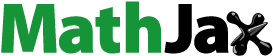
ABSTRACT
Mixing carpet fibre in sand offers great potential for enhancing soil properties. In this study, direct shear tests were conducted on two different sands mixed with varying carpet fibre percentages to investigate the effects on soil strength, stiffness, and deformation. Artificial intelligence techniques were used to analyse the data and develop predictive models, including an empirical equation that predicts the shear strength. The results showed that the addition of carpet fibre improved soil properties, with increased strength, stiffness, and reduced deformation. The AI models, including the empirical equation, accurately predicted the mixture's shear strength. Furthermore, this study investigated the importance of each input parameter in predicting the mixture's shear strength. The input parameters are normal stress, void ratio, mean particle size, and the ratio of carpet fibre content to specific gravity. According to the results, normal stress is the most important parameter, and mean particle size is the least important.
1. Introduction
Fibres have become increasingly significant in geotechnical engineering as a result of their ability to improve the mechanical properties of soil and other geotechnical materials (Almeida et al. Citation2023). Fibres are substances that are longer than they are wide, and they can be classified into two categories: natural and synthetic. There are many natural fibres found in nature, such as coconut fibre, palm fibre, jute, flax, bamboo, cane, sisal, and barely straw (Hejazi et al. Citation2012). In contrast, synthetic fibres are man-made fibres, such as polypropylene, polyester, polyethylene, glass, and nylon (Hejazi et al. Citation2012). The use of fibres in geotechnical engineering is often used to strengthen soil. list the mechanical and physical properties of natural and synthetic fibres.
Table 1. Mechanical and physical properties of the natural fibres (Sriram and Sidhaarth Citation2022.).
Table 2. Mechanical and physical properties of the synthetic fibres (Sriram and Sidhaarth Citation2022.).
Carpet fibre has recently emerged as a potential reinforcement material for geotechnical engineering applications (Gowthaman, Nakashima, and Kawasaki Citation2018). The use of carpet fibres as a reinforcement material can provide a low-cost, environmentally friendly solution to improve the mechanical properties of soil and other geotechnical materials (Rahman, Siddiqua, and Cherian Citation2022). Carpet fibre falls under the category of synthetic or man-made fibres (Oksman Citation2000). Carpet fibres are non-degradable and are made to endure overuse and are resistant to mechanical wear which also makes them difficult to recycle. Carpet production requires large amounts of chemicals, the majority of which are derived from non-renewable petroleum. These chemicals may also be released during carpet disposal emitting harmful volatile organic components (VOCs) into the air, making them significant pollutants to the environment (Realff et al. Citation2005).
In 2004, the worldwide fibre production has reached 64 million tonnes per year (Wang Citation2006). On the contrary, a vast amount of waste carpet fibre is discarded into landfills annually (Wang Citation2007). The worldwide waste carpet disposal rate is 4–6 million tons per year (Fashandi, Pakravan, and Latifi Citation2019) which makes recycling them crucial to contribute to a more sustainable world. Within the engineering field, sustainability is defined as projects that are environmentally friendly causing minimal damage to the environment over their life cycle and also consider factors including safety, durability, efficiency, ecology, environmental protection, carbon reduction, landscape, energy saving, and waste reduction (Chou et al. Citation2020; Liu Citation2020; Liu, Chen, and Chou Citation2019; Nguyen et al. Citation2023). One of the ancient ideas for reusing these waste carpet fibre materials was in soil reinforcements. Soil reinforcement has gained increased attention throughout the years, and it can be defined as a technique to improve the stability and enhance the engineering characteristics of soil to develop parameters including, shear strength, compressibility, density, and hydraulic conductivity (Kazemian et al. Citation2010). Fibre reinforced soil has appealing properties including its tensile strength, increased flexibility, greater weather resistance, drainage capacity, its low cost, and its increased peak strength and stiffness properties (Harrison Citation2006; Kumar and Roy Citation2022). All these properties make fibres advantageous in many engineering applications. The application of soil reinforcement can be implemented in geo projects including retaining walls, railway embankments, protection of slopes, earthquakes, and soil-foundation engineering (Hejazi et al. Citation2012). Numerous studies investigated the behaviour of soil reinforced with fibre and found that by combining fibre with soil, the shear strength of the mixture increased due to the tensile resistance of the carpet fibre that intersects with the shear failure and holds the mixture together (Hejazi et al. Citation2012).
Research by Ghiassian et al. (Citation2004), Sadek et al. (Citation2010), and Nguyen (Citation2022) has demonstrated the importance of inclusion fibres in improving different soil properties. Using carpet waste as a soil reinforcement material, Ghiassian et al. (Citation2004) found that carpet strips were able to significantly increase the strength and ductility of fine sand, particularly at higher aspect ratios (the ratio of fibre length to diameter), and therefore recycling carpet waste suggests a promising and environmentally friendly alternative to landfilling. Sadek et al. (Citation2010), in the same way, emphasized the impact of fibre length on the shear strength of fibre reinforced sand mixtures. Their research discovered that, even with the same aspect ratio, using longer fibres resulted in a significant improvement in the shear strength of the mixture. Nguyen (Citation2022) investigated the mechanical characteristics of clayey sand soils reinforced with Polypropylene (PP) fibres in the same way. They performed compaction, direct shear, and triaxial compression experiments with different fibre lengths (10 mm, 15 mm, and 20 mm) and fibre contents (0%, 0.5%, 1%, and 1.5%). Their findings indicated that fibre reinforcement significantly enhanced the mechanical characteristics of the soil. The study found that fibre lengths of 20 mm and 15 mm, with a fibre content of 1%, produced the best results in terms of maximum dry density and better cohesiveness. The friction angle, on the other hand, did not improve at the same rate. Nguyen (Citation2022) also proposed that fibre reinforcement efficiency could be further maximized by increasing the compaction energy. These studies demonstrate that fibre reinforcements, whether recycled carpet strips, longer fibres, or synthetic PP fibres, could significantly improve the mechanical and structural properties of soils.
