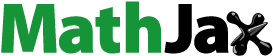
ABSTRACT
Sustainable food systems require mechanisms that assure consumers about the sustainability of agricultural production. Building on the existing literature on the impact of sustainability standards, this study is the first to assess the effectiveness of participatory guarantee systems (PGS) for the certification of organic produce. The study uses representative farm-level data on local vegetable value chains in northern Vietnam and uses a broad set of sustainability outcomes as well as counterfactual analysis, including systematic robustness checks. The results show that PGS significantly improves farm profitability (+117%), agroecology performance (+40%), and gives farmers more choice of sales channels (+23%). However, PGS had no significant effect on returns to labour and reduced the average crop yield. Capacity development on nursery practices, transplanting of healthy seedlings rather than direct seeding, reduced tillage, and collective crop planning and management are some of the innovations that can counter adverse effects on crop yields, increase soil health as well as improve returns to labour, and thus attract more youth to farming. Overall, the study shows that organic PGS can make vegetable production more economically viable and more agroecologically sustainable.
1. Introduction
It has been estimated that the external health and environmental costs of global food systems are double the value of all food sold (von Braun & Hendriks, Citation2023). At the same time, environmental degradation increases the risk of food shortages, especially in low- and middle-income countries. There is a widespread consensus among researchers and policymakers that healthier, more sustainable and more inclusive food systems are needed to tackle critical problems, such as soil degradation, water scarcity, reduced ecosystem services, climate change, economic inequality, food insecurity and unhealthy diets UNSG, Citation2020). Agroecology has evolved from a science of sustainable agriculture to a holistic approach to the redesign of agricultural and food systems based on community empowerment, diversity and systems thinking (Altieri, Citation1995; IPES-Food, Citation2016; Gliessman, Citation2018). It is now increasingly recognized by experts, policymakers and the public as a promising strategy to profoundly change the way food is produced (CFS, Citation2021; FAO, Citation2018a; HLPE, Citation2019). The concept of agroecology goes beyond a set of sustainable production practices by including aspects of knowledge sharing, social equity, and circularity, while addressing issues of environmental integrity, food and nutrition security, and livelihoods (FAO, Citation2019; Kerr et al., Citation2021; van der Ploeg et al., Citation2019; Wezel et al., Citation2020). Agroecology therefore requires embedded solutions that systematically combine technical, social and institutional innovations addressing key bottlenecks in the food system.
Participatory guarantee systems (PGS) are one such approach, which supports the diffusion of agroecology, especially in low- and middle-income countries where third-party certification systems are less developed and expensive. PGS promotes fair prices, reduces dependence on external inputs, and strengthens interactions between buyers, growers, and other stakeholders (Home et al., Citation2017; Loconto and Hakanata, Citation2018). Most PGS schemes are certified based on the principles of organic agriculture (IFOAM, Citation2019), but the approach can also be used for other types of farming. Based on trust and monitoring through social and business networks, PGS offers a locally focused lower-cost alternative to third-party organic certification, while providing quality assurance in local value chains (Home et al., Citation2017; Loconto and Hakanata, Citation2018). In 2022, 323 PGS initiatives were recorded in 76 countries globally, with around 1.3 million producers and 1 million hectares of land certified (Willer et al., Citation2023).
Southeast Asia, just like many other regions, has undergone important changes in its food systems in recent decades, mostly due to rapid economic growth, increasing population and demographic shifts, rapid urbanization, changing food consumption behaviour and climate change (FAO, Citation2018b). The region is not only facing biodiversity loss and climate change impacts, but also the burden of unsafe food and malnutrition. There is a growing distrust of consumers towards food quality and food safety and increasing threats to the health of farm families, both caused by high and rising levels of agricultural pesticide use (Ha et al., Citation2019; Schreinemachers et al., Citation2015). Problems are particularly acute in high-value and pest-vulnerable crops such as fruit and vegetables (Praneetvatakul et al., Citation2013; Wanwimolruk et al., Citation2016). It has been estimated that about 75% of pesticides used in vegetable production in Southeast Asia are in excess of the economic optimum (Grovermann et al., Citation2013; Schreinemachers et al., Citation2020). In response to the chronic overuse and misuse of pesticides in agriculture, governments in Southeast Asia have sought to improve food safety by promoting integrated pest management (IPM) alongside standards of good agricultural practices (GAP). These standards help to assure the quality of farm produce by defining how food should be produced and handled. However, GAP standards may not give farmers a strong enough incentive to adopt more sustainable crop management methods (Amekawa, Citation2013; Schreinemachers et al., Citation2012). Organic certification aims to eliminate the use of agrochemicals in agricultural production altogether and organic labels for domestic markets are now common across Southeast Asia.
The impacts of sustainability standards, including organic vegetable certification, have been widely analysed. Economic effects have generally received the most attention in the literature (Bolwig et al., Citation2009; Crowder & Reganold, Citation2015; Grovermann et al., Citation2021; Mendoza, Citation2004; Schleifer & Sun, Citation2020: Ssebunya et al., Citation2019; Tran & Goto, Citation2019). Others have studied the effects on the adoption of good agricultural practices, gender equality, or pesticide use (Ibanez & Blackman, Citation2016; Meemken & Qaim, Citation2018a; Schreinemachers et al., Citation2012). Very few studies have quantified the effect on agroecological outcomes, one notable exception being Blockeel et al. (Citation2023). We address this gap by combining various economic and agroecology performance indicators in a holistic evaluation of the impact of organic PGS adoption among vegetable farmers in Vietnam.
