ABSTRACT
The Pan-Eurasian Experiment Modelling Platform (PEEX-MP) is one of the key blocks of the PEEX Research Programme. The PEEX MP has more than 30 models and is directed towards seamless environmental prediction. The main focus area is the Arctic-boreal regions and China. The models used in PEEX-MP cover several main components of the Earth’s system, such as the atmosphere, hydrosphere, pedosphere and biosphere, and resolve the physical-chemical-biological processes at different spatial and temporal scales and resolutions. This paper introduces and discusses PEEX MP multi-scale modelling concept for the Earth system, online integrated, forward/inverse, and socioeconomical modelling, and other approaches with a particular focus on applications in the PEEX geographical domain. The employed high-performance computing facilities, capabilities, and PEEX dataflow for modelling results are described. Several virtual research platforms (PEEX-View, Virtual Research Environment, Web-based Atlas) for handling PEEX modelling and observational results are introduced. The overall approach allows us to understand better physical-chemical-biological processes, Earth’s system interactions and feedbacks and to provide valuable information for assessment studies on evaluating risks, impact, consequences, etc. for population, environment and climate in the PEEX domain. This work was also one of the last projects of Prof. Sergej Zilitinkevich, who passed away on 15 February 2021. Since the finalization took time, the paper was actually submitted in 2023 and we could not argue that the final paper text was agreed with him.
1. Introduction
The Pan-Eurasian EXperiment (PEEX; https://www.atm.helsinki.fi/peex) is a multidisciplinary, multi-scale research programme aimed at resolving the major uncertainties in Earth system science and global sustainability issues concerning the Arctic and boreal Northern Eurasian regions and China (Kulmala et al., Citation2016; Kulmala et al., Citation2015; Lappalainen et al., Citation2018; Lappalainen et al., Citation2022) contexts. PEEX started in 2012 and is motivated by the well-known fact that the land, atmosphere and ocean areas located in Eurasian regions north of 45°N latitude have undergone rapid warming compared to global mean temperature increases in recent decades (IPCC, Citation2014; Rantanen et al., Citation2022) and are predicted to be subject to substantial changes over the coming decades. The Arctic-boreal natural environment is set to play a major role in global climate change via changes in surface albedo, changes in carbon sinks and emissions, methane emissions and aerosol production via biogenic volatile organic compounds (BVOCs) (PEEX, Citation2015).
Global climate models do reproduce and project this accelerated and amplified warming in the northern high latitudes. At the same time, the models still diverge on magnitude, physical connections, geographical patterns, and not least on future implications of this amplified warming (Cohen et al., Citation2020; Previdi et al., Citation2021; Taylor et al., Citation2022). Moreover, satellite observations clearly indicate that physical process at smaller scales compared with larger scales has different influence on the regional changes (Duncan et al., Citation2020; Esau et al., Citation2023; Goosse et al., Citation2018). One of the most prominent examples is given by changes in frozen soils and permafrost (Smith et al., Citation2022). Multi-scale integrated model framework is needed to resolve this disconnection between small-scale climate processes and their persistent large-scale climate impact.
PEEX program develops and utilizes an integrated observational and modelling framework to identify and quantify different climate forcing and feedback mechanisms in the northern high-latitude Earth system, and therefore, enable more reliable predictions of future regional and global climate and resulting impacts on humans and ecosystems (Lappalainen et al., Citation2016). Efficient climate feedback processes drive rapid warming at high latitudes. One such example is the ice-albedo feedback (IPCC, Citation2014); however, there are other local feedbacks, and non-local climate system feedbacks that may be important for high latitude temperature response, which are much less well understood (e.g. Arneth et al., Citation2010; Scott et al., Citation2018). An increasing body of work demonstrates important linkages between rapid changes in the Arctic system and changes in mid-latitude weather and climate variability. Sea-ice loss in the Arctic results in anomalies in mid-latitude weather and circulation (Overland et al., Citation2015). Responses in mid-latitude circulation to Arctic changes have been implicated in degrading air quality in China (Zou et al., Citation2017). Because of the already observable effects of climate change on society, and the specific role of the Arctic and boreal regions in this context, PEEX emphasizes the need to establish next-generation research and Research Infrastructures (RIs) in this area, and in particular, for ground-based observations to already existing stations (Alekseychik et al., Citation2016; Petäjä et al., Citation2021). Moreover, a network of state-of-the-art SMEAR (Station for Measuring Ecosystem-Atmosphere Relations) stations is needed, including establishing sites in the PEEX regions of interest (Kulmala, Citation2018). This programme’s geographical domain covers the Eurasian boreal zone and Arctic regions of the Northern Hemisphere, including marine areas such as the Baltic, the North and the Arctic Ocean seas (Vihma et al., Citation2019). Rapid urbanization, environmental and climate changes are all making people, organisations and businesses more vulnerable to meteorological and environmental conditions and hazards. Hence, it requires detailed knowledge of climate, weather, and air-water-soil quality (Zilitinkevich et al., Citation2015). PEEX is committed to advancing the Earth system science agenda and influencing climate policy on issues critical and important to the Pan-Eurasian environment. Additionally, it strives to assist the societies of this region fostering a sustainable future.