The distribution of fibre for reinforcement with sand is a huge area of study that has attracted increasing attention in geotechnical applications. Many researchers dedicated their lab studies to investigate the shear strength of fibres in different distributions including random and discrete distribution. Gray and Ohashi (Citation1983) used the concept of root reinforcement of soil (sand) where the fibres were tested in both perpendicular and inclined orientations to the shear plane and concluded that the greatest shear strength was achieved with fibre orientation of 60 degrees with respect to the shear surface. Diambra et al. (Citation2010) undertook an experimental investigation using polypropylene fibres with Hostun RF sand. The mixture was tested in conventional triaxial compression and extension. It was noticed that the contribution of fibre to the strength was significant in compression but limited in extension confirming that it depends primarily on their orientation with respect to tensile strains.
Despite the importance of fibre carpets in soil, a comprehensive model for predicting their shear strength has not yet been developed. There are several reasons for this, including the multiplicity of effective factors and the non-linearity of the relationships between them. Over the last two decades, artificial intelligence (AI) methods have attracted the attention of engineering and geotechnical researchers. Various AI methods have been successfully applied to slope stability (Baghbani et al. Citation2022; Bardhan and Samui Citation2022), soil mechanics (Daghistani and Abuel-Naga Citation2023 Inazumi et al. Citation2020; Singh et al. Citation2021), soil dynamics (Lee, Lee, and Kim Citation2003; Baghbani et al. Citation2023a,; Gatto and Montrasio Citation2023), recycles materials (Baghbani et al. Citation2023; Daghistani et al. Citation2023; Saad et al. Citation2023; Baghbani et al., Citation2022) and soil cracking (Baghbani et al. Citation2022; Xu et al. Citation2022; Zhang et al. Citation2023). In spite of this, AI methods have not yet been used to predict the shear strength of soil and carpet fibre mixtures and thus form a gap in the history of studies that must be addressed. AI models were used in several studies to predict shear strength of soil. For example, Zhang et al., (Citation2021a) addressed the accurate assessment of undrained shear strength (USS) in soft sensitive clays. It applied novel data-driven methods, including extreme gradient boosting (XGBoost) and random forest (RF), to capture the relationships between USS and various soil parameters. The models were developed using a general approach with five key feature variables and are compared with other machine learning methods. The XGBoost and RF models outperformed the alternatives, providing higher predictive accuracy and feature importance rankings. These models offered promising tools for USS prediction and enhance the interpretability of geotechnical parameters. In another study, Zhang et al., (Citation2021b) explored the application of deep learning (DL) in geotechnical engineering. DL algorithms have powerful feature learning capabilities and are widely used in various fields. Zhang et al., (Citation2021a) discussed the major DL algorithms and their applications in geotechnical engineering. It provided a summary of published literature, reference cases, and adopted DL algorithms in this field. The challenges and future prospects of DL in geotechnical engineering were also highlighted.
After conducting and analysing a series of direct shear tests on the mixture of sand and carpet fibre, two statistical methods and three AI methods were used to predict the shear strength of the mixture. The statistical methods were multiple linear regression (MLR) and partial least squares (PLS), and the AI methods were classification and regression random forest (CRRF), genetic programming (GP), and Genetic Algorithm-Emotional Neural Network (GA-ENN). Among the strengths of this study is the use of both a black box AI method, i.e. CRRF, and a white box AI method, i.e. GP. Methods of white box AI produce equations or trees that readers can use for a database. Based on four inputs of carpet fibre content/specific gravity, normal stress, void ratio, and mean particle size, this study presents a new equation for predicting the shear strength of carpet fibre and sand mixture using the GP method. After reviewing the results of the mathematical models, the best models are presented and the importance of the parameters in different models is discussed.
2. Material and method
Two types of sand were used, namely L-sand and G-sand, with mean particle sizes (D50) 0.52 and 0.25 mm, respectively. These sands have coefficients of uniformity (Cu) of 2.04 and 1.62, respectively, and coefficients of curvature (Cc) of 0.96. It appears that both sands are poorly graded, and their sieve particle sizes represent in . The sieve analysis was conducted according to the Australian standard (AS1774.19 Citation2003). The specific gravity of the L-sand was 2.57, while that of the G-sand was 3.79. These specific gravity measurements were taken according to the Australian standard (AS1289.3.5.1 Citation2006). In addition, scanning electron microscopes (SEM) were used to look for the sand particle shape. The SEM results based on 50 particles revealed that sands had sphericity of 0.91 of L-sand and 0.88 of G-sand, where one means a complete sphere. The sphericity is measured using EquationEquation (1)(1)
(1) .
where S is the sphericity, and Re is circle with area equal to projected particle area.
The carpet fibres employed in this study were unused and shredded into lengths ranging from 15 mm to 25 mm, with an average length of 20 mm (). The tensile strength and density, as shown in the literature, are 800 MPa and 0.9 g/cm3, respectively (Sriram and Sidhaarth Citation2022). The carpet fibre content (CFC) in the mixture was measured with EquationEquation (2)(2)
(2) .
