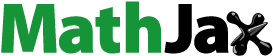
ABSTRACT
Model-informed drug discovery advocates the use of mathematical modeling and simulation for improved efficacy in drug discovery. In the case of monoclonal antibodies (mAbs) against cell membrane antigens, this requires quantitative insight into the target tissue concentration levels. Protein mass spectrometry data are often available but the values are expressed in relative, rather than in molar concentration units that are easier to incorporate into pharmacokinetic models. Here, we present an empirical correlation that converts the parts per million (ppm) concentrations in the PaxDb database to their molar equivalents that are more suitable for pharmacokinetic modeling. We evaluate the insight afforded to target tissue distribution by analyzing the likely tumor-targeting accuracy of mAbs recognizing either epidermal growth factor receptor or its homolog HER2. Surprisingly, the predicted tissue concentrations of both these targets exceed the Kd values of their respective therapeutic mAbs. Physiologically based pharmacokinetic (PBPK) modeling indicates that in these conditions only about 0.05% of the dosed mAb is likely to reach the solid tumor target cells. The rest of the dose is eliminated in healthy tissues via both nonspecific and target-mediated processes. The presented approach allows evaluation of the interplay between the target expression level in different tissues that determines the overall pharmacokinetic properties of the drug and the fraction that reaches the cells of interest. This methodology can help to evaluate the efficacy and safety properties of novel drugs, especially if the off-target cell degradation has cytotoxic outcomes, as in the case of antibody-drug conjugates.
Introduction
Monoclonal antibodies are used successfully in all major therapy areas due to their exquisite specificity and low intrinsic toxicity.Citation1 Despite the progress made, drug attrition, which is partly due to target-related uncertainties, remains an issue.Citation2 This is especially relevant in the case of antibody-drug conjugates (ADCs) where toxicity remains the dose-limiting factor.Citation3 We therefore decided to explore the organ-targeting specificity of mAbs within the context of physiologically based pharmacokinetics (PBPK), which takes a first-principles holistic approach to the body as a whole. An integral part of the process is the accurate incorporation of organ-specific target expression levels estimated from independent experimental data.
Approximately 65% of mAb targets are membrane proteins, such as CD20, HER2, EGFR, CD4, PD-1, and PD-L1.Citation4 Upon antibody binding, these mAb-target complexes undergo cellular internalization and degradation that manifests as target-mediated drug disposition (TMDD) where the pharmacokinetic (PK) behavior of the drug depends on its dose, concentration, and time.Citation5 Whilst TMDD can complicate the dose-response analysis and prediction, it also lends support to the three pillars of therapeutic drug discoveryCitation6 since the process characterizes the tissue penetration and target engagement properties of the drug at the site of action and elsewhere.
In this work we focus on cell membrane target expression level data mining from the mass-spectrometric PaxDb databaseCitation7,Citation8 and describe the quantitative correlation between the relative ‘parts per million’ (ppm) and absolute (molar) concentration values. We use the insight gained within the framework of biologics PBPKCitation9,Citation10 to evaluate the impact of target expression in healthy organs on the tumor tissue-targeting efficacy of mAbs. In the first instance, we focus on two well-studied cancer targets: epidermal growth factor receptor (EGFR) and its HER2 homolog, as they are often present not only in tumor cells, but also elsewhereCitation11 where they have a normal physiological role to play.Citation12
Methods
Protein expression data
The combined human liver proteome dataset in fmol/μg of total protein units was obtained from the proteomic study by Wegler et al.Citation13 Matching protein concentration values in ppm units for the brain, colon, heart, kidney, liver, lung, pancreas, skin, as well as the whole-body estimate, were obtained from the PaxDb database v5.0.Citation7,Citation8 Only the “integrated” data were used to establish the correlation between ppm and molarity, as these represent the consensus estimates. Proteins were classified as membrane, intracellular, membrane and/or secreted proteins according to the Human Protein Atlas database v23.Citation14 Data for the membrane proteins formed the training data set. Intracellular, membrane, and/or secreted proteins formed the validation data set.
Protein concentration unit conversion from fmol/μg of total protein to molarity
A schematic diagram of the unit conversion is shown in Supplementary Figure S1. The typical human liver volume of 2143 mL contains 183 mL of plasma and 429 mL of interstitial fluid,Citation15 leaving a cellular volume at 1531 mL. Total protein concentration is estimated to reach 200 g/L in liver cells,Citation16,Citation17 60 g/L in plasmaCitation18 and 40 g/L in the interstitial space.Citation19 Whilst lumping the red blood cell volume of approximately 120 mL with the hepatocytes and other liver cells, the total liver protein mass amounts to 334.3 g, of which 306.2 g is found in cellular space, 11.0 g in plasma, and 17.2 g in interstitial space. At these values, the average liver protein concentration amounts to 156 g/L, assuming a tissue density of 1 g/mL. Therefore, 1 fmol/μg (i.e., 1 nmol/g) liver protein corresponds to approximately 156 nM.
Correlation between liver protein molarity and ppm abundance
Just as in the case of soluble targets,Citation20 we used an empirical sigmoidal-shape model (EquationEquation 1(1)
(1) ) to describe the relationship between the concentrations expressed in liver protein molarity and ppm units:
Whereby Base and MaxDV represent the minimum and maximum predicted protein concentrations (in nM), ppm50 represents the ppm value where the predicted concentration is at 50% of MaxDV and hill is the power function. Additive residual error (on the log10 transformed protein concentrations) was used according to EquationEquation 2(2)
(2) :
Whereby PRED represents the predicted concentration (in log10 nM) and ε the estimated residual error, which is assumed to follow a normal distribution with mean zero and variance σ2. The 90% prediction interval of the predicted tissue concentration was calculated as PRED (in log 10 nM) ± 1.645*σ.