The programme agenda is divided into four focus areas: Research agenda, Infrastructures, Impact on society and Knowledge transfer (), and it is built on research collaboration between different Eurasian parties. The Research Infrastructure (RI) comprises state-of-the-art in-situ and satellite observation and data systems in the Pan-Eurasian region and modelling platform. It presents a framework for future coherent and coordinated research infrastructures in the PEEX domain.
The aim of this paper is to introduce and discuss the PEEX-Modelling-Platform (PEEX-MP) multi-scale concept (Section 2); seamless modelling approaches (Earth system, online integrated, process-based, socioeconomic), high-performance computing and data infrastructure (Section 3); and virtual research platforms (Section 4). We are motivated by the opportunities of the seamless multi-scale and -processes modelling. For modelling and its applications, “seamless” refers to processes and systems which simultaneously, continuously, and efficiently interact without interruptions. Seamless modelling allows to better understand physical-chemical-biological processes, Earth’s system interactions and feedbacks and to provide valuable information for assessment studies on evaluation of risks, impact, consequences, etc. for population, environment and climate in the Arctic-boreal regions and China.
2. Multi-scale modelling concept
As the societal impacts of hazardous/unfavourable weather and other environmental pressures, especially under changing climate conditions, grow, there is a need for integrated predictions that can reliably represent the numerous feedbacks and linkages between sub-systems of our environment. This has led to the development of a new generation of high-resolution coupled prediction tools to represent the two-way interactions between different components of the environment. For example, a new generation of online integrated Atmospheric Chemical Transport (ACT) and Meteorology (Numerical Weather Prediction, NWP and Climate) models represent the interactions between different atmospheric processes, including chemistry (both gases and aerosols), clouds, radiation, boundary layer, emissions, meteorology and climate (Baklanov et al., Citation2017; Baklanov et al., Citation2014). For the ocean system, a seamless earth system approach has been adopted by EuroGOOS for implementing EuroGOOS scientific strategy and a roadmap for the implementation has been under development (She et al., Citation2021). Seamless models for resolving hydrodynamics and pollutant transport nutrient in land-to-ocean aquatic continuum has been developed by PEEX partners in national, regional and pan-European scales (She & Murawski, Citation2018; She et al., Citation2023). In parallel, coupled environmental prediction at km-scale, which includes feedback between the atmosphere, land surface, coastal areas and oceans, aims to better represent interactions in the water cycle and provide tools for improved natural hazard response or water management, for example. Global Earth system models simulate the atmosphere, cryosphere, biosphere, and oceans, allowing investigation of interactions and feedback within and between these spheres, including how these affect climate and biogeochemistry on timescales of hours to millennia.
Different aspects of integrated model development, evaluation and understanding are considered within the PEEX Modelling Platform (PEEX-MP). The Platform outlines a strategy for the best and optimal use of current generation modelling tools to improve process understanding and predictability across varying timescales within the PEEX domain. It also sheds a light and presents potential for future developments and advancements. Several application areas of new integrated modelling developments are expected to be considered, including:
improved NWP and chemical weather forecasting (CWF) with short-term feedback of aerosols and chemistry on meteorological variables;
two-way interactions between atmospheric composition and climate variability and change;
better prediction of atmosphere and/or ocean state through closer coupling between the component models to represent the two-way feedback and exchange of the atmospheric and ocean boundary layer properties;
more complete detailed simulation of the hydrological cycle through linking atmospheric, land surface, ecosystems, hydrological and ocean circulation models;
improved simulation of the biosphere and C-cycle response to high-latitude environmental change.
The PEEX-MP focuses on a new generation of integrated models and is based on the seamless Earth System Modelling (ESM) approach (WWRP, Citation2015) to evolve from separate model components to seamless meteorology-composition-environment models to address challenges in weather, climate and atmospheric composition fields whose interests, applications and challenges are now overlapping. The highly coupled nature of the atmosphere-land-ice-ocean system at high latitudes and the rapid nature of ongoing changes within and of interactions and feedback between these components means that a highly coupled system capable of considering non-linear interactions across various timescales is highly desirable. “Seamless” is considered with at least two aspects. Firstly, at the physical, chemical, biological, or other processes scale. For example, the coupling within a model of meteorology and atmospheric composition processes to represent the two-way interactions between chemical species and aerosols with radiative processes, or with cloud microphysics, or with the consistent water phases transition such as phenomena of melting, freezing, evaporation, condensation, sublimation, and deposition. As for chemical processes, there are chemical reactions and transformations between gases, aerosols and water in the air and below- and in-the-clouds. Secondly, to be considered in terms of time-space-scales, it refers to the absence of discontinuities in model behaviour when used at multiple temporal or spatial resolutions to have, for example, consistent treatment of black carbon for air quality and climate applications or consistent coupling interval between land, ocean and atmosphere.
