ABSTRACT
The development of bispecific antibodies that bind at least two different targets relies on bringing together multiple binding domains with different binding properties and biophysical characteristics to produce a drug-like therapeutic. These building blocks play an important role in the overall quality of the molecule and can influence many important aspects from potency and specificity to stability and half-life. Single-domain antibodies, particularly camelid-derived variable heavy domain of heavy chain (VHH) antibodies, are becoming an increasingly popular choice for bispecific construction due to their single-domain modularity, favorable biophysical properties, and potential to work in multiple antibody formats. Here, we review the use of VHH domains as building blocks in the construction of multispecific antibodies and the challenges in creating optimized molecules. In addition to exploring traditional approaches to VHH development, we review the integration of machine learning techniques at various stages of the process. Specifically, the utilization of machine learning for structural prediction, lead identification, lead optimization, and humanization of VHH antibodies.
Introduction
Over the past 10 y bispecific antibodies (BsAbs) have emerged as a promising therapeutic for a wide variety of disease indications, most notably in oncology, autoimmune disease, and inflammation. Due to the unique ability to engage at least two separate binding sites within the same antibody format, BsAbs have been used to engage multiple soluble targets (e.g. cytokines), act as bispecific cell engagers (e.g. immune cell engagers) or bind to two different epitopes on a single molecule (e.g. biparatopic antibodies).Citation1 BsAbs can consist of different types of antibody-based fragments, such as single-chain variable fragments (scFv) and fragment antigen-binding regions (Fabs), within the same antibody scaffold. Single-domain antibodies are an increasingly popular choice of building blocks for bispecific antibody construction due to their small size, ease of production, and stability at a wide range of temperatures and chemical environments. In particular, the variable heavy domains of heavy-chain-only antibodies (VHH), derived from camelid heavy-chain-only antibodies (HCAbs) are emerging as integral building blocks, increasing the number of formats available to researchers for BsAb construction. A key feature of VHHs lies in the absence of a light chain, offering a streamlined approach to assembly and production. The absence of a light chain provides modularity, enhancing their suitability as building blocks for bispecifics and enabling the creation of versatile multispecific formats beyond traditional bispecific antibody structures. Although VHH therapeutics such as Sanofi’s caplacizumab (CABLIVI) and Ablynx-developed ozoralizumab (Nanozora) have been approved, the exploitation of VHHs as components of bispecific antibodies is still in its infancy. Here, we discuss recent applications and challenges of using VHH domains in bi- and multispecific antibody production. In addition, we explore how machine learning-based approaches can be used to help design optimal VHH-based bispecific and multispecific antibodies.
The structure and engineering of VHHs
Conventional antibodies are composed of two heavy chains and two light chains forming a tetrameric immunoglobulin G (IgG) molecule (). These chains have variable regions that bind the target antigen and constant regions to determine the mechanism of antigen elimination. The variable heavy chain and variable light chain domains are each composed of three complementarity-determining regions (CDRs) that together form the antigen binding site. The serum from camelids, including camels, llamas, and alpacas contains not only the conventional IgG isotype antibodies but also homodimeric HCAbs, which lack the light chain and the first constant Ig domain of the heavy chain (CH1) ().Citation2 These HCAbs possess a single variable binding domain responsible for antigen binding, termed VHH (). Similarly, sharks produce a unique type of single-domain antibody known as VNARs which are likewise composed exclusively of heavy chains, demonstrating convergent evolutionary adaptations in immune defense mechanisms across species.Citation3 As with variable domains from conventional antibodies, VHHs are composed of four separate frameworks (FW1-4), which are separated by three hypervariable complementarity-determining loops (CDR1-3) and adopt the typical Ig fold structure consisting of nine β-strands in two closely packed layers of anti-parallel β-pleated sheets, resulting in a β-barrel structure ().Citation4–6 In VHH domains, CDR3 loops are generally longer compared to those found in human VH domains.Citation7,Citation8 A comparison of CDR3 lengths in human VH sequences and VHH sequences within the Observed Antibody Space (OAS)Citation9 revealed that, on average, VHH domains have CDR3 loops that are 1.4 residues longer.Citation10 Due to the long CDR3s, VHH domains can exist with structural conformations beyond those seen in conventional antibodies.Citation11 Indeed, camel and llama VHHs may possess an additional disulfide bond between CDR1 and CDR3 or CDR2 and CDR3, respectively, thereby increasing conformational stability of these long CDR3 loop structures.Citation12,Citation13 The longer extension of the CDR3 loop is thought to allow the VHH to bind concave epitopes such as active sites of enzymes and pore regions of membrane receptors.Citation14–16
Figure 1. Schematic representation of a conventional antibody vs a camelid-derived heavy chain-only antibody. (A) Conventional antibodies are composed of two heterodimeric chains, two identical heavy chain fragments consisting of the constant heavy domains CH1-CH3 and the antigen binding variable heavy domain (highlighted in pink) and two identical light chains composed of a constant light-chain domain and a variable light chain domain (highlighted in blue). (B) HCAb antibodies are composed of two identical heavy chain-only fragments that lack a CH1 domain and only have a single variable heavy domain (VHH). (C) Ribbon representation of the crystal structure of a VHH domain (PDB: 1I3V) showing the complementary-determining loops, CDR1 in green, CDR2 in magenta and CDR3 in orange. Framework regions are shown in gray. 3D structure created using Pymol.

Why are VHHs good building blocks?
In the traditional antibody format, the pairing of the VH and VL chains typically depends on hydrophobic interactions. These interactions often limit the solubility of human VHs when they are not paired with a light chain. A distinctive feature of VHH domains is the presence of hallmark residues in FW2 at positions 37 (F/Y), 44 (E), 45 (R) and 47 (G) that help form a hydrophilic surface patch, providing VHH domains with increased solubility and stability in the absence of a variable light domain.Citation4,Citation17,Citation18 Conventional VH domains pair these positions with the light chain variable domain, but VHH domains have evolved to function in the absence of this pairing and therefore have escaped those evolutionary constraints (). In addition, the long CDR3 in VHHs is also known to fold back over FW2, providing additional stability in the absence of a light chain.Citation19,Citation20 The presence of these hallmark residues enables improved expression of VHH domains compared to a human VH domain, which may display both yield and aggregation issues upon expression. Extensive engineering work is often required to improve human VH domain expression and solubility.Citation21,Citation22 “Camelization” can involve mutating the corresponding framework 2 positions in a human VHs to those observed in a VHH.Citation21,Citation23,Citation24
Figure 2. Structural representation of framework 2 residues in human VH and camelid VHHs. Ribbon representation of the crystal structure of (A) human VH (PDB: 7OBF) and (B) camelid-derived VHH (PDB: 1JTT) highlighting the framework 2 positions for each domain. Position 37: Human Valine, Camelid Phenylalanine (red), Position 44: Human Glycine, Camelid Glutamic acid (blue), position 45: Human Leucine, Camelid Arginine (yellow) and position 47: Human Tryptophan, Camelid Glycine (cyan); Kabat numbering. The interaction position of the human variable light chain with the human variable heavy chain is represented by the dotted line. The complementary-determining loops, CDR1 in green, CDR2 in magenta and CDR3 in orange. Framework regions are shown in gray. 3D structure created using Pymol.
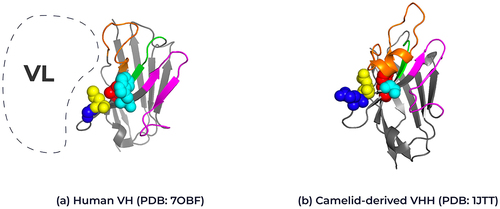
In addition to the increased solubility, VHHs can also display enhanced thermal stability, with some VHHs reaching melting temperatures above 60°C.Citation25,Citation26 More importantly, VHHs can also retain antigen binding after heat denaturation due to their ability to refold into a functionally relevant conformation.Citation27–29 The ability to refold is an attractive property, particularly if refolding is independent of formulation conditions. Despite high levels of thermal stability, there have been many attempts to improve VHH stability even further by mutagenesis of critical residues. Detailed analysis of CDR loop formation during library construction has shown that the CDR loops of VHH domains may adopt a particular structure depending on several factors.Citation30–32 Murakami et al. postulated that VHHs may prefer antigenic epitopes that form clefts or deep pockets that the longer CDR3 loops may be able to penetrate. To this end, they analyzed the crystal structures of VHHs in complex with antigens and proposed a classification for paratope formation. Depending on CDR3 loop length and structure, they define the loop formations as either upright, half-roll or roll. Interestingly, for upright loops, they showed that FW2 residues are involved in paratope formation, and therefore these residues may need to be considered when designing VHH-based phage libraries. Moreover, Kinoshita et al. have shown that variation in FW2 residues, along with CDR1–3 loop length, may also influence CDR conformation.Citation33
Compared to immunoglobulins from other species such as mouse and rat, camelid VHH domains share a higher sequence similarity with human VH domains, for example, > 80% for VH3–23, therefore reducing the potential for immunogenicity.Citation12,Citation34 Although several studies have shown a lack of immune response to VHH-based antibodies in both mice and humans,Citation35,Citation36 a successful humanization strategy has been adopted to generate a universal VHH-based scaffold.Citation37 Despite numerous studies showing minimal immune responses to VHHs, individuals have been identified with preexisting anti-drug reactivity (PEAR) against the C-termini of some VHHs, which complicates the immunogenicity assessment of these biotherapeutics.Citation38,Citation39 The detection of PEAR during clinical immunogenicity assessment is significant because it can obscure the true immunogenic response to the drug and potentially affect both the safety and efficacy profiles of the therapeutic agents. This phenomenon is also observed in fully human heavy chains, Fabs and scFvs.Citation40,Citation41 A comprehensive understanding of the biological mechanisms underlying this occurrence is still required.Citation42 In one study, the addition of two proline residues at the VHH C-terminus was shown to substantially reduce PEAR.Citation38 In another study, the introduction of a C-terminal alanine proved effective in diminishing the binding of preexisting human anti-VH antibodies during in vitro screening.Citation43 These findings suggest potential pathways for mitigating the immunogenicity challenges posed by these antibodies in therapeutic applications.
