ABSTRACT
In Uganda, farmers in arid and semi-arid areas lack sweetpotato seed after dry spells. This constrains the crop’s potential to contribute to food security. Triple S (sand, storage, sprouting) is a root-based technology addressing seed scarcity. Despite the technology’s efficacy, a systematic assessment of its demand has not been done. A study was undertaken to assess how Knowledge, Attitudes, Norms and Perceptions influence farmers uptake of the Triple S, using a structured questionnaire with 255 farmers stratified into users (n = 132) and non-users (n = 123). Sex-disaggregated focus groups were conducted with 40 farmers. The Theory of Planned Behavior and Technology Adoption Model were used to test hypotheses on Triple S adoption. Perceived Behavior Control, Perceived Usefulness and Knowledge positively predicted Behavior Intention (BI) only for non-users, while Attitude, Subjective Norms and Agency predicted BI for users. Gender Norms negatively influenced Agency for both groups. Gender-responsive adoption strategies may enhance technology uptake.
1. Introduction
In Uganda, the northern and eastern regions are the driest parts of the country, and also have the highest poverty and weakest food security status (Wichern et al., Citation2017). The dry seasons there have become longer and harsher, ravaging crop production and disrupting the already vulnerable food systems (Phiiri et al., Citation2016).
Sweet potato (Ipomoea batatas (L) Lam.) is a versatile, low-input root crop grown widely in sub-Saharan Africa (SSA). The crop is adaptable to drier areas with poor soils. It is easy to cultivate and matures quickly, bridging the hunger gap (Mwanga & Ssemakula, Citation2011). Sweet potato is nutritious, especially the orange-fleshed, beta-carotene-rich varieties, which provide Vitamin A (Hill & Johnson, Citation2012). So, sweet potato is suitable for areas with unreliable rainfall, and as an emergency response crop following weather disaster or other shocks (Heck et al., Citation2020).
In Uganda, sweet potato is the third most important staple crop (Mwanga & Ssemakula, Citation2011), produced mainly in the eastern and northern regions. The crop is grown for food and income, mostly by women, who also play an important role in seed conservation (McEwan et al., Citation2015). Therefore, sweet potato is a major contributor to food security (Obong et al., Citation2017). However, sweet potato seed is perishable, bulky, and has low multiplication rates, so seed is often unavailable (Gibson, Citation2013). In this paper, ‘seed’ is defined as vegetative planting material, especially sweet potato vines.
Several strategies have been developed to break the seed bottleneck, including the promotion of new improved varieties, and community seed multiplication and protection technologies such as net tunnels (Ssemakula et al., Citation2014). One of the seed conservation techniques that has attracted interest, especially in drought-prone areas, is Triple S (sand, storage, sprouting). Triple S is a climate-smart technology designed to help smallholder farmers in arid areas gain access to planting material earlier in the season, especially following a long dry period, where conventional conservation methods are less effective. Triple S involves storing quality sweet potato roots under layers of sand inside a container, such as a plastic tub, or a cardboard box (Namanda et al., Citation2011). Shortly before the rainy season, the roots are allowed to sprout, to plant in irrigated gardens near the homestead. The vines are watered, carefully tended to and are ready to plant in farmers’ open fields in just three weeks ().
Figure 1. The Triple S: storage in sand, sprouting and planting out. Explanatory drawing from extension literature for farmers. Source: Stathers et al., Citation2017.

Since its development between 2007 and 2011, Triple S has shown great potential to address seed scarcity (Parker et al., Citation2019; Hundayehu et al., Citation2022). Triple S is more convenient than collecting volunteer vines as planting material. Triple S makes vines available sooner after a long drought, and farmers can sell excess vines (Namanda et al., Citation2013). This technology has now been introduced to several countries where sweet potatoes are grown in the drylands, and seed is hard to get, for example, Malawi, Mozambique Nigeria, Ghana and Ethiopia (McEwan et al., Citation2022).
This paper is the first systematic assessment of farmers’ perceptions and intentions to use Triple S. Users’ perceptions have been documented (Namanda et al., Citation2013), but little is known about why farmers who were exposed to the technology did not adopt it. Such knowledge can help to refine Triple S and improve its uptake. In this study, we assess the influences on farmers’ intentions to use Triple S, especially the differences between technology users and non-users regarding:
How knowledge, attitude, perceptions, norms and agency affect their uptake of Triple S.
The extent to which adoption behavior predicts their implementation intention ().
How gender mediates psychosocial factors predicting implementation intention.
Figure 2. Theory of planned behavior and technology acceptance model (TPB-TAM) framework extended with knowledge, gender norms, agency and implementation intention (II).

The findings of this study will shed light on the adoption of other low-input innovations by smallholders elsewhere, especially women.
2. The context
2.1. Evolution of Triple S technology in SSA
Triple S was first trialed by farmers in northern and eastern Uganda, and was later validated in Meatu, an arid district in Tanzania, in 2010. In Tanzania, farmers who had previously had to source seed from over 100 km away could now produce vines at their homestead (Namanda et al., Citation2013). In Uganda, farmers made handsome profits by using Triple S to produce seed to sell to South Sudan (Namanda et al., Citation2011). These positive results later spurred the introduction of the technology to other African countries.
The sweet potato seed system in Uganda is largely informal. Over 90% of farmers depend on local networks for seed (Gibson et al., Citation2008). Given the long dry seasons (December to April), farmers find it hard to save seed. They depend on local multipliers, mostly women, who grow local sweet potato varieties during the dry season in small wetlands and sell vines to traders and smallholders at the start of the rainy season (Gibson, Citation2013).
However, rising temperatures and greater rainfall variability have played havoc with sweet potato productivity (Lunyolo et al., Citation2021). Smallholder farmers can only afford to buy a few vines (Gibson, Citation2013). So, they often depend on vines from volunteer crops which are not available until two months after the rains start. These vines often have a heavy load of viruses and insect pests, lowering the quantity and the quality of harvests. The late harvest also stretches out the hungry season, when granaries are empty (Namanda et al., Citation2011).
2.2. Agricultural technology adoption behavior
Previous assessments of sweet potato technology adoption focused on socio-economic determinants (Mwiti et al., Citation2020). Yet, psychosocial factors such as knowledge, perceptions and attitudes (KAPs) play a crucial role in technology adoption (Kamrath et al., Citation2018). KAPs are the first key steps to the adoption of new technologies by farmers (Ongachi et al., Citation2018). Inadequate knowledge, negative attitude and poor perception of technologies lead to low uptake (Musemakweri, Citation2007).