In a more general sense, the seamless approach considers several dimensions of the integration and coupling (Baklanov & Zhang, Citation2020), including (see ):
Figure 2. Seamless-coupling approach: multi-dimensions (tools, scales, components and processes) and linking to services and end-users through evaluation of impacts and consequences and assessments.

Time scales: from seconds and nowcasting to decadal and centennial (climate) time-scale;
Spatial scales: from street-level to global scale (downscaling and upscaling);
Processes: physical, chemical, biological, social;
Earth system elements/environments/components: atmosphere, hydrosphere, lithosphere/pedosphere, ecosystems/biosphere;
Different types of observations and modelling as tools: observations-model fusion, data processing and assimilation, validation and verification;
Links with health and social consequences, impact, assessment, services, and end-users.
Some aspects of methodology and research needed to realise the Seamless Prediction Systems are presented and discussed in the book “Seamless Prediction of the Earth System: From Minutes to Months” (WWRP, Citation2015).
The PEEX-MP is characterized by a complex integrated Earth System Modelling (ESM) approach, combined with specific models of different processes and system elements, acting on different temporal and spatial scales. The ensemble approach is more preferable because it allows to integrate results from different models developed by PEEX partners from different countries. Selection of specific models as members of an ensemble depends on formulated scientific problem, physical processes to be considered, selected geographical area of PEEX domain, and application focus. This allows information on model process diversity to inform uncertainty in our understanding of Earth system linkages, responses and impact assessments. PEEX can utilize, depending on mentioned above points, a hierarchy of available models: scenario analysis, forward and inverse modelling, and tailored modelling based on measurement needs and processes. The models are evaluated and constrained by available in-situ and remote sensing data of various spatial and temporal scales using data assimilation and top-down modelling. The analyses of the anticipated large volumes of data produced by available models (listed in Appendix A1) and sensors will be supported by a dedicated virtual research platform (see Section 4) developed for these purposes.
Linking between the temporal and spatial scales for modelling and observations within the PEEX domain is a key aspect of the PEEX-MP. Modelling in a downscaling chain from global- to regional-, and subregional-, urban-scales, covering processes from climate- to synoptic- and meso-, micro- scales, allows seamless interaction of physical and chemical processes. In particular, some regional models have similar physical and chemical processes as global models and linked in a seamless way. Both short- and long-term measurements from different observational platforms, including field campaigns, laboratory, chamber experiments, satellite, etc., represent valuable contributions to the evaluation and verification of the different models as well as for studying and modelling of physical-chemical-biological processes at multi-scales and integrated assessment for environment, population, ecosystems of the PEEX region. As part of the PEEX initiative, a hierarchy/framework of modern multi-scale models for different elements of the Earth’s system, integrated with the observation system, is needed. This will support the PEEX observational system and help answer the PEEX scientific questions (Kulmala et al., Citation2015). Moreover, the models will inform the development of the in-situ monitoring component of the PEEX Research Infrastructure by providing information on regions where specific processes or interactions may be important to measure with new observational capability.
Results of Earth System Modelling are among key topics in the international debate on land–atmosphere interactions in relation to global change. The important question is to what extent the ESM components are well represent the Earth’s systems. The ESMs consist of equations describing processes in the atmosphere, ocean, cryosphere, and in the terrestrial and marine biospheres. ESMs are the best tools for analysing the effect of different environmental changes on future climate or for studying the role of different processes in the entire Earth system. These types of analyses and predictions of future change are especially important in the high northern latitudes, where climate change is proceeding the fastest and near-surface warming has been about twice the global average in recent decades.
The processes, and hence, the parameterizations included in ESMs are still based on insufficient knowledge of the physical, chemical, and biological mechanisms involved in the climate system. Also, the spatial or temporal resolution of known processes in the models is often insufficient. Global-scale modelling of land-atmosphere-ocean interactions using ESMs provides a way to explore the influence of spatial and temporal variations in the activities of land systems, and on the climate. However, methods for using new process understanding to improve model process parameterization effectively in ESMs, and subsequently linking this to the decision-making process, are lacking.