In terms of production, VHH domains can be made in most heterologous expression systems, including E. coli, S. cerevisiae, P. pastoris and mammalian cells. E. coli systems are often the preferred choice due to the ease of use, cost of production and yield. However, the lack of relevant post-translational modifications in E. coli may affect physicochemical properties and function of VHHs, particularly when compared to production in mammalian cells.Citation44–46
One potential issue of using VHHs for therapeutic applications is their small size (~15 kDa). This may lead to fast renal clearance, resulting in poor pharmacokinetics and limited biodistribution. For example, the 55 kDa bispecific T-cell engager blinatumomab has a serum half-life of several hours, thereby requiring continuous intravenous infusion.Citation47 However, the rapid clearance of VHHs has a distinct advantage for some diagnostic applications, such as bio-imaging or for therapeutic applications where a short plasma half-life is sufficient or preferred.Citation48,Citation49 To extend the half-life of VHH, binding domains against albumin can be fused into small antibody fragments such as scFv and single-domain antibody (sdAb) or an IgG Fc domain can be incorporated in the molecule. The inclusion of albumin-binding sdAb is an effective strategy to improve the serum half-life of small nanobody therapeutics, as the extended half-life of albumin is approximately 3 weeks mainly due to neonatal Fc receptor (FcRn)-mediated recycling, a property it has in common with the Fc domain of canonical IgG antibodies.Citation50 The genetic fusion to an IgG Fc domain also results in a longer plasma half-life and offers the added advantage of being able to use Fc-mediated purification and analytical strategies.
Generation of VHH antibody domains
VHH antibodies are typically sourced from the blood of naive or immunized llamas or alpacas and used to generate a VHH antibody phage library or from synthetic libraries. Muyldermans recently published a comprehensive overview covering different methods for nanobody generation.Citation51 One of the main advantages of an immunized VHH library is that the VHH domains have been affinity matured in vivo by somatic hypermutation and expressed on the B cells, which may translate into a more favorable developability profile in vitro. As described above, camelid serum contains both conventional IgG antibodies and HCAbs. After serum collection from immunized animals, HCAb-specific IgGs are preferentially amplified by PCR and cloned into a phage display vector for in vitro selection purposes.
Recently, advances in computational approaches and data-mining techniques have allowed the development of synthetic VHH antibody library construction. Open source next-generation sequencing databases such as the Observed Antibody Space (OAS) and the Integrated Nanobody Database for Immunoinformatics (INDI) combined with structural information from the Protein Data Bank (PDB) has enabled the ability to construct VHH domain-based antibody libraries and allow complete control of structure-related sequences and removal of sequence-based manufacturing liabilities.Citation52–54 The application of large sets of antibody sequence analysis, combined with structural information, has led to the production of several sophisticated VHH-based libraries that focus on the unique properties of VHH CDR loop structure.Citation30,Citation32,Citation55,Citation56
Using either an immunized or synthetic VHH-based phage display library, the combination of sequence and functional characterization and novel machine learning approaches is a powerful method for identifying therapeutic antibodies ( and ML sections below).
Figure 3. Overview of VHH antibody discovery methods. VHH antibodies are typically sourced from either alpacas or llamas after immunization with an antigen of choice. An alternative source can be a naive library from llamas or alpacas, or a synthetic library design using a combination of sequence and structural information. Constructed libraries can be interrogated by phage display and combined with a machine learning approach to identify key attributes for affinity, developability and function.

VHH architecture in bispecific antibodies
VHH topology can play a key role in the potency and biophysical properties of VHH-based bispecific antibodies, with the orientation of specific VHH domains known to affect binding and potency toward some targets as demonstrated by De Bruin et al. They generated two multispecific constructs consisting of an anti-Vγ9 Vδ2-TCR VHH domain, 6H4, and an anti-EGFR VHH domain, 7D12 fused via a flexible Gly4Ser linker. Both orientations were generated, with one construct having the anti-Vγ9 Vδ2-TCR VHH domain at the N-terminus, and the other having the anti-EGFR VHH domain at the N-terminus. De Bruin et al. reported that the orientation for an anti-Vγ9 Vδ2-TCR VHH 6H4 domain was not important to retain binding to the T-cell receptor (TCR) because the anti-Vγ9 Vδ2-TCR VHH 6H4 domain could bind at either the N- or C-terminal position. In contrast, the anti-epidermal growth factor receptor (EGFR) VHH position was critical with an N-terminal position favored. Moreover, the length of the Gly4Ser linker between the VHH domains had no influence on activity.Citation57
Positional effects were also documented for a tandem VHH bispecific molecule targeting CD16 (a natural killer (NK) cell marker) and EGFR.Citation58 The authors noted an N-terminal preference for each targeting VHH domain, with an N-terminal anti-CD16 VHH displaying a higher binding capacity for NK cells compared to an N-terminal anti-EGFR VHH domain and the reverse orientation displaying opposite effects. Interestingly, both molecules were effective at inducing NK cell-specific degranulation of EGFR-positive cells, but the bispecific with the N-terminal CD16-binding VHH domain was able to induce a stronger NK cell degranulation at lower concentrations.
The positional flexibility of VHH domains may depend on the targeted antigen and accessibility of the antigen-binding loops when the VHH is placed C-terminal to another protein domain. Alvarez-Cienfuegos et al. recently described an elegant example of producing trimeric molecules called trimerbodies. By fusing an anti-carcinoembryonic antigen (CEA) VHH N-terminally of a collagen trimerization domain (TIEXVIII) they were able to express and purify monospecific trimeric VHH molecules that bound to CEA.Citation59 The addition of a Gly-Ser linker between each VHH to make a tandem trimerbody ensured stoichiometric presentation. The authors went on to show that the presentation of three different VHHs could produce a functional trispecific molecule, termed ATTACK (Asymmetric Tandem Trimerbody for T cell Activation and Cancer Killing).Citation60
While multispecific antibody constructs allow binding or neutralization of two or more targets with one molecule, they can also be engineered to target cells selectively. Using avidity as a guiding principle, a bispecific antibody could be engineered to bind only when two different targets are co-expressed on a cell, while ignoring cells that express only one of the two targets. Such an AND gate can be used to design, for instance, immune cell engagers with superior on-target off-tumor selectivity, resulting in better therapeutic windows while killing tumor cells expressing both targets and leaving normal cells expressing just one or the other target unharmed.Citation61,Citation62 Here too, VHHs are ideally suited as building blocks for such multispecific immune cell engagers.
Importance of geometry/affinity/valency in VHH-based bispecifics
Engineering in multivalency either to multiple different targets or to the same target is an approach that could dramatically improve the selectivity and therefore the safety profile of, for example, an immuno-oncology antibody.Citation63
Increasing the valency by introducing multiple VHHs, which, for example, bind a target such as a tumor-associated antigen (TAA) at a lower affinity, can deliver avidity-driven selectivity. Such a design provides an inherent receptor density sensor enabling engagement only when the target TAA is present at a higher level than that found in healthy tissue, a property typically observed for many tumor targets. Since many of these targets retain low expression in healthy tissue, nonselective tumor-targeting antibodies (e.g. antibody–drug conjugate, immune cell engager molecules) would still engage normal cells and generate unwanted adverse events during treatment (). A similar avidity-driven approach can be used to target two receptors co-expressed on a tumor cell but not on normal cells. An avidity-driven selective antibody should provide a much safer drug profile and reduce therapeutic adverse events.
Figure 4. Targeting tumor cells co-expressing two different receptors at the cell surface (left) not co-expressed on normal cells with a multispecific VHH, thereby minimizing antibody engagement against healthy cells.
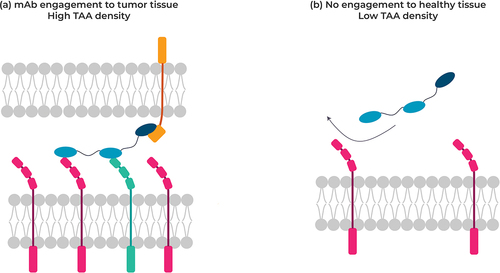
Development of VHHs as bi-and multispecific therapeutics
Over the past 10 y many different formats for bispecific antibodies have been introduced, with many displaying more than two different antigen-binding sites on each arm (). As complexity increased, the ability to purify homogeneous samples has also become more challenging. In particular, the standard monoclonal antibody format requirement to pair different light chains with their cognate heavy chains has resulted in unequal mixtures, often leading to extensive engineering solutions to address the problems. Several strategies have successfully overcome these challenges, such as the use of a common light chain or electrostatic engineering between heavy and light chains.Citation64–66 The use of single-domain VHHs overcomes these shortfalls due to the removal of the light chain pairing heterodimerization, dramatically improving the homogeneity of an overexpressed recombinant product. Along with greater product homogeneity, VHH biophysical properties, such as increased solubility and higher thermal stability compared to VH or VL alone, often also translate to a bispecific molecule with enhanced biophysical characteristics.
As an initial step, VHHs have been genetically fused together into a tandem VHH format using a flexible peptide linker such as a glycine-serine linker, yielding a bispecific molecule. The relatively small size of a tandem VHH molecule may provide improved tissue penetration compared to full IgG-based therapies. Caplacizumab, the first such tandem bivalent, but monospecific, VHH-based molecule to be approved for marketing, consists of two VHH domains separated by a short peptide linker (three alanine residues) illustrated a prototype architecture for similar bispecific formats.Citation67 Caplacizumab binds the von Willebrand factor protein and prevents platelet aggregation, the main contributing factor in acquired thrombotic thrombocytopenic purpura. Caplacizumab has no built-in mechanism to extend its circulation in plasma and, as such, has a short plasma half-life (~16–27 h), therefore requiring daily injections. Ozoralizumab, a bispecific and trivalent tandem VHH molecule originally developed by Ablynx and licensed to Taisho Pharmaceutical, has been approved for rheumatoid arthritis and is marketed in Japan. It consists of two anti-TNFα VHHs separated by an anti-human serum albumin (HSA) VHH.Citation68 These VHHs are fused via a simple G4Sx2 peptide linker to improve flexibility, while the fusion to an anti-HSA nanobody offers an increase in plasma half-life to 18 d. Gefurulimab (ALXN-1720, Alexion) is another example of a bispecific VHH where the half-life of an anti-complement 5 VHH is improved by genetically fusing it to an anti-HSA VHH linked by a G4Sx3 flexible peptide.Citation69 Gefurulimab is currently undergoing evaluation in a Phase 3 clinical trial as a treatment for myasthenia gravis (NCT05556096).