The mixture contents of carpet fibres in the sand were varied at levels of 0%, 0.25%, 0.5%, and 1% of sample dry weight. A direct shear apparatus was used in the experiments, with a mould of 60 × 60 mm. Fifty-two tests were conducted according to the Australian standard (AS1289.6.2.2 Citation2020), where each sample was subjected to four normal stresses of 25, 50, 100, and 200 kPa and a shear rate of 1 mm/min.
In term of mathematical models, five different statistical techniques (MLR, PLS, CRRF, GP, GA-ENN) were considered in machine learning. Here is a brief identification about the using techniques. The MLR (multiple linear regression) is a statistical model that can predict dependent variables based on multiple independent variables. PLS (partial least squares) is another statistical method for handling highly correlated data and reducing dimensionality, CRRF (classification and regression random forest) is an AI black-box method that combine decision trees and random forest to classify and predict variables, GP (genetic programming) is an AI grey-box technique that utilizes natural selection and genetic principles to evolve computer programs and model, and GA-ENN (Genetic Algorithm-Emotional Neural Network) is a new hybrid method that Genetic Algorithm is employed to optimize the architecture and ENN is a modified version of ANN as it includes neural parameters which enhance the network learning process.
3. Experimental results
3.1. Shear strength, dilation, and contraction
As displayed in , the shear strength of a sand-carpet fibre mixture can be influenced by the mixture density, CFC, and normal stress. Higher density mixtures tend to have higher shear strength due to increased interlocking of the sample particles which strengthens the mixture. The CFC can enhance shear strength by improving stability and rigidity, which can resist shear deformation. The normal stress also affects the shear strength of the mixture by increasing interlocking between sand particles and carpet fibres, making the mixture more stable and durable.
Figure 3. Shear strength versus dry density of the mixture at different density and normal stresses.

In a sand-carpet fibre mixture, dilation and contraction during shear can occur due to the changes in the size of the sample when it is subjected to shear forces. As in the dense states, the sand-carpet fibre mixture has a low void ratio, leading the sand particles to move apart and causing the sample to dilate. Conversely, in a loose state, the sample may contract due to the high void ratio within it. In this scenario, the carpet fibres may tend to align themselves in the direction of the applied shear force. According to , when the mixture is in its loose state, it exhibits contraction during shear while subjected to normal stresses of 200, 100, and 50 kPa. This contraction phenomenon becomes more pronounced with an increase in the CFC, which indicates that the carpet fibres increase the void in the sample. In terms of shear stress response, the peak and residual shear strengths are very similar in the loose state, and the shear strength of the mixture increases as CFC increases.
Figure 4. Shear stress and vertical deformation versus horizontal displacement for loose mixture at different normal stresses, a) 200 kPa, b) 100 kPa, and c) 50 kPa.

In the dense state, under the normal stresses 200, 100, and 50 kPa and as showing in . The mixture slightly contracts in the beginning where the sand particle adjusted in the existed void and then dilated during the shear, and it is showing that as the CFC increases, the mixture had more void and takes longer time to dilate comparing with less CFC mixture. In terms of shear strength characteristics, it is evident that the dense state mixture exhibits higher shear strength compared to the loose state. Additionally, as the CFC increases, the mixture tends to show a transition towards behaving more like a loose state, where there is not a significant change in the shear strength. Thus, as the carpet fibre content increases in the mixture, the voids increase, resulting in a looser mixture that experiences more contraction.
Figure 5. Shear stress and vertical deformation versus horizontal displacement for dense mixture at different normal stresses, a) 200 kPa, b) 100 kPa, and c) 50 kPa.

Furthermore, the dilation and contraction of a sand-carpet fibre mixture during shear are influenced by CFC, mixture density, and normal stresses. According to , as the CFC increases in the mixture, it takes longer to reach the maximum shear strength. Also, a loose state takes longer to reach its maximum shear strength than a dense one. Meanwhile, normal stress has a slight influence compared to other factors. The optimal values for these factors will depend on the intended use of the material and the shear loads that it is expected to experience.
3.2. Mixture density and compressibility
The sand-carpet fibre mixture was prepared with two densities: a loose state and a dense state, where the carpet fibre content was 0%, 0.25%, 0.5%, and 1% of the sample dry weight. The relative density values (Dr) for the loose state were 9.09%, 7.41%, 5.56%, and 3.23%, respectively. In contrast, for the dense state, the Dr values were 90.91%, 92.59%, 94.44%, and 96.77%, respectively. The inclusion of carpet fibres in the sand mixture has a noticeable effect on the dry density and void ratio, as observed in . The inclusion of carpet fibres decreases the mixture’s density due to their lower specific gravity compared to sand, and they increase the void ratio in the sample by filling the space between sand particles.
The coefficient of volume compressibility (mv) is an important parameter for evaluating the settlement and consolidation behaviour of the mixture under different loading conditions. The mv decreases with an increase in dry density and a decrease in CFC, as shown in . This means that the mixture becomes more resistant to compression as it becomes denser and has less carpet fibre.
4. Data-driven modelling results
Statistical data from direct shear tests (), including 52 observations of five variables: shear strength, normal stress, void ratio, mean particle size (D50), and CFC/Gs. Mean and standard deviation values are provided for each variable, which are useful for developing an AI model to predict mixture shear strength based on normal stress, void ratio, mean particle size, and CFC/Gs.
Table 3. The statistical information of experimental database.
The experimental database was divided into training (80%) and testing (20%) sets to develop an AI model. provide statistical information about these sets, and their similarity is important to improve model accuracy. The similar values of means and standard deviations indicate that the training set is representative of the testing set, which suggests that the AI model will perform well on new data. MATLAB (R2021a: The MathWorks Inc., Natick, MA, U.S.A.) was used for all mathematical modelling in this study.
Table 4. Statistical information about the training database.