Ndwandwe (Citation2018) found that integrating scientific and local knowledge transformed perceptions and willingness to adopt pro-vitamin A fortified maize. Marechera and Ndigwa (Citation2014) highlight the importance of understanding farmers’ knowledge and perceptions in designing local solutions to agricultural challenges. This followed farmers dis-adoption of modern aflatoxin control technologies citing limited access to modern technologies, cost and safety concerns.
Attitudes are powerful predictors of behavior. A strong attitude is manifested by resistance to change, persistence and strong impact on thought and behavior (Levitan & Visser, Citation2008). Attitudes supporting adoption can be reinforced by good communication strategies tailored to the technology and farmer audience (Garforth et al., Citation2004). Cavane (Citation2016) found that extension agents can strengthen farmers’ attitudes towards new seed technologies through education campaigns and farm trials.
Farmer’s perceptions towards innovations and technologies are heterogenous, driven mostly by anticipated utility and benefits (Meijer et al., Citation2015). Perceptions are moderated by social capital, norms and gender (Ali & Yousuf, Citation2019). Mangheni et al. (Citation2019) posit that perceptions and the latent ability of smallholder farmers to mitigate climate-related effects in seed systems will be influenced by gender norms. Mudege et al. (Citation2017) found that gender and cultural norms mediate access to information, inadvertently impacting women’s ability to access training on innovations.
2.3. Theoretical framework
This study adapts and extends the combined theory of planned behavior and the technology acceptance model (TPB-TAM). According to the theory of planned behavior (TPB) attitude, subjective norms (SN) and perceived behavioral control (PBC) influence a person's behavioral intentions (BI). In other words, if you have a positive attitude towards a behavior valued by your social group, coupled with access to the required resources, you may form an intention to adopt the behavior, e.g. to stop smoking, recycle more, or adopt Triple S ().
Key elements of the TPB/TAM model:
Attitude: farmer’s attitudes towards Triple S were related to their beliefs and behaviors about sweet potato vine conservation. Farmers valuing timely access to seed should have a positive attitude towards Triple S.
Subjective norms: if local peers and opinion leaders encourage the adoption of Triple S, farmers will be more likely to use it.
Perceived behavioral control (PBC): farmers think they can use Triple S and have the resources to do so. Farmers’ can enhance their confidence in adopting Triple S (their PBC), through technical training, by acquiring the required inputs, and by belonging to a cohesive group.
Behavioral Intention: The readiness to promptly perform a behavior (Ajzen & Fishbein, Citation1975). BI stems from PBC (Ajzen, Citation2011). The stronger the intention, the higher the likelihood that farmers will adopt Triple S.
The technology acceptance model (TAM) was incorporated into the TPB to strengthen the prediction of attitude. In the TAM, attitude is explained by (i) perceived ease of use (PEOU), i.e. the potential users’ perception that a technology is easy to use and (ii) perceived usefulness (PU), i.e. potential users’ notion that the technology will improve their enterprise (Davis et al., Citation1989). PU has a direct and positive effect on BI. Based on the TPB-TAM, we derived the following hypothesis:
Hypothesis 1: Attitude, perceived usefulness (PU), subjective norms (SN) and perceived behavior control (PBC) influence the behavioral intention (BI) to use Triple S.
Tama et al. (Citation2021) added knowledge to the TPB. They found that knowledge had the highest total impact on farmers’ intention to keep using conservation agriculture. Ha et al. (Citation2023) also integrated knowledge into the TPB and found that the variable had a strong influence on adoption of intercropping technologies. Knowledge of Triple S includes (i) how to keep the roots healthy during storage and (ii) the value-added benefits of the technology. Our second hypothesis incorporates the notion of knowledge:
Hypothesis 2: Knowledge is positively related to behavioral intention (BI)
Agency is an individual’s capability to seize opportunities and take action to achieve their goals (Kabeer, Citation1999). Agency can be enabled or constrained by norms and social institutions (Hobson, Citation2011). Agency is gendered, with inequalities reinforced by norms governing the roles, responsibilities and expectations ascribed to women and men (Lawless et al., Citation2019). Our third hypothesis includes gender norms and agency:
Hypothesis 3: Agency influences behavioral intention (BI) positively, but is negatively mediated by gender norms.
Hypothesis 4: Behavioral intention (BI) is positively related to implementation intention (II)
Hypothesis 5: The gender of the farmer mediates the determinants predicting II.
3. Methods
3.1. Data and sampling procedure
Most of Uganda’s 10 agroecological regions experience bimodal rainfall and moderate temperatures, enabling consistent conservation of sweet potato vines. Triple S was first introduced in the Northern and Eastern regions, in the Northwestern and Northeastern Savannah grassland agroecological zones, where the long dry seasons inhibit vine conservation.
In the Northern region, the study was conducted in Gulu, Omoro and Kole districts. In the Eastern region, the study was conducted in Serere and Kumi districts (). Within each district, one parish was selected based on the number of active Triple S groups, i.e. Bungatira parish in Gulu, Bobi in Omoro, Aboke in Kole, Kyere in Serere and Kanyum in Kumi.
The study involved a questionnaire survey of farmers’ knowledge, attitudes and behaviors related to Triple S. The survey stratified 255 farmers into users of the technology (n = 132) and non-users (n = 123). Technology users were defined as farmers who had been trained and used the Triple S for at least two seasons. Non-users were farmers who had never used the technology and received little or no training on the Triple S. The study districts and parishes were purposively selected. Probability proportionate to size sampling was used to select households within a parish, i.e. larger samples were drawn from parishes with more Triple S users. After determining the sample size, respondents were sampled using the research randomizer, a web-based resource for generating random numbers. For the non-users, an equal number of respondents were also randomly sampled from the parishes. A structured questionnaire was developed, tested and used to collect data on household and farm assets, sweet potato production, knowledge and use of Triple S, yields of roots and of vines, and income. We also collected data on attitudes, perceptions, and gender dynamics relating to the use of sweet potato technologies.