Further examples of tandem multispecific VHHs include M1095 and BI836880. M1095/ALX0761 (sonelokimab) is a trispecific tandem VHH targeting the cytokines IL17A and IL17F as well as HSA. An N-terminal anti-IL17F VHH is linked via a glycine-serine linker to an anti-HSA VHH followed by a C-terminally positioned anti-IL17A VHH. Originally discovered at Ablynx in collaboration with Merck Serono, it has been investigated as a treatment for patients with moderate-to-severe psoriasis (Phase 2b, NCT03384745), moderate-to-severe hidradenitis suppurativa (Phase 2, NCT05322473) and for patients with active psoriatic arthritis (Phase 2, NCT05640245).Citation70
BI836880 is a humanized trispecific nanobody that targets vascular endothelial growth factor (VEGF), angiopoietin 2 and HSA. Targeting the latter supports a longer plasma half-life of the molecule. Similar to M1095, the overall architecture of the therapeutic consists of two VHHs each binding to their respective ligands, separated by an anti-HSA VHH. In blocking two ligands and two separate downstream pathways, the bispecific aims to block both angiogenesis and tumor cell proliferation. It thus has potential for the treatment of solid tumors.Citation71,Citation72
Unless a short plasma half-life is preferred (e.g., for imaging purposes or agonistic antibodies), a moiety that provides a half-life extension is usually added to the antibody construct. This can be a genetic fusion to a VHH that binds to HSA, as described above for M1095 and BI836880, or a genetic fusion to the Fc portion of IgG. In both instances, adding an Fc not only makes the molecules larger and above the typical cutoff size for renal clearance, but importantly, it also facilitates recycling via the FcRn receptor. An example of the latter is the anti-CXCR2 COH105.Citation73 In this construct, two VHHs each binding a different epitope on the CXCR2 chemokine receptor and linked together via a glycine-serine linker are fused to an Fc. As such, this forms a symmetric dimer of a biparatopic receptor antagonist with a half-life in cynomolgus monkeys and humans similar to an IgG. Here too, the final topology of the molecule was arrived at after experimental evaluation of constructs with different glycine-serine linker lengths and different sequential order of the individual anti-CXCR2 VHH elements. Other bispecifics built with VHHs have used a similar architecture. For instance, Alphamed Oncology has evaluated Erfonrilimab (KN046), a VHH-based bispecific targeting PD-L1 and CTLA4, as a treatment for cancer (NCT05149326, see and for a topological representation of some key molecules).Citation74
Figure 5. Topological representation of examples of clinically relevant VHH-based therapeutic bispecifics.

Table 1. Preclinical and clinical stage VHH-based bispecific molecules. All data correct as of 1st September 2023.
Inhibrx’s bispecific tetravalent antibody construct (INBRX105) is another example of sdAb domains fused to an Fc domain for half-life extension. The sdAb modules of INBRX105 target PD-L1 and CD137 (4-1BB). The molecule is currently in a Phase 2 clinical trial in combination with pembrolizumab (NCT03809624).
Trispecific T cell activating constructs (TriTACs) offer yet a different architecture incorporating single-domain antibodies. Here, two sdAbs, one targeting a TAA and one binding to HSA, are genetically fused to an anti-CD3 scFv. With a molecular weight of only about 50KDa, these molecules offer the potential for better tumor penetration yet should have a longer plasma half-life mediated by binding to HSA. Such constructs targeting DLL3 (HPN328,) BCMA (HPN217) and MSLN (HPN536) are currently in early-phase clinical trials (NCT04471727, NCT04184050, NCT03872206, respectively; see and for a topological representation of some key molecules).Citation75,Citation76
VHH-based bispecific antibodies can also be part of the targeting component of CAR-T cells, such as in the CD19/CD20-bispecific CAR-T for the treatment of B-cell lymphoma, currently in Phase 1 clinical trials (NCT03881761).Citation77 For each of these molecules, different individual VHH building blocks will be tested with different linkers of varying length to arrive at an optimally efficacious, potent and manufacturable bispecific molecule.
The application of VHHs in immune-cell recruitment has been elegantly demonstrated by LAVA Therapeutics. LAVA-051 is a humanized VHH-based bispecific antibody that binds specifically to the Vδ2-TCR chain of gamma/delta T-cells (Vγ9 Vδ2) and the MHC class-I like molecule CD1d that is expressed on tumor cells. Interestingly, LAVA-051 is able to recruit not only Vγ9 Vδ2 T-cells but also type-I NK cells (iNKT) cells, resulting in a novel dual immune cell-mediated killing response on CLL and MM cells.Citation78,Citation79 LAVA has extended their GammaBodyTM technology with LAVA-1207, a humanized bispecific antibody containing a modified human IgG1-Fc domain for half-life extension that engages Vγ9 Vδ2 and the prostate-specific antigen (PMSA). LAVA-1207 has shown potent and selective tumor cell killing with low treatment emergent adverse events (NCT05369000).Citation80
The superior utilization of VHHs in a trispecific antibody format has been shown in the application to trispecific killer cell engagers (TriKESTM). Initial studies of scFv16-m15–33, a TriKE consisting of anti-CD16 scFv linked to a mutated IL15 followed by an anti-CD33 scFv displayed potent NK recruitment and cytotoxicity, albeit with increased T-cell proliferation.Citation81 However, previous formats of this TriKE using a wild-type IL15 molecule were nonfunctional, leading the authors to conclude anti-CD16 scFv folding may affect the function of wild-type IL15. Importantly, the direct replacement of the anti-CD16 scFv with a humanized llama anti-CD16 VHH generated a TriKE, cam16-wtIL15–33, that not only allowed the utilization of wild-type IL15 but displayed superior NK cell proliferation, activation and cell killing.Citation82 scFv16-m15–33 (GTB-3550) was evaluated in clinical studies as a treatment for CD33-positive myelodysplastic syndromes but then terminated due to the development of the superior nanobody containing TriKE product (GTB-3650, Clinical Trials.gov NCT03214666).
Although camelid-derived VHHs offer a distinct advantage over human single-domain antibodies in terms of favorable biophysical characteristics, recent advances in the production of human VH domain-only platforms have been documented by Harbour Biomed, Nona Biomed and Crescendo Biologics. However, the production of transgenic mice platforms expressing human VH antibody fragments remains a challenging and costly exercise compared to the relatively simple approach of llama immunizations combined with phage display.
VHH design using machine learning
The application of machine learning to VHH design is a rapidly emerging area of research. Given the recency of machine learning methods being applied to VHH design, the relative scarcity of literature in this field highlights an opportunity for substantial breakthroughs. In this section, we highlight both methods for generic antibody design that may be applicable to VHH and those tailored specifically toward VHHs. We highlight methods that include structural prediction, lead identification, synthetic library design, sequence optimization methods, and humanization.Citation83–85
Computational structural modeling of VHHs
The three-dimensional structure of both the protein target and the VHH is key to developing fully de novo antibodies against a given target. While sequence information provides considerable insight into how the molecule behaves, structural information can reveal important insights, such as which residues are on the surface of the protein, what are the side-chain orientations, and which residues are involved in target binding. In the absence of an experimentally determined structure generated using X-ray crystallography or cryo-EM, computational methods can be used to predict the structure of the protein.Citation86–89 Traditionally, structural prediction has been performed using homology modeling and an energy minimization refinement step to predict the structures of proteins based on a template structure. The introduction of AlphaFold and subsequently AlphaFold2 (AF2) marked a major breakthrough in the protein structural prediction space.Citation87,Citation90 AlphaFold uses a deep learning model that efficiently uses evolutionary and structural information to accurately predict the three-dimensional structure of a given protein. Building on this breakthrough, antibody and VHH-specific structural prediction models that show notable progress in predicting these immune molecules have been developed. These include IgFold, ABLooper, NanoNet, ABodyBuilder2, and also its VHH-specific counterpart NanoBodyBuilder2 ().Citation84,Citation86,Citation88,Citation89 It is important to highlight the disparity in the availability of structural data between VHHs and human antibodies that are available for model training and evaluation. As of December 2023, there are 7,977 antibody structures on the Structural Antibody Database (SAbDab),Citation91 but only 1,186 of these are VHH structures, potentially making VHH structural prediction more challenging. However, given the absence of a light chain or VH/VL interface to predict, VHH structures could be considered less complex to predict structurally compared to traditional antibodies. The advances in antibody and VHH structure prediction have enabled more accurate prediction of other important protein properties, such as solubility, thermostability, aggregation, and binding affinity.Citation92,Citation93 Despite advances in structural prediction, CDR loop prediction remains a challenge, in particular for CDR3, the most diverse loop in sequence and length.Citation94 Additionally, predicting the structure of antibodies and nanobodies using these tools can yield similar yet different predicted structures, introducing uncertainty when using these predicted models to characterize other properties of the molecule.
Figure 6. Overview of machine learning tools for antibodies and VHHs. (A) Tools for structural prediction, encompassing the ImmuneBuilder suite, IgFold, and Nanonet. (B) Language model-based sequence optimization with AbLang, AntiBERTy, IgLM, Sapiens and NanoBERT, alongside Bayesian Optimization techniques like AntBO and LaMBO2. Additionally, humanization tools include Hu-Mab, PLAN and CUMAb.

Predicting the structure of multivalent molecules creates an additional challenge given the conformational flexibility provided by the adjoining linkers used between the individual units of the monovalent molecule. Huang et al. used molecular dynamics simulations in conjunction with AF2 structural predictions to rationalize why multivalent anti-VEGF designed VHHs exhibited more than 100-fold improvement in affinity and ~six-fold increase in VEGF-VEGFR pathway inhibition compared with the monovalent molecule.Citation95 This demonstrates the potential of coupling physics-based approaches such as molecular dynamics simulations with machine learning-based structural predictions for the development of superior multivalent VHHs.