Anti-EGFR and -HER2 mAb pharmacokinetic data
Trastuzumab and cetuximab were chosen as prototype anti-HER2 and anti-EGFR mAbs, respectively. Plasma concentration versus time profiles after a single intravenous (IV) dose of 1–8 mg/kg trastuzumab,Citation21 or a single dose of 50–500 mg/m2 IV cetuximab,Citation22 were digitized using WebPlotDigitizer.Citation23 HER2 binding affinity of trastuzumab was used at Kd = 1.9 nM, with an association rate constant kon = 0.612 nM−1h−1 and a dissociation rate constant koff = 1.188 h−1.Citation24 The EGFR-binding affinity of cetuximab was used at Kd = 5 nM, with kon = 0.792 nM−1h−1 and koff = 3.96 h−1.Citation25
PBPK modelling
General model structure
The cross-species/cross-modality biologics PBPK model, containing all major organs and a solid tumor compartment, was built in Matlab 2022b SimBiology using scripted assembly.Citation10 Briefly, each organ contains vascular, interstitial, and endosomal compartments, with the kidney, brain and lungs further modified with organ-specific adaptations. Vascular and interstitial compartments contain soluble molecular species (free drug, free soluble extracellular domain of the target and a complex of the two) that are subject to plasma and lymphatic circulation within the framework of the two-pore hypothesis. All tissue compartments contain the membrane-bound target protein that does not circulate, but can engage the drug to form membrane-bound drug-target complexes that are subject to TMDD. Both free receptor and its complex with the mAb can be cleaved from the cell surface, upon which both molecular species become the respective soluble species. Receptor-target complex internalization is assumed to be irreversible. Non-specific mAb and soluble complex clearance through macropinocytosis and endosomal recycling takes place via neonatal Fc receptor (FcRn) recycling. The outline of the reactions modeled in each organ’s vascular and interstitial compartments is shown in , while the detailed organ-level SimBiology representation for automated model assembly is presented in Supplementary Figure S2.
Figure 1. Schematic representation of the reaction scheme in the vascular and interstitial spaces of all organs of the body. Target receptor (brown structure) exists in surface-bound and soluble forms. Receptor shedding (kshed) is denoted by the black arrows. Internalization of receptor is denoted by the red arrows (kint). We assume that kshed and kint are not affected by mAb binding.

Parameterization of target expression in the PBPK model
The plasma concentrations of soluble HER2 and EGFR were calculated from PaxDb data using our previously published approach.Citation17 The tissue concentrations of HER2 and EGFR for brain, heart, kidneys, liver, lung, pancreas, and skin were calculated from organ-specific PaxDb data. We assumed the intracellular target fraction to be negligible both for HER2 and EGFR,Citation26–28 but decided to analyze more closely two alternative scenarios for the location of the membrane-bound receptor. In the first instance, we allocated the membrane-bound target entirely to the interstitial space only. This corresponds to the scenario where expression takes place in parenchymal cells only. In the second scenario, we allocated the membrane target at identical levels to the organ vascular and interstitial spaces. This simulates the scenario where target expression takes place both in parenchymal and endothelial cells.
In the case where the membrane-bound target was allocated entirely to the interstitial space, the organ-specific target concentration mRInt,Organ was calculated according to EquationEquation 3(3)
(3) :
Whereby tRorgan denotes the target total molar tissue concentration, Vorgan stands for the organ volume, sRpl denotes the plasma concentration of the target soluble fraction, Vpl,organ stands for organ plasma volume and Vint,organ for organ interstitial volume.
In the case where the membrane-bound target was split equally between the organ interstitial and vascular spaces, the membrane target concentrations mRint+vas,organ were calculated according to EquationEquation 4(4)
(4) :
Whereby Vpl,organ stands for organ capillary plasma volume and the rest of the parameters are described in EquationEquation 3.(3)
(3)
The rest of the organs represented in the PBPK model, but for which there was no tissue-specific data in the database (skeletal muscle, adipose, bone, gastrointestinal tract, thymus, and spleen), were assumed to have the target present at the same concentration. This was calculated as the difference between the database-derived total body value and the sum of all measured organ values.
In the case where the target was confined to the interstitium, the average concentration mRint,RoB in the interstitial compartments of the organs not listed in the database was calculated from EquationEquation 5(5)
(5) :
Whereby tRav denotes the average body molar concentration of the receptor, Vbody denotes total body volume and Vpl denotes total plasma volume. tRorgan,i and Vorgan,i are the total organ concentrations and organ volume values for tissues, respectively, listed in PaxDb database (heart, kidney, liver, lung, pancreas, brain, and skin). Vint,j denotes the interstitial values of the rest of the organs included in the model but not listed in the PaxDb database (muscle, adipose, bone, GI tract, thymus, spleen, lymph nodes).
In the case where the membrane-bound target was split between the organ interstitial and vascular spaces, the effective concentrations mTargetint+vas,Organ in organ interstitial and vascular plasma compartments were calculated according to EquationEquation 6(6)
(6) :
Whereby denotes the vascular capillary volume of an organ not measured in the PaxDb database (muscle, adipose, bone, GI tract, thymus, spleen, lymph nodes).
Soluble target turnover in the PBPK model
Free and mAb-bound cell membrane targets (mTarget and mTarget_mAb in Supplementary Figure S2) in vascular and interstitium are subject to first-order degradation with rate constant kint_R and shedding with rate constant kshed. Organ and compartment-dependent target expression rates kexp_ORGAN_va in organ vascular and kexp_ORGAN_in in organ interstitial compartments were calculated according to EquationEquations 7(7)
(7) and Equation8
(8)
(8) , respectively:
Whereby mTarget_ORGAN_M stands for total concentration of the target in the respective tissue compartment of an ORGAN in the PBPK model. Fr_tot is an empirically adjustable factor that proportionally affects concentration values to a similar effect in all tissues (default value = 1). Fr_in_all assigns the target to interstitium only (if equal to 1) or both to vascular and interstitial spaces (if equal to zero). ORGAN_va and ORGAN_in denote the organ vascular and interstitial volumes, respectively, while kint_R and kshed denote the target receptor (and its complex with the drug) degradation and shedding rate constants, respectively. The model assumes that the target-mAb complex internalizes/degrades and is shed at the same rate as the free target, meaning the total membrane-bound target is constant in time.
