauters, E., & Mathijs, E. (2014). The adoption of farm level soil conservation practices in developed countries: A meta-analytic review. International Journal of Agricultural Resources, Governance and Ecology, 10(1), 78–102. https://doi.org/10.1504/IJARGE.2014.061058
- Wheeler, S., Bjornlund, H., Shanahan, M., & Zuo, A. (2009). Who trades water allocations? Evidence of the characteristics of early adopters in the Goulburn-Murray Irrigation District, Australia 1998-1999*. Agricultural Economics, 40(6), 631–643. https://doi.org/10.1111/j.1574-0862.2009.00404.x
- Wickham, H. (2016). Ggplot2: Elegant graphics for data analysis. Springer Nature. https://doi.org/10.1007/978-3-319-24277-4
- Wiggins, S., & Keats, S. (2013). Smallholder agriculture’s contribution to better nutrition. In Report commissioned by the Hunger Alliance. Overseas Development Institute (ODI). https://odi.org/en/publications/smallholder-agricultures-contribution-to-better-nutrition/
- Zhong, W., Chen, Y., & Xie, L. (2023). How does internet use promote joint adoption of sustainable agricultural practices? Evidence from rice farmers in China. International Journal of Agricultural Sustainability, 21(1), 1–16. https://doi.org/10.1080/14735903.2023.2270244
Appendix
Robustness test for four probit models that consist the multivariate probit model are provided below.
Table A1. Estimates of the probit models.
Table A2. Estimates of the probit models.
Table A3. Estimates of the probit models.
Table A4. Estimates of the probit models.