In the context of low- and middle-income countries, most previous studies have looked into the impact of labels for export crops such as coffee and cocoa, while there is little evidence for the impact of labels for food sold in local markets. This study therefore focuses on organic PGS certification of local vegetable value chains. The Vietnamese public third-party organic standard (TCVN 11041-1 issued in 2017) exists alongside various international third-party standards (e.g. ‘USDA Organic’ and ‘Organic JAS’) as well as numerous private organic PGS standards. Established in 2008, PGS-Vietnam is the first and most notable PGS standard and documenting its impact has clear policy relevance. The national food system action plan of Vietnam specifies a minimum target of 2.5% of the agricultural area for organic production by 2030 as well as a doubling of organic fertilizer from 2020 levels (USDA, Citation2023).
Value-chain actors and policymakers need a better understanding of the socioeconomic and ecological impact of adopting organic PGS by vegetable farmers. We aim to determine the causal effect of farmers’ participation in PGS-Vietnam on a broad set of sustainability outcomes. We used a representative sample of vegetable farms and employed entropy balancing combined with regression adjustment for counterfactual analysis. We postulate that PGS certification delivers economic, environmental, and social benefits, inducing a shift towards more viable and agroecological vegetable production. To our knowledge, this is the first impact study on organic PGS in Vietnam and elsewhere. We investigate the sensitivity of our findings to model assumptions and use alternative model specifications to identify the underlying mechanisms and to improve the accuracy of our results. The analysis finds that PGS certification can be instrumental in achieving sustainability goals, but adoption is constrained by lower yields and high labour requirements. The next section explains the data sources and econometric methods used to assess impact. Impact estimates are then presented in Section 3 and discussed in Section 4. The final section derives policy implications from the results.
2. Material and methods
2.1. Analytical framework
Causal attribution in impact evaluation studies involves determining the difference between two potential outcomes for the same unit, one is observed, and the other is unobserved (Imbens & Rubin, Citation2015; Imbens & Wooldridge, Citation2009). The observed outcome is what actually occurred, while the unobserved outcome is what would have occurred had no or a different intervention been assigned, i.e. the counterfactual. In the case of a binary treatment, the two potential outcomes for each individual i are denoted as for state 1 (intervention) and
for state 0 (counterfactual). In reality, data for each individual is only available for one state, while it is missing for the other state. This requires the careful construction of the counterfactual using a control group. Consequently, mean outcome differences between the intervention group and the control group are used to estimate average treatment effects rather than calculating individual treatment effects (Schafer & Kang, Citation2008). Other than in randomized experiments self-selection bias is a key challenge for causal inference in many ex-post impact evaluations, due to non-random assignment of the intervention (Imbens & Wooldridge, Citation2009). In our case, self-selection bias stems from the fact that producers who choose to participate in the standard may be different from producers who do not participate even before they adopted it (Meemken & Qaim, Citation2018a; Ssebunya et al., Citation2019). It was therefore necessary to process the data so that producers in the intervention and comparison groups no longer significantly differed in observable characteristics that are unrelated to certification, but that may affect the outcomes. Propensity score matching and reweighting are widely used techniques in ex-post impact evaluation to adjust for differences in known confounders and mitigate self-selection bias (Austin, Citation2011; Hirano et al., Citation2003; Knook et al., Citation2018; Lopez-Avila et al., Citation2017). Unlike instrumental variable (IV) regression and other regression-based approaches, matching and reweighting do not assume a normal distribution of the residuals. However, these methods only correct selection bias for the observed confounding variables (Hirano et al., Citation2003; White & Raitzer, Citation2017), not the unobserved covariates, such as farmer self-motivation for instance. It is however difficult to find one or more appropriate instrumentsFootnote1 that work for multiple separate IV estimations as there are nine outcome variables in this study. Therefore, this study relies on inverse probability and entropy weights (Austin, Citation2011; Hainmueller, Citation2012). We used these two alternative model specifications to verify the robustness of results. We also tested the sensitivity of our estimates to omitted variable bias.
2.2. Identification of counterfactual and data collection
Data for this study come from a survey of peri-urban vegetable farmers who produced white cabbage from August 2022 to March 2023 with data collected from March to April 2023. White cabbage was chosen as a focus crop as it is widely grown, commercially important and representative of the local vegetable cropping system. The survey covered 9 districts of three Vietnamese provinces (Hoabinh, Hanam, Hanoi). The organic PGS-Vietnam scheme is active in this peri-urban area near the capital Hanoi and farmers supply vegetables to markets and supermarkets in the city ().
The identification of a counterfactual, representing the situation without PGS certification, is important for attributing outcomes to standard adoption. We thus rely on a multistage sampling strategy: First, all 119 farmers from the PGS-Vietnam scheme growing white cabbage were included in the sample for the intervention group. This represents 80% of the 149 PGS-certified farms around Hanoi. For the comparison group, power calculations on profitability, a key outcome variable, suggested a minimum group size of 279 farms (alpha = 0.05, power = 0.8, intervention group = 120 farms). We added 5% in case that some observations had to be discarded in the analysis. The power calculations were based on a one-sided two-means comparison and a fixed intervention group size of 120. Without similar studies in Vietnam or neighbouring countries, averages and standard deviations for organic and conventional cabbage profitability were based on expert opinion and compared to the global literature. The following approach was subsequently used to select farmers into the comparison group: for each of the 9 vegetable-producing districts, major white cabbage production villages (defined as villages with at least 50 farmers growing cabbage in a conventional way) were identified with the help of local extension officers. Next, twenty farmers were randomly selected from a list of all cabbage farmers per village; and 10–14 of these were selected by village head for interviews based on their availability. We continued to add selected villages until we had completed 301 interviews. The total sample included 420 cabbage farmers spread over 26 villages in 9 districts, of which 119 farmers were PGS-certified.