Results
Correlation between liver protein concentrations expressed in molar and ppm units
The combined final human liver proteome dataset extracted from a global proteomic study by Wegler et al.Citation13 contained 317,572 absolute concentrations (from 54 donors), representing 6679 unique proteins. Of these 29,021 liver concentrations from 644 proteins that were classified as membrane proteins were used for model training, whilst the remaining concentrations were reserved for model validation purposes. Matching ppm values were obtained from the PaxDb database, which contains tissue-specific expression levels of approximately 19,000 proteins.Citation7
As shown in , a sigmoidal function accurately describes the correlation between the liver membrane protein concentrations expressed in molar and ppm units. The corresponding parameter values and standard errors are listed in .
Figure 2. Fitted sigmoidal function (solid red line) describes the correlation between liver membrane protein concentrations expressed in molar and ppm units. Circles represent the observed data. Solid red lines and the shaded area represent the predicted median liver concentrations and the corresponding 90% prediction confidence interval.

Table 1. The sigmoidal function parameter estimates that describe the correlation between the molar and ppm concentrations for liver membrane proteins.
Notably, data in the validation dataset also demonstrated good model predictive performance for the intracellular and secreted proteins, as shown in .
Calculation of EGFR and HER2 concentrations in human tissues
We used EquationEquation 1(1)
(1) with parameter values listed in and mass spectrometric ppm tissue concentration values from the PaxDb database to calculate EGFR and HER2 concentrations in the brain, heart, kidney, liver, lung, pancreas, skin, and the body as a whole. The tissue concentrations were thereafter used in two scenarios according to EquationEquations 3
(3)
(3) -6. In the first instance the target was assumed to be confined to the interstitium only. In the second scenario we assumed the target to be present at equal effective concentrations in organ vascular and interstitial spaces. The resulting values are listed in .
Table 2. The calculated tissue concentrations of EGFR and HER2 and their allocation to interstitial and vascular spaces for alternative PBPK modeling purposes. Numbers between parentheses represent the 90% prediction interval. tRorgan denotes the average tissue concentration of the target. mRint,organ and mRint+vas,organ denote target concentrations for the cases where the location for membrane-bound target is assigned to interstitial space only or was split equally between organ interstitial and vascular compartments, respectively.
Soluble target PBPK model optimization for the membrane target shedding rate constant
In the PBPK model, the soluble target is formed from the shedding of membrane-bound receptor species. The value of the rate constant kshed was manually adjusted for the model to accurately describe the pre-dosing steady state plasma concentrations of soluble EGFR and HER2 as listed in . The corresponding shedding rate constants are listed in . The steady-state plasma and interstitial concentration values of the soluble target were thereafter used to define the initial state of the PBPK model for mAb dosing simulations (Supplementary Figure S3).
Table 3. PBPK model parameter estimates for EGFR (A) and HER2 (B). Fr_tot denotes the tissue concentration dimensionless adjustment factor, which accommodates the deviation from the tissue concentration model prediction calculated from EquationEquation 1(1)
(1) , while kint denotes the internalization rate constant. kshed denotes the shedding rate constant for the membrane-bound target and RL is the relative likelihood of the given model vs one with the lowest AIC within a group.
Antibody PBPK model optimization for the membrane target internalization rate constant and abundance
Four fitting scenarios were applied both to EGFR and HER2. In the first instance, only the internalization rate constant kint was fitted whilst the target was confined to interstitium only. In the second case, the overall target concentration and kint were co-optimized. In the third scenario, kint was fitted whilst the target was confined to both tissue interstitial and vascular spaces. In the fourth scenario, the overall target concentration and kint were co-optimized. The results are shown in , while the corresponding parameter values are listed in . During data fitting we interpreted the plasma concentrations to measure the sum of free and soluble target complexed mAb species at the same time.
Figure 4. EGFR and HER2 PBPK model parameter fitting. a-b) cetuximab IV dosing at 50 (beige), 100 (purple), 250 (green), 400 (red) and 500 mg/m2 (blue).Citation22 solid line: internalization rate constant kint and target concentrations are adjusted, dashed line: only kint is adjusted. A: membrane EGFR is allocated to organ interstitium only, B: membrane EGFR is allocated to organ vascular and interstitial spaces at equal concentrations. c-d) trastuzumab IV dosing at 1 (purple), 2 (green), 4 (red) and 8 (blue) mg/kg.Citation21 solid line: internalization rate constant kint and target concentrations are adjusted, dashed line: only kint is adjusted. C: membrane HER2 is allocated to organ interstitium only, D: membrane HER2 is allocated to organ vascular and interstitial spaces at equal concentrations.

Visual inspection of the plots in and the Akaike Information Criterion (AIC)Citation33 values presented in indicate that the best fit is achieved when the membrane target is assigned to organ vascular and interstitial spaces whilst co-fitting the internalization rate constant kint and adjusting the overall target expression level. The best fitting scenarios imply 3-fold lower tissue concentrations for EGFR and 6-fold lower concentrations for HER2 compared to the concentrations predicted from the PaxDb proteomics data presented in the sigmoidal correlation model ().
On the basis of parameter estimation and relative likelihood, we adopted model variants (4) as the best fitting scenarios for EGFR and HER2. In these models, the target is assigned both to vascular and interstitial spaces with the inclusion of the corresponding adjustment to overall tissue concentration.