Table 5. Statistical information about the testing database.
4.1. Multiple linear regression (MLR)
After performing a series of MLR modelling, the best model was identified. In , the values predicted by the MLR model are compared to the actual values of shear strength in direct shear tests. According to the results, the MLR model has a moderate level of accuracy.
The accuracy of MLR model for predicting shear strength was evaluated using a training database of 41 observations and a testing database of 11 observations. The model’s accuracy was measured () using several statistical metrics, including MAE, RMSE, RMSLE, and R2. The MAE for the testing database was slightly higher at 21.724 compared to 17.369 for the training database. Similarly, the RMSE for the testing database was higher at 22.833 than the RMSE for the training database at 19.785. The R2 value for the training database was 0.787, indicating that the model explains 78.7% of the data’s variability. However, the R2 value for the testing database was slightly lower at 0.653, suggesting that the model may not perform as well on unseen data as on training data. Overall, the MLR model demonstrated moderate accuracy in predicting shear strength, but its performance on new data may vary.
Table 6. The performance of the MLR model to predict shear strength.
4.2. Partial least squares (PLS)
To find the best PLS model, several simulations were carried out under different conditions. illustrates the results of the best PLS model in terms of values predicted by the PLS model versus actual values obtained from the direct shear test. Based on the results, PLS model has been found to be more accurate at predicting the shear strength of sand and carpet fibre mixtures.
The performance of a PLS model for predicting the shear strength of sand and carpet fibre mixtures is presented in , using 41 observations in the training dataset and 11 observations in the testing dataset. The model’s accuracy is evaluated using statistical metrics such as MAE, RMSE, RMSLE, and R2. The PLS model exhibits a mean absolute error of 10.662 and 15.952 for the training and testing databases, respectively. Additionally, the RMSE values for the training and testing databases are 14.941 and 16.966, respectively. The model’s RMSLE values for the training and testing databases are 0.228 and 0.438, respectively, showing smaller errors on a logarithmic scale than the RMSE values. The R2 values for the training and testing datasets are 0.878 and 0.808, respectively, indicating that the model explains around 80% to 88% of the variance in shear strength values. Overall, the PLS model performs well in estimating shear strength, but its accuracy may vary. For higher accuracy, better models like AI models may be needed.
Table 7. The performance of the PLS model to predict shear strength.
4.3. Classification and regression random forest (CRRF)
The specifications of the best CRRF model based on a thorough evaluation process are presented in . The CRRF model is a machine learning algorithm that combines the strengths of regression and random forest models, and specific parameters are optimized through a search process. The CRRF trees have a minimum node size of 2 and a minimum son size of 1, which determine the minimum number of observations required to split a node and create a new child node, respectively. The maximum depth of the tree is set at 7, indicating the maximum number of splits. The CRRF forest parameter has a Mtry value of 4 and a CP value of 0.00001, indicating the number of predictor variables randomly selected at each split and the minimum decrease in the mean squared error required to split a node, respectively. Each tree in the forest is constructed by random sampling with replacement, with a sample size of 41. The final model consists of 200 trees.
Table 8. The specifications of the best CRRF.
Based on the best CRRF model, shows the shear strength values predicted by the CRRF model versus the actual shear strength values obtained by the direct shear test. According to the results, the CRRF model predicted shear strength values more accurately than the two statistical models.
The performance of the CRRF model is presented in . The CRRF model was trained and tested on datasets with 41 and 11 observations, respectively. The MAE for the testing dataset was 4.857, indicating a difference of approximately 4.8 units between the predicted and actual values. The model’s RMSE for the testing dataset was 6.695, which is lower than the RMSE of the training dataset at 9.324, suggesting better performance on unseen data. The RMSLE values for the testing and training datasets were 0.118 and 0.098, respectively, indicating high accuracy in predicting shear strength. The R2 values for the testing and training datasets were 0.970 and 0.953, respectively, demonstrating a good fit between the model and the data. Overall, the CRRF model accurately predicts shear strength, but slightly higher error metrics for the training dataset suggest overfitting.
Table 9. The performance of the CRRF model to predict shear strength.
4.4. Genetic programming
After having successful results of using a black box AI method (CRRF), a white box AI method (GP), was used. presents a comparison of predicted and actual shear strength values for the best GP model, which was determined after conducting numerous GP modelling analyses. According to the obtained results, the GP model is more accurate than the CRRF model.
The performance of the GP model in predicting the shear strength of sand and carpet fibre mixtures is presented in , including both training and testing databases, evaluated using MAE, RMSE, RMSLE, and R2. The training database comprised 41 observations, while the testing database had 11 observations. The GP model’s MAE was 5.294 for the training dataset and 3.495 for the testing dataset, indicating higher accuracy in predicting shear strength in the testing dataset. Similarly, the RMSE of the GP model was 7.270 in the training dataset and 4.335 in the testing dataset, indicating higher accuracy in the testing dataset. The RMSLE for the training dataset was 0.081, while for the testing dataset, it was 0.110. The R2 values for the training and testing datasets were 0.971 and 0.987, respectively, indicating high predictive ability of the GP model for the shear strength of the sand and carpet fibre mixture. Overall, the GP model demonstrates good predictive ability for the shear strength of the sand and carpet fibre mixture.
Table 10. The performance of the GP model to predict shear strength.
Through normalization of the parameter values, the optimal equation (Eq. 3) for the genetic model is obtained.
where r1, r2, r3, and r4 are constants and CFC is carpet fibre content, is void ratio, Gs is specific gravity,
is mean particle size, and
is normal stress. shows the values of constants in EquationEquation 3
(3)
(3) .