The structured questionnaire included approximately 200 questions and was tested with farmers in a conventional and a PGS location before the start of the data collection. It was approved by the ethics committee of the Research Institute of Organic Agriculture (FiBL; Approval Nr. FSS-2023-07). The survey was implemented in the Kobo Toolbox, with answers recorded on tablets and exported into a customized MS Excel database. The respondent was identified as the farm manager responsible for vegetable production. Interviews took about 90 min on average. At the start of the interview, enumerators explained the purpose and content of the survey and ensured that data would be kept confidential. All respondents were asked for their verbal consent to participate. Data quality was checked daily by a designated data quality control officer. The anonymized data are available from the authors upon request.
The data included information about farm and farmer characteristics, certification, and production, including output quantities and values as well as and input use for white cabbage. Agroecological outcomes were measured using part of the Tool for Agroecology Performance Evaluation (TAPE; FAO, Citation2019), which was deemed relevant to Vietnamese farming context. Step 1 of TAPE operationalizes the ten elements of agroecology through a multi-criteria scoring approach. The ten elements of agroecology characterize the sustainability of agricultural production systems, highlighting their level of transition to agroecology. The TAPE diagnostic can be used to identify the strengths and weaknesses of these systems. For this purpose, each element is divided into multiple sub-elements, which are measured on a scale from zero to four.Footnote2 The diversity score for instance contains ratings for the diversity of trees, livestock, trees, and other economic activities (FAO, Citation2019). All data were analysed using the statistical software Stata17. Monetary values were converted from Vietnamese Dong (VND) to US dollars (USD), while local area units were converted from sao (360 m2) to hectares.Footnote3 Extreme outliers in the cost, yield and price variables were winsorized at the 1st and 99th percentiles, with replacement.
2.3. Outcome and control variables
We consulted with experts from Vietnam, Switzerland, and FAO to define a broad set of sustainability outcomes for this study, as shown in . Variable choice is informed by the 13 principles of agroecology (Wezel et al., Citation2020) as well as FAO’s ten elements of agroecology (FAO, Citation2018a). It follows an impact pathway logic leading from awareness to adoption, and further to improved farm performance.
Table 1. Summary statistics of the outcome variables used in the analysis.
2.3.1. Economic outcomes
White cabbage productivity and profitability were used as economic performance measures. Data were collected on farmers’ expenditures on all inputs (organic and mineral fertilisers, synthetic and bio-based pesticides, growth regulators, hired labour, planting materials, etc.). The main variables of interest are white cabbage yield (in t/ha), gross margin (including hired labour cost) and return to labour, as the ratio between gross margin and all labour input. This is important to capture also differences in own labour, which, other than hired labour, is not valued at market prices.
2.3.2. Environmental outcomes
Eleven agroecological practices relevant to vegetable production in northern Vietnam were selected by the project team with questions about awareness, uptake and confidence (A). As shown in , outcomes include the number of agroecological practices the respondent is aware of, the number of these practices used, and the respondent’s confidence in these practices (0–1 score). While aware and use are count variables, conf is measured as a mean score on a 3-point scale, from no confidence (0) to high confidence (2) for each practice, with individual ratings averaged over all eleven practices and then standardized from zero to unity (0-1).
Figure 2. Descriptive group comparison for the individual components of aggregate environmental outcome variables ‘use’ (graph A) and ‘tape5’ (graph B).

Table 2. Description of agroecological practices used as outcomes.
FAO’s TAPE indicator set was used to quantify the ecological performance of farms (FAO, Citation2019). We selected five out of ten metrics from TAPE Step 1: diversity, synergy, efficiency, recycling, and resilience (B). The other five metrics (culture and food traditions, knowledge co-creation, human and social values, circular and solidarity economy, and responsible governance) are also important, but less relevant to measure environmental sustainability. We then created the aggregate outcome variable tape5 and transformed it into a score ranging from zero to unity. This represents the average of five sustainability metrics.
2.3.3. Social outcomes
The two variables used in this category are both considered proxies for social empowerment. The first variable captures farmers’ holistic understanding of organic farming. It is measured as the number of keywords mentioned when asking respondents to explain in their own words the meaning of organic farming. Eleven keywords were defined and could be marked.Footnote4 The second variable captures choice vs. no choice of sales channel, asking farmers if they can only sell to one outlet or if they have a choice of multiple outlets. These two social outcomes indicate the strength of rural communities and the local economy.
2.3.4. Controls
Fourteen covariates conceptually related to PGS participation were selected based on previous studies (e.g. Gautam et al., Citation2017; Meemken & Qaim, Citation2018a) and the expertise of the researchers: sex, age, education and vegetable growing experience of the farm manager, household size (in terms of persons sharing a meal), non-farm income, loan from bank, farm size, the proportion of rented farmland, size of vegetable area, proportion of vegetable area with title, training attended in the last five years, extension visit in the last five years (1 = yes), frequency of contact with neighbours, membership in the national farmer organization ().
Table 3. Summary statistics of control variables in the analysis.
2.4. Econometric estimation
Rather than determining the average effect at the level of the entire population, our interest is to understand the effectiveness of certification for the farmers who took part in and complied with the PGS standard. Therefore, we estimate the average treatment effect on the treated (ATT), which is the average impact on those subjects who ultimately participate in the intervention (Austin, Citation2011; Imbens & Wooldridge, Citation2009). Contrary to a weighted mean comparison for ATT estimation, the advantage of a weighted regression adjustment estimator is that it provides correct standard errors for the ATT (Jann, Citation2021). This is a function of the doubly robust properties of this estimator (Kurz, Citation2022; Zhao & Percival, Citation2016). The weighted regression adjustment implies that weights are determined in the first step for subsequent inclusion in an outcome model in the second step. Both steps include the use of the same covariates (Jann, Citation2021).
