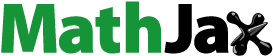
Abstract
Despite the surge in efficacy studies, the relative effectiveness of any two math interventions is largely unknown and replications are rare. To ensure that students benefit from high-quality learning experiences, the current conceptual replication of Wang and Woodworth, a randomized control trial, evaluated the relative impacts of two computer programs used in a school district as supplements to students’ regular education math instruction, DreamBox Learning and Zearn Math. For 115 students in kindergarten and first grade, data were collected before and after an 11-week implementation period. Measures of effect size, but not null hypothesis statistical tests, suggested that participants in the DreamBox condition had higher test scores on posttest numeracy and geometry (Hedges g = .32 and .14). There was also a statistically significant group by pretest interaction, evidence of “Matthew Effects” for numeracy. Although some support for adopting DreamBox as a supplemental method for improving math competencies of students in early elementary school was provided, more research is needed as the true effect may be smaller than what the present study reports. The study’s implications are discussed.
Fueled by findings that U.S. students underperform in math compared to students in other countries (e.g., Provasnik et al., Citation2016; Schleicher, Citation2018), math instruction in the U.S. has faced increased scrutiny. Research on early numeracy is also increasing (Methe et al., Citation2011), including studies evaluating the effectiveness of math interventions for children in early childhood through first grade. Even with this surge in research, the effectiveness of many math interventions remains untested, the relative effectiveness of any two math interventions is largely unknown, and most intervention studies are not replicated (Makel & Plucker, Citation2014). In fact, Nelson and McMaster’s (Citation2019) meta-analysis suggested that from among empirical studies of numeracy interventions implemented with kindergarteners and first-graders, only 4% included control conditions with an alternative math intervention. In addition, the Director of the Institute of Education Sciences has brought attention to the need to replicate studies to determine what works for whom, under what conditions (Schneider, Citation2018, Citation2021). Therefore, the present study was designed to replicate Wang and Woodworth (Citation2011), determine if their findings hold up to variations in settings and contextual factors, and in doing so, evaluate the effectiveness of two math interventions relative to one another when used with kindergarteners and first-graders.
Early Mathematics
Competencies such as understanding number concepts, number relations, and arithmetic (referred to as “numeracy” herein) are central to early math instruction. Research suggests that numeracy skills such as counting, number knowledge, quantity comparison, story problems, and number-word linkages are important to children’s growth in math achievement across elementary school (e.g., Aunola et al., Citation2004; Jordan, et al., Citation2009; Krajewski & Schneider, Citation2009; Nguyen et al., Citation2016). Numeracy skills build upon each other such that children who begin with the lowest levels of numeracy show the lowest levels of growth in math from kindergarten to fifth grade in the absence of effective intervention (Bodovski & Farkas, Citation2007; Geary et al., Citation2012; Morgan et al., Citation2009). This suggests that low numeracy performance is relatively stable and the gap between the lowest and highest performing children increases (referred to as Matthew Effects; Bodovski & Farakas, Citation2007). Indeed, Aunola et al. (Citation2004) demonstrated that children who started preschool with more knowledge of numerical skills showed faster rates of learning than children with less knowledge through second grade.
Early mathematical learning is optimized when instructional tasks are matched to a child’s skill level (Clements & Sarama, Citation2009). This allows misunderstandings in a child’s foundational numeracy concepts and skills to be remediated, leading to accelerated learning of math. Such children follow a compensatory achievement trajectory (Huang et al., Citation2014; Leppänen et al., Citation2004) where lower performing children experience an acceleration in growth relative to their higher performing peers. Early math instruction that is differentiated to each learner’s skill level is therefore especially promising. Such instruction can benefit children with the lowest levels of numeracy, allowing them to catch up with their high-performing peers. Regardless of a child’s learning trajectory, comprehending numeracy provides a stronger understanding of more complex math problems that involve measurement, data analysis, and geometry (National Research Council [NRC], Citation2009).
Early Mathematics Instruction
Building from their informal knowledge of numeracy, students develop formal knowledge of math in kindergarten and first grade. These skills include writing numerals and symbols, fluency with basic arithmetic facts, and understanding place value (National Governors Association Center for Best Practices [NGACBP], Council of Chief State School [CCSSO], Citation2010). High-quality early math instruction helps mitigate and prevent math learning difficulties (Clements & Sarama, Citation2011; Cross et al., Citation2009; Foster et al., Citation2016; Magnuson et al., Citation2004). Despite the benefits of high-quality math instruction, the effectiveness of many math curricula and interventions, defined as a supplement to the general education curricula (Nelson & McMaster, Citation2019), remains untested and most intervention studies are not replicated (Makel & Plucker, Citation2014). Of the 155 math curricula, interventions, and approaches to math instruction listed in the What Works Clearinghouse (WWC), only 17 were characterized as having a potentially positive or positive effect on outcomes and only three pertained to kindergartners or first-graders (Institute of Education Sciences [IES], National Center for Education Evaluation and Regional Assistance [NCEERA], 2022). Thus, there is a clear need to identify math curricula, interventions, and approaches that are effective with early elementary school students.
Computer-Assisted Instruction
In the present study, computer-assisted instruction (CAI) in math refers to educational computer software/applications and web-based programs for providing instruction that helps students learn and apply math concepts and skills (Foster et al., Citation2018; Harskamp, Citation2014; Ran et al., Citation2021). CAI is advantageous in numerous ways: It is easy to implement, provides a standardized scope and sequence, includes adaptive algorithms that adjust instruction according to individual children’s progress, and teaches each fundamental skill to mastery (Clements & Sarama, Citation2014; NRC, Citation2009; Sarama & Clements, Citation2009). However, not all CAI programs are equally beneficial, and schools have reasonable concerns about their developmental appropriateness, implementation logistics, and alignment with core curricula, which historically interfered with widespread use of CAI during the early school years (Clements & Sarama, Citation2003; Cuban, Citation2001). However, increased remote learning over the past decade, predicted and unpredicted, has renewed interest in CAI. It is therefore increasingly important that schools select computer programs that are effective.
Reviews of the scientific literature generally conclude that CAI is most often used as a supplement to classroom instruction (Slavin & Lake, Citation2008) and can provide substantial benefits for children’s learning of math (Clements & Sarama, Citation2010; Cross et al., Citation2009; Li & Ma, Citation2010; Ragosta, Citation1982; Räsänen et al., Citation2009; Slavin & Lake, Citation2008). A National Mathematics Advisory Panel (Citation2008) review indicated that well-designed and well-implemented CAI programs can improve math performance, a conclusion supported by empirical study (e.g., Foster et al., Citation2016, Citation2018; Harskamp, Citation2014; Moradmand et al., Citation2013; Nusir et al., Citation2013; Ragosta, Citation1982). A meta-analysis of rigorous studies also found positive effects of CAI in math when used as a supplement to classroom instruction (Cheung & Slavin, Citation2013); and yet another meta-analysis examining the use of CAI for early math instruction found a moderate effect (Harskamp, Citation2014).
In addition to CAI’s viability, the recent pandemic forced public school districts to close their buildings and increase their use of CAI. Almost all teachers use computer programs for some math instruction (Gray et al., Citation2010) and the use of CAI for math instruction is expected to increase. Therefore, school personnel and policy makers need to identify effective CAI math programs, making the issue a next step for education researchers.
DreamBox Learning
DreamBox Learning, a web-based math program, is based on the National Council of Teachers of Mathematics [NCTM] (Citation2006) focal points and is aligned with the Common Core State Standards of Mathematics (NGACBP & CCSSO, 2010), Florida State Mathematics Standards, and Eureka Math, the print-based curriculum used in the school district in which this study took place. Its interactive lessons use virtual manipulatives, verbal descriptions, tables, equations, and graphs to ensure that students have the opportunity to engage in reasoning about math structures, strategies, concepts, and skills. The learning strands support the development of students’ mental structuring as their math reasoning increases in complexity and their access to multiple contexts expands (Treffers, Citation1987; Fosnot & Jacob, Citation2010). DreamBox’s kindergarten and first-grade content aims to develop conceptual understanding and skill fluency in the domains of counting and cardinality, operations and algebraic thinking, and numbers and operations in base ten, covering grade-level standards in each of the three areas. While use of the number line in its activities touches on measurement and geometry (Wang & Woodworth, Citation2011), DreamBox does not include activities focused specifically on measurement or geometry in kindergarten or first grade.