PBPK simulation of mAb interaction with soluble target
We simulated the plasma concentrations of soluble molecular species for the best fitting EGFR and HER2 model variants at the highest doses used in the respective studies. The results shown in display the TMDD-affected time course.Citation5 The nonspecific clearance dominates approximately for the first 30 days when the relative contribution of the TMDD pathway is negligible. During the next 20 days, the clearance accelerates temporarily as the TMDD contribution becomes relatively significant before fading away as the drug concentration falls below the respective Kd value of the target. The model predicts modest temporary accumulation of soluble EGFR and HER2 in complex with the mAb, before returning to the baseline level. We are not aware of this having been previously measured for plasma EGFR and HER2, but is otherwise expected and observed for other soluble antibody targets.Citation34,Citation35
Figure 5. Simulated time course values for a single IV dose of a mAb. Total drug (red) and total target (green) are denoted by solid lines. Dashed lines indicate free drug (red), free soluble target (green) and complex of the two (blue). a) 500 mg/m2 cetuximab interacting with soluble EGFR and b) 8 mg/kg trastuzumab interacting with soluble HER2.

PBPK simulation of mAb interaction with membrane targets
Most of EGFR and HER2 are found in tissues where the expression level and vascular permeability varies from organ to organ. The results in show the predicted concentration time profiles for the free mAb, membrane-bound-free EGFR or HER2 and the target complex with the drug in liver and solid tumor. These two tissues have identical vascular permeabilities in the model, but without lymphatics and convection-driven extravasation in the latter. The results for the rest of the organs are shown in Supplementary Figures S4 and S5. For both antibodies, in liver interstitium the model predicts a free mAb time course which follows closely to that observed in plasma, while a lag period of 3–4 days is expected in the solid tumor microenvironment (TME). The lag period observed in the solid tumor reflects the absence of convectional paracellular transport across the endothelial barrier due to a lack of lymphatic flow that in normal tissues dominates the extravasation flux by diffusion and transcytosis. In the case of both antibodies, full target engagement is expected to last for about 40 days from a single IV dose simulated.
Figure 6. Predicted time course values for interstitial free drug (red), free membrane-bound target (green) and the complex of the two (blue). a) membrane EGFR in liver interstitium after a 500 mg/m2 IV dose of cetuximab, b) membrane EGFR in tumor interstitium after a 500 mg/m2 IV dose of cetuximab, c) membrane HER2 in liver interstitium after an 8 mg/kg IV dose of trastuzumab, d) membrane HER2 in tumor interstitium after an 8 mg/kg IV dose of trastuzumab.

Model prediction for organ-dependent degradation of dosed mAbs
There are three parallel pathways in the model for the elimination of the dosed mAbs: renal filtration, macropinocytosis, and TMDD. Of these, renal filtration is negligible due to the hydrodynamic radius of mAbs, leaving macropinocytosis and TMDD to dominate the elimination process. The fraction of the dose eliminated in an organ depends on the mAb size, vascular permeability, and target concentration and turnover. The rate of organ-dependent elimination varies 3- to 4-fold between different tissues, with most of the dose being eliminated within 50 to 60 days, as can be seen in Supplementary Figure S6.
We characterized the organ-dependent mAb degradation process by dividing the fraction of the dose that degraded per tissue volume with the value for the tumor compartment. As shown in , with the exception of skin and lungs in the case of cetuximab, more mAb was degraded per organ volume in tumor than in any other tissue. However, the difference is only around 4-fold, despite a 25- to 50-fold higher target expression level on tumor cells. In the case of ADCs, this would mean only a modest accumulation of the payload toxin in tumor compared to healthy tissues and relatively narrow therapeutic window. In absolute terms, as seen in , an average solid tumor is likely to take up around 0.06% of the administered dose.
Discussion
In this study, we demonstrate that the ppm tissue protein concentration values stored in the mass spectrometric PaxDb database can be related to molarity. This complements our previous work where we established a similar correlation for plasma proteinsCitation20 and thus allows organ molar concentration estimates for cell-linked proteins listed in the PaxDb, which contains data for thousands of proteins across many species and organs, to be obtained.Citation7,Citation8
Although the formalism is the same as that applied to plasma and tissue proteins, the parameter values are different for the two data sets. In the case of plasma proteins, both molar and relative concentration values stretch to eight orders of magnitude, while in the case of tissue proteins, the variation is about six orders of magnitude for ppm values from the PaxDb database and about three orders of magnitude for the molar concentration values that we calculated from the results obtained by Wegler et al.Citation13 As a result and due to nonlinearity, the maximum and minimum values of the two correlation functions are expected to be different, with the power function Hill coefficient, whilst less than one in both cases, is 3-fold higher in tissue proteins. However, the function parameters are empirical and not necessarily identifiable with a process or a property. Importantly, the tissue protein model (trained on membrane proteins) accurately predicted the validation data set values for intracellular and other proteins (as shown in ).
For the development of the tissue protein model, we assumed that the ppm values from the PaxDb database represent liver protein expression in healthy humans. Protein concentration data from Wegler et al. on the other hand predominantly featured samples from donors with obesity (37 of 54 subjects). However, considering that the majority of the liver proteins were not differently expressed in donors with or without obesity,Citation13 no distinction was made between obese and healthy donors in the present analysis. Although there is a distinct possibility that some proteins may be up- or downregulated in the obese patient cohort, we expect those to approximately cancel out in the overall correlation between the thousands of individual measurements used. Nevertheless, one should always keep in mind that predicted baseline levels in healthy subjects may deviate from levels in the patient group of interest, due to differences in health status, genetics, or other factors. For example, overexpression of target levels in patients may cause the mAb PK to be significantly different than in healthy volunteers due to stronger TMDD. Consequently, we recommend use of the measured tissue concentrations in the patient group of interest, if that data is available. The PaxDb database would be a fallback choice for when no such information is available, a situation most likely to be encountered in the case of tissue-embedded targets or early stage predictive evaluation of target likely druggability.