Weighting has advantages over the more widely used propensity score matching since observations without an exact or approximate match are not dropped from the analysis (Hirano et al., Citation2003). This allows for maintaining the full comparison group (Meemken & Qaim, Citation2018a). We used entropy weights and inverse probability weights as alternative approaches. Both rely on the same set of covariates, as shown in , and address systematic differences in the distribution of these observed covariates between the intervention group of certified farms and the comparison group of non-certified farms. The covariates in are assumed to simultaneously affect the certification decision and the outcome variables and are used to compute the weights. When systematic differences are controlled for through weighting, the two groups become similar to the extent that remaining differences in the outcome variables can be interpreted as ‘treatment effects’ (Meemken & Qaim, Citation2018a).
Entropy balancing, as proposed by Hainmueller (Citation2012), generates weights that exactly balance all covariates according to their mean and higher moments if convergence is achieved. The method is implemented in Stata through the ebalance routine (Hainmueller & Xu, Citation2013). Weights are assigned to each farm in the comparison group, but not to the farms in the intervention group, resulting in the estimation of the ATT as E(Y1 − Y0|Ui = 1) = E(Y1|Ti = 1) − E(Y0|Ui = 1), where Y1 is the outcome with certification, Y0 is the outcome without certification and Ti refers to farmers’ participation in the PGS scheme. Entropy balancing generally achieves higher estimation accuracy than inverse probability weighting (Harvey et al. Citation2017). When computing the entropy weights in our analysis, convergence was achieved by balancing all covariates for both mean and variance. This produced almost identical statistics for the intervention and comparison groups, while inverse probability weights provided only approximate balance between groups (appendix, Table A). As inverse probability weights are commonly used in ex-post impact evaluation and are slightly less prone (as compared to entropy weights) to contain extreme weighting values for individual observations, they were used to verify the results. Inverse probability weights are generated by predicting the propensity score (probability of treatment) from a logit regression (Austin, Citation2011), including the covariates from as predictors. Weights were calculated as 1/(1 − propensity score) for the untreated group, while the treated group was not weighted when estimating the ATT. To obtain correct standard errors, the computed weights were entered into the regression adjustment model, which is run for each set of weights, using the same covariates as used in the first step of the data processing (appendix, Table B). Regression adjustment was performed using the teffects ra command in Stata according to the distribution of the outcome variables. Linear outcome models were specified for the variables gm, labreturn and yield. For the count variables aware, use and orgknow, Poisson models were applied. Fractional outcome models were used for the variables tape5 and conf, which are bounded between zero and unity, while a binary outcome model was used for the outcome variable choice. We assumed that observations within a district are not completely independent from one another and therefore clustered the standard errors by district.
2.5. Robustness checks
As a robustness check, we assessed the extent to which our results are sensitive to omitted variable bias as weighted regression adjustment does not control for the risk of this bias. This may be an issue in our study because it is not possible to be aware of and collect data on all possible confounders. For instance, information on certain personal characteristics of farmers (e.g. attitudes and beliefs), that might influence the outcomes of interest, is not available and might influence the results. To understand the potential effect of omitted variables on our results, we applied the regression sensitivity approach proposed by Diegert et al. (Citation2022), implemented in Stata as regsensitivity. Contrary to Oster bounds for selection on unobservables (Oster, Citation2019), it allows for the included control variables to be correlated with unobserved control variables, comparing the magnitude of selection on observables with the magnitude of selection on unobservables. A so-called breakdown point (rxbar) defines the threshold for the results to be overturned by omitted variable bias (Diegert et al., Citation2022). The higher the breakdown point, the less likely it is for the results to be heavily influenced by unobserved factors. Using the same covariates as in the main treatment effect estimations, we applied this robustness check to all outcome variables. Breakdown points are calculated for five levels of correlation (cbar) between observed and unobserved variables (0.00, 0.25, 0.50, 0.75, 1.00).
We also verified the results to understand if there are underlying structural biases. To reflect the importance of different income sources, we used subsamples of the variable measuring on-farm income. The robustness of the results was checked when excluding farms with low on-farm income (1) as well as those with high on-farm income (4).
3. Results
Significant positive results were obtained for most outcome variables, with effect sizes ranging from 23% to over 100% (). This demonstrates that organic PGS has a relevant role in making vegetable production more viable and more agroecological. However, some key challenges also became evident as there are large and negative effect sizes for crop yield and return to labour, albeit only significant for yield. The estimated treatment effects are very similar in terms of direction and significance for both approaches, which gives confidence to the results. Nevertheless, some of the effect sizes differ, e.g. for crop yield or knowledge of organic farming. This can be explained by the fact that entropy weights can be more extreme and provide exact balance among groups, while the balance is more approximate in the case of inverse probability weights, where observations are less likely to receive extremely small weights ().
Table 4. Estimated treatment effects.
PGS has a large and positive impact on the profitability of white cabbage production, as shown by the results for gm. However, the return to labour is not significant for both estimations as PGS uses substantially more labour. Crop yield is negatively affected by PGS participation with the effect size ranging from −29% to −38%.
Environmental performance of PGS participation is measured through five elements from FAO’s TAPE score as well as through awareness and adoption of agroecological practices. This is complemented by a confidence score for these practices. Across all outcome variables (tape5, aware, use and conf) we determined a positive and significant impact of PGS participation, ranging from 41% to 46% for the TAPE score and from 33% to 45% for the confidence score.
Knowledge of organic farming, measured in terms of mentioned keywords and choice of market channel, is used as a proxy for social empowerment of farmers. The effect sizes are positive and significant, ranging from 63% to 104% for knowledge and from 23% to 33% for choice of market channel.