Table 11. Constant values in EquationEquation (3)(3)
(3) .
4.5. GA-ENN (genetic algorithm-emotional neural network)
Lotfi and Akbarzadeh (2014) have recently developed a limbic-based Emotional Neural Network (ENN), which adopts a single-layer structure inspired by the emotional processes of the brain. Unlike Artificial Neural Networks (ANNs) that mimic biological neurons, ENNs are designed to simulate the interactions between four specific neural areas of the emotional brain: the thalamus, sensory cortex, orbitofrontal cortex (OFC), and amygdala.
In this study, a hybrid method (GA-ENN) was used to predict shear strength of sand-carpet fibre mixtures. Following several analyses, the optimal GA-ENN model has been identified and introduced. provides an overview of the specifications of the best proposed model.
Table 12. Characteristics of developed GA-ENN model.
A comparison between the predicted and actual shear strength values is illustrated in for the best GA-ENN model. This model was determined after conducting extensive GA-ENN modelling analyses. The results obtained demonstrate that the GA-ENN model outperforms all previous models in terms of accuracy.
provides an assessment of the GA-ENN model’s performance in predicting the shear strength of sand and carpet fibre mixtures, considering both the training and testing databases. Evaluation metrics such as MAE, RMSE, RMSLE, and R2 were utilized. The training database consisted of 41 observations, while the testing database contained 11 observations. For the training dataset, the GA-ENN model achieved an MAE of 2.639 and for the testing dataset, the MAE was 3.118. These results indicate a higher level of accuracy in predicting shear strength for the training dataset. Similarly, the RMSE values were 3.271 for the training dataset and 3.342 for the testing dataset, again showcasing higher accuracy in the training dataset. The RMSLE value for the training dataset was 0.063, while for the testing dataset, it was 0.101. This indicates the level of accuracy in predicting the logarithmic differences between the predicted and actual shear strength values, with lower values suggesting higher accuracy. Furthermore, the R2 values for the training and testing datasets were 0.994 and 0.993, respectively. These high R2 values indicate the strong predictive ability of the GA-ENN model for estimating the shear strength of the sand and carpet fibre mixture.
Table 13. The performance of the GA-ENN model to predict shear strength.
5. Discussion
The shear strength of a sand-carpet fibre mixture can be impacted by the mixture’s density, and confining pressure, as well as the carpet’s fibre content, aspect ratio, and orientation. For a mixture to perform effectively in a variety of situations, it is essential to understand how these factors influence its performance.
5.1. Carpet’s fibre content, aspect ratio, and orientation
The type and amount of carpet fibre present in the mixtures have a significant influence on its shear strength. The shear strength of the mixture increases as the CFC increases. Fibres, as reinforcement, give the mixture load-bearing capacity as well as resistance to shear stress. However, there is a certain limit to the amount of carpet fibre that can be added to the mixture beyond which no further increase in shear strength will be observed. By neglecting the influence of dry density, and as shown in , the gap between loose and dense varies less with increasing carpet fibre content. Furthermore, the figure indicates that CFCs have a greater impact on loose mixtures than on dense mixtures.
Shear strength is also affected by the aspect ratio of the fibres (the ratio of the length to the diameter) as well as their orientation. As the aspect ratio of the fibre increases, the surface area of the fibre also increases, and thus, aspect ratio must be considered along with fibre quantity, orientation, and sand particle morphology. Orientation vertically is associated with a higher shear strength than orientation horizontally. It is, however, difficult to manage the carpet fibre orientation in real-life situations. illustrates a schematic diagram of sand reinforced with carpet fibres at different particle shapes, carpet fibre orientations, and aspect ratios.
5.2. Active lateral pressure
Lateral earth pressure in a sand-carpet fibre mixture can be affected by carpet fibre content, mixture density, and confining pressure (). A carpet’s fibre content can have an impact on the lateral earth pressure in several ways. By increasing the carpet fibre content, the interlocking mechanism between the sand and fibre particles can be improved, resulting in an increase in shear strength. Consequently, the active lateral earth pressure increases. However, the inclusion of carpet fibre to the mixture reduces the density and increases the void ratio between the sand particle which affects and reduces the lateral pressure. As carpet fibre has a low specific gravity in comparison to sand, the density of the mixture decreases as we add more carpet fibre, and therefore the active lateral pressure decreases. The density of the mixture has an impact on the lateral earth pressure. By increasing the total weight of the soil mass, a higher mixture density can increase the lateral earth pressure. In contrast, a lower mixture density may result in a lower lateral earth pressure. Normal stress (confined pressure) can also affect lateral earth pressure. If the confining pressure increases, the lateral earth pressure will increase, whereas if the confining pressure decreases, the lateral earth pressure will decrease. In most cases, confined pressures are caused by the weight of soil and the normal load applied, which increases the shear strength and active lateral pressure.
5.3. Comparison of mathematical models
The performance of five distinct mathematical models in predicting the shear strength of sand and carpet fibre mixtures, namely MLR, PLS, CRRF, GP, and GA-ENN, is compared in . The evaluation was based on various statistical measures computed on both training and testing datasets. The findings showed that GA-ENN outperformed the other models, exhibiting the lowest MAE on the testing database. GP and CRRF closely followed GA-ENN in performance. All AI models including GA-ENN, CRRF, and GP had the lowest MAE values on the training database, while MLR and PLS recorded considerably higher values. Moreover, GA-ENN demonstrated the lowest RMSE values on both the training and testing databases, followed by GP and CRRF. On the other hand, MLR and PLS models recorded relatively higher RMSE values. The logarithmic predictions’ accuracy was assessed using RMSLE, where GA-ENN, CRRF and GP models showed the lowest values on both training and testing databases. All models exhibited relatively high R2 values on both datasets, with GA-ENN, CRRF, and GP showing the highest R2 values. The findings revealed that the GA-ENN model performed the best on both the training and testing databases. Also, MLR and PLS models may not be the most appropriate models for predicting shear strength of this mixture.