To complement the survey, four sex-disaggregated focus group discussions (FGDs) targeting 20 users and 20 non-users were held. FGD respondents were purposively selected at the parish level with the support of local and farmer group leaders. Discussions were guided with a protocol to capture knowledge, attitudes and perceptions towards Triple S. The study was conducted from December 2018 through January 2019.
3.2. Empirical methods
3.2.1. Measurement of psychosocial constructs
We used a five-point Likert scale (strongly agree = 5; strongly disagree = 1) for most statements, except for ‘I will make an effort to use the Triple S.’ Here we used: (5) This coming season, (4) The second coming season, (3) A year from now, (2) Not soon and (1) Never.
3.2.2. Data analysis
We tested hypotheses H1 – H4 using structural equation modelling (SEM) in SPSS_AMOS Graphics 24 because of its suitability to assess causal relationships between multiple explanatory and response variables. Assessment of goodness of fit included comparative fit index (CFI), Tucker-Lewis index (TLI), root mean squared error of approximation (RMSEA), and standardized root mean squared residual (SRMSR). These indices are superior in detecting model misspecification and have independence from sample size. Measures of CFI > .90, TLI > .90, RMSEA < 0.08, and SRMSR < .08 indicate a good fit (Hu & Bentler, Citation1999). The content validity and internal consistency aligned with the recommended cut-off representing acceptable reliability (). Composite reliability, a measure of internal consistency, values were above the acceptable threshold for exploratory research (0.70), except for attitude; however, its Cronbach's alpha was above 0.70.
Table 1. Content validity and internal consistency of the model constructs.
The average variance extracted (AVE) was above 0.5 for all constructs, indicating that convergent validity was established. The standardized loadings for most constructs were above 0.5, and confirmatory factor analysis of the measurement model was satisfactory with CFI of 0.908, SRMR = 0.072, RMSEA = 0.047, TLI = 0.90 and p = 0.795 implying strong construct validity.
Since we conducted a multi-group analysis between the technology users and non-users, we also tested for model invariance (Reise et al., Citation1993). First, an unconstrained model was tested simultaneously on both groups. Then, the fit of a constrained model was tested, with all parameters set equal across the groups. The model was considered invariant: the difference in the χ2 (966.1, p = 1.000) of the unconstrained and constrained models was statistically insignificant. Constraining the model’s parameters to be equal across the two groups did not significantly worsen the model’s fit. Finally, we ran standardized residuals and modification indices diagnostics to check for potential improvements to the model or specific problems not detected by previous steps (Hair et al., Citation2009).
Estimating an extended TPB-TAM framework while controlling for the influence of gender norms on BI through agency and knowledge on BI yielded an acceptable fit of the SEM model. For the full sample model, CFI was = 0.942, SRMR = 0.095, RMSEA = 0.039, TLI = 0.933 and p = 1.000; all indicative of a good fit. Further, the multi-group model testing difference between users and non-users fell in line with a CFI of .914; TLI = .93, SRMR = 0.089, RMSEA 0.045 p = 0.949.
To assess how the gender of farmers mediates determinants influencing II, we applied the probabilistic conditional binary recursive inference algorithm. The probabilistic inference relates to conditional probability distributions between the dependent variable and the set of explanatory variables. The algorithm performs successive binary splits of the data set, leading to the segmentation of participants into groups (nodes) characterized by the gender-specific relevant explanatory variables. A 10% significance level was chosen with Bonferroni correction and a minimum sub-sample size set to 50 participants. The estimation was done using the R-package ‘Party’ (Zeileis et al., Citation2008) in R software version 4.2.1.
4. Results
4.1. Demographics of participants
The average age of the survey respondents was 40.9 (). Most respondents were in monogamous relationships (67.5%) and defined themselves as household heads (52.5%). Women had received an average of six years of education, while men had nine years. Low education, especially for women, could limit their ability to learn new technologies. The sampled farmers knew the sweet potato crop well. They had grown sweet potatoes for an average of 17 years: 18.1 years for users and 16.1 for non-users. At least 74% of the respondents were members of farmers’ groups.
Table 2. Socio-economic characteristics of survey respondents.
The adoption of Triple S is linked closely to income. Total household income was nearly twice as high for Triple S users than for non-users, while income from the sale of roots and vines was over four times higher for the users of Triple S. This is probably because new agricultural technologies like Triple S are introduced to farmers in organized groups. Organized farmers tend to be early adopters, apply good agriculture practices, and are usually better off. Triple S users had significantly shorter travel distances to extension services than non-users, which could favor access to agricultural knowledge and technologies. Respondents in the northern region had better access to vine multipliers (p = 0.12) and group meeting places (p = 0.013) than those in the eastern region. This could be attributed to the entry of agricultural projects aiming to rehabilitate northern Uganda following the 20-year long civil strife with the Lord’s Resistance Army, ending in 2006.
Summary statistics for the psychosocial constructs and the Chi-square test were done to assess differences in rating amongst the groups. Most farmers reported that they intended to use Triple S. They had high scores for implementation intention (II), the final outcome of all the other variables. II was the highest-rated variable for non-users (3.97), while average scores of II were higher users (4.84) and the full sample (4.42) (p = 0.000). Triple S users had significantly higher scores for the TPB-TAM framework variables than the non-users (p = 0.000) ().
Table 3. Summary statistics of model constructs.
The FGDs revealed that users generally had more positive attitudes about Triple S. In Kumi (a district in the Eastern region), women and men users perceived the technology as being useful and simple to apply. However, men non-users in Serere noted that the technology would be difficult to implement without training, while women non-users mentioned that traditional vine conservation methods were sufficient. The male users in Kumi were commercial seed producers. They criticized Triple S’s low vine output, observing that it would not create value for commercial root producers.
Knowledge and gender norms were not significantly different among the two groups. Triple S knowledge was initially obtained from research and development (R&D) personnel and cascaded down by extension agents and trained farmers, but R&D sources were most valued. In FGDs, users elaborated the knowledge needed to apply the Triple S. This included selecting healthy roots, good soil texture, lining storage containers, positioning roots in adjacent layers, sprouting and planting. Women in Omoro, however, noted that setting up a Triple S storage required time to master. Thus, the need for refresher training targeting newly recruited group members.