Molecular docking can be used to model the structure of an antibody or VHH in complex with its target, but its application is complicated by the flexibility of CDR loops and limited experimental data on epitope–paratope interactions. General multimeric protein structure prediction tools do not yet generalize well to antibody-antigen binding prediction, though recent versions of AF2 demonstrate consistent improvement.Citation96,Citation97 Notably, AF2 exhibits greater success modeling nanobody-antigen complexes than larger antibody-antigen complexes.Citation97 Larger antibody sequence datasets have enabled improved paratope prediction. For instance, Parapred uses deep learning to predict the paratope residues that may be involved in target binding from the CDR sequence alone.Citation98 Epitope prediction remains a significant challenge in the field; nonetheless, the application of deep learning techniques has demonstrated improvements in antibody-antigen docking.Citation99–101 DLAB-Re is one example of a machine learning method designed for the accurate identification and ranking of docked poses.Citation102 NbX has further demonstrated an ability to improve the ranking of VHH-antigen binding poses.Citation103 One challenge with docking predictions is understanding the energy of the systems. Hummer et al. observed that there is currently insufficient experimental data to predict ∆∆G accurately and robustly, with orders of magnitude more likely needed.Citation104 In contrast, Gaudreault et al. derived a novel scoring method using native output values from the AF2 model that is better calibrated for scoring binding energy than physics-based approaches.Citation96
It is clear that limitations still exist with antibody and VHH structural prediction, particularly around CDR prediction, B-cell epitope prediction, and generating accurate antibody-antigen poses. However, structure-based design is accelerating due to the increased availability of accurate antibody and antigen structure models. As more antibody and VHH structures become available and as foundational machine learning models improve, we expect to see substantial progress in the computational prediction of antibody and VHH structure. Hummer et al. notes that although full in silico antibody design is not yet viable, the foundations have been laid.Citation105 The progress in computational structural prediction not only promises a more comprehensive understanding of antibodies and VHHs but also paves the way for the design of bispecific VHHs by providing crucial insights into the biophysical properties and binding characteristics of individual domains.
Generative machine learning-based approaches to VHH lead identification
Generative machine learning is a class of machine learning that goes beyond predicting values given some input and is capable of generating new data, e.g. sequences or structures, that are distinct from the training data.Citation106 Generative ML approaches for antibodies typically involve the use of deep learning models, such as graph neural networks, diffusion networks, and large language models (LLMs).Citation107 These models are trained on a large dataset of antibody or VHH sequences and/or structural data with the goal of learning the underlying patterns inherent to the molecules. These patterns can then be used to inform the design of novel molecules, with some models capable of co-generating sequence and structure simultaneously.Citation108
While a large set of general protein LLMs (including ProtGPT2,Citation109 ProGen2,Citation110 ESM-2,Citation111 and ProtTrans)Citation112 have been trained on the UniRefCitation113 corpus of protein sequences, the nuances of antibody sequences have stimulated interest in developing antibody-specific LLMs. A burgeoning set of LLMs tuned to the antibody sequence space have been made available (including AntiBERTY,Citation114 AbLang,Citation115 and IgLM,Citation107 []), all drawing from the OAS database that collates antibody sequences and contains nearly 2 billion sequences spanning 68 different studiesCitation9 [as of September 2023]. The models are distinct in the subsets of OAS they trained on and the model architecture (BERT vs. GPT2). Importantly, the models differ in the way they handle different subsets of the antibody space: IgLM is conditional on species (human, camelid, etc.); AbLang has separate models for the heavy and light chain; and AntiBERTy uses a single, unconditional model. nanoBERT was recently developedCitation116 and is even more specialized as it is an LLM trained exclusively on VHH sequences from the Integrated Nanobody Database for Immunoinformatics.Citation53
Table 2. Summary of language models applicable to antibodies and VHHs.
In practice, generative approaches for antibodies have gained the most traction with diversifying sequences to modulate functional properties. To give the reader a sample of interesting applications, we highlight some case studies below. Shin et al. used a deep generative model to develop a 10Citation5 VHH library that illustrated better expression than a 1000-fold larger synthetic library.Citation56 Generative deep learning models applied to the CDRs of preexisting binders were able to produce some antibodies that improved binding over wildtype.Citation114 DiffAb uses antibody-antigen bound structure as input into diffusion probabilistic frameworks and equivariant neural networks to generate novel CDRs that are predicted to preserve target binding (though the manuscript lacks experimental validation).Citation117 Hie et al. apply LLMs to a two-round affinity maturation campaign and see variants with improvement in 7- to 170-fold improvements in affinity.Citation118 Remarkably, Hie et al. noted that general protein LLMs exhibited superior performance compared to antibody-specific LLMs in their case studies. We highlight the need for rigorous evaluation and comparison of the LLMs in the context of the target application. For instance, the application of LLMs to the design of multispecific antibodies is likely to require substantial design constraints to overcome the lack of similar training data (linker regions, multivalent designs, etc.).
Computational approaches to VHH lead optimization
Due to the vast sequence space largely resulting from the sequence and length diversity of CDR3 in the heavy chain, performing an efficient search for the optimal sequence across therapeutically relevant parameters (e.g. affinity, stability, purity) using computational approaches is highly challenging.
Several promising machine learning approaches for antibody sequence optimization include Bayesian optimization (BO), genetic algorithms, reinforcement learning, and active learning.Citation119,Citation120 BO is particularly well suited for navigating the complex design space of antibody and VHH molecules due to its efficient exploration, ability to optimize multiple properties, and flexibility to incorporate diverse input feature spaces.Citation119 By using probabilistic models and making informed decisions about where to sample next, BO effectively balances the trade-off between exploration and exploitation of the design space to converge toward optimal sequences with desirable therapeutic properties. As a first example, AntBO combinatorial BO framework used for the in silico design of CDRH3 that restricts the search to sequences with a favorable developability profile.Citation121 Similarly, Li et al. coupled LLMs for feature representation with BO to navigate a machine learning-derived fitness landscape, leading to the design of high-affinity scFv libraries.Citation122 Finally, LaMBO2 integrates two approaches (NOS for the generation of protein sequences and LaMBO for multiple objective BO with edit-based constraints) to improve antibody expression and binding affinity while maintaining developability.Citation123,Citation124 Collectively, these methods demonstrate that BO is a powerful tool for designing optimized building blocks of single-domain antibodies, which can be subsequently employed in the construction of multi-specific VHHs with enhanced therapeutic properties.
BO offers a promising approach for optimizing multispecific VHHs and antibodies, presenting opportunities not only at the sequence level but also for optimizing additional features of multispecific antibodies and VHHs. These additional features include linker length, linker composition, the number of VHH or antibody units (valency), and the molecule’s topology. By framing the optimization problem in terms of abstract geometric, topological and biological features, rather than as sequence optimization, the search space can be dramatically reduced whilst explicitly focusing design on geometric and biological features directly relevant to identifying multispecific VHHs with the potential for optimal selectivity, efficacy, and developability. Additionally, optimization of the individual parts of the multispecific molecules helps expedite the design, build, test and learn cycle, crucial in enabling swift iterations and the generation of ample data for refining the models and ultimately reducing the time required to identify lead molecules.
Once a lead VHH has been chosen, humanization is often performed to reduce the risk of immunogenicity. While general strategies for VHH humanization have been established,Citation125 several machine learning-based tools, including Hu-Mab,Citation126 Sapiens,Citation127 PLANCitation85 and CUMab,Citation128 have been developed for antibody humanization (). The applicability of these machine learning tools for VHH humanization is in doubt as humanizing hallmark residues in framework region 2 unique to VHHs are generally, but not always, found to have a detrimental effect on properties such as binding affinity, solubility, and purity.Citation37,Citation129,Citation130 Consequently, the machine learning models trained on traditional antibody sequences may not be applicable for VHH humanization. Recently, AbNatiV was developed to assess the degree of humanness and VHH-nativeness of a given sequence. This enables users to fine-tune VHH humanization by ensuring the retention of crucial camelid residues while avoiding especially immunogenic non-human residues.Citation131
Conclusion
VHHs are a promising class of therapeutic molecules due to their size, modularity, and stability. The modular nature of VHHs allows for the creation of bi- and multispecific antibodies with high specificity and affinity. VHHs are also capable of being grafted together in a highly flexible fashion, allowing the ability to explore a vast combinatorial space in a range of highly adaptable formats, a distinct advantage in the multispecific space. Machine learning is already enhancing various stages of the development of therapeutic VHHs by aiding in processes such as lead discovery, lead optimization, and structural prediction. However, it is evident that the application of machine learning in nanobody development lags behind its use in antibody development. Although there is a critical need for the generation of high-quality, domain-specific data to support the analysis of fragment interactions, with this data there exists a notable opportunity for researchers to propel future breakthroughs in VHH-enabled drug discovery by exploring and enhancing machine learning approaches specifically tailored for VHHs.
Abbreviations
Ab | = | Antibody |
ADA | = | Anti-drug antibody |
AML | = | Acute myeloid leukemia |
APC | = | Antigen-presenting cell |
ATUE | = | Antibody understanding evaluation language model |
BiTE | = | Bispecific T-cell engager |
BO | = | Bayesian optimization |
BsAbs | = | Bispecific antibodies |
CAR-T | = | chimeric antigen receptor T-cell |
CDR | = | Complementarity-determining region (1–3) |
CEA | = | Carcinoembryonic antigen |
CH1 | = | Constant heavy domain 1 |
CODV | = | Cross-over dual variable |
CRS | = | Cytokine release syndrome |
Cryo-EM | = | Cryogenic electron microscopy |
EGFR | = | Epidermal growth factor receptor |
EpCAM | = | Epithelial cell adhesion molecule |
Fabs | = | Fragment antigen-binding regions |
Fc domain | = | Fragment crystallizable domain |
FcRn | = | Neonatal Fc receptor |
FW | = | Framework region of an antibody (1–4) |
GANS | = | Generative adversarial networks |
GEPIA2 | = | Gene expression profiling interactive analysis |
HCAbs | = | Heavy chain-only antibodies |
IgG | = | Immunoglobulin G |
IgLM | = | Immunoglobulin language model |
kDa | = | Kilodalton |
LLMs | = | Large language models |
Mab | = | Monoclonal antibody |
MD | = | Molecular dynamics |
ML | = | Machine learning |
NK | = | Natural killer |
NLP | = | Natural language processing |
NOS | = | Diffusion optimized sampling |
OAS | = | Observed antibody space |
PEAR | = | Pre-existing anti-drug reactivity |
PCR | = | Polymerase chain reaction |
PDB | = | Protein data bank |
PD-L1 | = | Programmed cell death ligand 1 |
Pmhc | = | Peptide loaded major histocompatibility complex |
PLM | = | Protein language model |
ROR1 | = | Receptor tyrosine kinase like orphan receptor 1 |
scFv | = | Single-chain variable fragments |
sdAb | = | Single-domain antibody |
VAEs | = | Variational autoencoders |
VHH | = | Variable heavy domain of heavy chain |
VH | = | Variable heavy (domain of an antibody) |
VL | = | Variable light (domain of an antibody) |
TCE | = | T-cell engager |
TCR | = | T-cell receptor |
TriTE | = | Trispecific T-cell engager |
TsAb | = | Tribody format of antibody |
Acknowledgments
We would like to thank members of LabGenius for the scientific discussion of the review. In particular, we would like to thank Lucy Shaw, Tonya Frolov and Leonard Wossnig for detailed comments and careful review of the manuscript.