The Northern Hemisphere has more potential sources of emissions than the Southern Hemisphere. And in particular, the middle and northern latitudes, including boreal and arctic regions, play a significant role as a source of natural and anthropogenic greenhouse gases, aerosols and other emissions in the Earth system. Due to dominating atmospheric transport, dispersion and deposition, these are spatially distributed mostly in the Northern Hemisphere. An example (snapshot) of the spatial distribution of cloud condensation nuclei is shown in . In addition, changes in radiatively active gases and aerosols at lower latitudes can result in changes to high latitude climate through radiative forcing at lower latitudes and resultant changes in poleward heat transport (e.g. see Sand et al. (Citation2016)).
Figure 3. Examples (snapshots) of modelling results: (a) EC-Earth – cloud condensation nuclei, CCN spatial distribution in the Northern Hemisphere (note relatively lower CCN in the Arctic regions, dominating lower values over ocean/sea areas compared to land); and (b) CESM/ocean-ice – surface speed in the eddy-resolving version (note fine-scale filaments along the Norwegian and Siberian coast, indicative of the turbulent mixing processes that bring the fresh estuary waters into the interior ocean).

In the PEEX domain, the Arctic Ocean water-mass properties remain challenging to simulate in global circulation models (Ilıcak et al., Citation2016). This poses a problem in predicting the future of the Arctic region. Model challenges arise early in the Nordic Seas (which connect to the Arctic Ocean through the two important pathways Fram Strait and the Barents Sea), where models fail to reproduce the Norwegian Current satisfactorily, a consequence of the way meso-scale eddies are parameterized in coarse resolution GCMs. This results in the distribution of temperature and salt being poorly represented in the Arctic. Another problem stems from the sparse number of observations of the Arctic Ocean. In particular, the Siberian shelves have been observed very little due to accessibility. The shelves make out a large part of the Arctic Ocean area (Nikiforov & Shpaikher, Citation1980) and are important in the production of dense Arctic water masses (Aagaard et al., Citation1981), thus constituting an important but poorly understood region of the Arctic.
Numerical models of high spatial resolution (0.1 degree or finer) can aid in understanding the important processes in the Arctic. In particular, the inflow of the warm Atlantic layer water into the Arctic, and the cross-shelf mixing processes that bring cold, fresh estuary waters into the open ocean, are represented with high fidelity and can therefore be used to identify key climate-relevant processes and determine how well they are represented in current earth system models. By filling the gaps in observations, they can provide new knowledge of shelf processes, the formation of dense and halocline water on the shelves, and how these are distributed in the Arctic interior through, e.g. turbulent fluxes (Ivanov & Golovin, Citation2007). In addition, the high resolution (0.1 degrees) eddy-resolving model output can shed light on the turbulent Norwegian Current and its important heat and salt transport to the Arctic (see and, on larger time scales, improve current parameterizations in coarser resolution climate models used for future predictions (such as those used in, e.g. IPCC, Citation2014), which form a key role in decision-making with regard to climate and environmental politics. Thus, the high-resolution models can contribute greatly to our understanding of the Arctic Ocean, which is crucial to the Arctic-boreal regions of the PEEX domain and local and global climate and environment.
A network of monitoring stations that can quantify the interactions between neighbouring areas (ranging from the Arctic and the Mediterranean to the Chinese industrial areas and the Asian steppes) is needed. For example, in addition to the development of various types of stations in the PEEX Arctic-Boreal area, strong cooperation with surrounding research infrastructures needs to be established along the lines of the WMO GAW (Global Atmosphere Watch; https://community.wmo.int/en/activity-areas/gaw) observation system and European ICOS (Integrated Carbon Observation System; https://www.icos-cp.eu) and ACTRIS (Aerosol, Clouds, and Trace gases Research InfraStructure Network; http://www.actris.net) networks. This allows PEEX to obtain a global perspective on emissions transport and the transformation and ageing of pollutants entering and exiting the PEEX area.
The concept also includes elaboration of services for end-users. Services can be used for studies on impact, assessment, consequences, risks, etc. for environment and population. In particular, the creation of virtual research platforms for archiving is required to meet challenges related to the growing volumes of global and PEEX-domain environmental data. This enables researchers to process structured and qualitative data in virtual workspaces. Such platforms should integrate data, networks and computing resources, allowing the interdisciplinary research community to understand ongoing and possible future climatic, environmental and other changes and their consequences for the targeted regions.