We observed a subtle change in gendered roles in production. Following training, men users began to conserve vines, previously a women’s responsibility. However, while women sold Triple S vines, men controlled the proceeds, as emphasized below:
We are ‘the marketing managers’ and decision makers. This is ingrained from childhood – boys are told by their mothers that they own everything. The man must be in charge. Only the kitchen is for women. Even your wife’s degree belongs to you.
Men Triple S users,-FGD respondents, Kyere, Serere.
There was a significant difference in agency between users and non-users. Women users were able to experiment and compare the performance of Triple S vines with locally conserved vines. In Kole district, in the Northern region, women users collaborated to obtain Triple S inputs (e.g. basins and roots for storage) as a group. This enabled them to obtain vines for timely planting, early harvests and income from vine sales. Consequently, some members were able to apply Triple S individually.
4.2. Farmers behavioral intention towards using Triple S
As predicted by the theory of planned behavior (TPB): perceived behavioral change (PBC), attitude, and social norms jointly and significantly predicted behavioral intention (BI) to use Triple S, for users and non-users. The Wald test of the joint hypothesis yielded significant statistics (p < 0.001), 130.78 and 81.65 for users and non-users, respectively. The squared multiple correlations for intention for users and non-users models were 0.61 and 0.86, respectively, implying all research variables adequately accounted for the total variance.
The technology acceptance model (TAM) variables, perceived usefulness (PU) and perceived ease of use (PEOU), jointly and positively influenced attitude. The Wald joint test generated significant statistics (p < 0.001), 115.34 and 60.66 for users and non-users, respectively. Further, PU and PEOU jointly explained 60.8% and 72.4% of the variance in farmers’ attitudes for users and non-users, respectively. FGDs showed how a positive attitude towards the Triple S was shaped:
We planted vines from Triple S and volunteer crops and observed that the volunteer crop vines take longer to mature. The roots from Triple S were larger.
Respondents, women’s FGD, Kumi
Testing revealed mixed results for H1 (attitude, PU, social norms and PBC individually and positively are related to BI) (). Perceived behavioral control (PBC) significantly and positively predicted BI only for non-users, where a unit change in PBC increases BI by 47%. To explain this, Triple S users elaborated some of the difficulties experienced with the technology. Men users in Omoro noted that limited training and extension services curtailed diffusion of Triple S. Roots stored in sand require frequent monitoring, which farmers were not used to. In Kumi, women users highlighted that inaccessibility to the required resources was a barrier to applying Triple S.
Figure 4. Theory of planned behavior and technology acceptance model (TPB-TAM) extended framework showing results of the multi-group analysis.
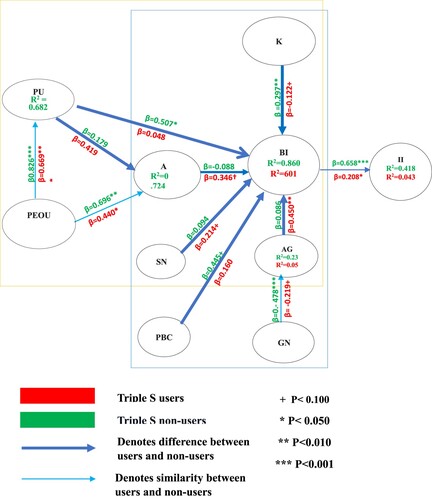
Social norms and attitude significantly and positively predicted behavioral intentions (BI) only for users, increasing BI by 22.1% and 34%, respectively. Women who produced more sweet potato said that they were held in high regard by community leaders. This inspired others to try the Triple S:
People noticed our early crop and asked US how we do it. They also noticed we were the only ones offering vines for sale. They got interested and are now using Triple S
Women FGD respondents, Kumi
We accept H2 (knowledge is positively related to BI) only for non-users, where knowledge increases BI by 29%. For users, knowledge actually influenced BI negatively. Users mentioned that more men than women attended Triple S training because they were held in distant locations. Thus, men learned more about Triple S, even though women would benefit more from the technology given that they are responsible for sourcing seed. Women highlighted Triple S related information gaps. For example, the women wondered why mostly newspapers were used to line the storage containers, and whether locally sourced materials could be used instead. Such information gaps could have contributed to the negative influence of knowledge on BI.
H3 (agency influences BI positively but is negatively mediated by gender norms) was partially supported. Gender norms significantly and negatively affected agency. A unit increase in restrictive gender norms, as measured by a one-point increase on the Likert scale, lowers agency by 47.8% for non-users and 22.5% for users. Gender norms restrict farmers’ agency and their BI.
Agency and BI were related, but only for users, where a unit change in agency resulted in a 22% increase in BI: partially supporting the first part of H3. This is probably because only the users attended trainings and participated in groups, which strengthened their agency, as explained below:
At first, I thought I couldn’t apply the Triple S. The process seemed very difficult. My family was against it, saying I would forget our traditional conservation practices. Despite this, I gave it a try. Surprisingly, I got a lot of clean vines and sold the excess. When you are determined, you succeed.
Woman farmer, Omoro district.
4.3. Farmers’ gender is correlated with II
To test H5, we estimated a probabilistic recursive regression model to assess which determinants of II were significantly mediated by farmers’ gender. With control for user groups, respondents’ gender split the sample into four groups; therefore, H5 is accepted. BI, PU and PBC were important in explaining gender differences and defining the group partition ().
Group one is Node 2 (n = 34): farmers with the lowest II and BI scores. Men had slightly lower II scores than women. None of the farmers in this node are likely to try Triple S, saying they will use it ‘not soon.’
Group two is Node 4 (n = 49). These farmers had above average BI scores of >3, but low PU scores of ≤ 3.77. Women’s average II scores were lower than men’s. Women responded that they intended to use Triple S ‘a year from now,’ while the men intended to implement Triple S sooner, in ‘the coming season (the next planting season).’ Recalling the FGDs, these were the men who were starting to develop an interest in vine conservation following exposure to Triple S.
Farmers in group three – Node 6 (n = 32). This group had higher PU scores >3.667, and a slightly above average PBC score of ≤ 3.33. Men and women in this group were early adopters, and both had high II scores. Women in this group had high implementation intention (II) scores. These women were organized in farmers’ groups, which gave them access to the resources needed to implement Triple S. Having the resources to adopt a behavior increases one’s perceived behavioral control (PBC). These women also perceived Triple S to be useful (high PU). Women users in the FGD in Koro village in Omoro district, Northern region, said that Triple S was easier than traditional vine conservation, which required more labor, time and expense to protect vines from foraging livestock.