As robustness checks, subsampling and omitted variable tests were carried out. Initially, we performed the econometric estimations with two subsets of the data. Farms with low non-farm income were excluded, so that the remaining sample contained 108 PGS-certified farms and 243 non-PGS farms. This resulted in very similar effect sizes and, apart from the variable choice, there was no chance in significance levels. When farms with the highest on-farm incomes were excluded, we observed the same results, having 101 PGS-certified farms and 248 farms in the comparison group. In addition to subsets of the data, sensitivity tests were carried out to assess the risk of omitted variable bias for all outcome variables in the analysis. The breakdown points (rxbar) shown in indicate at what point the direction of our estimated treatment effects will be overturned (also see appendix Table A). The percentages for each outcome variable give us an indication of the extent of selection on unobservables, as compared to selection on observables, which would result in this reversal. A range of breakdown points were computed for different levels of correlation (cbar) between unobserved and observed variables, with 1 indicating full correlation and 0 indicating no correlation. Likely some slight to moderate correlation can be expected. No clear cut-off exists for ruling out the risk of omitted variable bias through the regression sensitivity approach. The method rather helps to quantify the risk, with low breakdown points indicating a high likelihood of selection on unobservables occurring alongside selection on observables. As we have access to a comprehensive set of co-variates, we do not expect that more than 50% of the selected bias corrected by observables would be caused by unobserved factors. We consider this a reasonable assumption and conclude that estimations for two variables (returnlab and choice) might be prone to bias from omitted variables, with the return to labour analysis more seriously affected than the analysis of the sales channel choice. It appears that our impact model for return to labour might be missing some variables that were not captured in the survey. Yet, judging from the descriptive data for labour and gross margin, the general trend of the result for return to labour seems plausible (appendix, Table B).
4. Discussion
As the first impact evaluation of organic PGS certification, our analysis confirms the original premise that organic PGS certification delivers economic, environmental, and social benefits. The impact of PGS is positive across all indicators of agroecology uptake and agroecological performance but mixed for the economic indicators. Gross margins were positively affected by PGS participation, but high labour requirements and a substantial yield penalty reduced the economic viability and scalability of current PGS-certified vegetable production in northern Vietnam. Robustness of the findings is confirmed by using two weighting approaches, checking sensitivity to omitted variables, and examining the underlying structural biases. However, the focus on one season and one crop for the quantification of economic outcomes may somewhat affect the validity of the results. While white cabbage is the most important crop for vegetable farmers in the study area, the overall economic performance of farms depends on a larger range of crops.
For profitability, our results are in line with evidence from meta-analyses (Crowder & Reganold, Citation2015; Seufert & Ramankutty, Citation2017), but do not confirm the findings by Pham and Shively (Citation2019). The latter study, examining upland vegetable production in northern Vietnam, showed that organic certification did not increase farm profitability. Their analysis focused on the third-party TCVN standard and used OLS regression or a relatively small sample covering multiple crops. Our evaluation is focused on the main commercial crop in the study area for the winter cropping season and relies on a more robust counterfactual analysis. The highly significant positive effect on white cabbage profits, which the analysis revealed, is driven by considerable price premiums for organic vegetables and by lower variable input costs as farmers use fewer chemical inputs.
Meemken and Qaim (Citation2018b) pointed out that many studies on the profitability of organic agriculture in low- and middle-income countries do not account for the opportunity cost of family labour. Our study addresses this issue by including returns to labour as an outcome variable. Once own labour is accounted for, the positive effect on farm profitability disappears. The review by Crowder and Reganold (Citation2015) found that organic agriculture uses 13% more labour on average. According to their analysis, when factoring in labour costs then the benefit–cost ratios of organic vegetables are lower than for all other crops but on par with those of conventionally produced vegetables. For vegetable production in Europe, labour requirements have been reported to be twice as high on organic vegetable farms as on conventional farms (Jansen, Citation2000). We could not find comparative studies on labour use on vegetable farms in Asia, but our descriptive data suggest that organic PGS farms use 2.5 times more labour than conventional farms. When considering labour tasks, we find that the labour input on PGS-certified farms is higher for land preparation, harvesting and sorting, and especially for compost application and weeding. Labour shortages will challenge the economic viability of organic PGS vegetable production, but organic PGS also creates jobs in areas with fewer employment opportunities. As many farmers are close to retirement age and labour markets are highly competitive in peri-urban contexts such as Hanoi where youth have opportunities outside agriculture, labour-saving technologies are needed for PGS organic development. Expert opinions suggest that improved seed for organic farming conditions, exact transplanting of seedlings and less soil disturbance through reduced tillage can be promising strategies for reducing the number of person-days required in land preparation and weeding. Furthermore, collective crop planning and growing schemes for PGS vegetable cultivation among farmer group members have the potential to facilitate shared input use and plot size optimization, thus reducing labour requirements.