There are many urgent scientific questions which could be addressed in the frameworks of the proposed concept and employing PEEX-MP models, components, and infrastructure (see Section 3). These questions address the northern latitude regions, and especially the Arctic territories. These regions are expected to experience temperature changes higher than the global mean while being large enough to feedback to regional and global climate systems. For now, our understanding of the relevant physical processes is hampered by a lack of concurrent measurements of aerosols, clouds, radiation, snow, and sea-ice processes. Measurements and accurate estimates of BVOC emissions are required. Improved understanding will require a better description of BVOC emission responses to air chemistry-related impacts (such as ozone induction, secondary organic aerosol formation, nitrogen dependency, and sulphur dioxide effects) considering both climatic conditions and phenology and physiology of plants. This will also necessitate a more precise quantification of air pollutant deposition into vegetation, considering differences between stomatal- and non-stomatal deposition pathways. Due to observing and expected changes in temperature and precipitation patterns, the carbon-cycle responses and deposition of pollutants such as ozone are important to investigate, especially on how these interactions will affect the distribution of vegetation, emissions of BVOCs, and resultant feedback. Current and expected future effects of biomass burning, forest fires, and local pollution emissions (including shipping) on radiative forcing and atmospheric composition in the region should also be evaluated (see Arnold et al., Citation2016).
3. Models, components, infrastructure
Currently, the PEEX-Modelling-Platform (PEEX-MP) includes more than 30 different models (see ) with more than 100 members of the PEEX-MP network. The presented models include different Earth system components (shown in ) such as atmosphere-hydrosphere-pedosphere-biosphere as well as cover different processes such as physical-chemical-biological. A complete list of these models and selected key references are available in Appendix A1. More details (including also information on contact persons; possible modes of the model runs – research, operational, semi-operational; brief model descriptions with an extended list of references) about each PEEX-MP model are available at: https://www.atm.helsinki.fi/peex/index.php/portfolio-items/modelling-platform. Among these models, there are those which are employed for realization of the PEEX research agenda, and in particular, there are the Earth System Models (such EC-Earth, CESM, MPI-ESM, HadGEM2-ES) coupled with different components such as atmosphere-ocean-sea/ice-etc.; online integrated meteorology-chemistry-aerosols multi-scale modelling systems (Enviro-HIRLAM, WRF-Chem) providing simultaneously meteorological and atmospheric composition fields; multi-scale atmospheric chemical transport models (SILAM, EurCTM, CAM-Chem, TOMCAT-GLOMAP, GEOS-Chem, ATMES, MMAD&IT, FLEXPART) with different complexity of description of physical-chemical processes, gas-phase/aerosol chemistry and meteorological input required to drive the simulations; ocean-sea-ice-wave modelling systems (HYCOM-CICE, HBM, WAM, SWAN); models supporting research on atmospheric processes at different scales (GLOBO, BOLAM, MOLOCH); models for studying atmosphere-vegetation-ecosystems physical-chemical/aerosol processes and interactions taking into account various types of emissions (AVIM2, Agro-C, CH4MOD, SIM-BIM, EMBE, EMSR, EMGPP, DNDC-HONO, CNMM-DNDC, SOSAA), large eddy simulation models (PALM, UCLALES-SALSA); tools for inverse modelling for emissions of pollutants including greenhouse gases (FLEXPART, CTDAS, IMDAF); and even tools (such as DERMA, FLEXPART) for emergency response on nuclear-biological-chemical danger in case of accidental releases.
Figure 4. The components (atmosphere – for air, hydrosphere – for water, pedosphere – for soil, biosphere – for ecosystems) and processes of the models being a part of the PEEX-Modelling-Platform (PEEX-MP).

In a hierarchy of above-mentioned models, the ESMs runs cover spatially entire globe and simulate required input for finer resolution runs of other models covering hemispheric, regional, sub-regional scales. Subsequently, these models’ runs provide input for urban, local, and street scales runs by models at micro-scale resolution. Functionally, the larger scale output can be used or interconnected as input from outer into nested smaller size domains. Moreover, each of the models can be used depending on specific application in the PEEX domain and can be run stand-alone.
3.1. Earth system modelling
In the PEEX-MP, the EC-Earth model is used for the global scale. This model is a recent Earth System Model developed jointly by almost 30 European research institutes (Döscher et al., Citation2022; Hazeleger et al., Citation2010; Hazeleger et al., Citation2012). It comprises atmosphere model IFS (Integrated Forecasting System; with detailed documentation available at https://www.ecmwf.int), ocean model NEMO (Nucleus for European Modelling of the Ocean; the NEMO team, Madec & the NEMO team, Citation2008) and vegetation model LPJ-GUESS (Lund-Potsdam-Jena General Ecosystem Simulator; Smith et al., Citation2001), coupled with OASIS (Ocean Atmosphere Sea Ice Soil; Redler et al., Citation2010) coupler (). Aerosols and chemistry are included with TM5 (Transport Model 5; Huijnen et al., Citation2010) model. Aerosols are represented with seven log-normal modes, while chemistry is covered with 51 prognostic species and 156 chemical reactions. The EC-Earth participated in the Coupled Model Intercomparison Project (CMIP6), supporting the IPCC Sixth Assessment Report (AR6).