We used liver data to parameterize the correlation between the ppm and molar unit protein concentration values, but we expect the same relationship to extend to other tissues. This rests on the assumption that the average eukaryotic cell protein concentrations are invariant between organs, while the average organ plasma (5%) or interstitial volume (20%) fractions vary little from organ to organ and across species from mouse to humans, as shown in Supplementary Figure S7.
The devised approach was then applied to obtain the plasma and tissue concentration estimates for EGFR and HER2. Histochemical and transcriptomic data indicate a wide tissue distribution of these two key cancer targets. Both targets have been detected on many epithelial, mesenchymal, and other cells, where they play important roles in proliferation, differentiation, and development.Citation11,Citation14,Citation36 By adopting the estimate of 37 trillion cells in the human body according to Bianconi et al.Citation37) the proteomic data for the average tissue expression level indicate the presence of, on average, around 40,300 EGFR and 28,400 HER2 molecules per cell, respectively. These estimates align well with the experimental values of 40,000 to 100,000 molecules of EGFRCitation38,Citation39 and 10,000 to 50,000 molecules of HER2,Citation40,Citation41 per cell. The receptor number on tumor cells is expected to be higher, reaching about one million for EGFRCitation38 and two million for HER2,Citation32 but their impact on the body average is negligible at moderate tumor volume values. Surprisingly, the resulting effective concentrations of both targets in membrane-bound form substantially exceed the respective Kd values for the two mAbs simulated in this study. In these conditions, the law of mass action implies that if the mAb concentration is at similar or lower level to that of the target, it predominantly exists in complex with the receptor. Conversely, if the mAb is in excess, the majority of the target is always engaged, as clearly presented in . From an antibody engineering perspective, this means there is probably very little that can be gained by further affinity maturation of the drug in terms of tumor-targeting specificity or efficacy.
Drug–target interaction involves reversible mAb binding to the receptor where dissociation, shedding, and internalization are the three pathways facing the membrane-bound complex. Of these three, only the receptor-mediated internalization route is linked to TMDDCitation42,Citation43 and hence provides good evidence of target engagement at the site of action according to Three Pillar framework.Citation6 Extensive research on EGFR and HER2 internalization and turnover dynamics in the absence and presence of different ligands, such as EGF in the case of EGFR or a mAb in the case of HER2, has revealed an intricate system whereby receptor-ligand complex internalization, recycling and degradation processes interlink depending on the cell type, receptor surface concentration, any homo- and hetero-dimerization, the presence, concentration and nature of the ligands, if known, and even the epitope/s involved.Citation28,Citation39,Citation44–52 In principle, these features can be studied and characterized in vitro, but the effort is considerable with no guarantee that the cells in culture are quantitatively representative to those in tissue environment. For example, the internalization step rate constant values that are measured in vitro tend to be substantially higher (Supplementary Table S2) than the composite internalization rate constant values estimated from the curve fitting of plasma PK data, as listed in . In the context of overall receptor-ligand complex dynamics, the internalization rate constant estimated from the PK data is therefore more closely identifiable as the irreversible degradation reaction for the internalized drug-target complex rather than the internalization step per se. This is especially noticeable in the case of fitting scenarios (3) where a fraction of the target was allocated in the vascular space where it would exert an effect onto mAb PK almost immediately, as can be seen in both for EGFR and HER2.
The simulation results outlined in illustrate the distribution of antibody dose in the different organs. First of all, despite a 25- to 50-fold difference in receptor number between cancer and healthy cells, solid cancer tissue is predicted to catabolize only about 3- to 4-fold more mAb per volume unit (), with the lungs and skin reaching even higher levels in the case of EGFR due to a high local concentration of the target and convection-carried extravasation of mAbs in normal tissues. Interestingly, the presence of high levels of EGFR in the skin aligns well with preclinical and clinical observations of skin-related toxicities for EGFR-neutralizing interventions.Citation53 Surprisingly, in absolute terms, solid tumor mAb uptake translates to only about 0.05% of the dose in the model (), which is in agreement with the experimental clinical data which measures around 0.1%.Citation54,Citation55 Hence, mAbs acting as ‘magic bullets’Citation56 miss the mark most of the time due to system-wide distribution of the dose in addition to the combined effect of nonspecific catabolism through macropinocytosis and target-specific elimination through TMDD. Unlike small molecule drugs, which are predominantly eliminated in the liver and kidney, biologics are catabolized in all organs of the body. Whilst the non-recycled fraction of unconjugated mAbs are broken down into harmless amino acids in the cells, the payload of ADCs can be retained if it is of low plasma membrane permeability even after the carrier mAb is degraded. The modeling results can therefore be extrapolated to the ADCs, which are similar to the parent mAbs in their target and FcRn-binding affinities, as well as plasma PK. This potential toxicity of ADCs therefore needs to be considered since the conjugated small molecule payloads can affect every cell that it ends up in and not only the intended tumor target cells. This can be especially relevant in the context of preclinical xenograft disease models where the mAb does not cross-react with endogenous antigen, thus exposing the target to a higher level of the drug than expected to prevail in patient populations.
In summary, we have established a framework that allows the incorporation of quantitative mass spectrometric protein tissue concentration data into the PBPK modeling framework in order to facilitate a mechanistic insight into target engagement in different organs of the body, including solid tumors. The framework presented complements the previous and current modeling and simulation efforts where the focus was/is on tissue distribution of biologics, as per Reig-Lopez et al. who also focused on EGFR and HER2Citation57 in the context of PBPK in relation to target expression level and turnover. The mass spectrometric tissue concentration data are critical in this aspect since they allow the elimination of one of the unknowns in the TMDD formalism, which otherwise is strongly cross-correlated with the internalization rate constant value. The approach described herein allows one to analyze the predicted level and extent of drug-target engagement in different organs and to identify factors that may have an impact on specificity and bystander cell exposure, with significant implications associated with drug efficacy and safety. Although the best-fitting results both for EGFR and HER2 data incorporated adjustments to the overall calculated expression levels, the corrections remained within the uncertainty of the model and may also have reflected the intracellular fractions of the targets. This suggests that PK and engagement predictions for novel mAbs can be considered if there is reliable estimate for the target-mediated drug degradation rate constant. Given that the PaxDb database describes endogenous proteins, the information retrieved can also be expected to guide quantitative systems pharmacology models.