Based in part on the principles espoused in the WWC practices guides (Siegler et al., Citation2010; Woodward et al., Citation2012), DreamBox (a) assists students in monitoring and reflecting on the problem-solving process, (b) teaches students how to use visual representations, (c) teaches students to use multiple problem-solving strategies, and (d) helps students recognize and apply math concepts and notation. The features of DreamBox are also aligned with recent meta-analytic research focused on best practices for teaching math (Fuchs et al., Citation2021). For example, DreamBox provides systematic instruction to develop students’ understanding of math concepts incrementally over time (Steedly et al., Citation2008). In addition, DreamBox provides students with many opportunities to use representation and to connect them with mathematical ideas through the concrete–representational–abstract (CRA) instructional framework. The CRA instructional framework, a pedagogical approach based on multiple decades of research and conceptualization (Underhill, Citation1977; Underhill et al., Citation1980), is a graduated instructional sequence that supports students in learning math (Agrawal & Morin, Citation2016; Powell, Citation2015) and is a recommended pedagogical approach for elementary school age students (Baker et al., Citation2002; Gersten et al., Citation2009; Powell, Citation2015).
Finally, DreamBox uses the number line to facilitate learning of mathematical concepts and procedures, and in kindergarten and first grade, understanding of magnitude and operations for whole numbers, which are important for learning fractions (e.g., Dyson et al., Citation2020). Students who are proficient in math often construct a mental number line as they solve problems (e.g., Lannin et al., Citation2020) and thus gradually develop the ability to visualize a number when considering the magnitude of a number.
A series of studies have supported DreamBox’s use; however, of the 11 studies listed in a WWC Intervention Report (IES, 2013), 10 were ineligible for review due to the absence of a comparison group design and/or the absence of a single-case design. Further, DreamBox conducted eight of the 11 studies. A noteworthy exception was Wang and Woodworth’s (Citation2011) evaluation, which included 583 kindergarteners and first-graders. Many of their participants were Hispanic (87%), English learners (ELs; 81%), and eligible for free or reduced-price meals (FRPMs; 88%). Participants in Wang and Woodworth (Citation2011) who received DreamBox supplemental math instruction outperformed students who received supplemental literacy instruction on a broad test of math achievement and on its measurement and geometry subtest, but not on its other subtests. The WWC Report characterizes Wang and Woodworth’s (Citation2011) study as meeting the WWC standard of “without reservations” and as evidence of a potentially positive effect with no overriding contrary evidence, though the magnitudes of DreamBox’s effects were small (0.06–0.16).
Zearn Math
Zearn Math is a print-based curriculum and set of independent computer-based digital lessons aligned with the CCSSM (NGACBP & CCSSO, 2010), Florida State Mathematics Standards, and Eureka Math. The kindergarten and first-grade content of Zearn’s digital lessons aims to develop conceptual understanding and skill fluency in counting and cardinality, operations and algebraic thinking, numbers and operations in base ten, measurement and data, and geometry, covering grade-level standards in each of the five areas. Similar to DreamBox, Zearn follows the CRA instructional framework. Developing conceptual understanding through explicit instruction is central to the CRA instructional framework. Students transition from solving math problems (e.g., subtraction) with virtual manipulatives (e.g., unfix cubes) to solving problems with representational drawings (e.g., dots) before solving problems without support, abstractly. The present study focuses on Zearn’s digital lessons, which include lessons focused on fluency, guided practice, and independent practice.
Zearn is not yet listed in the WWC repository of math curricula and interventions. However, a recent literature review shows an increase in elementary schools adopting Zearn (e.g., PR Newswire, Citation2018a, Citation2018b, Citation2019, Citation2020). Notably, Zearn received a top rating by EdReports (Citation2017). Research on Zearn’s efficacy has just begun to emerge. Morrison et al. (Citation2019) collaborated with Zearn to evaluate its efficacy in a large urban school district. The majority of the study’s 9,000 participants were African American (58%) or Hispanic (28%), and eligible for FRPMs (90%). Participants in the Zearn condition received instruction with the print-based curriculum and its digital lessons; participants in the control condition received instruction with Eureka Math, the print-based math curriculum the school district previously used. After controlling for covariates (e.g., prior math and reading achievement, race/ethnicity, gender, and special education status), Morrison et al. (Citation2019) found no statistically significant difference between treatment and comparison group students. Although subgroup analyses were not conducted with kindergartners, subgroup analyses on first graders also failed to identify a statistically significant treatment effect. Correlational results related to the digital lessons suggested students would need to spend roughly 26.5 h on the computer-based activities in addition to their regular classroom math instruction to increase their scores on a standardized math measure by one point.
The Present Study
Roughly 4% of math intervention studies have examined the effectiveness of one intervention relative to another (Nelson & McMaster, Citation2019). As a result, the possibility that gains made by students in math intervention group are due to lack of exposure to math instruction for control group students cannot be ruled out. Wang and Woodworth’s (Citation2011) study suffers from the same threat to internal validity–the effectiveness of DreamBox compared to other math CAI programs during the early school years is unknown. Other supplemental math CAI programs, tutoring, and even math homework could be as beneficial as DreamBox. Therefore, the present study will address this limitation by providing the most rigorous test of DreamBox to date, testing the effectiveness of DreamBox relative Zearn’s digital lessons.
The lack of replication studies in education research is alarmingly low. Given the replication crisis in psychological science (see Pashler & Wagenmakers, Citation2012) and the lack of replication studies in education research (Makel & Plucker, Citation2014), there is a need to replicate intervention studies to determine what works for whom, under what conditions (Schneider, Citation2018, Citation2021). In order to help local education agencies determine which CAI math programs to adopt, the results of Wang and Woodworth (Citation2011), the first and only rigorous study of DreamBox, should be replicated. Therefore, the present study sought to determine whether key results of Wang and Woodworth (Citation2011) are robust across studies. In doing so, the results shed light
In contrast to a direct replication, which is conducted using the same methods and under the same conditions of the original investigation, the present study is a conceptual replication of Wang and Woodworth (Citation2011); that is, it uses different methods from the original study to test hypotheses (Makel & Plucker, Citation2014). Similar to the original investigation, the current study tested the same intervention when used as a supplement to regular education math instruction, used a pretest-posttest design, randomized students to experimental conditions from within their kindergarten and first-grade classroom, and used similar main effects analyses.
The present study differed from the original investigation on several important contextual and instructional dimensions. One, the geographical location varied between the two studies. Wang and Woodworth (Citation2011) investigated DreamBox in a suburban setting in California; the present study occurred in a suburban setting in Florida. Two, the population (or demographics) of participants varied between the two studies. Most participants in Wang and Woodworth (Citation2011) were Hispanic, ELs, and participated in their school’s FRPM program. In contrast, most participants in the present study were Caucasian, few were English learners, and few participated in their school’s FRPM program. Three, unlike the original investigation, which used a supplemental literacy program in the comparison condition, the present study controlled for additional time doing math using Zearn’s digital lessons.
In addition, the implementation of DreamBox varied across the two studies. In the original study, participants interacted with DreamBox during their afterschool program whereas participants in the present study interacted with DreamBox during the designated classroom time for supplemental math instruction. Five, the dosage of DreamBox varied across the two studies. Rather than receiving CAI instruction for 70 days over 16 weeks as in Wang and Woodworth (Citation2011), students in the present study received CAI for 33 days across 11 weeks of the school year. This yielded 18.5 fewer hours of CAI on average in the current study than in Wang and Woodworth (Citation2011). Six, the original study utilized the Northwest Evaluation Association’s math tests whereas the present study utilized the Research-Based Early Math Assessment (Clements et al., Citation2008). Given these differences, the present study was expected to increase the credibility and generalizability of DreamBox, as participants in the DreamBox condition were expected to outperform their peers in the Zearn condition. The current study also tested for a differential response to CAI among participants as a function of their numeracy skills prior to receiving CAI and explored possible Matthew Effects in math (cf. Bodovski & Farkas, Citation2007).
Method
Participants
Following Institutional Review Board approval by the University of South Florida (Approval #00037635), participants were recruited from a school in the southeastern U.S. Informed consent was obtained from a parent of each participant who provided assent verbally. The participating school was characterized by the following student demographics: Caucasian (82.0%), Hispanic (9.6%), African American (2.4%), Asian (3.5%), and students of two or more races (2.5%). The school population included 16.75% students with disabilities and 7.4% ELs; 28.35% of students were eligible for FRPMs.
Across two consecutive annual cohorts, two kindergarten and four first-grade teachers participated. All teachers were college educated (three had bachelor’s and three had master’s degrees), held state teaching certifications, and were non-Hispanic, White females. Because two cohorts of children participated in the study and because some teachers taught the same grade multiple years, a few teachers participated in both years. Specifically, three teachers participated both years and three participated one year, resulting in nine (four kindergarten and five first-grade) classrooms. On average, classroom teachers had 10.44 (SD = 4.33) years of experience.