Figure 5. Schematics of the EC-Earth model components: TM5 (aerosols and chemistry), NEMO (ocean), IFS (atmosphere), LPJ-GUESS (vegetation); the submodels are connected via the OASIS coupler.
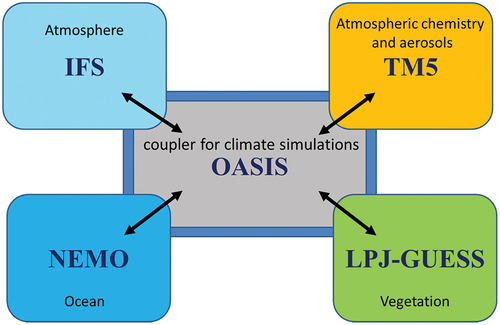
The IFS is the atmospheric model developed at the European Centre for Medium-Range Weather Forecasts (ECMWF, UK). The IFS is coupled to the ocean model NEMO, which is run with 1º horizontal resolution and 42 vertical levels. The ice model LIM (The Louvain-la-Neuve Sea Ice Model; Rousset et al., Citation2015; Vancoppenolle et al., Citation2009) is coupled directly to the ocean model.
For EC-Earth simulations (van Noije et al., Citation2021; van Noije et al., Citation2014), the TM5 model describes aerosols using a 7-mode size distribution (Vignati et al., Citation2004), with four soluble and three insoluble modes. It includes the most abundant aerosol species: sulphate, black carbon, organic carbon, sea salt and mineral dust. Several modifications are included for the current CMIP6 version of EC-Earth ESM: e.g. TM5 aerosol fields are communicated via OASIS to IFS for aerosol indirect (cloud) effect and secondary organic aerosol schemes were implemented (Bergman et al., Citation2022). The model uses a grid of 3º×2º for aerosols and chemistry.
The LPJ-GUESS (Smith et al., Citation2001) is the terrestrial vegetation component of EC-Earth. It is a dynamic process-based ecosystem model which can predict structural and compositional ecosystem properties. In EC-Earth, the atmospheric model IFS communicates temperature, radiation, precipitation and soil state to LPJ-GUESS. Concurrently, LPJ-GUESS updates simulated low and high vegetation fractions and leaf area index (LAI) to the IFS land surface module. In addition to land surface processes, gas-phase transport of CO2, aerosols and precursors is communicated between TM5 and LPJ-GUESS. The CMIP6-version of EC-Earth includes version 4 of LPJ-GUESS, together with, e.g. a new land albedo parameterization and fire module. LPJ-GUESS is being updated with speciated monoterpene emissions for detailed SOA formation in the atmosphere.
Another ESM model, the Community Earth System Model (CESM), is a fully coupled, global climate model that provides state-of-the-art computer simulations of the Earth’s past, present, and future climate states. It is developed by the National Center for Atmospheric Research (NCAR, USA) and includes several sub-models, such as the Community Atmospheric Model (CAM5), Community Land Model (CLM4.5), Community Ice CodE (CICE) and Parallel Ocean Program (POP2). Each CESM model component is fully customizable concerning selecting various parameterizations, grid resolutions and input datasets.
The globally online-coupled CAM5 model offers various dynamical cores, including Eulerian, semi-Lagrangian, spectral element, and finite volume, across a wide spectrum of horizontal and vertical resolutions with time stepping (Neale et al., Citation2012). The model includes state-of-the-art parameterizations of atmospheric physics, such as moist turbulence, shallow and deep convection schemes, and an updated cloud microphysics module (Gettelman et al., Citation2008; Morrison & Gettelman, Citation2008). Aerosol microphysics is modeled using either the 3-mode or 7-mode modal aerosol scheme (MAM-3 and MAM-7, Liu & Ghan, Citation2010) with an expanded representation of the secondary-organic aerosols. The MAM-3 scheme is also coupled with tropospheric and stratospheric gas-phase chemistry mechanisms (Neale et al., Citation2012).
The POP2 global ocean model has a finite volume dynamical core with two displaced-pole grids centred in Greenland, at nominal 1° and 3° horizontal resolution and 62 vertical levels. It also supports a high-resolution tri-pole grid at a nominal 0.1° horizontal resolution in which two poles are placed opposite each other in landmasses near the North Pole to provide more uniform grid spacing in the Arctic Ocean (Small et al., Citation2014). The POP model was one of the first to introduce the local boundary condition for the surface pressure at a land–ocean interface point, which eliminates the non-local line-integral and replaces “rigid-lid” boundary condition by an implicit free-surface boundary condition that allows the air–sea interface to evolve freely and makes sea-surface height a prognostic variable. There are several parameterizations for vertical mixing and convection, including the KPP mixing scheme (Large et al., Citation1994) as well as horizontal mixing parameterizations such as the Gent-McWilliams isopycnal tracer diffusion scheme (Gent & McWilliams, Citation1990). The POP2 model contains the Biogeochemical Elemental Cycling (BEC) module for ecosystem and biogeochemistry simulation with multiple phytoplankton functional groups and multiple potentially growth-limiting nutrients (Moore et al., Citation2002; Moore et al., Citation2004). The BEC module is coupled with a carbonate chemistry module (Doney et al., Citation2009), allowing dynamic computation of surface ocean pCO2 and air-sea CO2 flux exchange.