For vegetable yields, reviews suggest a gap ranging between 13% and 33% (see in Meemken & Qaim, Citation2018b). Based on farm data rather than experimental data analysis, Kniss et al. (Citation2016) reported yield gaps of 50% and more for vegetables in the US. For Asia, we found no research on vegetable yield gaps, but the meta-analysis of De Ponti et al. (Citation2012) suggests 11% across major crops. For our case, yield reduction due to participation in organic PGS is at the upper end of the range that meta-analyses suggest. To the knowledge of the authors, this can be mainly explained by the absence of synthetic inputs in organic PGS-certified production systems, a lack of availability of organic vegetable seed and suboptimal soil management. Reduced tillage and better organic amendments can help improve soil structure, organic input effectiveness, and yields in the PGS vegetable systems. From an economic perspective, high price premiums compensate for lower yields. However, not many consumers can, on a regular basis, afford organic produce that is twice or even three times as expensive as conventionally produced vegetables. Therefore, a reduced yield gap and lower labour requirements in PGS-certified production systems may also translate into more affordable organic vegetables, with price premiums below a 100% markup. The study by Hai et al. (Citation2013) suggested that consumers in Hanoi are willing to pay 70% more for organic vegetables. Besides productivity increases, direct marketing can also help in lowering the cost of organic vegetables for consumers and improve farmers’ income (Wang et al., Citation2014). Our results show that PGS participation leads to more choice in terms of marketing channels, however, direct marketing to consumers is done by very few PGS farmers. Digital tools and collective arrangements can help to develop this option into a more widely used sales outlet. Navarette et al. (Citation2015) point out that crop management, labour organization, and marketing are crucial issues for the sustainability of organic vegetable production. While the findings are based on data from France, the same challenges seem to also apply to our case study in Vietnam.
Despite lower productivity, organic farming has been shown to provide a range of environmental benefits, such as increased biodiversity (Gong et al., Citation2022) and resilience. To determine impacts on environmental sustainability, a range of outcomes related to agroecological growing practices were evaluated. In addition, five elements of the TAPE tool were used in the analysis to assess the effects of organic PGS certification on agroecology performance. Substantial positive changes in awareness, uptake and confidence regarding practices, as well as an increase in the TAPE score, can be attributed to PGS participation. This demonstrates that organic vegetable production based on credible participatory certification can generate clear benefits in terms of environmental sustainability. The scoring results from the TAPE assessment are confirmed by the data on the uptake of agroecological practices. While the application of TAPE is generally straightforward, the wording for scores needed to be contextualized and not all scoring categories are always applicable to a specific case. In addition, compound statements can complicate the exact measurement of concepts.Footnote5 While in some instances clarity of scores can be improved, the overall application of a selection of TAPE elements has proved to be a valuable addition to the set of outcome indicators, allowing for a better understanding of the impacts of PGS certification on the sustainability of vegetable production in our study area. A more complete application of TAPE, including metrics, such as knowledge co-creation, human and social values or circular and solidarity economy, would allow researchers to better capture social outcomes of certification. Empowerment and revitalization of rural communities may thus be considered in future impact studies on certification standards.
5. Conclusion
Organic PGS standards have been around for more than two decades and are increasingly used as a certification tool in middle-income countries, such as Vietnam, where there is a rising consumer demand for safe food. This is the first quantitative evaluation of the impact of organic PGS certification in Vietnam. The article is based on a counterfactual analysis, state-of-the-art robustness checks and a broad set of sustainability outcomes. The study shows that the production of PGS-certified organic vegetables in Vietnam leads to higher profits and better agroecological performance, but also faces challenges, such as lower crop yield and higher labour requirements, which are effects consistent with studies for other countries. While these trade-offs are well established, they are not a given and it remains important to quantify the extent of positive and negative effects of PGS certification.
To attract more farmers, especially youth, and realize the agroecological potential of organic PGS production systems, the challenges can be addressed through improved advisory services for PGS farmers. Our study identifies several areas for improvements in organic PGS vegetable production, which can be integrated into capacity development interventions. Suggested improvements involve transplanting of healthy seedlings rather than direct seeding as well as applying reduced tillage for enhanced soil structure, nutrient holding capacity and weed suppression. Collective crop planning and management are also important to enhance labour efficiency, farming structure and overall farming landscape ecosystem services. Government and other stakeholders’ investments and efforts to promote these practices appear well justified, considering the positive environmental externalities and food safety advantages obtained in PGS-certified vegetable production. To reduce uncertainty, labour-saving techniques and improved soil fertility management need to first be piloted among selected organic vegetable farmers. Future research should then systematically evaluate benefits and risks before more widely scaling recommended practices.
Additional information
Funding
Notes
1 Valid instruments need to be correlated with the intervention (such as standard participation), but must not be correlated with the outcomes, except through their indirect effect on the intervention (White & Raitzer, Citation2017).
2 The TAPE methodology and examples of the tool’s application are available on the TAPE website of FAO: https://www.fao.org/agroecology/tools-tape/en/
3 At the time of the survey, the prevailing exchange rate was 23,500 VND = 1 USD.
4 Such as: no synthetic pesticides, no chemical fertilisers, natural nutrient cycles, natural inputs, beneficial organisms, local seeds, crop diversity, balanced agroecosystems, premium prices, specialty markets, other.
5 E.g. the description for the highest score of ‘Crop diversity’ under the ‘Diversity’ element: ‘more than 3 crops and varieties adapted to local conditions. Spatially diversified farm by multi-, poly- or intercropping’.
References
- Altieri, M. A. (1995). Agroecology: The science of sustainable agriculture (2nd ed.). Boulder, CO: Westview Press.
- Amekawa, Y. (2013). Can a public GAP approach ensure safety and fairness? A comparative study of Q-GAP in Thailand. The Journal of Peasant Studies, 40, 189–217.
- Austin, P.C. (2011). An introduction to propensity score methods for reducing the effects of confounding in observational studies. Multivariate Behavioral Research, 46(3), pp. 399–424.
- Blockeel, J., Schader, C., Heidenreich, A., Grovermann, C., Kadzere, I., Egyir, I.S., Muriuki, A., Bandanaa, J., Tanga, C.M., Clottey, J., & Ndungu, J. (2023). Do organic farming initiatives in Sub-saharan Africa improve the sustainability of smallholder farmers? Evidence from five case studies in Ghana and Kenya. Journal of Rural Studies, 98, 34–58.
- Bolwig, S., Gibbon, P., & Jones, S. (2009). The economics of smallholder organic contract farming in tropical Africa. World Development, 37, 1094–1104.