Disclosure statement
No potential conflict of interest was reported by the author(s).
Additional information
Funding
References
- Labrijn AF, Janmaat ML, Reichert JM, Parren PWHI. Bispecific antibodies: a mechanistic review of the pipeline. Nat Rev Drug Discov. 2019;18(8):585–17. doi:10.1038/s41573-019-0028-1.
- Hamers-Casterman C, Atarhouch T, Muyldermans S, Robinson G, Hammers C, Songa EB, Bendahman N, Hammers R. Naturally occurring antibodies devoid of light chains. Nature. 1993;363(6428):446–48. doi:10.1038/363446a0.
- Barelle C, Porter A. Vnars: an ancient and unique repertoire of molecules that deliver small, soluble, stable and high affinity binders of proteins. Antibod. 2015;4(3):240–58. doi:10.3390/antib4030240.
- Muyldermans S, Atarhouch T, Saldanha J, Barbosa JARG, Hamers R. Sequence and structure of V H domain from naturally occurring camel heavy chain immunoglobulins lacking light chains. Protein Eng Des Sel. 1994;7(9):1129–35. doi:10.1093/protein/7.9.1129.
- Spinelli S, Frenken L, Bourgeois D, De Ron L, Bos W, Verrips T, Anguille C, Cambillau C, Tegonil M. The crystal structure of a llama heavy chain variable domain. Nat Struct Mol Biol. 1996;3(9):752–57. doi:10.1038/nsb0996-752.
- Muyldermans S. Nanobodies: natural single-domain antibodies. Annu Rev Biochem. 2013;82(1):775–97. doi:10.1146/annurev-biochem-063011-092449.
- Li X, Duan X, Yang K, Zhang W, Zhang C, Fu L, Ren Z, Wang C, Wu J, Lu R. et al. Comparative analysis of immune repertoires between bactrian camel’s conventional and heavy-chain antibodies. PloS One. 2016;11(9):e0161801. doi:10.1371/journal.pone.0161801.
- Tu Z, Huang X, Fu J, Hu N, Zheng W, Li Y, Zhang Y. Landscape of variable domain of heavy‐chain‐only antibody repertoire from alpaca. Immunology. 2020;161(1):53–65. doi:10.1111/imm.13224.
- Olsen TH, Boyles F, Deane CM. Observed antibody space: a diverse database of cleaned, annotated, and translated unpaired and paired antibody sequences. Protein Sci. 2022;31(1):141–46. doi:10.1002/pro.4205.
- Gordon GL, Capel HL, Guloglu B, Richardson E, Stafford RL, Deane CM. A comparison of the binding sites of antibodies and single-domain antibodies. Front Immunol. 2023;14:1231623. doi:10.3389/fimmu.2023.1231623.
- Vattekatte AM, Shinada NK, Narwani TJ, Noël F, Bertrand O, Meyniel J-P, Malpertuy A, Gelly J-C, Cadet F, de Brevern AG. Discrete analysis of camelid variable domains: sequences, structures, and in-silico structure prediction. PeerJ. 2020;8:e8408. doi:10.7717/peerj.8408.
- Nguyen VK, Hamers R, Wyns L, Muyldermans S. Camel heavy‐chain antibodies: diverse germline VHH and specific mechanisms enlarge the antigen‐binding repertoire. Embo J. 2000;19(5):921–30. doi:10.1093/emboj/19.5.921.
- Mendoza MN, Jian M, King MT, Brooks CL. Role of a noncanonical disulfide bond in the stability, affinity, and flexibility of a VHH specific for the listeria virulence factor InlB. Protein Sci. 2020;29(4):990–1003. doi:10.1002/pro.3831.
- Jähnichen S, Blanchetot C, Maussang D, Gonzalez-Pajuelo M, Chow KY, Bosch L, Vrieze SD, Serruys B, Ulrichts H, Vandevelde W. et al. CXCR4 nanobodies (VHH-based single variable domains) potently inhibit chemotaxis and HIV-1 replication and mobilize stem cells. Proc Natl Acad Sci U S A. 2010;107(47):20565–70. doi:10.1073/pnas.1012865107.
- Low S, Wu H, Jerath K, Tibolla A, Fogal B, Conrad R, Webb D, MacDougall M, Kerr S, Berger V. et al. VHH antibody targeting the chemokine receptor CX3CR1 inhibits progression of atherosclerosis. Mabs-austin. 2020;12(1):1709322. doi:10.1080/19420862.2019.1709322.
- Ring AM, Manglik A, Kruse AC, Enos MD, Weis WI, Garcia KC, Kobilka BK. Adrenaline-activated structure of β2-adrenoceptor stabilized by an engineered nanobody. Nature. 2013;502(7472):575–79. doi:10.1038/nature12572.
- Kabat EA, Wu TT, Bilofsky H. Unusual distributions of amino acids in complementarity-determining (hypervariable) segments of heavy and light chains of immunoglobulins and their possible roles in specificity of antibody-combining sites. J Biol Chem. 1977;252(19):6609–16. doi:10.1016/S0021-9258(17)39891-5.
- Soler MA, Medagli B, Wang J, Oloketuyi S, Bajc G, Huang H, Fortuna S, de Marco A. Effect of humanizing mutations on the stability of the llama single-domain variable region. Biomolecules. 2021;11(2):163. doi:10.3390/biom11020163.
- Desmyter A, Transue TR, Gharoudi MA, Thi MD, Poortmans F, Hamers R, Muyldermans S, Wyns L. Crystal structure of a camel single-domain VH antibody fragment in complex with lysozyme. Nature Struct Molecul Biol. 1997;3(9):803–11. doi:10.1038/nsb0996-803.
- Bond CJ, Marsters JC, Sidhu SS. Contributions of CDR3 to V H H domain stability and the design of monobody scaffolds for naive antibody libraries. J Mol Biol. 2003;332(3):643–55. doi:10.1016/S0022-2836(03)00967-7.
- Barthelemy PA, Raab H, Appleton BA, Bond CJ, Wu P, Wiesmann C, Sidhu SS. Comprehensive analysis of the factors contributing to the stability and solubility of autonomous human VH domains. J Biol Chem. 2008;283(6):3639–54. doi:10.1074/jbc.M708536200.
- Rouet R, Dudgeon K, Christie M, Langley D, Christ D. Fully human VH single domains that rival the stability and cleft recognition of camelid antibodies. J Biol Chem. 2015;290(19):11905–17. doi:10.1074/jbc.M114.614842.
- Riechmann L, Muyldermans S. Single domain antibodies: comparison of camel VH and camelised human VH domains. J Immunol Methods. 1999;231(1–2):25–38. doi:10.1016/S0022-1759(99)00138-6.
- Tanha J, Nguyen T-D, Ng A, Ryan S, Ni F, MacKenzie R. Improving solubility and refolding efficiency of human VHs by a novel mutational approach. Protein Eng Des Sel. 2006;19(11):503–09. doi:10.1093/protein/gzl037.
- van der Linden RHJ, Frenken LGJ, de Geus B, Harmsen MM, Ruuls RC, Stok W, de Ron L, Wilson S, Davis P, Verrips CT. Comparison of physical chemical properties of llama VHH antibody fragments and mouse monoclonal antibodies. Biochimica et Biophysica Acta (BBA) - Protein Structure and Molecular Enzymology. 1999;1431(1):37–46. doi:10.1016/S0167-4838(99)00030-8.
- Valdés-Tresanco MS, Valdés-Tresanco ME, Molina-Abad E, Moreno E. NbThermo: a new thermostability database for nanobodies. Database. 2023;2023:baad021. doi:10.1093/database/baad021.
- Ewert S, Cambillau C, Conrath K, Plückthun A. Biophysical properties of camelid V HH domains compared to those of human V H 3 domains. Biochem. 2002;41(11):3628–36. doi:10.1021/bi011239a.
- Ladenson RC, Crimmins DL, Landt Y, Ladenson JH. Isolation and characterization of a thermally stable recombinant anti-caffeine heavy-chain antibody fragment. Anal Chem. 2006;78(13):4501–08. doi:10.1021/ac058044j.
- Kunz P, Zinner K, Mücke N, Bartoschik T, Muyldermans S, Hoheisel JD. The structural basis of nanobody unfolding reversibility and thermoresistance. Sci Rep. 2018;8(1):7934. doi:10.1038/s41598-018-26338-z.
- Zimmermann I, Egloff P, Hutter CA, Arnold FM, Stohler P, Bocquet N, Hug MN, Huber S, Siegrist M, Hetemann L. et al. Synthetic single domain antibodies for the conformational trapping of membrane proteins. eLife. 2018;7:e34317. doi:10.7554/eLife.34317.
- Moreno E, Valdés-Tresanco MS, Molina-Zapata A, Sánchez-Ramos O. Structure-based design and construction of a synthetic phage display nanobody library. BMC Res Notes. 2022;15(1):124. doi:10.1186/s13104-022-06001-7.
- Murakami T, Kumachi S, Matsunaga Y, Sato M, Wakabayashi-Nakao K, Masaki H, Yonehara R, Motohashi M, Nemoto N, Tsuchiya M. Construction of a humanized artificial VHH library reproducing structural features of camelid VHHs for therapeutics. Antibod. 2022;11(1):10. doi:10.3390/antib11010010.