The CICE (v5.1.2) model provides information on sea-ice distribution, area fraction, ice volume, and snow volume. The model is supported on the same high- and low-resolution Greenland Pole and tri-pole grids, identical to those used by the POP2 ocean model. Its basic equations comprise horizontal and vertical transport due to thermodynamic growth and melting. The modified elastic-viscous-plastic (EVP) ice dynamics scheme (Connolley et al., Citation2004; Hunke, Citation2001; Hunke & Dukowicz, Citation1997) or a new elastic-anisotropic-plastic model (Tsamados et al., Citation2013; Wilchinsky & Feltham, Citation2006) is used to simulate transport due to ridging and other mechanical processes.
The CLM4.5 incorporates a comprehensive set of parameterizations for hydrology processes, stomatal resistance, revised photosynthesis and biogeochemistry schemes, a new lake model, new crop model features such as fertilization and grain fill, and multiple urban classes. A revised fire model includes natural and anthropogenic triggers and suppression. The CLM4.5 supports transient land cover and dynamical global vegetation changes. The MEGAN v2 (Model of Emissions of Gases and Aerosols from Nature; Guenther et al., Citation2006; Mogensen et al., Citation2011) is implemented in CLM4.5 and includes a flexible framework for assigning MEGAN emissions to species represented in the CAM5 chemistry.
All CESM model components representing the complete climate system are coupled with the Community Coupler (CPL7). The coupler controls the dataflow and fluxes exchange between the various model components and ensures their consistency while performing the execution and time evolution of the complete system.
3.2. Online integrated modelling
The other approach – fully online integration or online coupling of meteorological and chemical models – can be used in place of couplers (Baklanov et al., Citation2017; Baklanov et al., Citation2008). In particular, the online integration of meteorology and atmospheric chemical transport (ACT) was realized in the Enviro-HIRLAM (Environment – High Resolution Limited Area Model) modelling system (see schematics in ). It is used to research and forecast meteorological, chemical and biological weather. The integrated modelling system was initially developed by Danish Meteorological Institute (DMI) and other international collaborators and included as the baseline system of the Chemical Branch of the HIRLAM consortium. The first version of Enviro-HIRLAM was based on the DMI-HIRLAM numerical weather prediction (NWP) model with online integrated pollutant transport and dispersion (Chenevez et al., Citation2004), chemistry, deposition and indirect effects (Korsholm, Citation2009; Korsholm et al., Citation2008); aerosol dynamics (Baklanov, Citation2003; Gross & Baklanov, Citation2004), and sub-layer parametrizations for urban areas (Baklanov, Mestayer, et al., Citation2008; Mahura, Petersen, Baklanov, & Amstrup, Citation2008; Mahura, Petersen, Baklanov, Amstrup, Korsholm, et al., Citation2008). The model’s dynamic core was improved by adding a locally mass-conserving semi-Lagrangian numerical advection scheme (Kaas, Citation2008; Sørensen et al., Citation2013) to improve forecast accuracy. Further, a more sophisticated and effective chemistry scheme, multi-compound modal approach aerosol dynamics modules, aerosol feedbacks on radiation (direct and semi-direct effects) and on cloud microphysics (first and second indirect effects) were implemented (Nuterman et al., Citation2013, Citation2015). Baklanov et al. (Citation2017) summarise more details and corresponding references.
Figure 6. Schematics of the Enviro-HIRLAM – seamless meteorology-chemistry-aerosols multi-scale modelling system.

The Enviro-HIRLAM modelling system has been used for operational pollen forecasting in Denmark, and recently it was used for downscaling (regional-subregional-urban scales) forecasting of meteorology and aerosols for China (Mahura et al., Citation2017) (; with focus on the Shanghai metropolitan area) as well as for different research studies (being a part of EU Framework Programme, Horizon-2020 and national projects) since 2004. Following the main strategic development within HIRLAM (HIRLAM-B,C projects), further developments of Enviro-HIRLAM will smoothly shift to a new HARMONIE NWP platform incorporating the Enviro-components (such as chemistry modules, aerosol – radiation – cloud interactions, etc.) into the future HARMONIE integrated system (Baklanov, Citation2008; Baklanov et al., Citation2011, Citation2011).