The average class size was 19 students and all students received full-day programming. On average, 95% of the students were native English and 5% were native Spanish speakers. The teachers provided all of their math instruction in English and their daily math instruction ranged from 60 to 90 min (M = 74.44, SD = 13.10). Teachers reported their goals for math instruction and individual teaching opportunities on a 5-point Likert scale that reflected relative proportions of emphasis on practicing math versus understanding math concepts. Of the nine classroom teachers, 89% emphasized building conceptual understanding with some repeated practice. The remaining 11% (i.e., one classroom) emphasized practicing math and building conceptual understanding. No classrooms reported emphasizing exclusively the understanding math concepts, practicing math with some focus on math concepts, or emphasizing repeated practice and memorization of math skills.
Participating teachers described their math instruction as based on discovery learning, inquiry, and planned activities (44%); mostly through discovery, inquiry, and activities with a little explicit instruction (11%); equal amounts of discovery and explicit instruction (33%); and mostly through explicit instruction with a little discovery (11%).Footnote1 All classrooms followed the Eureka Math (Great Minds, Citation2015) curriculum, which is aligned with the CCSSM (NGACBP & CCSSO, 2010) and the Florida State Standards for Mathematics. Eureka Math aims to develop students’ conceptual understanding using mental strategies to solve problems and focus on math processes rather than a problem’s answer (Williams, Citation2015). Teachers reported following the pace provided in Eureka Math’s Curriculum Map for their grade level and adhering to the school district’s written expectations for student learning.
Parents provided active, informed consent for children to be enrolled in the study. However, all children in participating classrooms received some form of supplemental CAI. Of the 115 participants (64 kindergartners and 51 first graders), 4% were ELs and 23% were Hispanic. In terms of ethnicity, 70% were Caucasian, 7% were African American, 8% were Asian, 10% were mixed ethnicity, and 5% of parents did not report their child’s race on the demographic questionnaire. The sample was roughly half female (49%), half male (51%). Of the families, 82% reported speaking English as the primary language in the home, 4% reported speaking Spanish, and 14% reported speaking a language other than English and Spanish in the home (e.g., Arabic, Portuguese, Tagalog, Russian, German, and Serbian). Children ranged in age from 59 to 87 months (M = 72.47, SD = 6.88) when they entered the study. Of the participating children, 97% were from middle-income families while 3% were from low-income families. Thus, the present sample was different from Wang and Woodworth’s (Citation2011), which was 87% Hispanic, 4% students with disabilities, 81% ELs, and 81% eligible for FRPMs.
Research Design and Experimental Conditions
Study Design
Participants were randomized with equal probability from within each classroom to CAI in math delivered by DreamBox or Zearn. Such randomization ensured that children in the two experimental groups experienced the exact same classroom instruction and that group differences in outcomes were necessarily a consequence of the experimental conditions. The study design allowed for a rigorous test of the impact of DreamBox relative to the impact of Zearn on children’s math outcomes. Classroom teachers were blind to students’ assignments to conditions because CAI was overseen by intervention supervisors. The computerized nature of each experimental condition ensured that any positive gains associated with DreamBox and Zearn were not due to enhanced computer skills, enhanced attentional abilities, increased motivation, increased interactions with adults around CAI, or increased instructional time devoted to learning math.
General CAI Procedures
Students worked individually on computers in their school’s computer lab during the supplemental instruction portion of their math instructional block. Intervention specialists logged students in and out of their assigned online program and assisted with non-instructional aspects of supplemental CAI (e.g., behavioral supervision, technical assistance, and explanation of the CAI procedures) as needed.
CAI was delivered simultaneously to all children. To minimize background noise distractions during CAI, students wore headphones. All responses were made using an external mouse. Compared to the design of the Wang and Woodworth (Citation2011) study, which planned for 20–40 min of CAI 5 days a week for 16 weeks, the present study delivered 90 min of CAI per week (three 30-min sessions) to both cohorts of students for 11 weeks. However, participants in the second cohort did not return to school following spring break due to the COVID-19 pandemic. Posttest data were therefore not gathered on these students. See the Data Analytic Approach section below for how missing data were handled.
Computer Programs
In DreamBox, students in kindergarten and first grade choose their character and explore an adventure park. The adventure park has several areas to explore, each with its own theme (e.g., Pirate Cove, Discovering Dinosaurs, the Enchanted World of Pixies). Students are free to choose activities within the area of the adventure park they want to explore. Students earn tokens for completing activities, which can be redeemed to play games in a carnival within the DreamBox environment. As students complete activities, they also gain access to additional activities that are of increasing difficulty. The activities are leveled such that a given activity becomes more and more difficult until either all levels of the activity are successfully completed or a child selects a different activity. At any time, a student can decide to stop working on an activity, return to the adventure park, and choose a different area and activity to work on. This feature allows students to direct their own instruction, moving freely from activity to activity. The adaptive platform in DreamBox differentiates activity recommendations for each student and makes determinations about when students need additional scaffolding (and removal of scaffolding), concept development, or skill practice. Participants in the DreamBox condition were given access to all of its instructional activities, which are appropriate for students in preschool through eighth grade.
The Zearn digital lessons are activity based (i.e., the focus is on completing a specific activity). The digital activities for kindergartners are short and engaging with virtual manipulatives designed to build number sense through an intentional progression from Numbers to 5 and Numbers to 20. The first-grade digital activities are mission based and include the following: adaptive fluency activity focused on number sense, lesson-aligned fluency activity, guided practice, independent practice, and a paper exit ticket. Students can access the next digital activity in the sequence only after completing their currently assigned activity. As in DreamBox, students advance through the lessons at their own pace and the activities are leveled—a given activity becomes more and more difficult until all levels of the activity are successfully completed. As students complete activities, they earn badges, which are akin to digital stickers. Participants in the Zearn condition were given access to all of its instructional activities, which are appropriate for children in kindergarten through fifth grade.
Fidelity of Implementation
The school district restricted students’ computer access so they could only use the program in their experimental condition. Each day, the intervention specialists checked all computers and online programs to ensure proper functioning. They also supervised students’ interaction with CAI, ensured that they wore headphones, and verified that they remained on task. Reports generated online by each computer program assisted the intervention specialists in monitoring participants’ usage and learning. Such monitoring helped ensure that participants were progressing in their learning trajectories. Further, the intervention specialists maintained attendance records and logs of any technical problems. Missed sessions were due to child absences, district-wide standardized testing, holidays, or technical difficulties.
Assessment Measures and Data Collection Plan
Each participant was assessed individually over three days. Academic skills were measured at two points. Numeracy and nonverbal reasoning were assessed at Wave 1 (i.e., pretest), during the fall, preceding CAI by two or three weeks. Numeracy and geometry were assessed at Wave 2 (i.e., posttest), in the last month of the school year, following CAI by one or two weeks. Each assessment followed standardized administration and scoring procedures. During the pretest, the Research-Based Early Math Assessment (REMA) numeracy strand was administered first, followed by subtests from the Differential Ability Scale. During the posttest, the REMA’s numeracy strand was administered first, followed by the REMA geometry strand. Measures of numeracy and geometry were included in the present study because Wang and Woodworth (Citation2011) identified intervention effects for geometry and measurement in favor of students in the DreamBox condition.
The Research-Based Early Math Assessment (REMA; Clements et al., Citation2008) was chosen for three reasons. First, it is validated and designed to assess progressions in early math development (Clements et al., Citation2008). Second, it has the sensitivity to detect both differences in the early math performance (Clements et al., Citation2008) and the effects of CAI programs (e.g., Foster et al., Citation2016, Citation2018). Third, the REMA is one of the only measures that assess early numeracy and geometry and is also aligned to national standards such as those developed by the National Council of Teachers of Mathematics (Citation2000, Citation2006). The numeracy strand includes four subscales: number recognition and subitizing, composition of number, arithmetic, and number comparison and sequencing. Core math skills assessed within the numeracy strand include verbal counting, object counting, number recognition and subitizing, number comparison, number sequencing, numeral recognition, number composition and decomposition, and adding and subtracting.
To protect participants’ time away from classroom instruction, the REMA’s geometry strand was only administered at the posttest. The geometry strand includes four subscales: identifying and comparing shapes, composition of shape, measuring, and patterning. Core math skills assessed in the geometry strand include shape identification, shape composition and decomposition, comparison and congruence, construction of shapes, and transformations; measurement; and patterns. General concepts and processes such as part–whole thinking and the corresponding processes of composition and decomposition, classification, and seriation were woven throughout all of the REMA’s core areas. For a detailed description of the REMA’s development, see Clements et al. (Citation2008). Internal consistency, measured by Cronbach’s alpha—the ratio of true score variance to the total score variance—was high for the REMA’s numeracy and geometry strands (≥.92; see ).
Table 1. Simple correlations among all variables used in analyses.