Table 14. The results of five mathematical models for training and testing databases.
5.4. Importance of parameters
The significance of input parameters in mathematical models is a crucial aspect of research in this domain. To assess their relative importance, a sensitivity analysis was conducted by varying one parameter by ± 100%, while keeping the other parameters constant, and measuring the introduced error. This procedure was applied to all the models developed in this study, and the outcomes are presented in , where larger errors indicate greater sensitivity of the model to that particular parameter, and hence higher importance of the parameter. Mean increase error parameter quantifies the average increase in error resulting from sensitivity analysis for each individual parameter.
For all models, shows the results of ranking the importance of parameters. The results indicate that, generally, normal stress parameter is the most significant, while mean particle size (D50) parameter is the least significant. According to the results, follow normal stress, CFC/Gs, and void ratio, respectively, are the most important parameters in four models MLR, PLS, CRRF, and GA-ENN.
Table 15. The results of variable importance for all AI models.
5.5. Limitations
While the study provides valuable insights into the effects of adding carpet fibre on soil properties and develops predictive models, it is important to acknowledge some limitations. Firstly, the study focused on the effects of carpet fibre on soil properties in direct shear tests, which may not fully capture all potential geotechnical applications or scenarios. Further research is needed to explore a broader range of soil tests and field conditions. Secondly, the study examined the effects of carpet fibre on two specific types of sand, and it is uncertain how applicable the findings are to other soil types. The generalizability of the results to different soil compositions and conditions needs to be further investigated. Thirdly, the study mainly focused on the immediate effects of carpet fibre addition on soil properties. The long-term performance and durability of the modified soil need to be assessed to determine its sustainability and effectiveness over time. Fourthly, while the AI models developed in the study exhibit high predictive accuracy for shear strength, they are based on simplified assumptions and may not fully capture the complexity and variability of real-world geotechnical systems. Further refinement and validation of the models are necessary. Next, although the study examines the importance of input parameters in predicting soil behaviour, it only considers a specific set of parameters. Other factors, such as environmental conditions or additional soil properties, may also influence the effectiveness of carpet fibre and should be considered in future research. Finally, the current study acknowledged a limitation regarding the limited number of datasets utilized. This limitation restricts the scope and applicability of the findings. To enhance the robustness and generalizability of the study, it is recommended to utilize a larger and more comprehensive database for future research. By expanding the dataset, a more comprehensive understanding of the subject matter can be obtained, leading to more reliable and widely applicable conclusions.
6. Conclusion
The inclusion of carpet fibre in sand is a crucial step in improving the strength and stability of the mixture. The experiments in this study were carried out under various conditions, with varying percentages of carpet fibre (0%, 0.25%, 0.5%, and 1%) mixed with two different types of sand, to assess the effectiveness of using carpet fibre in sand. For the first time, two black-box and one grey-box AI models, namely genetic algorithm-emotional neural network (GA-ENN), classification and regression random forest (CRRF), and genetic programming (GP), and two statistical models, namely multiple linear regression (MLR) and partial least squares (PLS), were used to analyse the data obtained from the experiments and to develop predictive models. The following are the main results in this study:
The shear strength of sand-carpet fibre mixtures is influenced by the mixture’s density and confining pressure, as well as the carpet’s fibre content, aspect ratio, and orientation. It is important to understand these factors to predict the performance of the mixtures in various applications and to optimize the mixture design for specific requirements.
The inclusion of carpet fibre to sand decreases the mixture density, and as known, low density is equal to low shear strength. In contrast, the fibre reinforcement increases the shear strength by increasing the interlocking between the sand particles. Overall, the experiment revealed that loose reinforced mixture at specific reinforcement percentage, and in comparison, with dense pure sand, could produce higher shear strength. This depends on the carpet fibre content and instalment orientation.
Equation was created using GP model to predict the shear strength of the mixture using the following inputs: normal stress, void ratio, mean particle size, and CFC/Gs. The accuracy of the model was above 97%.
GA-ENN, CRRF, and GP were the most accurate models in predicting the mixture shear strength, with higher R2 values and lower RMSE and RMSLE. In contrast, MLR and PLS models are less suitable.
Normal stress is identified as the most significant parameter in all models. Conversely, mean particle size was identified as the least important parameter in all models.
Disclosure statement
No potential conflict of interest was reported by the author(s).
References
- Almeida, J. L. D., Y. M. Ordoñez, P. C. A. Pudell, D. L. Brandão, M. A. Orioli, and R. L. D. S. Izzo. 2023. “Improvements in the Mechanical Behavior of a Silty Soil Treated with Rice Husk Silica, Lime, and Polypropylene Fiber.” International Journal of Geotechnical Engineering 17 (3): 1–15. https://doi.org/10.1080/19386362.2023.2227503.
- AS1289.3.5.1. 2006. “Australian Standard”. In: Methods of Testing Soil for Engineering Purposes. Sydney, NSW, Australia: Standards Australia.
- AS1289.6.2.2. 2020. “Australian Standard”. In: Soil Strength and Consolidation Tests - Determination of Shear Strength of a Soil - Direct Shear Test Using a Shear Box. Sydney, NSW, Australia: Standards Australia.
- AS1774.19. 2003. “Australian Standard”. In: The Determination of Sieve Analysis and Moisture Content. Sydney, NSW, Australia: Standards Australia.