Group four, i.e. Node 7 was the largest group of farmers (n = 140), with the highest II, those who intended to use Triple S in the ‘coming season.’ Women and men in this node all had high II scores, influenced by the highest scores for BI, PU and PBC. Farmers in this category had a high perception of the usefulness of Triple S and thought that they could easily obtain the resources needed to use it as evidenced by high PBC scores.
5. Discussion
This study provided an understanding of farmers’ intention to adopt a climate-smart technology to improve sweet potato seed access in arid and semi-arid areas. By extending the TPB-TAM framework with knowledge, gender norms, agency and II, we explained the difference in psychosocial factors driving intention to adopt the Triple S among users and non-users. The extended TBP-TAM framework was parsimonious and adequate in explaining farmers’ intention to use Triple S, evidenced by the adequacy of RMSEA.
The TPB constructs jointly and positively influenced farmers intention to use Triple S. However, the individual effects of the constructs showed mixed results. For non-users, PBC had a significant positive effect on their BI. Their perception that they had the capability to establish Triple S storage strengthened their BI.
The relationship between PBC and BI was insignificant for users. This was surprising since men-users indicated individual ability to obtain the inputs needed for Triple S. Also, collective action enabled women users to acquire these resources. However, farmers may be wary of free riders (Di Falco & Bulte, Citation2013), neighbors and relations who would want to be given Triple S vines for free, thus dampening users’ PBC.
The effect of social norms on BI was only supported for users, suggesting peers and opinion leaders mediated the uptake of Triple S. Martínez-García et al. (Citation2013) note that farmers value their leaders’ and peer’s opinion before committing to new practices or values. Also, Buyinza et al. (Citation2022) argued that a lack of social pressure limited farmers’ intention to adopt agroforestry.
Attitude was the strongest predictor of BI (34.6%) for users, contrary to studies using TPB alone, further supporting the extension with TAM (Ajzen, Citation2020). The TPB-TAM framework also resulted in PU being the strongest predictor of BI (50.7%) for non-users, a reflection of the Triple S advantages as seen by the non-users.
Contrary to our expectations, knowledge was negatively related to BI for the users. Knowledge was measured to capture training and extension support for Triple S. The limited access to training opportunities for women users and identified knowledge gaps probably contributed to this negative relationship. Elsewhere, Kansiime et al. (Citation2022) have recommended that gender responsive extension approaches should be adopted to enhance learning and participation of all gender categories.
Gender norms negatively influenced agency, as anticipated. Negative stereotypes of ‘illiterate, ignorant’ women can stymy their participation in training and technology adoption (Mudege et al., Citation2017). Women’s limited education, lower membership in agricultural organizations and limited social capital reduce their agency (Rola-Rubzen et al., Citation2020). Sweet potato is seen as a woman’s crop in Uganda, but new technologies and increased agricultural extension may signal its impending commercialization. This would attract men who could displace women from profitable nodes of the chain and may call for re-negotiation of gender norms and relations in a bid to promote individual agency (Lawless et al., Citation2019).
Agency was a significant predictor of BI only for users who were organized in farmer groups. As women explained in the FGDs, they surmounted technology-input challenges that habitually affect women (Badstue et al., Citation2020) to benefit from Triple S. Women took up leadership positions which strengthened their negotiation ability and augmented individual agency (Mayanja et al., Citation2022). Local leaders applauded women users because they had food in times of scarcity, with surplus to sell. This social recognition helped to elevate their status in the community. Thus, collective agency played a role in countering the negative effects of gender norms.
BI was a stronger predictor of II for non-users, but weak for users. This is similar to where conventional farmers expressed higher intention than organic producers to adopt organic farming practices (Cakirli Akyüz & Theuvsen, Citation2020). Nonetheless, a weak BI-II relationship does not diminish II’s utility in reducing the intention-behavior gap. After all, people who already practice a behavior have less need for embracing it.
BI, PU and PBC accounted for the observed gender differences in II in descending order of importance. Men dominated node 4 where BI and PU were strong predictors of II. Even though sweet potato was perceived as a woman’s crop, these men were eager to adopt Triple S, as in Kenya, where men displaced women following commercialization of the banana value chain (Nyabaro et al., Citation2019). PBC, PU and BI were stronger predictors of II for farmers in Node 6, especially women who were able to catch up with men. This increase in women’s II could be aligned to PBC stemming from collective input resources. This aligns with Venkatesh et al. (Citation2000) who argue that men and women have different decision processes in adopting new technologies. Women still need support to access resources required to adopt new technologies, which should be taken into account for equitable technology dissemination (Rola-Rubzen et al., Citation2020).
6. Conclusions
Farmers may accept a technology if they see it as useful, and if they have the knowledge and resources to implement it. Our study assessed the psychosocial influences on sweet potato farmers’ intentions to use Triple S. For non-users, uptake and sustained use will be propelled by extension work to give farmers positive attitudes and more knowledge about Triple S. Farmers also need certain inputs to use Triple S, besides perceiving the technology as useful. Gender norms may be altered, e.g. empowering women to attend trainings, manage their own produce, and decide how to use money earned from sales.
For Triple S users, belonging to a farmers’ group and being recognized as high performers by community leaders will encourage more women farmers to consistently use the technology. Policy-makers and technocrats could provide early adopters with social incentives to attract other farmers. It is also important to continuously improve Triple S to align it with farmers’ local resource availability (e.g. substitute for newspapers). The technology could be promoted along with training on household seed enterprises, with male and female family members attending together. Transforming sweet potato into a priority crop will improve farmers’ attitudes, although it may also induce men to hijack sweet potato farming.
Since the Triple S requires some basic knowledge to use, training will improve attitudes and knowledge. The better off farmers, and the ones organized in groups, are more likely to use Triple S; these farmers are often targeted by extension agents. Larger-scale farmers who are more committed to growing sweet potatoes also have more resources, more access to information, and are more open to new ideas to boost their production.
Ethics declarations
This article is based on research that was undertaken in 2018–2019 under a study titled ‘Effect of the Triple S technology on farmers food security in northern and eastern Uganda. The study was registered and obtained ethical the St Francis Hospital Nsambya Research and Ethical Committee; Uganda; vide SFHN-2021-26.