The Early Number Concepts subtest of the Differential Ability Scale, second edition (DAS-II; Elliott, Citation2007), was also administered at pretest and served as an auxiliary variable in the analyses (see the Data Analytic Approach section below). This subtest measures knowledge of prenumerical and numerical concepts and requires participants to count objects and answer questions about numerical and mathematical concepts. Its internal consistency was high, .93.
Nonverbal Reasoning
To characterize the present sample of students’ nonverbal reasoning ability, the Matrices subtest was administered from the DAS-II. This subtest measures perception and application of relationships from abstract figures by having the child select a picture or figure closest to a target picture or figure, thereby completing an abstract visual pattern. Its internal consistency was good, .76.
Examiners
A team of examiners administered the assessment battery to participants individually. The team consisted of a professional development specialist, postdoctoral fellow, certified teacher, and undergraduate students. In each year of the study, examiners attended a three-day workshop led by the professional development specialist. After training and ample role-playing, examiners demonstrated competence in administration and scoring.
Data Analytic Approach
Approach for Missing Data
Consistent with Graham’s (Citation2009, Citation2012) definitions of missingness, posttest data were missing completely at random. Although the second cohort of participants (n = 54) did not complete the study due to the pandemic (i.e., missingness was systematic), missingness was unrelated to the variables measured in this study (i.e., math and nonverbal ability; see Attrition subsection below). As a result, 55 children were missing posttest scores (ns = 30 and 25 for the DreamBox and the Zearn groups, respectively). To avoid biased estimates and loss of statistical power from listwise deletion of cases missing posttest math data, full information maximum likelihood (FIML) was used in Mplus (version 8.4). FIML estimation is one of the best methods for handling missing data (Muthén et al., Citation1987; Worthke, Citation2000).
As recommended by Graham (Citation2009, Citation2012), an auxiliary variable (i.e., pretest Early Number Concepts scores from the DAS-II) was included in analyses but not in the statistical models to help recover some of the information lost to missingness. Using an auxiliary variable in conjunction with FIML allowed regression coefficients to be estimated, including all available data, thereby maintaining the variance structure and not losing observations with incomplete data (Foster et al., Citation2018). FIML handles missing data and parameter estimation in a single step, and including auxiliary variables in this step can increase precision and reduce bias in parameter estimates (e.g., Collins et al., Citation2001). Simulations ran by Collins et al. (Citation2001) demonstrated that even in the most extreme conditions tested (50% missing data in the dependent variable), bias in parameter estimates when using FIML was not significant. In the present study, 49% of each dependent variable was missing, which is below the threshold examined by Collins et al. (Citation2001).
The Impact of CAI
Following preliminary analyses, a univariate approach was used to evaluate the impact of DreamBox and Zearn on the domains of numeracy and geometry, the domains that students were expected to develop conceptual understanding and skill fluency. Pretest by group interactions were also examined to monitor the possibility of a differential response to CAI (i.e., Matthew Effects or compensatory growth). The REMA pretest numeracy scores served as the covariate in the prediction of posttest numeracy and geometry scores. Raw scores were used in all analyses and completely standardized results are reported, including R2 and Hedges g for each model.
Results
Preanalysis Analyses
Preliminary analyses of the raw data suggested there were three outliers from the second cohort of students due to incomplete administrations of the REMA at pretest. Removing these three observations reduced the analytic sample to 112 participants. displays participants’ raw scores on each measure by wave and by group. On average, norm-referenced standard scores suggested that participants had high numeracy (M = 128.97, SD = 21.63) but low nonverbal reasoning (M = 72.93, SD = 8.77) ability as measured by the DAS-II subtests, which have a mean of 100 and standard deviation of 10. On average, participants interacted with DreamBox and Zearn between 230 and 830 min (M = 604.69, SD = 160.14). Participants in both conditions showed statistically significant improvement from pre- to post-test for numeracy (F = 69.33, p < .0001; F = 34.39, p < .0001, respectively) and geometry (F = 16.14, p < .001; F = 4.34, p = .05), indicating they significantly increased their math scores across the school year.
Table 2. Descriptive statistics according to experimental condition.
Intraclass Correlations
Intraclass correlations (ICCs), which measure the total variance of a variable accounted for by clustering (e.g., classrooms), were computed for the full sample’s math outcomes using models conditional on pretest numeracy scores. The ICCs for these conditional models were .00 for the posttest numeracy and geometry scores. This suggests that very little if any variance in posttest math scores were due to classroom-level differences. Given the low ICCs, the small number of classrooms, and that the effect of classroom nesting was controlled methodologically, analyses of math outcomes did not model the nesting of students within classrooms.
Attrition
Of the 112 participants, 54 were unable to complete the posttest assessment due to the pandemic. Only one other participant, who was in the DreamBox condition, was unable to complete the study due to relocating after the pretest assessment. ANOVAs were used to compare participants who completed the posttest to those who did not. These groups evidenced equivalent pretest distributions for math (Fs = 0.09 and 0.52, ps = .77 to .47, respectively) and nonverbal ability (F = 0.04, p = .84). Since the two groups of participants were equivalent on all three pretest measures, all observations were retained for subsequent analyses.
Pretest Differences
To verify the success of random assignment on demographic variables and pretest competencies, chi-square and ANOVAs were used. The groups did not demonstrate differences for sex ( = 0.13, p = .72), ethnicity (
= 1.83, p = .18), or race (
= 7.38, p = .12). The groups also did not evidence differences for age in months (F = 1.89, p = .17), nonverbal ability (F = 0.02, p = .88), numeracy (F = 0.01 and 0.28, p = .91 and .60 for the REMA and DAS, respectively), or the time spent working with the computer programs (F = 0.84, p = .36). Therefore, randomization to experimental conditions was successful.
The Prediction of Posttest Numeracy
On average, students in the DreamBox condition gained 13.14 points for numeracy from pretest to posttest, which was 4.46 points more than that gained by Zearn students (see ). reports the prediction of posttest numeracy scores from pretest numeracy scores and group (Model 1). The regression estimates from these models represent the unique effects of each variable, adjusting for all other terms in the model. Model 1 indicated that pretest numeracy, but not experimental condition, was a statistically significant predictor of posttest numeracy. A closer look at the confidence interval around the group effect suggest that the population value is between −0.04 and 0.24. Together, these predictors accounted for 70% (R2 = .70) of the variance in posttest numeracy and Hedges g was 0.32 [−.05, .69], which exceeds the WWC threshold of a 0.25 effect size to be considered of “substantive importance” ([IES, NCEERA, 2022). Thus, DreamBox appeared to be associated with substantively important positive effects on posttest numeracy scores, but null hypothesis statistical testing did not confirm this relationship.
Table 3. Prediction of posttest math achievement.
Expanding Model 1 by including the interaction of group with pretest numeracy (Model 2) improved the prediction of posttest numeracy. Participants with higher pretest numeracy scores in the DreamBox condition made greater improvements than participants with lower pretest numeracy scores in the DreamBox condition and participants in the Zearn condition (see ). The interaction term was statistically significant and accounted for an additional 9% of the variance in posttest numeracy scores relative to Model 1 (ΔR2 = .09). A closer look at the confidence interval around the interaction effect suggests that the population value is between 0.00 and 1.32, in favor of students in the DreamBox condition.
The Prediction of Posttest Geometry
On average, students in the DreamBox condition scored 1.25 points higher than students in the Zearn condition. (see Model 1) details the results from the prediction of posttest geometry scores compared to pretest numeracy scores and group. Although the group of predictors explained 29% of the variance in posttest geometry, group was not a statistically significant predictor of this outcome and the confidence interval ranged from −0.19 to 0.25. Hedges g corresponding to the group effect was 0.14 [−.23, .51].
Expanding Model 1 by including the interaction of pretest numeracy scores and group did not result in improved prediction of posttest geometry. Thus, neither group nor its interaction with pretest numeracy was a statistically significant predictor of geometry at posttest, although the interaction term accounted for 15% additional variance in posttest geometry scores relative to Model 1 (ΔR2 = .15). In looking at the confidence interval for the interaction effect, the width of the lower (−1.17) and upper (1.16) limits suggests that this estimate is less accurate than the other estimates reported in the present study.
Discussion
The COVID-19 pandemic forced school districts to close their doors, provide instruction online, and increase their use of CAI. It is therefore paramount that schools utilize the data from empirical studies when choosing supplemental CAI math programs. School personnel must maintain high expectations for all students and increase their learning opportunities (NCTM, Citation2014). Few studies, however, have considered the effects of one math program relative to another (Nelson & McMaster, Citation2019). The present study accomplished this objective by conducting a conceptual replication of Wang and Woodworth (Citation2011), providing the most rigorous test DreamBox to date, testing its effectiveness to another CAI math program, Zearn. In doing so, this study investigated whether or not the results of the original study could be obtained when varying several important contextual and instructional dimensions; thereby providing evidence about what works for whom, under what conditions.