- Baghbani, A., H. Abuel-Naga, R. Shirani Faradonbeh, S. Costa, and R. Almasoudi. 2023a. “Ultrasonic Characterization of Compacted Salty Kaolin–Sand Mixtures Under Nearly Zero Vertical Stress Using Experimental Study and Machine Learning.” Geotechnical and Geological Engineering 41 (5): 1–26. https://doi.org/10.1007/s10706-023-02441-5.
- Baghbani, A., S. Costa, T. Choundhury, and R. S. Faradonbeh. 2022. “Prediction of Parallel Desiccation Cracks of Clays Using a Classification and Regression Tree (CART) Technique”. Paper presented at the Proceedings of the 8th International Symposium on Geotechnical Safety and Risk (ISGSR), Newcastle, Australia.
- Baghbani, A., F. Daghistani, H. A. Naga, and S. Costa. 2022. “Development of a Support Vector Machine (SVM) and a Classification and Regression Tree (CART) to Predict the Shear Strength of Sand Rubber Mixtures”. Paper presented at the Proceedings of the 8th International Symposium on Geotechnical Safety and Risk (ISGSR), Newcastle, Australia.
- Baghbani, A., M. D. Nguyen, A. Alnedawi, N. Milne, T. Baumgartl, and H. Abuel-Naga. 2023. “Improving Soil Stability with Alum Sludge: An AI-Enabled Approach for Accurate Prediction of California Bearing Ratio.” Applied Sciences 13 (8): 4934. https://doi.org/10.3390/app13084934.
- Bardhan, A., and P. Samui. 2022. “Application of Artificial Intelligence Techniques in Slope Stability Analysis: A Short Review and Future Prospects.” International Journal of Geotechnical Earthquake Engineering (IJGEE) 13 (1): 1–22. https://doi.org/10.4018/IJGEE.298988.
- Chou, N. N., K.-H. Yang, B. Barrett, H.-M. Wu, and T.-Y. Liu. 2020. “Sustainable Characteristics of Reinforced Soil Structures–From Ancient Great Walls to Modern GRS Walls.” Transportation Infrastructure Geotechnology 7 (3): 445–460. https://doi.org/10.1007/s40515-020-00121-y.
- Daghistani, F, and Abuel-Naga, H. (2023). Evaluating the Influence of Sand Particle Morphology on Shear Strength: A Comparison of Experimental and Machine Learning Approaches. Applied Sciences, 13(14), 8160 10.3390/app13148160
- Daghistani, F., A. Baghbani, H. Abuel Naga, and R. S. Faradonbeh. 2023. “Internal Friction Angle of Cohesionless Binary Mixture Sand–Granular Rubber Using Experimental Study and Machine Learning.” Geosciences 13 (7): 197. https://doi.org/10.3390/geosciences13070197.
- Diambra, A., E. Ibraim, D. M. Wood, and A. Russell. 2010. “Fibre Reinforced Sands: Experiments and Modelling.” Geotextiles and Geomembranes 28 (3): 238–250. https://doi.org/10.1016/j.geotexmem.2009.09.010.
- Fashandi, H., H. R. Pakravan, and M. Latifi. 2019. “Application of Modified Carpet Waste Cuttings for Production of Eco-Efficient Lightweight Concrete.” Construction and Building Materials 198:629–637. https://doi.org/10.1016/j.conbuildmat.2018.11.163.
- Gatto, M. P. A., and L. Montrasio. 2023. “Artificial Neural Network Model to Predict the Dynamic Properties of Sand-Polyurethane Composite Materials for GSI Applications.” Soil Dynamics and Earthquake Engineering 172:108032. https://doi.org/10.1016/j.soildyn.2023.108032.
- Ghiassian, H., G. Poorebrahim, and D. H. Gray. 2004. “Soil Reinforcement with Recycled Carpet Wastes.” Waste Management & Research 22 (2): 108–114. https://doi.org/10.1177/0734242X04043938.
- Gowthaman, S., K. Nakashima, and S. Kawasaki. 2018. “A State-Of-The-Art Review on Soil Reinforcement Technology Using Natural Plant Fiber Materials: Past Findings, Present Trends and Future Directions.” Materials 11 (4): 553. https://doi.org/10.3390/ma11040553.
- Gray, D. H., and H. Ohashi. 1983. “Mechanics of Fiber Reinforcement in Sand.” Journal of Geotechnical Engineering 109 (3): 335–353. https://doi.org/10.1061/(ASCE)0733-9410(1983)109:3(335).
- Harrison, A. T. 2006. A Performance Framework for the Soil Strengthening Properties of Fibre-Reinforced Sand. Bristol, United Kingdom: University of Bristol.
- Hejazi, S. M., M. Sheikhzadeh, S. M. Abtahi, and A. Zadhoush. 2012. “A Simple Review of Soil Reinforcement by Using Natural and Synthetic Fibers.” Construction and Building Materials 30:100–116. https://doi.org/10.1016/j.conbuildmat.2011.11.045.
- Inazumi, S., S. Intui, A. Jotisankasa, S. Chaiprakaikeow, and K. Kojima. 2020. “Artificial Intelligence System for Supporting Soil Classification.” Results in Engineering 8:100188. https://doi.org/10.1016/j.rineng.2020.100188.
- Kazemian, S., B. B. Huat, P. Arun, and M. Barghchi. 2010. “A Review of Stabilization of Soft Soils by Injection of Chemical Grouting.” Australian Journal of Basic and Applied Sciences 4 (12): 5862–5868.