- Kinoshita S, Nakakido M, Mori C, Kuroda D, Caaveiro JMM, Tsumoto K. Molecular basis for thermal stability and affinity in a VHH: contribution of the framework region and its influence in the conformation of the CDR3. Protein Sci. 2022;31(11):e4450. doi:10.1002/pro.4450.
- Klarenbeek A, Mazouari KE, Desmyter A, Blanchetot C, Hultberg A, de Jonge JN, Roovers RC, Cambillau C, Spinelli S, Del-Favero J. et al. Camelid Ig V genes reveal significant human homology not seen in therapeutic target genes, providing for a powerful therapeutic antibody platform. Mabs-austin. 2015;7(4):693–706. doi:10.1080/19420862.2015.1046648.
- Cortez-Retamozo V, Backmann N, Senter PD, Wernery U, Baetselier PD, Muyldermans S, Revets H. Efficient cancer therapy with a nanobody-based conjugate. Cancer Res. 2004;64(8):2853–57. doi:10.1158/0008-5472.CAN-03-3935.
- Coppieters K, Dreier T, Silence K, de Haard H, Lauwereys M, Casteels P, Beirnaert E, Jonckheere H, de Wiele CV, Staelens L. et al. Formatted anti–tumor necrosis factor α VHH proteins derived from camelids show superior potency and targeting to inflamed joints in a murine model of collagen-induced arthritis. Arthritis & Rheumatism. 2006;54(6):1856–66. doi:10.1002/art.21827.
- Vincke C, Loris R, Saerens D, Martinez-Rodriguez S, Muyldermans S, Conrath K. General strategy to humanize a camelid single-domain antibody and identification of a universal humanized nanobody scaffold. J Biol Chem. 2009;284(5):3273–84. doi:10.1074/jbc.M806889200.
- Gorovits B, Clements-Egan A, Birchler M, Liang M, Myler H, Peng K, Purushothama S, Rajadhyaksha M, Salazar-Fontana L, Sung C. et al. Pre-existing antibody: biotherapeutic modality-based review. Aaps J. 2016;18(2):311–20. doi:10.1208/s12248-016-9878-1.
- van Schie KA, Wolbink G-J, Rispens T. Cross-reactive and pre-existing antibodies to therapeutic antibodies—effects on treatment and immunogenicity. Mabs-austin. 2015;7(4):662–71. doi:10.1080/19420862.2015.1048411.
- Rossotti MA, Bélanger K, Henry KA, Tanha J. Immunogenicity and humanization of single‐domain antibodies. FEBS J. 2022;289(14):4304–27. doi:10.1111/febs.15809.
- Lin J, Lee SL, Russell AM, Huang RF, Batt MA, Chang SS, Ferrante A, Verdino P, Henry KA. A structure-based engineering approach to abrogate pre-existing antibody binding to biotherapeutics. PloS One. 2021;16(7):e0254944. doi:10.1371/journal.pone.0254944.
- Kim HS, Kim I, Zheng L, Vernes J-M, Meng YG, Spiess C. Evading pre-existing anti-hinge antibody binding by hinge engineering. Mabs-austin. 2016;8(8):1536–47. doi:10.1080/19420862.2016.1219006.
- Cordy JC, Morley PJ, Wright TJ, Birchler MA, Lewis AP, Emmins R, Chen YZ, Powley WM, Bareille PJ, Wilson R. et al. Specificity of human anti‐variable heavy (VH) chain autoantibodies and impact on the design and clinical testing of a VH domain antibody antagonist of tumour necrosis factor‐α receptor 1. Clin Exp Immunol. 2015;182(2):139–48. doi:10.1111/cei.12680.
- Salema V, Fernández LÁ. High yield purification of nanobodies from the periplasm of E. coli as fusions with the maltose binding protein. Protein Express Purificat. 2013;91(1):42–48. doi:10.1016/j.pep.2013.07.001.
- Kariuki CK, Magez S. Improving the yield of recalcitrant nanobodies® by simple modifications to the standard protocol. Protein Expr Purif. 2021;185:105906. doi:10.1016/j.pep.2021.105906.
- Chen SW, Zhang W. Current trends and challenges in the downstream purification of bispecific antibodies. Antibody Ther. 2021;4(2):73–88. doi:10.1093/abt/tbab007.
- Viardot A, Goebeler M-E, Hess G, Neumann S, Pfreundschuh M, Adrian N, Zettl F, Libicher M, Sayehli C, Stieglmaier J. et al. Phase 2 study of the bispecific T-cell engager (BiTE) antibody blinatumomab in relapsed/refractory diffuse large B-cell lymphoma. Blood. 2016;127(11):1410–16. doi:10.1182/blood-2015-06-651380.
- Guo R, Meng X, Wang F, Yu J, Xie Q, Zhao W, Zhu L, Kung HF, Yang Z, Li N. 68Ga-P15-041, a novel bone imaging agent for diagnosis of bone metastases. Front Oncol. 2021;11:766851. doi:10.3389/fonc.2021.766851.
- Huang L, Gainkam LOT, Caveliers V, Vanhove C, Keyaerts M, Baetselier PD, Bossuyt A, Revets H, Lahoutte T. SPECT imaging with 99mTc-labeled EGFR-specific nanobody for in vivo monitoring of EGFR expression. Mol Imaging Biol. 2008;10(3):167–75. doi:10.1007/s11307-008-0133-8.
- Andersen JT, Dalhus B, Viuff D, Ravn BT, Gunnarsen KS, Plumridge A, Bunting K, Antunes F, Williamson R, Athwal S. et al. Extending serum half-life of albumin by engineering neonatal Fc receptor (FcRn) binding*. J Biol Chem. 2014;289(19):13492–502. doi:10.1074/jbc.M114.549832.
- Muyldermans S. A guide to: generation and design of nanobodies. The FEBS J. 2021;288(7):2084–102. doi:10.1111/febs.15515.
- Kovaltsuk A, Leem J, Kelm S, Snowden J, Deane CM, Krawczyk K. Observed antibody space: a resource for data mining next-generation sequencing of antibody repertoires. J Immunol. 2018;201(8):2502–09. doi:10.4049/jimmunol.1800708.
- Deszyński P, Młokosiewicz J, Volanakis A, Jaszczyszyn I, Castellana N, Bonissone S, Ganesan R, Krawczyk K. INDI—integrated nanobody database for immunoinformatics. Nucleic Acids Res. 2022;50(D1):D1273–81. doi:10.1093/nar/gkab1021.
- Abanades B, Olsen TH, Raybould MIJ, Aguilar-Sanjuan B, Wong WK, Georges G, Bujotzek A, Deane CM. The patent and literature antibody database (PLAbDab): an evolving reference set of functionally diverse, literature-annotated antibody sequences and structures. Nucleic Acids Res. 2024;52(D1):D545–51. doi:10.1093/nar/gkad1056.
- Contreras MA, Serrano-Rivero Y, González-Pose A, Salazar-Uribe J, Rubio-Carrasquilla M, Soares-Alves M, Parra NC, Camacho-Casanova F, Sánchez-Ramos O, Moreno E. Design and construction of a synthetic nanobody library: testing its potential with a single selection round strategy. Molecules. 2023;28(9):3708. doi:10.3390/molecules28093708.
- Shin J-E, Riesselman AJ, Kollasch AW, McMahon C, Simon E, Sander C, Manglik A, Kruse AC, Marks DS. Protein design and variant prediction using autoregressive generative models. Nat Commun. 2021;12(1):2403. doi:10.1038/s41467-021-22732-w.
- de Bruin RCG, Veluchamy JP, Lougheed SM, Schneiders FL, Lopez-Lastra S, Lameris R, Stam AG, Sebestyen Z, Kuball J, Molthoff CFM. et al. A bispecific nanobody approach to leverage the potent and widely applicable tumor cytolytic capacity of Vγ9Vδ2-T cells. OncoImmunol. 2018;7(1):e1375641. doi:10.1080/2162402X.2017.1375641.
- Toffoli EC, Sheikhi A, Lameris R, King LA, van Vliet A, Walcheck B, Verheul HMW, Spanholtz J, Tuynman J, de Gruijl TD. et al. Enhancement of NK cell antitumor effector functions using a bispecific single domain antibody targeting CD16 and the epidermal growth factor receptor. Cancers. 2021;13(21):5446. doi:10.3390/cancers13215446.
- Alvarez-Cienfuegos A, Nuñez-Prado N, Compte M, Cuesta AM, Blanco-Toribio A, Harwood SL, Villate M, Merino N, Bonet J, Navarro R. et al. Intramolecular trimerization, a novel strategy for making multispecific antibodies with controlled orientation of the antigen binding domains. Sci Rep. 2016;6(1):28643. doi:10.1038/srep28643.
- Harwood SL, Alvarez-Cienfuegos A, Nuñez-Prado N, Compte M, Hernández-Pérez S, Merino N, Bonet J, Navarro R, En Henegouwen PMPVB, Lykkemark S. et al. ATTACK, a novel bispecific T cell-recruiting antibody with trivalent EGFR binding and monovalent CD3 binding for cancer immunotherapy. Oncoimmunol. 2018;7(1):e1377874. doi:10.1080/2162402X.2017.1377874.
- Mazor Y, Sachsenmeier KF, Yang C, Hansen A, Filderman J, Mulgrew K, Wu H, Dall’acqua WF. Enhanced tumor-targeting selectivity by modulating bispecific antibody binding affinity and format valence. Sci Rep-Uk. 2017;7(1):40098. doi:10.1038/srep40098.
- Dicara DM, Bhakta S, Go MA, Ziai J, Firestein R, Forrest B, Gu C, Leong SR, Lee G, Yu S-F. et al. Development of T-cell engagers selective for cells co-expressing two antigens. Mabs-austin. 2022;14(1):2115213. doi:10.1080/19420862.2022.2115213.
- Tapia-Galisteo A, Rodríguez ÍS, Aguilar-Sopeña O, Harwood SL, Narbona J, Gutierrez MF, Navarro R, Martín-García L, Corbacho C, Compte M. et al. Trispecific T-cell engagers for dual tumor-targeting of colorectal cancer. Oncoimmunol. 2022;11(1):2034355. doi:10.1080/2162402X.2022.2034355.