Compared to Wang and Woodworth (Citation2011) (n = 583), the present study had a relatively small number of observations (n = 112), which likely affected the null hypothesis significance testing (NHST) results. Namely, despite the effect size for numeracy (g = 0.32) exceeding those reported in Wang and Woodworth (Citation2011; 0.06 to 0.16) and the 0.25 threshold to be considered substantively important (IES, NCEERA, 2014), the main effect for group was not statistically significant. Likewise, the effect size for geometry (g = 0.14) was similar to the effect size (0.16) reported in Wang and Woodworth (Citation2011), but again, the main effect for group was not statistically significant. Given that the null hypothesis can often be rejected with a large enough sample size (for review, see Jones & Sommerlund, Citation2007), measures of effect size provide one way to interpret an intervention’s substantive significance; and unlike NHST, measures of effect size are independent of sample size. The measures of effect size in the present study strengthen the argument that DreamBox is effective; however, it remains possible that the true effect of DreamBox is smaller than that reported in the present study. Based on the confidence interval estimates, the true effect of DreamBox is likely between −0.05 and 0.69 for numeracy and between −0.23 and 0.51 for geometry.
Despite the lack of statistically significant effects, students in the DreamBox condition gained more points for numeracy from pretest to posttest than student in the Zearn condition. The differences in CAI effects, in favor of students in the DreamBox condition, may be due to two mechanisms. First, the adaptive learning platform in DreamBox helps students make sense of math whether they start below, at, or above grade level. The adaptive learning platform’s assessment system monitors proficiency as students choose activities within and across mathematical domains. The assessment system uses data to make interim determinations about student understanding and progress, which in turn informs real-time software decisions about scaffolding and instructional recommendations. This results in students choosing a variety of lessons in such a way that they experience interleaved lessons and practice, which has been shown to be an effective approach for improving mathematical learning (Rohrer et al., Citation2014).
The second mechanism that may be responsible for differences in the CAI effects is the motivational environment of DreamBox. After students choose their character, DreamBox provides an exploratory environment where they can choose to work in different areas of the adventure park and on different activities within each area. This results in self-directed learning, which has positive impacts on learning math (e.g., Sumantri & Satriani, Citation2016; Yang & Li, Citation2013). In addition, when students’ complete activities successfully in DreamBox, they gain access to activities of increasing difficulty and earn tokens, a form of positive reinforcement, which can be redeemed to play games at the carnival in DreamBox. Token economies are not new to education settings. They have been used successfully with students to decrease disruptive behaviors, increase academic engagement, and increase academic achievement (see Matson & Boisjoli, Citation2009, for review). Whether through self-directed learning or positive reinforcement, the motivational environment in DreamBox may have led to the observed differences in the present study, in favor of students in the DreamBox condition.
In addition to understanding the mechanisms that may be responsible for differences in the effects between the two CAI programs, some of the differences between the present study and Wang and Woodworth’s (Citation2011) original evaluation warrant consideration. First, the present sample was drastically different from that in the original evaluation of DreamBox. Of the students in the original evaluation, 81% were ELs and 88% were eligible for FRPMs compared to 4% and 3% of the present sample, respectively. Considering the effect size and confidence interval reported for numeracy in the present study, it is possible that the effects of DreamBox are robust across populations; however, in the absence of statistically significant findings this inference is attenuated. It is unclear if DreamBox is more effective for ELsFootnote2 from low-income backgrounds or if DreamBox is more effective for middle-income students who are not ELs.
Second, the present study provided a more stringent test of DreamBox, which Wang and Woodworth (Citation2011) compared to an online literacy program. Wang and Woodworth (Citation2011) could not rule out the threat to internal validity—that math gains in their study could be attributed not to DreamBox but to additional time spent learning math. In the present study, the results are a direct result of using DreamBox relative to Zearn. Third, the average dose of DreamBox delivered in the present study was 18.5 h (range = 6.83–30.17 h) less than in Wang and Woodworth (Citation2011). Rather than providing DreamBox to students five days a week for 20–40 min a day for 16 weeks (33 days), the present study provided just three 30-min sessions a week for only 11 weeks (70 days). Although more research is needed, these findings are encouraging and suggest that school personnel may be able to see substantively important positive effects on student’s numeracy scores with a substantially lower time commitment. This inference is consistent with Nelson and McMaster (Citation2019), who demonstrated that numeracy interventions delivered for longer durations of time yield significantly smaller effects than shorter interventions.
Also worth noting, the effect size corresponding to the impact of DreamBox on numeracy outcomes in the present study is consistent with the extant research of CAI in math (e.g., Cheung & Slavin, Citation2013; Foster et al., Citation2016, Citation2018; Harskamp, Citation2014). For example, Foster et al. (Citation2018) evaluated the impact of Building Blocks math software’s (Clements & Sarama, Citation2016) numeracy activities when used to supplement kindergartners’ regular education math instruction. Using Building Blocks improved numeracy scores as measured by the REMA (g = 0.43). While the effect on numeracy was slightly higher in Foster et al. (Citation2018) than in the present study, this may be due to the REMA being more closely aligned to the instructional activities in Building Blocks than in DreamBox. Notably, the instructional designs of Building Blocks and DreamBox are based on developmental learning trajectories in math, which research has shown to be effective (e.g., Clements & Sarama, Citation2016; Clements et al., Citation2011; Foster et al., Citation2016, Citation2018).
Compared to small group (non-CAI) numeracy instruction, the results of the present study bode well, producing similar or better numeracy outcomes. For example, Dyson et al. (Citation2015) found that a supplemental small group numeracy intervention had statistically significant positive effects on kindergartners’ number sense, arithmetic fluency, and math calculation (g = 0.32–0.82). Using teachers in small group instruction can be advantageous and lead to similar if not stronger math outcomes than CAI. Supplemental small group instruction, however, can be teacher intensive. In contrast, teachers can implement supplemental CAI with DreamBox for an entire classroom with minimal oversight while not interrupting regular classroom math instruction or other academic programming if implemented as in the present study (i.e., by delivering CAI during students’ math block). Despite larger effect sizes reported in Dyson et al. (Citation2015), the effect sizes reported in the present study are educationally meaningful.
The pretest numeracy by group interaction suggested that participants with higher levels of pretest numeracy in the DreamBox condition benefited more than either participants with lower levels of pretest numeracy in the DreamBox condition or participants in the Zearn condition. The statistically significant interaction term is evidence of a Matthew Effect rather than a compensatory achievement trajectory, accounting for an additional 9% of the variance in posttest numeracy scores over that accounted for by pretest numeracy scores and group. On average, the low numeracy performance of participants in the Zearn condition was stable from pretest to posttest, and the gap in their numeracy performance compared to that of their peers in the DreamBox condition grew over the school year. In addition, participants in the DreamBox condition with higher rather than lower levels of numeracy experienced the greatest benefits.
The pretest numeracy by group interaction is consistent with developmental and cognitive theories (e.g., cognitive skill-building framework), which predict that early knowledge of math leads to later achievement because early numerical skills help students acquire math concepts (Aunola et al., Citation2004; Entwisle & Alexander, Citation1990; Gersten et al., Citation2009; Jordan et al., Citation2009; Watts et al., Citation2014). This finding also points to a complex interaction between the online learning environment and numeracy proficiency. Consistent with the bioecological model of human development (Bronfenbrenner & Morris, Citation2006), participants with higher pretest numeracy may have been able to complete more lessons within DreamBox and experienced more positive feedback in the online environment, thereby mastering more numerical concepts than their peers.
According to the NRC (Citation2009), understanding numeracy concepts better enables children to solve problems that involve measurement, data analysis, and geometry. Some results in the present study are consistent with the NRC’s (2009) conclusion, as the correlations between numeracy and geometry were .52 and .53, suggesting that numeracy accounted for 27–28% of variance in geometry. Pretest numeracy was also a statistically significant predictor of posttest geometry. These findings are consistent with that of others who have argued that children need to understand length in order to solve simple measurement problems, which require quantifying the distance between an object’s two endpoints (Clements & Stephan, Citation2004). Similarly, solving simple geometry problems may be impossible without understanding distance and length (Spelke et al., Citation2010). However, the present study is among the first to empirically demonstrate that numeracy predicts geometry, but there was not conclusive evidence that numeracy instruction led to improved posttest geometry scores.