Abbreviations
mAb | = | Monoclonal antibody |
ADC | = | Antibody-drug conjugate |
PBPK | = | Physiologically-based pharmacokinetics |
EGFR | = | Epidermal growth factor receptor |
HER2 | = | Erb-b2 receptor tyrosine kinase 2 |
TMDD | = | Target-mediated drug disposition |
ppm | = | Part per million |
RL | = | Relative likelihood |
AIC | = | Akaike information criterion |
Author contributions
M.M. and A.S. designed the research, performed the research, analyzed the data and wrote the manuscript.
KMAB-2023-0230R1_supp material_final.docx
Download MS Word (3.2 MB)Acknowledgments
The authors wish to acknowledge expert help from Ms Ruth Clayton in preparing this manuscript and thank Dr Tamara van Steeg for her valuable comments.
Disclosure statement
No potential conflict of interest was reported by the author(s).
Supplementary material
Supplemental data for this article can be accessed online at https://doi.org/10.1080/19420862.2024.2324485
Additional information
Funding
References
- Crescioli , S, Kaplon H, Chenoweth, A, Wang, L, Visweswaraiah, J, Reichert JM. Antibodies to watch in 2024. MAbs. 2024;16(1):2297450. doi:10.1080/19420862.2023.2297450.
- Dowden H, Munro J. Trends in clinical success rates and therapeutic focus. Nat Rev Drug Discov. 2019;18(7):495–12. doi:10.1038/d41573-019-00074-z.
- Tarantino P, Tolaney SM. The dawn of the antibody–drug conjugates era: how T-DM1 reinvented the future of chemotherapy for solid tumors. Cancer Res. 2022;82(20):3659–3661. doi:10.1158/0008-5472.CAN-22-2324.
- Martineau P, Watier H, Pèlegrin A, Turtoi A. Targets for MAbs: innovative approaches for their discovery & validation, LabEx MAbImprove 6th antibody industrial symposium, June 25-26, 2018, Montpellier, France. MAbs. 2019;11(5):812–25. doi:10.1080/19420862.2019.1612691.
- An G. Concept of Pharmacologic Target-Mediated Drug Disposition in Large-Molecule and Small-Molecule Compounds. J Clin Pharmacol. 2020;60(2):149–163. doi:10.1002/jcph.1545.
- Morgan P, Van Der Graaf PH, Arrowsmith J, Feltner DE, Drummond KS, Wegner CD, Street SDA. Can the flow of medicines be improved? Fundamental pharmacokinetic and pharmacological principles toward improving phase II survival. Drug Discovery Today. 2012;17(9–10):419–24. doi:10.1016/j.drudis.2011.12.020.
- Huang Q, Szklarczyk D, Wang M, Simonovic M, von Mering C. PaxDb 5.0: Curated Protein Quantification Data Suggests Adaptive Proteome Changes in Yeasts. Mol Cell Proteomics. 2023;22(10):100640. doi:10.1016/j.mcpro.2023.100640.
- Wang M, Weiss M, Simonovic M, Haertinger G, Schrimpf SP, Hengartner MO, von Mering C. PaxDb, a Database of Protein Abundance Averages Across All Three Domains of Life. Molecular & Cellular Proteomics: MCP. 2012;11(8):492–500. doi:10.1074/mcp.O111.014704.
- Sepp A, Bergström M, Davies M. Cross-species/cross-modality physiologically based pharmacokinetics for biologics: 89Zr-labelled albumin-binding domain antibody GSK3128349 in humans. MAbs. 2020;12(1):e1832861. doi:10.1080/19420862.2020.1832861.
- Sepp A, Meno-Tetang G, Weber A, Sanderson A, Schon O, Berges A. Computer-assembled cross-species/cross-modalities two-pore physiologically based pharmacokinetic model for biologics in mice and rats. J Pharmacokinet Pharmacodyn. 2019;46(4):339–359. doi:10.1007/s10928-019-09640-9.
- Hsu JL, Hung M-C. The role of HER2, EGFR, and other receptor tyrosine kinases in breast cancer. Cancer Metastasis Rev. 2016;35(4):575–588. doi:10.1007/s10555-016-9649-6.
- Bertelsen V, Stang E. The mysterious ways of ErbB2/HER2 trafficking. Membranes (Basel). 2014;4(3):424–46. doi:10.3390/membranes4030424.
- Wegler C, Wiśniewski JR, Robertsen I, Christensen H, Kristoffer Hertel J, Hjelmesæth J, Jansson-Löfmark R, Åsberg A, Andersson TB, Artursson P. Drug disposition protein quantification in matched human jejunum and liver from donors with obesity. Clin Pharma And Therapeutics. 2022;111(5):1142–54. doi:10.1002/cpt.2558.
- Uhlén M, Fagerberg L, Hallström BM, Lindskog C, Oksvold P, Mardinoglu A, Sivertsson Å, Kampf C, Sjöstedt E, Asplund A. et al. Tissue-based map of the human proteome. Science. 2015;347(6220):1260419. doi:10.1126/science.1260419.
- Graf JF, Scholz BJ, Zavodszky MI. BioDMET: a physiologically based pharmacokinetic simulation tool for assessing proposed solutions to complex biological problems. J Pharmacokinet Pharmacodyn. 2012;39(1):37–54. doi:10.1007/s10928-011-9229-x.
- Brown GC. Total cell protein concentration as an evolutionary constraint on the metabolic control distribution in cells. J Theor Biol. 1991;153(2):195–203. doi:10.1016/S0022-5193(05)80422-9.