Informed and signed consent was obtained from all individual farmers who participated in the study. Accordingly, all information that could potentially identify the participants has been removed and responses anonymized.
I warrant our manuscript is original work and has not been accepted for publication by another journal or periodical. I further warrant that our work (including figures, tables and other illustrative materials) does not infringe on the copyright or statutory rights of others and does not contain libelous statements.
Author contribution
Sarah Mayanja: Conceptualization (lead), Investigation (lead) Methodology (equal) Formal analysis (lead), Writing – original draft (lead), Writing – review and editing (lead) Software (equal), Janet Mwende Formal analysis (equal), Writing original draft (equal), Writing – review and editing (lead) Software (lead) Norman Kwikiriza Investigation (equal) Methodology (equal) Formal analysis (supporting), Writing – review and editing (supporting) Guy Hareau Funding acquisition (lead); Project administration (lead); Supervision (supporting); Writing-review & editing (supporting). Joseph Ssekandi Conceptualization (supporting); Supervision (equal), Writing-review & editing (supporting). Julius Okello Conceptualization (supporting); Methodology (equal) Funding acquisition (lead); Project administration (lead); Supervision (equal); Writing-review & editing (supporting).
Biosketches.docx
Download MS Word (22.6 KB)Acknowledgements
This research was undertaken as part of the CGIAR Research Program on Roots, Tubers and Bananas (RTB) and supported by CGIAR Trust Fund contributors http://www.cgiar.org/funders/ We are grateful to the sweetpotato farmers in the study communities who participated in this study. We also appreciate our research team for their commitment in data collection and collation. Special thanks go to the staff of World Vision and local leaders in the study districts for their support in mobilizing the respondents. Jeffery Bentley read and commented on a previous version of this paper.
Disclosure statement
No potential conflict of interest was reported by the author(s).
Data availability statement
The data that support the findings of this study are available from the International Potato Center (CIP) but restrictions may apply to the availability of these data, which were used under licence for the current study, and so are not publicly available. Data are however available from the authors upon reasonable request and with permission of CIP.
Additional information
Funding
References
- Ajzen, I. (2011). The theory of planned behaviour: Reactions and reflections. Psychology & Health, 26(9), 1113–1127. https://doi.org/10.1080/08870446.2011.613995
- Ajzen, I. (2020). The theory of planned behavior: Frequently asked questions. Human Behavior and Emerging Technologies, 2(4), 314–324. https://doi.org/10.1002/hbe2.195
- Ajzen, I., Czasch, C., & Flood, M. G. (2009). From intentions to behavior: Implementation intention, commitment, and conscientiousness. Journal of Applied Social Psychology, 39(6), 1356–1372. https://doi.org/10.1111/j.1559-1816.2009.00485.x
- Ajzen, I., & Fishbein, M. (1975). A Bayesian analysis of attribution processes. Psychological Bulletin, 82(2), 261. https://doi.org/10.1037/h0076477
- Ali, A., & Yousuf, S. (2019). Social capital and entrepreneurial intention: Empirical evidence from rural community of Pakistan. Journal of Global Entrepreneurship Research, 9(1), 64. https://doi.org/10.1186/s40497-019-0193-z
- Badstue, L., Eerdewijk, A. V., Danielsen, K., Hailemariam, M., & Mukewa, E. (2020). How local gender norms and intra-household dynamics shape women’s demand for labor-saving technologies: Insights from maize-based livelihoods in Ethiopia and Kenya. Gender, Technology and Development, 24(3), 341–361. https://doi.org/10.1080/09718524.2020.1830339
- Buyinza, J., Nuberg, I., Muthuri, C., & Denton, M. D. (2022). Psychological factors influencing farmers’ intention to adopt agroforestry: A structural equation modeling approach. Journal of Sustainable Forestry, 39(6), 1–12.
- Cakirli Akyüz, N., & Theuvsen, L. (2020). The impact of behavioral drivers on adoption of sustainable agricultural practices: The case of organic farming in Turkey. Sustainability, 12(17), 6875. https://doi.org/10.3390/su12176875
- Cavane, E. (2016). Farmers’ attitude and adoption of improved maize varieties and chemical fertilizers in Mozambique. Indian Research Journal of Extension Education, 11(1), 1–6.
- Cislaghi, B., & Heise, L. (2020). Gender norms and social norms: Differences, similarities and why they matter in prevention science. Sociology of Health & Illness, 42(2), 407–422. https://doi.org/10.1111/1467-9566.13008
- Davis, F. D., Bagozzi, R. P., & Warshaw, P. R. (1989). User acceptance of computer technology: A comparison of two theoretical models. Management Science, 35(8), 982–1003. https://doi.org/10.1287/mnsc.35.8.982
- DeBiasse, M. A., Bowen, D. J., Pagoto, S. L., Massaro, J. M., Istfan, N., & Quintiliani, L. M. (2017). Pilot and feasibility test of an implementation intention intervention to improve fruit and vegetable intake Among women with low socioeconomic status. Journal of Health Disparities Research and Practice, 10(2), 10.
- Diaz, I. I., Najjar, D., & Baruah, B. (2017). A review of gender norms, agency and the adoption of agricultural innovations. International Center for Agricultural Research in the Dry Areas (ICARDA). https://hdl.handle.net/20.500.11766/7667.
- Di Falco, S., & Bulte, E. (2013). The impact of kinship networks on the adoption of risk-mitigating strategies in Ethiopia. World Development, 43, 100–110. https://doi.org/10.1016/j.worlddev.2012.10.011
- Garforth, C., Rehman, T., McKemey, K., Tranter, R., Cooke, R., Yates, C., Park, J., & Dorward, P. (2004). Improving the design of knowledge transfer strategies by understanding farmer attitudes and behaviour. Journal of Farm Management, 12(1), 17–32.
- Gibson, R. (2013). How sweet potato varieties are distributed in Uganda: Actors, constraints and opportunities. Food Security, 5(6), 781–791. https://doi.org/10.1007/s12571-013-0302-8
- Gibson, R. W., Byamukama, E., Mpembe, I., Kayongo, J., & Mwanga, R. O. (2008). Working with farmer groups in Uganda to develop new sweet potato cultivars. Decentralisation and Building on Traditional Approaches.” Euphytica, 159(1), 217–228.