- Ridgway JBB, Presta LG, Carter P. ‘Knobs-into-holes’ engineering of antibody CH3 domains for heavy chain heterodimerization. Protein Eng Des Sel. 1996;9(7):617–21. doi:10.1093/protein/9.7.617.
- Merchant AM, Zhu Z, Yuan JQ, Goddard A, Adams CW, Presta LG, Carter P. An efficient route to human bispecific IgG. Nat Biotechnol. 1998;16(7):677–81. doi:10.1038/nbt0798-677.
- Liu Z, Leng EC, Gunasekaran K, Pentony M, Shen M, Howard M, Stoops J, Manchulenko K, Razinkov V, Liu H. et al. A novel antibody engineering strategy for making monovalent bispecific heterodimeric IgG antibodies by electrostatic steering mechanism. J Biol Chem. 2015;290(12):7535–62. doi:10.1074/jbc.M114.620260.
- Sargentini-Maier ML, Decker PD, Tersteeg C, Canvin J, Callewaert F, Winter HD. Clinical pharmacology of caplacizumab for the treatment of patients with acquired thrombotic thrombocytopenic purpura. Expert Rev Clin Pharmacol. 2019;12(6):537–45. doi:10.1080/17512433.2019.1607293.
- Takeuchi T, Chino Y, Kawanishi M, Nakanishi M, Watase H, Mano Y, Sato Y, Uchida S, Tanaka Y. Efficacy and pharmacokinetics of ozoralizumab, an anti-TNFα NANOBODY® compound, in patients with rheumatoid arthritis: 52-week results from the OHZORA and NATSUZORA trials. Arthritis Res Ther. 2023;25(1):60. doi:10.1186/s13075-023-03036-4.
- Ortiz S, McEneny A, Amancha P, Rice K, Scholz J, Rakhade S, Yee M. Safety, tolerability, pharmacokinetics, pharmacodynamics and immunogenicity of subcutaneous and intravenous ALXN1720 in healthy volunteers: a phase 1, randomized, double-blind, placebocontrolled, single and multiple ascending dose study (P1-5.016). Neurology. 2023;100(17_supplement_2):100. doi:10.1212/WNL.0000000000203482.
- Svecova D, Lubell MW, Casset-Semanaz F, Mackenzie H, Grenningloh R, Krueger JG. A randomized, double-blind, placebo-controlled phase 1 study of multiple ascending doses of subcutaneous M1095, an anti–interleukin 17A/F nanobody, in moderate-to-severe psoriasis. J Am Acad Dermatol. 2019;81(1):196–203. doi:10.1016/j.jaad.2019.03.056.
- Hofmann I, Baum A, Hofmann MH, Trapani F, Reichel-Voda C, Ehrensperger D, Aichinger M, Ebner F, Budano N, Schweifer N. et al. Pharmacodynamic and antitumor activity of BI 836880, a dual vascular endothelial growth factor and angiopoietin 2 inhibitor, alone and combined with programmed cell death protein-1 inhibition. J Pharmacol Exp Ther. 2023;384(3):331–42. doi:10.1124/jpet.122.001255.
- Tourneau CL, Becker H, Claus R, Elez E, Ricci F, Fritsch R, Silber Y, Hennequin A, Tabernero J, Jayadeva G. et al. Two phase I studies of BI 836880, a vascular endothelial growth factor/angiopoietin-2 inhibitor, administered once every 3 weeks or once weekly in patients with advanced solid tumors. ESMO Open. 2022;7(5):100576. doi:10.1016/j.esmoop.2022.100576.
- Bradley ME, Dombrecht B, Manini J, Willis J, Vlerick D, Taeye SD, den Heede HK, Roobrouck A, Grot E, Kent TC. et al. Potent and efficacious inhibition of CXCR2 signaling by biparatopic nanobodies combining two distinct modes of action. Mol Pharmacol. 2015;87(2):251–62. doi:10.1124/mol.114.094821.
- Ma Y, Xue J, Zhao Y, Zhang Y, Huang Y, Yang Y, Fang W, Guo Y, Li Q, Ge X. et al. Phase I trial of KN046, a novel bispecific antibody targeting PD-L1 and CTLA-4 in patients with advanced solid tumors. J Immunother Cancer. 2023;11(6):e006654. doi:10.1136/jitc-2022-006654.
- Johnson ML, Dy GK, Mamdani H, Dowlati A, Schoenfeld AJ, Pacheco JM, Sanborn RE, Menon SP, Santiago L, Yaron Y. et al. Interim results of an ongoing phase 1/2a study of HPN328, a tri-specific, half-life extended, DLL3-targeting, T-cell engager, in patients with small cell lung cancer and other neuroendocrine cancers. J Clin Oncol. 2022;40(16_suppl):8566–8566. doi:10.1200/JCO.2022.40.16_suppl.8566.
- Molloy ME, Austin RJ, Lemon BD, Aaron WH, Ganti V, Jones A, Jones SD, Strobel KL, Patnaik P, Sexton K. et al. Preclinical characterization of HPN536, a trispecific, T-Cell–activating protein construct for the treatment of mesothelin-expressing solid tumors. Clin Cancer Res. 2021;27(5):1452–62. doi:10.1158/1078-0432.CCR-20-3392.
- Hsieh EM, Rouce RH. Chimeric antigen receptor T cells for mature B-cell lymphoma and Burkitt lymphoma. Hematology. 2020;2020(1):487–93. doi:10.1182/hematology.2020000133.
- Lameris R, Shahine A, Pellicci DG, Uldrich AP, Gras S, Nours JL, Groen RWJ, Vree J, Reddiex SJJ, Quiñones-Parra SM. et al. A single-domain bispecific antibody targeting CD1d and the NKT T-cell receptor induces a potent antitumor response. Nat Cancer. 2020;1(11):1054–65. doi:10.1038/s43018-020-00111-6.
- Kater AP, Donk NWCJVD, Rodríguez-Otero P, Mateos MV, Bosch F, Tucci A, Ghia P, Adang AEP, Parren PWHI, Tuinhof I. et al. Lava-051, a novel bispecific gamma-Delta T-cell engager (Gammabody), in relapsed/refractory MM and CLL: pharmacodynamic and early clinical data. Blood. 2022;140(Supplement 1):4608–09. doi:10.1182/blood-2022-166851.
- Mehra N, Robbrecht D, Voortman J, Parren PW, Macia S, Veeneman J, Umarale S, Winograd B, Vliet HJ, van der Wise DR. Early dose escalation of LAVA-1207, a novel bispecific gamma-delta T-cell engager (gammabody), in patients with metastatic castration-resistant prostate cancer (mCRPC). J Col. 2023;41(6_suppl):153–153. doi:10.1200/JCO.2023.41.6_suppl.153.
- Vallera DA, Felices M, McElmurry R, McCullar V, Zhou X, Schmohl JU, Zhang B, Lenvik AJ, Panoskaltsis-Mortari A, Verneris MR. et al. IL15 trispecific killer engagers (TriKE) make natural killer cells specific to CD33+ targets while also inducing persistence, in vivo expansion, and enhanced function. Clin Cancer Res. 2016;22(14):3440–50. doi:10.1158/1078-0432.CCR-15-2710.
- Felices M, Lenvik TR, Kodal B, Lenvik AJ, Hinderlie P, Bendzick LE, Schirm DK, Kaminski MF, McElmurry RT, Geller MA. et al. Potent cytolytic activity and specific IL15 delivery in a second-generation trispecific killer engager. Cancer Immunol Res. 2020;8(9):1139–49. doi:10.1158/2326-6066.CIR-19-0837.
- Siramshetty VB, Grishagin I, Nguyễn Ð-T, Peryea T, Skovpen Y, Stroganov O, Katzel D, Sheils T, Jadhav A, Mathé EA. et al. NCATS inxight drugs: a comprehensive and curated portal for translational research. Nucleic Acids Res. 2022;50(D1):D1307–16. doi:10.1093/nar/gkab918.
- Abanades B, Wong WK, Boyles F, Georges G, Bujotzek A, Deane CM. ImmuneBuilder: deep-learning models for predicting the structures of immune proteins. Commun Biol. 2023;6:575. doi:10.1038/s42003-023-04927-7.
- Zou H, Yuan R, Lai B, Dou Y, Wei L, Xu J. Antibody humanization via protein language model and neighbor retrieval. bioRxiv. 2023. doi:10.1101/2023.09.04.556278.
- Ruffolo JA, Chu L-S, Mahajan SP, Gray JJ. Fast, accurate antibody structure prediction from deep learning on massive set of natural antibodies. Nat Commun. 2023;14(1):2389. doi:10.1038/s41467-023-38063-x.
- Jumper J, Evans R, Pritzel A, Green T, Figurnov M, Ronneberger O, Tunyasuvunakool K, Bates R, Žídek A, Potapenko A. et al. Highly accurate protein structure prediction with AlphaFold. Nature. 2021;596(7873):583–89. doi:10.1038/s41586-021-03819-2.
- Abanades B, Georges G, Bujotzek A, Deane CM, Xu J. Ablooper: fast accurate antibody CDR loop structure prediction with accuracy estimation. Bioinformatics. 2022;38(7):1877–80. doi:10.1093/bioinformatics/btac016.
- Cohen T, Halfon M, Schneidman-Duhovny D. NanoNet: Rapid and accurate end-to-end nanobody modeling by deep learning. Front Immunol. 2022;13:958584. doi:10.3389/fimmu.2022.958584.
- Senior AW, Evans R, Jumper J, Kirkpatrick J, Sifre L, Green T, Qin C, Žídek A, Nelson AWR, Bridgland A. et al. Improved protein structure prediction using potentials from deep learning. Nature. 2020;577(7792):706–10. doi:10.1038/s41586-019-1923-7.
- Schneider C, Raybould MIJ, Deane CM. SAbDab in the age of biotherapeutics: updates including SAbDab-nano, the nanobody structure tracker. Nucleic Acids Research. 2022;50(D1):D1368–72. doi:10.1093/nar/gkab1050.
- Sankar K, Krystek SR, Carl SM, Day T, Maier JKX. AggScore: prediction of aggregation-prone regions in proteins based on the distribution of surface patches. Struct, Funct Bioinform. 2018;86(11):1147–56. doi:10.1002/prot.25594.