- Wisniewski JR, Hein MY, Cox J, Mann M. A “proteomic ruler” for protein copy number and concentration estimation without spike-in standards. Molecular & Cellular Proteomics: MCP. 2014;13(12):3497–3506. doi:10.1074/mcp.M113.037309.
- Leeman M, Choi J, Hansson S, Storm MU, Nilsson L. Proteins and antibodies in serum, plasma, and whole blood—size characterization using asymmetrical flow field-flow fractionation (AF4). Anal Bioanal Chem. 2018;410(20):4867–4873. doi:10.1007/s00216-018-1127-2.
- Wiig H, Swartz MA. Interstitial fluid and lymph formation and transport: physiological regulation and roles in inflammation and cancer. Physiol Rev. 2012;92(3):1005–1060. doi:10.1152/physrev.00037.2011.
- Muliaditan M, Sepp A. Application of quantitative protein mass spectrometric data in the early predictive analysis of target engagement by monoclonal antibodies. Clin Transl Sci. 2022;15(7):1634–1643. doi:10.1111/cts.13278.
- Tokuda Y, Watanabe T, Omuro Y, Ando M, Katsumata N, Okumura A, Ohta M, Fujii H, Sasaki Y, Niwa T. et al. Dose escalation and pharmacokinetic study of a humanized anti-HER2 monoclonal antibody in patients with HER2/neu-overexpressing metastatic breast cancer. Br J Cancer. 1999;81(8):1419–1425. doi:10.1038/sj.bjc.6690343.
- Fracasso PM, Burris H III, Arquette MA, Govindan R, Gao F, Wright LP, Goodner SA, Greco FA, Jones SF, Willcut N. et al. A phase 1 escalating single-dose and weekly fixed-dose study of Cetuximab: pharmacokinetic and pharmacodynamic rationale for dosing. Clin Cancer Res. 2007;13(3):986–993. doi:10.1158/1078-0432.CCR-06-1542.
- Rohatgi A. WebPlotDigitizer. https://automeris.io/WebPlotDigitizer.
- Wang W, Yin L, Gonzalez-Malerva L, Wang S, Yu X, Eaton S, Zhang S, Chen H-Y, LaBaer J, Tao N. In situ drug-receptor binding kinetics in single cells: a quantitative label-free study of anti-tumor drug resistance. Sci Rep. 2014;4(1):6609. doi:10.1038/srep06609.
- Patel D, LahuiI A, Patel SP, Franklin M, Jiminez X, Hicklin DJ, Kang X. Monoclonal antibody cetuximab binds to and down-regulates constitutively activated epidermal growth factor receptor vIII on the cell surface. Anticancer Res. 2007;27:3355–66.
- Leyton JV. Improving receptor-mediated intracellular access and accumulation of antibody therapeutics—the tale of HER2. Antibodies. 2020;9(3):32. doi:10.3390/antib9030032.
- Sakai A, Tagami M, Kakehashi A, Katsuyama-Yoshikawa A, Misawa N, Wanibuchi H, Azumi A, Honda S. Expression, intracellular localization, and mutation of EGFR in conjunctival squamous cell carcinoma and the association with prognosis and treatment. PloS One. 2020;15(8):e0238120. doi:10.1371/journal.pone.0238120.
- Stüber JC, Kast F, Plückthun A. High-throughput quantification of surface protein internalization and degradation. ACS Chem Biol. 2019;14(6):1154–1163. doi:10.1021/acschembio.9b00016.
- Narod SA. Narod SA: disappearing breast cancers. Curr Oncol. 2012;19(2):59–60. doi:10.3747/co.19.1037.
- Majumder S, Islam MT, Righetti R. Non-invasive imaging of interstitial fluid transport parameters in solid tumors in vivo. Sci Rep. 2023;13(1):7132. doi:10.1038/s41598-023-33651-9.
- Gullick WJ, Marsden JJ, Whittle N, Ward B, Bobrow L, Waterfield MD. Expression of epidermal growth factor receptors on human Cervical, Ovarian, and Vulval Carcinomas. Cancer Res. 1986;46:285–92.
- Onsum MD, Geretti E, Paragas V, Kudla AJ, Moulis SP, Luus L, Wickham TJ, McDonagh CF, MacBeath G, Hendriks BS. Single-cell quantitative HER2 measurement identifies heterogeneity and distinct subgroups within traditionally defined HER2-positive patients. The American Journal Of Pathology. 2013;183(5):1446–60. doi:10.1016/j.ajpath.2013.07.015.
- Akaike H. An information criterion (AIC). Math Sci. 1976;14:5–9.
- Dunlap T, Cao Y. Physiological considerations for modeling in vivo antibody-target interactions. Front Pharmacol. 2022;13:13. doi:10.3389/fphar.2022.856961.
- Li X, Jusko W, Cao Y. Role of interstitial fluid turnover on target suppression by therapeutic biologics using a minimal physiologically-based pharmacokinetic (mPBPK) model. J Pharmacol Exp Ther. 2018;367(1):1–8. doi:10.1124/jpet.118.250134.
- Plum PS, Gebauer F, Krämer M, Alakus H, Berlth F, Chon S-H, Schiffmann L, Zander T, Büttner R, Hölscher AH. et al. HER2/neu (ERBB2) expression and gene amplification correlates with better survival in esophageal adenocarcinoma. BMC Cancer. 2019;19(1):38. doi:10.1186/s12885-018-5242-4.
- Bianconi E, Piovesan A, Facchin F, Beraudi A, Casadei R, Frabetti F, Vitale L, Pelleri MC, Tassani S, Piva F. et al. An estimation of the number of cells in the human body. Ann Hum Biol. 2013;40(6):463–471. doi:10.3109/03014460.2013.807878.
- Wee P, Wang Z. Epidermal growth factor receptor cell proliferation signaling pathways. Cancers. 2017;9(5):52. doi:10.3390/cancers9050052.