- CFS. (2021). Report of the 49th session: Making a difference in food security and nutrition. Committee on World Food Security.
- Crowder, D.W., & Reganold, J.P. (2015). Financial competitiveness of organic agriculture on a global scale. Proceedings of the National Academy of Sciences, 112, 7611–7616.
- De Ponti, T., Rijk, B., van Ittersum, M. K. (2012). The crop yield gap between organic and conventional agriculture. Agricultural Systems, 108, 1–9.
- Diegert, P., Masten, M. A., & Poirier, A. (2022). Assessing omitted variable bias when the controls are endogenous. arXiv: 2206.02303.
- FAO. (2018a). 10 elements of agroecology. UN Food and Agriculture Organization.
- FAO. (2018b). Asia and the Pacific regional overview of food security and nutrition 2018 – accelerating progress towards the SDGs. UN Food and Agriculture Organization.
- FAO. (2019). TAPE tool for agroecology performance evaluation – process of development and guidelines for application. Test version. UN Food and Agriculture Organization.
- Gautam, S., Schreinemachers, P., Uddin, M. N., & Srinivasan, R. (2017). Impact of training vegetable farmers in Bangladesh in integrated pest management (IPM). Crop Protection, 102, 161–169.
- Gliessman, S. (2018). Defining agroecology. Agroecology and Sustainable Food Systems, 42, 599–600.
- Gong, S., Hodgson, J.A., Tscharntke, T., Liu, Y., van der Werf, W., Batáry, P., Knops, J.M., & Zou, Y. (2022). Biodiversity and yield trade-offs for organic farming. Ecology Letters, 25, 1699–1710.
- Grovermann, C., Quiédeville, S., Muller, A., Leiber, F., Stolze, M., & Moakes, S. (2021). Does organic certification make economic sense for dairy farmers in Europe?–a latent class counterfactual analysis. Agricultural Economics, 52, 1001–1012.
- Grovermann, C., Schreinemachers, P., & Berger, T. (2013). Quantifying pesticide overuse from farmer and societal points of view: An application to Thailand. Crop Protection, 53, 161–168.
- Ha, T.M., Shakur, S., & Do, K.H. (2019). Consumer concern about food safety in Hanoi, Vietnam. Food Control, 98, 238–244.
- Hai, N.M., Moritaka, M., & Fukuda, S. (2013). Willingness to Pay for organic vegetables in Vietnam: An empirical analysis in Hanoi capital, journal of faculty of agriculture. Kyushu University, 58, 449–458.
- Hainmueller, J.(2012). Entropy balancing for causal effects: A multivariate reweighting method to produce balanced samples in observational studies. Political Analysis 20, 25–46.
- Hainmueller, J., & Xu, Y. (2013). Ebalance: A Stata package for entropy balancing. Journal of Statistical Software, 54, 1–18.
- Harvey, R.A., Hayden, J.D., Kamble, P.S., Bouchard, J.R., & Huang, J.C. (2017). A comparison of entropy balance and probability weighting methods to generalize observational cohorts to a population: A simulation and empirical example. Pharmacoepidemiology and Drug Safety, 26, 368–377.
- Hirano, K., Imbens, G.W., & Ridder, G. (2003). Efficient estimation of average treatment effects using the estimated propensity score. Econometrica, 71, 1161–1189.
- HLPE. (2019). Agroecological and other innovative approaches for sustainable agriculture and food systems that enhance food security and nutrition. High Level Panel of Experts on Food Security and Nutrition of the Committee on World Food Security.
- Home, R., Bouagnimbeck, H., Ugas, R., Arbenz, M., & Stolze, M. (2017). Participatory guarantee systems: Organic certification to empower farmers and strengthen communities. Agroecology and Sustainable Food Systems, 41, 526–545.
- Ibanez, M., & Blackman, A., 2016. Is eco-certification a win–win for developing country agriculture? Organic coffee certification in Colombia. World Development, 82, 14–27.
- IFOAM. (2019). PGS guidelines. How to develop and manage participatory guarantee systems for organic agriculture. IFOAM Organics International. Bonn.
- Imbens, G.W., & Rubin, D.B., 2015. Causal inference in statistics, social, and biomedical sciences. Cambridge University Press.
- Imbens, G.W., & Wooldridge, J.M. (2009). Recent developments in the econometrics of program evaluation. Journal of Economic Literature, 47, 5–86.
- IPES-Food. 2016. From uniformity to diversity: A paradigm shift from industrial agriculture to diversified agroecological systems. International panel of experts on sustainable food systems. Louvain-la-Neuve.
- Jann, B. (2021). Entropy balancing as an estimation command. Working Paper No. 39. University of Bern Social Sciences.
- Jansen, K. (2000). Labour, livelihoods and the quality of life in organic agriculture in Europe. Biological Agriculture & Horticulture, 17, 247–278.
- Kerr, R.B., Madsen, S., Stüber, M., Liebert, J., Enloe, S., Borghino, N., Parros, P., Mutyambai, D.M., Prudhon, M., & Wezel, A. (2021). Can agroecology improve food security and nutrition? A review. Global Food Security, 29, 100540.
- Kniss, A. R., Savage, S. D., & Jabbour, R. (2016). Commercial crop yields reveal strengths and weaknesses for organic agriculture in the United States. PLoS One, 11, e0161673.
- Knook, J., Eory, V., Brander, M., & Moran, D., 2018. Evaluation of farmer participatory extension programmes. The Journal of Agricultural Education and Extension 24, 309–100325.
- Kurz, C. F. (2022). Augmented inverse probability weighting and the double robustness property. Medical Decision Making, 42, 156–167.