- Ha, T. M., Manevska-Tasevska, G., Jäck, O., Weih, M., & Hansson, H. (2023). Farmers’ intention towards intercropping adoption: The role of socioeconomic and behavioural drivers. International Journal of Agricultural Sustainability, 21(1), 2270222. https://doi.org/10.1080/14735903.2023.2270222
- Hair, J. F., Black, W. C., Babin, B. J., & Anderson, R. E. (2009). Multivariate data analysis. In J. F. Hair (Ed.), In multivariate data analysis (pp. 785–785). Pearson Publication.
- Heck, S., Campos, H., Barker, I., Okello, J. J., Baral, A., Boy, E., Brown, L., & Birol, E. (2020). Resilient agri-food systems for nutrition amidst COVID-19: Evidence and lessons from food-based approaches to overcome micronutrient deficiency and rebuild livelihoods after crises. Food Security, 12(4), 823–830. https://doi.org/10.1007/s12571-020-01067-2
- Hill, G. E., & Johnson, J. D. (2012). The vitamin A–redox hypothesis: A biochemical basis for honest signaling via carotenoid pigmentation. The American Naturalist, 180(5), E127–E150. https://doi.org/10.1086/667861
- Hobson, B. (2011). The agency gap in work-life balance: Applying Sen's capabilities framework within European contexts. Social Politics: International Studies in Gender, State & Society, 18(2), 147–167. https://doi.org/10.1093/sp/jxr012
- Hu, L. T., & Bentler, P. M. (1999). Cutoff criteria for fit indexes in covariance structure analysis: Conventional criteria versus new alternatives. Structural Equation Modeling: A Multidisciplinary Journal, 6(1), 1–55. https://doi.org/10.1080/10705519909540118
- Hundayehu, M. C., McEwan, M., Namanda, S., Low, J. W., Vandamme, E., & Brouwer, R. (2022). Participatory validation and optimization of the Triple S method for sweetpotato planting material conservation in southern Ethiopia. Open Agriculture, 7(1), 120–131. https://doi.org/10.1515/opag-2021-0063
- Kabeer, N. (1999). Resources, agency, achievements: Reflections on the measurement of women's empowerment. Development and Change, 30(3), 435–464. https://doi.org/10.1111/1467-7660.00125
- Kamrath, C., Rajendran, S., Nenguwo, N., Afari-Sefa, V., & Bröring, S. (2018). Adoption behavior of market traders: An analysis based on technology acceptance model and theory of planned behavior. International Food and Agribusiness Management Review, 21(6), 771–790. https://doi.org/10.22434/IFAMR2017.0043
- Kansiime, M. K., Njunge, R., Okuku, I., Baars, E., Alokit, C., Duah, S., Gakuo, S., et al. (2022). Bringing sustainable agricultural intensification practices and technologies to scale through campaign-based extension approaches: Lessons from Africa Soil Health Consortium. International Journal of Agricultural Sustainability, 20(5), 743–757. https://doi.org/10.1080/14735903.2021.1976495
- Lawless, S., Cohen, P., McDougall, C., Orirana, G., Siota, F., & Doyle, K. (2019). Gender norms and relations: Implications for agency in coastal livelihoods. Maritime Studies, 18(3), 347–358. https://doi.org/10.1007/s40152-019-00147-0
- Levitan, L. C., & Visser, P. S. (2008). The impact of the social context on resistance to persuasion: Effortful versus effortless responses to counter-attitudinal information. Journal of Experimental Social Psychology, 44(3), 640–649. https://doi.org/10.1016/j.jesp.2007.03.004
- Lunyolo, L. D., Khalifa, M., & Ribbe, L. (2021). Assessing the interaction of land cover/land use dynamics, climate extremes and food systems in Uganda. Science of the Total Environment, 753, 142549. https://doi.org/10.1016/j.scitotenv.2020.142549
- Mangheni, M. N., Tufan, H. A., Nkengla, L., Aman, B. O., & Boonabaana, B. (2019). Gender norms, technology access, and women farmers’ vulnerability to climate change in Sub-saharan Africa. In Y. Bamutaze, S. Kyamanywa, B. R. Singh, G. Nabanoga, & R. Lal (Eds.), In agriculture and ecosystem resilience in Sub saharan Africa: Livelihood pathways under changing climate (pp. 715–728). Springer.
- Marechera, G., & Ndigwa, J. (2014). Farmer perceptions of aflatoxin management strategies in lower eastern Kenya. Journal of Agricultural Extension and Rural Development, 6(1-2), 382–392.
- Martínez-García, C. G., Dorward, P., & Rehman, T. (2013). Factors influencing adoption of improved grassland management by small-scale dairy farmers in central Mexico and the implications for future research on smallholder adoption in developing countries. Livestock Science, 152(2-3), 228–238. https://doi.org/10.1016/j.livsci.2012.10.007
- Mayanja, S., Mudege, N., Snyder, K. A., Kwikiriza, N., Munda, E., Achora, J., & Grant, F. (2022). Commercialisation of the sweetpotato value chain: Impacts on women producers in Mozambique. Outlook on Agriculture, 51(3), 349–358. https://doi.org/10.1177/00307270221105533
- McEwan, M., Almekinders, C., Abidin, P. E., Andrade, M., Carey, E. E., Gibson, R. W., Naico, A., Namanda, S., & Schulz, S. (2015). Can small still be beautiful? Moving local sweet potato seed systems to scale in Sub-saharan Africa. In C. Almekinders, & P. E. Abidin (Eds.), Potato and sweet potato in Africa. Transforming value chains for food and nutrition security (pp. 289–310). CABI.
- McEwan, M. A., van Mourik, T. A., Hundayehu, M. C., Asfaw, F., Namanda, S., Suleman, I., Mayanja, S., Imoro, S., & Etwire, P. M. (2022). Securing sweet potato planting material for farmers in dryland Africa: Gender-responsive communication approaches to scale triple S. In G. Thiele, M. Friedmann, H. Campos, V. Polar, & J. W. Bentley (Eds.), In root, tuber and banana food system innovations (pp. 353–388). Springer.