- Sorkin A, Duex JE. Quantitative analysis of endocytosis and turnover of epidermal growth factor (EGF) and EGF receptor. Curr Protoc Cell Biol. 2010;46(1):15–4. Chapter 15:Unit 15 14. doi:10.1002/0471143030.cb1514s46.
- Larson JS, Goodman LJ, Tan Y, Defazio-Eli L, Paquet AC, Cook JW, Rivera A, Frankson K, Bose J, Chen L. et al. Analytical validation of a highly quantitative, sensitive, accurate, and reproducible assay (HERmark®) for the measurement of HER2 total protein and HER2 homodimers in FFPE breast cancer tumor specimens. Patholog Res Int. 2010;2010:1–14. doi:10.4061/2010/814176.
- Gutierrez C, Schiff R. HER2: biology, detection, and clinical implications. Arch Pathol Lab Med. 2011;135(1):55–62. doi:10.5858/2010-0454-RAR.1.
- Mager DE, Jusko WJ. General pharmacokinetic model for drugs exhibiting target-mediated drug disposition. J Pharmacokinet Pharmacodyn. 2001;28(6):507–532. doi:10.1023/A:1014414520282.
- van Waterschoot RAB, Parrott NJ, Olivares-Morales A, Lavé T, Rowland M, Smith DA. Impact of target interactions on small-molecule drug disposition: an overlooked area. Nat Rev Drug Discov. 2018;17(4):299–299. doi:10.1038/nrd.2018.26.
- Hendriks BS, Opresko LK, Wiley HS, Lauffenburger D. Coregulation of Epidermal Growth Factor Receptor/Human Epidermal Growth Factor Receptor 2 (HER2) Levels and Locations. Quant Anal HER2 Overexpression Eff. 2003;63:1130–37.
- Durbin KR, Phipps C, Liao X. Mechanistic modeling of antibody–drug conjugate internalization at the cellular level reveals inefficient processing steps. Mol Cancer Ther. 2018;17(6):1341–1351. doi:10.1158/1535-7163.MCT-17-0672.
- Lammerts van Bueren JJ, Bleeker WK, Bøgh HO, Houtkamp M, Schuurman J, van de Winkel JGJ, Parren PWHI. Effect of target dynamics on pharmacokinetics of a novel therapeutic antibody against the epidermal growth factor receptor: implications for the mechanisms of action. 2006;66(15):7630–38. doi:10.1158/0008-5472.CAN-05-4010.
- Cheng J, Liang M, Carvalho MF, Tigue N, Faggioni R, Roskos LK, Vainshtein I. Molecular mechanism of HER2 rapid internalization and redirected trafficking induced by anti-HER2 biparatopic antibody. Antibodies (Basel). 2020;9(3):49. doi:10.3390/antib9030049.
- Austin CD, De Mazière AM, Pisacane PI, van Dijk SM, Eigenbrot C, Sliwkowski MX, Klumperman J, Scheller RH. Endocytosis and sorting of ErbB2 and the site of action of cancer therapeutics trastuzumab and Geldanamycin. Mol Biol Cell. 2004;15(12):5268–82. doi:10.1091/mbc.e04-07-0591.
- Fehling-Kaschek M, Peckys DB, Kaschek D, Timmer J, Jonge N. Mathematical modeling of drug-induced receptor internalization in the HER2-positive SKBR3 breast cancer cell-line. Sci Rep. 2019;9(1):12709. doi:10.1038/s41598-019-49019-x.
- Maass KF, Kulkarni C, Betts AM, Wittrup KD. Determination of cellular processing rates for a Trastuzumab-Maytansinoid Antibody-Drug Conjugate (ADC) highlights key parameters for ADC design. AAPS J. 2016;18(3):635–646. doi:10.1208/s12248-016-9892-3.
- Rudnick SI, Lou J, Shaller CC, Tang Y, Klein-Szanto AJP, Weiner LM, Marks JD, Adams GP. Influence of affinity and antigen internalization on the uptake and penetration of anti-HER2 antibodies in solid tumors. Cancer Res. 2011;71(6):2250–2259. doi:10.1158/0008-5472.CAN-10-2277.
- Pereira PMR, Sharma SK, Carter LM, Edwards KJ, Pourat J, Ragupathi A, Janjigian YY, Durack JC, Lewis JS. Caveolin-1 mediates cellular distribution of HER2 and affects trastuzumab binding and therapeutic efficacy. Nat Commun. 2018;9(1):5137. doi:10.1038/s41467-018-07608-w.
- Yano S, Kondo K, Yamaguchi M, Richmond G, Hutchison M, Wakeling A, Averbuch S, Wadsworth P. Distribution and function of EGFR in human tissue and the effect of EGFR tyrosine kinase inhibition. Anticancer Res. 2003;23:3639–50.
- Epenetos AA, Snook D, Durbin H, Johnson PM, Taylor-Papadimitriou J. Limitations of radiolabeled monoclonal antibodies for localization of human neoplasms. Cancer Res. 1986;46:3183–91.
- Mach J-P, Carrel S, Forni M, Ritschard J, Donath A, Alberto P. Tumor localization of radio-labeled antibodies against carcinoembryonic antigen in patients with carcinoma. N Engl J Med. 1980;303(1):5–10. doi:10.1056/NEJM198007033030102.
- Enever C, Batuwangala T, Plummer C, Sepp A. Next generation immunotherapeutics-honing the magic bullet. Curr Opin Biotechnol. 2009;20(4):405–11. doi:10.1016/j.copbio.2009.07.002.
- Reig-Lopez J, Tang W, Fernandez-Teruel C, Merino-Sanjuan M, Mangas-Sanjuan V, Boulton DW, Sharma P. Application of population physiologically based pharmacokinetic modelling to optimize target expression and clearance mechanisms of therapeutic monoclonal antibodies. Brit J Clinical Pharma. 2023;89(9):2691–702. doi:10.1111/bcp.15745.