- Myung Y, Pires DEV, Ascher DB, Martelli PL. CSM-AB: graph-based antibody–antigen binding affinity prediction and docking scoring function. Bioinformatics. 2022;38(4):1141–43. doi:10.1093/bioinformatics/btab762.
- Valdés-Tresanco MS, Valdés-Tresanco ME, Jiménez-Gutiérrez DE, Moreno E. Structural modeling of nanobodies: a benchmark of state-of-the-art artificial intelligence programs. Molecules. 2023;28(10):3991. doi:10.3390/molecules28103991.
- Huang C, Huang J, Zhu S, Tang T, Chen Y, Qian F. Multivalent nanobodies with rationally optimized linker and valency for intravitreal VEGF neutralization. Chem Eng Sci. 2023;270:118521. doi:10.1016/j.ces.2023.118521.
- Gaudreault F, Corbeil CR, Sulea T. Enhanced antibody-antigen structure prediction from molecular docking using AlphaFold2. Sci Rep. 2023;13(1):1507. doi:10.1038/s41598-023-42090-5.
- Yin R, Pierce BG. Evaluation of AlphaFold antibody-antigen modeling with implications for improving predictive accuracy. bioRxiv. 2023. doi:10.1101/2023.07.05.547832.
- Liberis E, Veličković P, Sormanni P, Vendruscolo M, Liò P, Hancock J. Parapred: antibody paratope prediction using convolutional and recurrent neural networks. Bioinformat. 2018;34(17):2944–50. doi:10.1093/bioinformatics/bty305.
- Jespersen MC, Mahajan S, Peters B, Nielsen M, Marcatili P. Antibody specific B-cell epitope predictions: leveraging information from antibody-antigen protein complexes. Front Immunol. 2019;10:298. doi:10.3389/fimmu.2019.00298.
- Collatz M, Mock F, Barth E, Hölzer M, Sachse K, Marz M, Lenore C. EpiDope: a deep neural network for linear B-cell epitope prediction. Bioinformat. 2021;37(4):448–55. doi:10.1093/bioinformatics/btaa773.
- McPartlon M, Xu J. Deep learning for flexible and site-specific protein docking and design. bioRxiv. 2023. doi:10.1101/2023.04.01.535079.
- Schneider C, Buchanan A, Taddese B, Deane CM, Valencia A. DLAB: deep learning methods for structure-based virtual screening of antibodies. Bioinformat. 2022;38(2):377–83. doi:10.1093/bioinformatics/btab660.
- Tam C, Kumar A, Zhang KYJ. NbX: machine learning-guided re-ranking of nanobody–antigen binding poses. Pharmaceut. 2021;14(10):968. doi:10.3390/ph14100968.
- Hummer AM, Schneider C, Chinery L, Deane CM. Investigating the volume and diversity of data needed for generalizable antibody-antigen ∆∆G prediction. bioRxiv. 2023. doi:10.1101/2023.05.17.541222v1.
- Hummer AM, Abanades B, Deane CM. Advances in computational structure-based antibody design. Curr Opin Struct Biol. 2022;74:102379. doi:10.1016/j.sbi.2022.102379.
- Martinelli DD. Generative machine learning for de novo drug discovery: a systematic review. Comput Biol Med. 2022;145:105403. doi:10.1016/j.compbiomed.2022.105403.
- Shuai RW, Ruffolo JA, Gray JJ. IgLM: infilling language modeling for antibody sequence design. Cell Syst. 2023;14(11):979–89.e4. doi:10.1016/j.cels.2023.10.001.
- Watson JL, Juergens D, Bennett NR, Trippe BL, Yim J, Eisenach HE, Ahern W, Borst AJ, Ragotte RJ, Milles LF. et al. De Novo design of protein structure and function with RFdiffusion. Nature. 2023;620(7976):1089–100. doi:10.1038/s41586-023-06415-8.
- Ferruz N, Schmidt S, Höcker B. ProtGPT2 is a deep unsupervised language model for protein design. Nat Commun. 2022;13(1):4348. doi:10.1038/s41467-022-32007-7.
- Nijkamp E, Ruffolo JA, Weinstein EN, Naik N, Madani A. ProGen2: exploring the boundaries of protein language models. Cell Syst. 2023;14(11):968–78.e3. doi:10.1016/j.cels.2023.10.002.
- Lin Z, Akin H, Rao R, Hie B, Zhu Z, Lu W, Smetanin N, Verkuil R, Kabeli O, Shmueli Y. et al. Language models of protein sequences at the scale of evolution enable accurate structure prediction. bioRxiv. 2022. doi:10.1101/2022.07.20.500902.
- Elnaggar A, Heinzinger M, Dallago C, Rehawi G, Wang Y, Jones L, Gibbs T, Feher T, Angerer C, Steinegger M. et al. ProtTrans: toward understanding the language of life through self-supervised learning. IEEE Trans Pattern Anal Mach Intell. 2022;44(10):7112–27. doi:10.1109/TPAMI.2021.3095381.
- Suzek BE, Wang Y, Huang H, McGarvey PB, Wu CH. UniRef clusters: a comprehensive and scalable alternative for improving sequence similarity searches. Bioinformat. 2015;31(6):926–32. doi:10.1093/bioinformatics/btu739.
- Ruffolo JA, Gray JJ, Sulam J. Deciphering antibody affinity maturation with language models and weakly supervised learning. arXiv 2021. 2021. doi: 10.1093/2112.07782.
- Olsen TH, Moal IH, Deane CM, Lengauer T. AbLang: an antibody language model for completing antibody sequences. Bioinf Adv. 2022;2(1):vbac046. doi:10.1093/bioadv/vbac046.
- Hadsund JT, Satława T, Janusz B, Shan L, Zhou L, Röttger R, Krawczyk K. nanoBERT: a deep learning model for gene agnostic navigation of the nanobody mutational space. bioRxiv. 2024. doi:10.1101/2024.01.31.578143.
- Luo S, Su Y, Peng X, Wang S, Peng J, Ma J. Antigen-specific antibody design and optimization with diffusion-based generative models for protein structures. bioRxiv. 2022. doi:10.1101/2022.07.10.499510.
- Hie BL, Shanker VR, Xu D, Bruun TUJ, Weidenbacher PA, Tang S, Wu W, Pak JE, Kim PS. Efficient evolution of human antibodies from general protein language models. Nat Biotechnol. 2024;42(2):275–83. doi:10.1038/s41587-023-01763-2.
- Bellamy H, Rehim AA, Orhobor OI, King R. Batched Bayesian optimization for drug design in noisy environments. J Chem Inf Model. 2022;62(17):3970–81. doi:10.1021/acs.jcim.2c00602.
- Seo S, Kwak MW, Kang E, Kim C, Park E, Kang TH, Kim J. Accelerating antibody design with active learning. bioRxiv. 2022. doi:10.1101/2022.09.12.507690.
- Khan A, Cowen-Rivers AI, Grosnit A, Deik D-G-X, Robert PA, Greiff V, Smorodina E, Rawat P, Akbar R, Dreczkowski K. et al. Toward real-world automated antibody design with combinatorial Bayesian optimization. Cell Rep Methods. 2023;3(1):100374. doi:10.1016/j.crmeth.2022.100374.
- Li L, Gupta E, Spaeth J, Shing L, Jaimes R, Engelhart E, Lopez R, Caceres RS, Bepler T, Walsh ME. Machine learning optimization of candidate antibody yields highly diverse sub-nanomolar affinity antibody libraries. Nat Commun. 2023;14(1):3454. doi:10.1038/s41467-023-39022-2.
- Stanton S, Maddox W, Gruver N, Maffettone P, Delaney E, Greenside P, Wilson AG. Accelerating Bayesian optimization for biological sequence design with denoising autoencoders. Int Conf Mach Learn. 2022;20459–20478.
- Gruver N, Stanton S, Frey NC, Rudner TGJ, Hotzel I, Lafrance-Vanasse J, Rajpal A, Cho K, Wilson AG. Protein design with guided discrete diffusion. 2023. arXiv 2023. doi:10.1093/2305.20009.
- Sulea T. Humanization of camelid single-domain antibodies. Methods Mol Bio. 2022;2446:299–312.
- Marks C, Hummer AM, Chin M, Deane CM, Martelli PL. Humanization of antibodies using a machine learning approach on large-scale repertoire data. Bioinformat. 2021;37(22):4041–47. doi:10.1093/bioinformatics/btab434.
- Prihoda D, Maamary J, Waight A, Juan V, Fayadat-Dilman L, Svozil D, Bitton DA. BioPhi: A platform for antibody design, humanization, and humanness evaluation based on natural antibody repertoires and deep learning. Mabs-austin. 2022;14(1):2020203. doi:10.1080/19420862.2021.2020203.
- Tennenhouse A, Khmelnitsky L, Khalaila R, Yeshaya N, Noronha A, Lindzen M, Makowski EK, Zaretsky I, Sirkis YF, Galon-Wolfenson Y. et al. Computational optimization of antibody humanness and stability by systematic energy-based ranking. Nat Biomed Eng. 2023;8(1):30–44. doi:10.1038/s41551-023-01079-1.
- Conrath K, Vincke C, Stijlemans B, Schymkowitz J, Decanniere K, Wyns L, Muyldermans S, Loris R. Antigen binding and solubility effects upon the veneering of a camel VHH in framework-2 to mimic a VH. J Mol Biol. 2005;350(1):112–25. doi:10.1016/j.jmb.2005.04.050.
- Abderrazek RB, Vincke C, Hmila I, Saerens D, Abidi N, Ayeb ME, Muyldermans S, Bouhaouala-Zahar B. Development of Cys38 knock-out and humanized version of NbAahII10 nanobody with improved neutralization of aahII scorpion toxin. Protein Eng Des Sel. 2011;24(9):727–35. doi:10.1093/protein/gzr037.
- Ramon A, Saturnino A, Didi K, Greenig M, Sormanni P, Visentin C, Ricagno S, Xu X, Greenig M, Sormanni P. Assessing antibody and nanobody nativeness for hit selection and humanization with AbNatiV. Nat Mach Intell. 2024;6(1):74–91. doi:10.1038/s42256-023-00778-3.