Implications
Notably, DreamBox activities are aligned with grade-level instructional standards—an important consideration for both developers of educational computer programs and consumers, making it an attractive option for school personnel. The present study is also the most rigorous test of DreamBox to date and one of the few studies to include two math programs and to evaluate their relative effects on student outcomes (for review, see Nelson & McMaster, Citation2019). Used as a supplement to daily classroom instruction, DreamBox leads to substantively important positive effects (cf., IES, NCEERA, 2022) on numeracy outcomes for kindergarteners and first-graders. However, NHST did not support the use of DreamBox over Zearn, even though the effect sizes reported in this study were comparable to, if not stronger than, those in Wang and Woodworth (Citation2011), consistent with similar studies of CAI (e.g., Foster et al., Citation2018). As that may be, it remains possible that the true effect of DreamBox is smaller than that reported in the present study, which were not supported through NHST. More research is needed to determine if DreamBox is a good option for school personnel interested in fostering the numerical competencies of students from diverse backgrounds.
Notably, this study finds evidence of a Matthew Effect specific to numeracy and the benefits afforded to students from a numeracy intervention. Namely, students in the DreamBox condition increasingly benefited from intervention as their pretest numeracy scores improved. Although much of the discussion of the Matthew Effect has been reserved for reading research and associated with Stanovich (Citation1986), Bodovski and Farkas (Citation2007) found that students’ knowledge of math at the beginning of kindergarten was proportional to their learning of math across elementary school. Evidence of the Matthew Effect specific to numeracy cannot be overlooked because numeracy is the foundation from which students develop increasingly complex understanding of math (e.g., NRC, Citation2009). In addition, evidence of the Matthew Effects means further individualized interventions are needed to support lower achieving students’ early math learning (Aunola et al., Citation2004). Providing differentiated instruction tailored to students’ needs in early elementary school can improve students’ academic trajectories and, ultimately, help ensure that all students graduate high school prepared for college, career, and life.
The present study also has implications for future replication studies. While the study was not able to conclude unequivocally that DreamBox is effective, a logical next step is to conduct an efficacy study of DreamBox that includes Zearn and a curricular contrast CAI condition. This would permit stronger inferences on the efficacy of the computer programs. In addition, planning a sufficiently large enough study that includes ELs and non-ELs could investigate whether DreamBox is differentially related to improved numeracy in these groups of children. Given the findings of the present and the original study, a future efficacy study could benefit from including the measures of math used in the original and present study, thereby ruling out the potential that lack of statistically significant findings may be due to measures used in each study.
Limitations and Future Directions
While this study has several strengths, there are a few limitations. First, more research is needed to evaluate the effectiveness of DreamBox across different populations of children, as some research suggests that children who have extensive educational needs and require intensive math intervention may not benefit from DreamBox (see Powell & Fuchs, Citation2015). In this study, 13 children had Individualized Education Plans (IEPs) and five received English language services. Services for children who have an IEP or who are ELs (e.g., pullout two to three times a week for small group math instruction) may affect their response to supplemental math intervention. The data in the study, however, do not permit close examination of the influence of math instructional practices used in classrooms. While such services can moderate the effects of a math program, they do not confound the results from the models of main effects because classroom instruction was methodologically controlled. Future studies could consider such moderators and distributed dosage effects, which the present study was not powered to identify.
Second, some readers may be concerned that Zearn included lessons on geometry and measurement while DreamBox did not when a study measure focused on geometry and measurement. As a result, the students that interacted with Zearn may have received a larger dose of geometry and measurement lessons than students that interacted with DreamBox. However, the effect size, not NHST, for geometry supported the use of DreamBox. This may be due to the Zearn lessons providing minimal coverage of geometry. Zearn is aligned with the CCSSM (NGACBP & CCSSO, 2010) and the Florida State Math Standards. Both sets of standards prioritize counting and cardinality, operations and algebraic thinking, number and operations in base ten, and data analysis and probability over geometry and measurement. Therefore, concerns that Zearn, but not DreamBox, included geometry and measurement lessons does not detract from the findings of the present study.
Third, future research may benefit from including additional measures of math achievement. Demonstrating the effects of DreamBox on measures of high-stakes math assessments accompanied by cost-effectiveness analyses may increase school personnel’s confidence when making data-driven decisions and purchasing supplemental math programs. Fourth, student motivation was not measured in the present study. Future studies could include a measure to capture motivation during the study, thereby providing stronger evidence that students using DreamBox are more motivated than students using Zearn. Fifth, this study may have been underpowered for NHST, stronger substantively important positive effects on numeracy and similar effects on geometry were shown than in prior research (Wang & Woodworth, Citation2011) in the absence of statistically significant NHST. Future studies comparing the effects of two math programs could benefit from recruiting more participants than in the present study, which was impacted by a global pandemic. Although the measures of effect size strengthen the argument that DreamBox is effective, it remains possible that the true effect of DreamBox is smaller than that reported in the present study.
Open Research Statements
This manuscript was not required to disclose open research practices, as it was initially submitted prior to JREE mandating open research statements in April 2022.
Supplemental Material
Download Zip (14.4 KB)Acknowledgments
The author wishes to acknowledge the invaluable support and contributions of the research department, administrators, teachers, and students of the Pasco County School District in Florida. Without their support and that of my research team led by Dr. Anna Garcia, this project would not have been possible. I would also like to thank Dr. Sara Smith and the peer reviewers for their constructive feedback. Finally, I would like to thank my institution whose support made this study possible. Thank you!
Correction Statement
This article has been corrected with minor changes. These changes do not impact the academic content of the article.
Notes
1 Percents do not equal 100% due to rounding.
2 English learners are defined as students who meet the Title III criteria of the Every Student Succeeds Act of 2015 to receive supplemental services in the public school system to improve their English language proficiency and academic achievement.
References
- Agrawal, J., & Morin, L. L. (2016). Evidence-based practices: Applications of concrete representational abstract framework across math concepts for students with mathematics disabilities. Learning Disabilities Research & Practice, 31(1), 34–44. https://doi.org/10.1111/ldrp.12093
- Aunola, K., Leskinen, E., Lerkkanen, M. K., & Nurmi, J. E. (2004). Developmental dynamics of math performance from preschool to grade 2. Journal of Educational Psychology, 96(4), 699–713. https://doi.org/10.1037/0022-0663.96.4.699
- Baker, S. K., Gersten, R. M., & Lee, D.-S. (2002). A synthesis of empirical research on teaching mathematics to low-achieving students. The Elementary School Journal, 103(1), 51–73. https://doi.org/10.1086/499715
- Bodovski, K., & Farkas, G. (2007). Mathematics growth in early elementary school: The roles of beginning knowledge, student engagement, and instruction. The Elementary School Journal, 108(2), 115–130. https://doi.org/10.1086/525550
- Bronfenbrenner, U., & Morris, P. A. (2006). The bioecological model of human development. In R. M. Lerner & W. Damon (Eds.), Handbook of child psychology: Theoretical models of human development: Vol. 1 (6th ed., pp. 793–828). John Wiley & Sons Inc.
- Cheung, A., & Slavin, R. E. (2013). The effectiveness of educational technology applications for enhancing mathematics achievement in K-12 classrooms: A meta-analysis. Educational Research Review, 9, 88–113. https://doi.org/10.1016/j.edurev.2013.01.001
- Clements, D. H., & Sarama, J. (2003). Strip mining for gold: Research and policy in educational technology—A response to “Fool’s Gold. Educational Technology Review, 11(1), 7–69. https://www.learntechlib.org/p/17793
- Clements, D. H., & Sarama, J. (2016). Building Blocks Software [Computer software]. McGraw-Hill Education. (Original work published 2008)
- Clements, D. H., & Sarama, J. (2009). Learning and teaching early math: The learning trajectories approach. Taylor & Francis.
- Clements, D. H., & Sarama, J. (2010). Technology. In V. Washington & J. Andrews (Eds.), Children of 2020: Creating a better tomorrow (pp. 119–123). Council for Professional Recognition/National Association for the Education of Young Children.
- Clements, D. H., & Sarama, J. (2011). Early childhood mathematics intervention. Science (New York, N.Y.), 333(6045), 968–970. http://science.sciencemag.org/content/333/6045/968.full https://doi.org/10.1126/science.1204537
- Clements, D. H., & Sarama, J. (2014). Learning and teaching early math: The learning trajectories approach (2nd ed.). Routledge.
- Clements, D. H., Sarama, J., & Liu, X. H. (2008). Development of a measure of early mathematics achievement using the Rasch model: The Research-Based Early Maths Assessment. Educational Psychology, 28(4), 457–482. https://doi.org/10.1080/01443410701777272
- Clements, D. H., Sarama, J., Spitler, M. E., Lange, A. A., & Wolfe, C. B. (2011). Mathematics learned by young children in an intervention based on learning trajectories: A large-scale cluster randomized trial. Journal for Research in Mathematics Education, 42(2), 127–166. https://doi.org/10.5951/jresematheduc.42.2.0127
- Clements, D. H., & Stephan, M. (2004). Measurement in pre-K to grade 2 mathematics. In D. H. Clements & J. Sarama (Eds.), Engaging young children in mathematics: Standards for early childhood mathematics education (pp. 299–320). Erlbaum.