- Meijer, S. S., Catacutan, D., Ajayi, O. C., Sileshi, G. W., & Nieuwenhuis, M. (2015). The role of knowledge, attitudes and perceptions in the uptake of agricultural and agroforestry innovations among smallholder farmers in Sub-saharan Africa. International Journal of Agricultural Sustainability, 13(1), 40–54. https://doi.org/10.1080/14735903.2014.912493
- Mudege, N. N., Mdege, N., Abidin, P. E., & Bhatasara, S. (2017). The role of gender norms in access to agricultural training in chikwawa and phalombe, Malawi. Gender, Place & Culture, 24(12), 1689–1710. https://doi.org/10.1080/0966369X.2017.1383363
- Musemakweri, J. (2007). Farmers’ experiences and perceptions of the NAADS agricultural extension system/programin kabale district, Uganda. Retrospective theses and dissertations available at digital repository @ Iowa State University.
- Mwanga, R. O., & Ssemakula, G. (2011). Orange-fleshed sweetpotatoes for food, health and wealth in Uganda. International Journal of Agricultural Sustainability, 9(1), 42–49. https://doi.org/10.3763/ijas.2010.0546
- Mwiti, F. K., Okello, J. J., Munei, K., & Low, J. (2020). Farmer demand for clean planting material of biofortified and non-biofortified vegetatively propagated crop varieties: The case of sweetpotato. Scientific African, 8, e00400. https://doi.org/10.1016/j.sciaf.2020.e00400
- Namanda, S., Amour, R., & Gibson, R. W. (2013). The triple S method of producing sweet potato planting material for areas in Africa with long dry seasons. Journal of Crop Improvement, 27(1), 67–84. https://doi.org/10.1080/15427528.2012.727376
- Namanda, S., Gibson, R., & Sindi, K. (2011). Sweetpotato seed systems in Uganda, Tanzania, and Rwanda. Journal of Sustainable Agriculture, 35(8), 870–884. https://doi.org/10.1080/10440046.2011.590572
- Ndwandwe, N. K. (2018). Sensory quality of provitamin A biofortified maize-based foods and the effect of a provitamin A biofortified maize awareness campaign on their acceptance in KwaZulu-natal, South Africa. PhD dissertation. University of KwaZulu-Natal.
- Nyabaro, V., Mburu, J., & Hutchinson, M. (2019). Factors enabling the participation of women in income sharing among banana (musa spp.) producing households in South Imenti, Meru County, Kenya. Gender, Technology and Development, 23(3), 277–292. https://doi.org/10.1080/09718524.2019.1669104
- Obong, Y., Omony, T., Rachkara, P., & Gibson, R. W. (2017). Disseminating modern sweet potato varieties using participatory variety demonstration trials on informal nodal multipliers’ fields at hub locations. Journal of Crop Improvement, 31(2), 229–246.
- Ongachi, W., Onwonga, R., Nyanganga, H., Wangia, S., Chimoita, E., & Okry, F. (2018). Farmers’ knowledge, attitude, and perception of video-mediated learning vis-à-vis farmer field school on striga weed management in western Kenya. International Journal of Education and Development Using Information and Communication Technology (IJEDICT), 14(2), 195–210.
- Parker, M. L., Low, J. W., Andrade, M., Schulte-Geldermann, E., & Andrade-Piedra, J. (2019). The climate-smart agriculture papers. The Climate-Smart Agriculture Papers: Investigating the Business of a Productive, Resilient and Low Emission Future, 99–111. https://doi.org/10.1007/978-3-319-92798-5_9
- Phiiri, G. K., Egeru, A., & Ekwamu, A. (2016). Climate change and agriculture nexus in Sub-saharan Africa: The agonizing reality for smallholder farmers. International Journal of Current Research and Review, 8(2), 57.
- Reise, S. P., Widaman, K. F., & Pugh, R. H. (1993). Confirmatory factor analysis and item response theory: Two approaches for exploring measurement invariance. Psychological Bulletin, 114(3), 552. https://doi.org/10.1037/0033-2909.114.3.552
- Rola-Rubzen, M. F., Paris, T., Hawkins, J., & Sapkota, B. (2020). Improving gender participation in agricultural technology adoption in Asia: From rhetoric to practical action. Applied Economic Perspectives and Policy, 42(1), 113–125. https://doi.org/10.1002/aepp.13011
- Ssemakula, G., Niringiye, C., Otema, M., Kyalo, G., Namakula, J., & Mwanga, R. O. M. (2014). Evaluation and delivery of disease-resistant and micronutrient dense sweet potato varieties to farmers in Uganda. Uganda Journal of Agricultural Sciences, 15(2), 101–111.
- Stathers, T., Namanda, S., Agili, S., Cherinet, M., Njoku, J., & McEwan, M. (2017). Guide for trainers – sweet potato planting material conservation triple S method: Sand, storage, sprouting (p. 42). International Potato Centre, Nairobi, Kenya.
- Tama, R. A. Z., Ying, L., Yu, M., Hoque, M. M., Adnan, K. M., & Sarker, S. A. (2021). Assessing farmers’ intention towards conservation agriculture by using the extended theory of planned behavior. Journal of Environmental Management, 280, 111654. https://doi.org/10.1016/j.jenvman.2020.111654
- van Dijk, W. F., Lokhorst, A. M., Berendse, F., & De Snoo, G. R. (2016). Factors affecting farmers' intention to perform unsubsidised agri-environmental measure. Land Use Policy, 59, 207–216.
- Venkatesh, V., Morris, M. G., & Ackerman, P. L. (2000). A longitudinal field investigation of gender differences in individual technology adoption decision-making processes. Organizational Behavior and Human Decision Processes, 83(1), 33–60. https://doi.org/10.1006/obhd.2000.2896
- Villamor, G. B., van Noordwijk, M., Djanibekov, U., Chiong-Javier, M. E., & Catacutan, D. (2014). Gender differences in land-use decisions: Shaping multifunctional landscapes? Current Opinion in Environmental Sustainability, 6, 128–133. https://doi.org/10.1016/j.cosust.2013.11.015
- Wichern, J., van Wijk, M. T., Descheemaeker, K., Frelat, R., van Asten, P. J., & Giller, K. E. (2017). Food availability and livelihood strategies among rural households across Uganda. Food Security, 9(6), 1385–1403. https://doi.org/10.1007/s12571-017-0732-9
- Zeileis, A., Hothorn, T., & Hornik, K. (2008). Model-based recursive partitioning. Journal of Computational and Graphical Statistics, 17(2), 492–514. doi:10.1198/106186008X319331