- Loconto, A., & Hatanaka, M. (2018). Participatory guarantee systems: Alternative ways of defining, measuring, and assessing ‘sustainability’. Sociologia Ruralis, 58, 412–432.
- Lopez-Avila, D., Husain, S., Bhatia, R., Nath, M., & Vinaygyam, R. (2017). Agricultural innovation: An evidence gap map. 3ie Evidence Gap Map Report 12. New Delhi, International Initiative for Impact Evaluation (3ie).
- Meemken, E.M., & Qaim, M. (2018a). Can private food standards promote gender equality in the small farm sector? Journal of Rural Studies, 58, 39–51.
- Meemken, E. M., & Qaim, M. (2018b). Organic agriculture, food security, and the environment. Annual Review of Resource Economics, 10, 39–63.
- Mendoza, T.C. (2004). Evaluating the benefits of organic farming in rice agroecosystems in the Philippines, Journal of Sustainable Agriculture 24, 93–115.
- Navarrete, M., Dupré, L., & Lamine, C., 2015. Crop management, labour organization, and marketing: Three key issues for improving sustainability in organic vegetable farming. International Journal of Agricultural Sustainability, 13, 257–274.
- Oster, E. (2019). Unobservable selection and coefficient stability: Theory and evidence. Journal of Business & Economic Statistics 37, 187–204.
- Pham, L., & Shively, G. (2019). Profitability of organic vegetable production in northwest Vietnam: Evidence from Tan Lac district, Hoa Binh Province. Organic Agriculture, 9, 211–223.
- Praneetvatakul, S., Schreinemachers, P., Pananurak, P., & Tipraqsa, P., 2013. Pesticides, external costs and policy options for Thai agriculture. Environmental Science & Policy, 27, 103–113.
- Schafer, J. L., & Kang, J. (2008). Average causal effects from nonrandomized studies: A practical guide and simulated example. Psychological Methods, 13, 279–313.
- Schleifer, P., & Sun, Y. (2020). Reviewing the impact of sustainability certification on food security in developing countries. Global Food Security, 24, 100337.
- Schreinemachers, P., Afari-Sefa, V., Heng, C.H., Dung, P.T.M., Praneetvatakul, S., & Srinivasan, R., 2015. Safe and sustainable crop protection in Southeast Asia: Status, challenges and policy options. Environmental Science & Policy, 54, 357–366.
- Schreinemachers, P., Grovermann, C., Praneetvatakul, S., Heng, P., Nguyen, T.T.L., Buntong, B., Le, N.T., & Pinn, T. (2020). How much is too much? Quantifying pesticide overuse in vegetable production in Southeast Asia. Journal of Cleaner Production, 244, 118738.
- Schreinemachers, P., Schad, I., Tipraqsa, P., Williams, P.M., Neef, A., Riwthong, S., Sangchan, W., & Grovermann, C. (2012). Can public GAP standards reduce agricultural pesticide use? The case of fruit and vegetable farming in northern Thailand. Agriculture and Human Values, 29, 519–118529.
- Seufert, V., & Ramankutty, N. (2017). Many shades of gray—the context-dependent performance of organic agriculture. Science Advances, 3(3), e1602638.
- Ssebunya, B., Morawetz, U., Schader, C., Stolze, M., Schmid, E. (2019). Group membership and certification effects on incomes of coffee farmers in Uganda. European Review of Agricultural Economics 46, 109–132.
- Tran, D., & Goto, D. (2019). Impacts of sustainability certification on farm income: Evidence from small-scale specialty green tea farmers in Vietnam. Food Policy 83, 70–82.
- UNSG. (2020). Report of the secretary-general: Progress towards the sustainable development goals. New York: United Nations Economic and Social Council.
- USDA. (2023). Vietnam issues national action plan on food systems transformation toward transparency responsibility and sustainability by 2030. United States Department of Agriculture – Foreign Agricultural Service Report VM 2023-0017.
- van der Ploeg, J.D., Barjolle, D., Bruil, J., Brunori, G., Costa Madureira, L.M., Dessein, J., … , Wezel, A. (2019). The economic potential of agroecology: Empirical evidence from Europe. Journal of Rural Studies 71, 46–61.
- von Braun, J., & Hendriks, S. (2023). Full-cost accounting and redefining the cost of food: Implications for agricultural economics research. Agricultural Economics 54, 451–454.
- Wang, H., Moustier, P., & Loc, N.T.T. (2014). Economic impact of direct marketing and contracts: The case of safe vegetable chains in northern Vietnam. Food Policy, 47, 13–23.
- Wanwimolruk, S., Phopin, K., Boonpangrak, S., & Prachayasittikul, V. (2016). Food safety in Thailand 4: Comparison of pesticide residues found in three commonly consumed vegetables purchased from local markets and supermarkets in Thailand. PeerJ, 4, e2432.
- Wezel, A., Herren, B.G., Kerr, R.B., Barrios, E., Gonçalves, A.L.R., & Sinclair, F. (2020). Agroecological principles and elements and their implications for transitioning to sustainable food systems: A review. Agronomy for Sustainable Development, 40, 1–e2413.
- White, H., & Raitzer, D.A. (2017). Impact evaluation of development interventions: A practical guide. Asian Development Bank.
- Willer, H., Schlatter, B., & Trávníček, J. (Eds.). (2023). The world of organic agriculture. Statistics and emerging trends 2023. Research Institute of Organic Agriculture FiBL and IFOAM – Organics International. Frick and Bonn.
- Zhao, Q. & Percival, D. (2016). Entropy balancing is doubly robust. Journal of Causal Inference 5(1).
Appendix
Table A. Balancing of covariates for both weighting approaches.
Table B. Exact results of sensitivity analysis with breakdown points for different correlation levels.