- Collins, L. M., Schafer, J. L., & Kam, C.-M. (2001). A comparison of inclusive and restrictive strategies in modern missing data procedures. Psychological Methods, 6(4), 330–351. https://doi.org/10.1037/1082-989X.6.4.330
- Cross, C. T., Woods, T. A., & Schweingruber, H. (Eds.). (2009). Mathematics learning in early childhood: Paths toward excellence and equity. National Academies Press. https://doi.org/10.17226/12519
- Cuban, L. (2001). Oversold and underused. Harvard University Press.
- Dyson, N., Jordan, N. C., Beliakoff, A., & Hassinger-Das, B. (2015). A kindergarten number-sense intervention with contrasting practice conditions for low-achieving children. Journal for Research in Mathematics Education, 46(3), 331–370. https://doi.org/10.5951/jresematheduc.46.3.0331
- Dyson, N. I., Jordan, N. C., Rodrigues, J., Barbieri, C., & Rinne, L. (2020). A fraction sense intervention for sixth graders with or at risk for mathematics difficulties. Remedial and Special Education, 41(4), 244–254. https://doi.org/10.1177/0741932518807139
- EdReports. (2017, October 17). Math K-8 summary of alignment and usability. https://edreports.org/reports/overview/zearn-2016
- Elliott, C. D. (2007). Differential ability scales (2nd ed.). The Psychological Corporation.
- Entwisle, D. R., & Alexander, K. L. (1990). Beginning school math competence: Minority and majority comparisons. Child Development, 61(2), 454–471. https://doi.org/10.2307/1131107
- Fosnot, C. T., & Jacob, B. (2010). Young mathematicians at work: Constructing algebra (1st ed.). Heinemann.
- Foster, M. E., Anthony, J. A., Clements, D. H., Sarama, J., & Williams, J. M. (2016). Improving mathematics learning of kindergarten students through computer-assisted instruction. Journal for Research in Mathematics Education, 47(3), 206–232. https://doi.org/10.5951/jresematheduc.47.3.0206
- Foster, M. E., Anthony, J. L., Clements, D. H., Sarama, J. H., & Williams, J. M. (2018). Hispanic dual language learning kindergarten students’ response to a numeracy intervention: A randomized control trial. Early Childhood Research Quarterly, 43, 83–95. https://doi.org/10.1016/j.ecresq.2018.01.009
- Fuchs, L. S., Newman-Gonchar, R., Schumacher, R., Dougherty, B., Bucka, N., Karp, K. S., Woodward, J., Clarke, B., Jordan, N. C., Gersten, R., Jayanthi, M., Keating, B., & Morgan, S. (2021). Assisting students struggling with mathematics: Intervention in elementary grades. National Center for Education Evaluation and Regional Assistance (NCEE), Institute of Education Sciences, U.S. Department of Education. http://whatworks.ed.gov/
- Geary, D. C., Hoard, M. K., Nugent, L., & Bailey, D. H. (2012). Mathematical cognition deficits in children with learning disabilities and persistent low achievement: A five-year prospective study. Journal of Educational Psychology, 104(1), 206–223. https://doi.org/10.1037/a0025398
- Gersten, R., Chard, D. J., Jayanthi, M., Baker, S. K., Morphy, P., & Flojo, J. (2009). Mathematics instruction for students with learning disabilities: A meta-analysis of instructional components. Review of Educational Research, 79(3), 1202–1242. https://doi.org/10.3102/0034654309334431
- Graham, J. (2009). Missing data analysis: Making it work in the real world. Annual Review of Psychology, 60, 549–576. https://doi.org/10.1146/annurev.psych.58.110405.085530
- Graham, J. (2012). Missing data: Analysis and design. Springer.
- Gray, L., Thomas, N., & Lewis, L. (2010). Teachers’ use of educational technology in U.S. public schools: 2009 (NCES 2010-040). National Center for Education Statistics, Institute of Education Sciences, U.S. Department of Education. rb.gy/klfghu.
- Great Minds. (2015). Eureka mathematics curriculum: A story of units. https://greatminds.org/math
- Harskamp, E. (2014). The effects of computer technology on primary school students’ mathematics achievement: A meta-analysis. In S. Chinn (Ed.), The Routledge international handbook of dyscalculia (pp. 383–392). Routledge.
- Huang, F. L., Moon, T. R., & Boren, R. (2014). Are the reading rich getting richer? Testing for the presence of the Matthew Effect. Reading & Writing Quarterly, 30(2), 95–115. https://doi.org/10.1080/10573569.2013.789784
- Institute of Education Sciences, What Works Clearinghouse. (2013). What Works Clearinghouse intervention report. U.S. Department of Education. https://ies.ed.gov/ncee/wwc/Docs/InterventionReports/wwc_dreambox_121013.pdf
- Institute of Education Sciences, National Center for Education Evaluation and Regional Assistance. (2022). What Works Clearinghouse. U.S. Department of Education. https://ies.ed.gov/ncee/wwc/
- Jones, A., & Sommerlund, B. (2007). A critical discussion of null hypothesis significance testing and statistical power analysis within psychological research. Nordic Psychology, 59(3), 223–230. https://doi.org/10.1027/1901-2276.59.3.223
- Jordan, N. C., Kaplan, D., Ramineni, C., & Locuniak, M. N. (2009). Early math matters: Kindergarten number competence and later mathematics outcomes. Developmental Psychology, 45(3), 850–867. https://doi.org/10.1037/a0014939
- Krajewski, K., & Schneider, W. (2009). Early development of quantity to number-word linkage as a precursor of mathematical school achievement and mathematical difficulties: Findings from a four-year longitudinal study. Learning and Instruction, 19(6), 513–526. https://doi.org/10.1016/j.learninstruc.2008.10.002
- Lannin, J., van Garderen, D., & Kamuru, J. (2020). Building a strong conception of the number line. Mathematics Teacher: Learning and Teaching PK-12, 113(1), 18–24. https://doi.org/10.5951/MTLT.2019.0061
- Leppänen, U., Niemi, P., Aunola, K., & Nurmi, J. (2004). Development of reading skills among preschool and primary school pupils. Reading Research Quarterly, 39(1), 72–93. https://doi.org/10.1598/RRQ.39.1.5
- Li, Q., & Ma, X. (2010). A meta-analysis of the effects of computer technology on school students’ mathematics learning. Educational Psychology Review, 22(3), 215–243. https://doi.org/10.1007/s10648-010-9125-8
- Magnuson, K. A., Meyers, M. K., Ruhm, C. J., & Waldfogel, J. (2004). Inequality in preschool education and school readiness. American Educational Research Journal, 41(1), 115–157. https://doi.org/10.3102/00028312041001115
- Makel, M. C., & Plucker, M. J. (2014). Facts are more important than novelty: Replication in education sciences. Educational Researcher, 43(6), 304–316. https://doi.org/10.3102/0013189X14545513
- Matson, J. L., & Boisjoli, J. A. (2009). The token economy for children with intellectual disability and/or autism: A review. Research in Developmental Disabilities, 30(2), 240–248. https://doi.org/10.1016/j.ridd.2008.04.001
- Methe, S. A., Hojnoski, R., Clarke, B., Owens, B. B., Lilley, P. K., Politylo, B. C., White, K. M., & Marcotte, A. M. (2011). Innovations and future directions for early numeracy curriculum-based measurement: Commentary on the special series. Assessment for Effective Intervention, 36(4), 200–209. https://doi.org/10.1177/1534508411414154
- Moradmand, N., Datta, A., & Oakley, G. (2013). My maths story: An application of a computer-assisted framework for teaching mathematics in the lower primary years [Paper presentation]. Society for Information Technology and Teacher Education International Conference. http://www.editlib.org/p/48603
- Morgan, P. L., Farkas, G., & Wu, Q. (2009). Five-year growth trajectories of kindergarten children with learning difficulties in mathematics. Journal of Learning Disabilities, 42(4), 306–321. https://doi.org/10.1177/0022219408331037
- Morrison, J. R., Wolf, B., Ross, S. M., Risman, K. L., & McLemore, C. C. (2019). Efficacy study of Zearn Math in a large urban school district. John Hopkins University. http://jhir.library.jhu.edu/handle/1774.2/62395
- Muthén, B., Kaplan, D., & Hollis, M. (1987). On structural equation modeling with data that are not missing completely at random. Psychometrika, 52(3), 431–462. https://doi.org/10.1007/BF02294365
- National Council of Teachers of Mathematics. (2000). Principles and standards for school mathematics.