- Kumar, S., and L. B. Roy. 2022. “Rainfall Induced Geotextile Reinforced Model Slope Embankment Subjected to Surcharge Loading: A Review Study.” Archives of Computational Methods in Engineering 29 (5): 1–19. https://doi.org/10.1007/s11831-021-09688-2.
- Lee, S. J., S. R. Lee, and Y. S. Kim. 2003. “An Approach to Estimate Unsaturated Shear Strength Using Artificial Neural Network and Hyperbolic Formulation.” Computers and Geotechnics 30 (6): 489–503. https://doi.org/10.1016/S0266-352X(03)00058-2.
- Liu, T. 2020. “Establishment of sustainability key indicators for civil engineering and their applications in green infrastructure projects”. Doctoral Dissertation, Department of Civil Engineering, College of Engineering, National Taiwan University
- Liu, T.-Y., P.-H. Chen, and N. N. Chou. 2019. “Comparison of Assessment Systems for Green Building and Green Civil Infrastructure.” Sustainability 11 (7): 2117. https://doi.org/10.3390/su11072117.
- Lotfi, E., and M. R. Akbarzadeh-T. 2014. “Practical Emotional Neural Networks.” Neural Networks 59:61–72. https://doi.org/10.1016/j.neunet.2014.06.012.
- Nguyen, S. H. 2022. Experimental Study on Behaviour of Clayey Sand Reinforced by Polypropylene Fibre. Paper presented at the CIGOS 2021, Emerging Technologies and Applications for Green Infrastructure: Proceedings of the 6th International Conference on Geotechnics, Civil Engineering and Structures. Ha Long, Vietnam.
- Nguyen, M. D., A. Baghbani, A. Alnedawi, S. Ullah, B. Kafle, M. Thomas, and N. A. Milne. 2023. “Investigation on the Suitability of Aluminium-Based Water Treatment Sludge as a Sustainable Soil Replacement for Road Construction.” Transportation Engineering 12:100175. https://doi.org/10.1016/j.treng.2023.100175.
- Oksman, K. 2000. “Mechanical Properties of Natural Fibre Mat Reinforced Thermoplastic.” Applied Composite Materials 7 (5/6): 403–414. https://doi.org/10.1023/A:1026546426764.
- Rahman, S. S., S. Siddiqua, and C. Cherian. 2022. “Sustainable Applications of Textile Waste Fiber in the Construction and Geotechnical Industries: A Retrospect.” Cleaner Engineering and Technology 6:100420. https://doi.org/10.1016/j.clet.2022.100420.
- Realff, M., P. Lemieux, S. Lucero, J. Mulholland, and P. Smith. 2005. “Characterization of Transient Puff Emissions from the Burning of Carpet Waste Charges in a Rotary Kiln Combustor”. Paper presented at the Conference Record Cement Industry Technical Conference, 2005. Kansas City, MO, USA.
- Saad, A. H., H. Nahazanan, B. Yusuf, S. F. Toha, A. Alnuaim, A. El-Mouchi, and A. A. Mohammed. 2023. “A Systematic Review of Machine Learning Techniques and Applications in Soil Improvement Using Green Materials.” Sustainability 15 (12): 9738. https://doi.org/10.3390/su15129738.
- Sadek, S., S. S. Najjar, and F. Freiha. 2010. “Shear Strength of Fiber-Reinforced Sands.” Journal of Geotechnical and Geoenvironmental Engineering 136 (3): 490–499. https://doi.org/10.1061/(ASCE)GT.1943-5606.0000235.
- Singh, B., P. Sihag, S. M. Pandhiani, S. Debnath, and S. Gautam. 2021. “Estimation of Permeability of Soil Using Easy Measured Soil Parameters: Assessing the Artificial Intelligence-Based Models.” ISH Journal of Hydraulic Engineering 27 (sup1): 38–48. https://doi.org/10.1080/09715010.2019.1574615.
- Sriram, M., and K. A. Sidhaarth. 2022. “Various Properties of Natural and Artificial Fibers with Cementitious Composites in Hybrid Form–A Review.” Materials Today: Proceedings 60:2018–2025. https://doi.org/10.1016/j.matpr.2022.01.266.
- Wang, Y. 2006. Recycling in Textiles. Cambridge, United Kingdom: Woodhead publishing.
- Wang, Y. 2007. “Carpet Fiber Recycling Technologies.” Ecotextiles. 26–32. https://doi.org/10.1533/9781845693039.1.26
- Xu, J. J., H. Zhang, C. S. Tang, Q. Cheng, B. G. Tian, B. Liu, and B. Shi. 2022. “Automatic Soil Crack Recognition Under Uneven Illumination Condition with the Application of Artificial Intelligence.” Engineering Geology 296:106495. https://doi.org/10.1016/j.enggeo.2021.106495.
- Zhang, F., Z. Hu, Y. Liang, and Q. Li. 2023. “Evaluation of Surface Crack Development and Soil Damage Based on UAV Images of Coal Mining Areas.” Land 12 (4): 774. https://doi.org/10.3390/land12040774.
- Zhang, W., H. Li, Y. Li, H. Liu, Y. Chen, and X. Ding. 2021. “CovidSens: A Vision on Reliable Social Sensing for COVID-19.” Artificial Intelligence Review 54 (1): 1–41. https://doi.org/10.1007/s10462-020-09852-3.
- Zhang, W., C. Wu, H. Zhong, Y. Li, and L. Wang. 2021. “Prediction of Undrained Shear Strength Using Extreme Gradient Boosting and Random Forest Based on Bayesian Optimization.” Geoscience Frontiers 12 (1): 469–477. https://doi.org/10.1016/j.gsf.2020.03.007.