- National Council of Teachers of Mathematics. (2006). Curriculum focal points for pre-kindergarten through grade 8 mathematics: A quest for coherence.
- National Council of Teachers of Mathematics. (2014). Access and equity in mathematics education. https://www.nctm.org/Standards-and-Positions/Position-Statements/Access-and-Equity-in-Mathematics-Education/
- National Governors Association Center for Best Practices, Council of Chief State School Officers. (2010). Common core state standards. https://learning.ccsso.org/common-core-state-standards-initiative
- National Mathematics Advisory Panel. (2008). Foundations for success: The final report of the National Mathematics Advisory Panel. U.S. Department of Education. https://www2.ed.gov/about/bdscomm/list/mathpanel/report/final-report.pdf
- National Research Council. (2009). Mathematics in early childhood: Learning paths toward excellence and equity. National Academy Press.
- Nelson, G., & McMaster, K. L. (2019). The effects of early numeracy interventions for students in preschool and early elementary: A meta-analysis. Journal of Educational Psychology, 111(6), 1001–1022. https://doi.org/10.1037/edu0000334
- Nguyen, T., Watts, T. W., Duncan, G. J., Clements, D. H., Sarama, J. S., Wolfe, C., & Spitler, M. E. (2016). Which preschool mathematics competencies are most predictive of fifth grade achievement? Early Childhood Research Quarterly, 36, 550–560. https://doi.org/10.1016/j.ecresq.2016.02.003
- Nusir, S., Alsmadi, I., Al-Kabi, M., & Sharadgah, F. (2013). Studying the impact of using multimedia interactive programs on children’s ability to learn basic math skills. E-Learning and Digital Media, 10(3), 305–319. https://doi.org/10.2304/elea.2013.10.3.3
- Pashler, H., & Wagenmakers, E. J. (2012). Introduction to the special section on replicability in psychological science: A crisis of confidence? Perspectives on Psychological Science, 7(6), 528–530. https://doi.org/10.1177/1745691612465253
- Powell, S. R. (2015). Connecting evidence-based practice with implementation opportunities in special education mathematics preparation. Intervention in School and Clinic, 51(2), 90–96. https://doi.org/10.1177/1053451215579269
- Powell, S. R., & Fuchs, L. S. (2015). Intensive intervention in mathematics. Learning Disabilities Research & Practice, 30(4), 182–192. https://doi.org/10.1111/ldrp.12087
- PR Newswire. (2018a, June 19). Bossier Parish adopting top-rated Zearn Math curriculum across all elementary schools for 2018-19. PR Newswire USA.
- PR Newswire. (2018b, July 31). Rochester City School District partners with Zearn Math to improve student math achievement. PR Newswire USA.
- PR Newswire. (2019, November 25). Zearn Math becomes the only math curriculum recommended for all grades K-5 in New Mexico. PR Newswire USA.
- PR Newswire. (2020, January 29). Zearn Math K-5 receives highest level of rating—‘Recommended Primary’—from Utah State Board of Education. PR Newswire USA.
- Provasnik, S., Malley, L., Stephens, M., Landeros, K., Perkins, R., & Tang, J. H. (2016). Highlights from TIMSS and TIMSS Advanced 2015: Mathematics and science achievement of U.S. students in grades 4 and 8 and in advanced courses at the end of high school in an international context (NCES 2017-002). U.S. Department of Education, National Center for Education Statistics. http://nces.ed.gov/pubsearch
- Ragosta, M. (1982). Computer-assisted instruction and compensatory education: The ETS/LAUSD study. The final report. U.S. Department of Education, National Institute of Education. https://files.eric.ed.gov/fulltext/ED222169.pdf
- Ran, H., Kasli, M., & Secada, W. G. (2021). A meta-analysis on computer technology intervention effects on mathematics achievement for low-performing students in K-12 classrooms. Journal of Educational Computing Research, 59(1), 119–153. https://doi.org/10.1177/0735633120952063
- Räsänen, P., Salminen, J., Wilson, A. J., Aunio, P., & Dehaene, S. (2009). Computer-assisted intervention for children with low numeracy skills. Cognitive Development, 24(4), 450–472. https://doi.org/10.1016/j.cogdev.2009.09.003
- Rohrer, D., Dedrick, R. F., & Burgess, K. (2014). The benefit of interleaved mathematics practice is not limited to superficially similar kinds of problems. Psychonomic Bulletin & Review, 21(5), 1323–1330. https://doi.org/10.3758/s13423-014-0588-3
- Sarama, J., & Clements, D. H. (2009). Early childhood mathematics education research: Learning trajectories for young children. Routledge.
- Schleicher, A. (2018). PISA 2018: Insights and interpretations. Organization for Economic Co-operation and Development Publishing. rb.gy/ti2xdk
- Schneider, M. (2018, December 17). Message from IES director: A more systematic approach to replicating research. https://ies.ed.gov/director/remarks/12-17-2018.asp
- Schneider, M. (2021, April 7). Reflecting on three years at IES. Institute of Education Sciences. https://ies.ed.gov/director/remarks/4-7-2021.asp
- Siegler, R., Carpenter, T., Fennell, F., Geary, D., Lewis, J., Okamoto, Y., Thompson, L., & Wray, J. (2010). Developing effective fractions instruction for kindergarten through 8th grade: A practice guide (NCEE #2010-4039). National Center for Education Evaluation and Regional Assistance, Institute of Education Sciences, U.S. Department of Education.
- Slavin, R. E., & Lake, C. (2008). Effective programs in elementary mathematics: A best-evidence synthesis. Review of Educational Research, 78(3), 427–515. https://doi.org/10.3102/0034654308317473
- Spelke, E., Lee, S. A., & Izard, V. (2010). Beyond core knowledge: Natural geometry. Cognitive Science, 34(5), 863–884. https://doi.org/10.1111/j.1551-6709.2010.01110.x
- Stanovich, K. E. (1986). Matthew effects in reading: Some consequences of individual differences in the acquisition of literacy. Reading Research Quarterly, 21(4), 360–407. https://doi.org/10.1177/0022057409189001-204
- Steedly, K., Dragoo, K., Arafeh, S., & Luke, S. D. (2008). Effective mathematics instruction. Evidence for Education, 3(1), 2–11. National Dissemination Center for Children with Disabilities. https://files.eric. ed.gov/fulltext/ED572704.pdf
- Sumantri, M. S., & Satriani, R. (2016). The effect of formative testing and self-directed learning on mathematics learning outcomes. International Journal of Elementary Education, 8(3), 507–524. https://www.iejee.com/index.php/IEJEE/article/view/128
- Treffers, A. (1987). Three dimensions: A model of goal and theory description in mathematics instruction—the Wiskobas Project. Springer.
- Underhill, R. (1977). Teaching elementary school mathematics (2nd ed.). Charles E. Merrill.
- Underhill, R. G., Uprichard, A. E., & Heddens, J. W. (1980). Diagnosing mathematical difficulties. Charles E. Merrill.
- Wang, H., & Woodworth, K. (2011). Evaluation of Rocketship Education’s use of DreamBox Learning’s online mathematics program. SRI International. http://www.dreambox.com/wp-content/uploads/downloads/pdf/DreamBox Results from SRI Rocketship Evaluation.pdf
- Watts, T. W., Duncan, G. J., Siegler, R. S., & Davis-Kean, P. E. (2014). What’s past is prologue: Relations between early mathematics knowledge and high school achievement. Educational Researcher, 43(7), 352–360. https://doi.org/10.3102/0013189X14553660
- Williams, J. (2015, March 13). Inside Eureka Math: Does a popular Common Core math curriculum move too fast for young students? The Hechinger Report. https://tinyurl.com/ms5a77z6
- Woodward, J., Beckmann, S., Driscoll, M., Franke, M., Herzig, P., Jitendra, A., Koedinger, K. R., & Ogbuehi, P. (2012). Improving mathematical problem solving in grades 4 through 8: A practice guide (NCEE 2012-4055). National Center for Education Evaluation and Regional Assistance, Institute of Education Sciences, Department of Education. http://ies.ed.gov/ncee/wwc/publications_reviews.aspx#pubsearch/
- Worthke, W. (2000). Longitudinal and multi-group modeling with missing data. In T. D. Little, K. U. Shnabel, & J. Baumert (Eds.), Modeling longitudinal and multilevel data: Practical issues, applied approaches, and specific examples. (pp. 219–240). Erlbaum.
- Yang, D.-C., & Li, M.-N. (2013). Assessment of animated self-directed learning activities modules for children’s number sense development. Educational Technology and Society, 16(3), 44–58. https://www.jstor.org/stable/jeductechsoci.16.3.44