Figure 7. Example of operational forecasts for PM2.5 in downscaling chain – regional-subregional-urban scales at (a) 15, (b) 5, and (c) 2.5 km horizontal resolutions, respectively – for China’s regions.

In recent years, online integrated modelling undergoes a rapid progress at the finest, turbulence-resolving spatial scales. The PALM modelling system has been coupled with ICON, COSMO, WRF, and some other meso-meteorological and weather prediction models (Maronga et al., Citation2020) as well as with aerosol module SALMA (Kurppa et al., Citation2019). The external (with other models) and internal (inside the same model) nesting functional of the PALM system open wide perspectives for seamless modelling down to turbulence scales.
3.3. Evaluation of process-models to improve global climate models parameterizations
The PEEX model infrastructure is developed through the laboratory and theoretical research platform and assimilated and evaluated towards the PEEX observation infrastructure (). The model infrastructure will be improved by simultaneously improving described processes in the models and optimising the observational system. Past studies have shown how the land-surface interactions and, thus, lower boundary conditions for ESMs are not necessarily well described within the PEEX domain (Karsisto et al., Citation2015) due to a lack of observations and better parameterisation. Thus, one of the main PEEX modelling activities is to evaluate process-models of chemistry-biosphere-atmosphere interactions in the Pan-Eurasian region and to improve at multi-scales, including the global climate models (GCM) parameterizations for processes relevant to these interactions. The model platform is applied for process understanding and generating future simulation pathways focusing on the PEEX region. The PEEX Science Plan (PEEX, Citation2015) serves as a research chain to advance our understanding of climate and air quality in the Pan-Eurasian region. It can be seen through a series of connected activities beginning at the molecular scale and extending to the sub-regional, regional and global scales. Past variations in climate in the Pan-Eurasian region and corresponding forcing agents would be revealed by analysis of firn and ice cores in glaciers and ice sheets.
A combination of forward and inverse modelling was applied to diagnosing, designing, monitoring, and forecasting air pollution in Siberia and Eurasia (Penenko et al., Citation2015a, Citation2015b; Penenko et al., Citation2012) is shown in . Such approach is useful for improving parameterizations of climatic, meteorological, chemical, and other processes in models, environmental assessments, optimization of mitigation strategies, etc. As seen in , for tasks of forward/inverse modelling and direct/adjoint problems, the problem statement should be clearly described. Here, the objects in the form of functionals describing characteristics of processes, data, and models are considered together with basic model components. The models of processes and observations are always subjected to some uncertainties. A set of algorithms for solving direct and adjoint problems; forward and inverse modelling; methods for models’ uncertainties; models’ validation/verification; observations and data assimilation procedures are continuously developed. Methods of quantitative estimations of uncertainty are of particular interest since uncertainty functions play a fundamental role in data assimilation, assessment of model quality, and inverse problem-solving. In problem statement, a scenario approach (including information on accidental releases and continuous emissions) is essential to the concept. Some methods of orthogonal decomposition of multi-dimensional phase spaces are used to reconstruct the hydrodynamic background fields from available data and to include climatic data in long-term prognostic scenarios. Input data and its variation (initial, boundary and environmental conditions) and feedbacks (interactions, numerics) are important as well. Assessment includes estimations for environmental impact (risks, consequences), state functions (for meteorology and atmospheric composition), and objective functionals (estimates, costs). Coupling between regional and global models by means of orthogonal decomposition methods allows correct information from the global processes to be passed to the regional level, where environmental quality control strategies are typically implemented (Baklanov et al., Citation2014).
Figure 9. Scheme of environmental risk assessment and mitigation strategy optimization based on forward and inverse modelling.
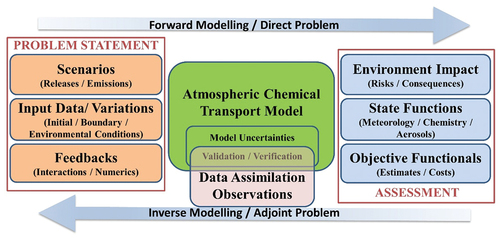
Moreover, a methodology, considering a view of “one-atmosphere” as two-way interacted meteorological and chemical processes, was suggested by Baklanov et al. (Citation2011) and Zhang et al (Citation2012a, Citation2012b). The Atmospheric Chemistry Transport (ACT) models should include not only health-affecting pollutants (air quality components) but also greenhouse gases and aerosols affecting climate, meteorological processes, etc. Such a concept requires a strategy of new-generation integrated chemistry-climate modelling systems for predicting atmospheric composition, meteorology and climate change. The online integration of meteorological/climate models and atmospheric aerosols and ACT models gives a possibility to utilise all meteorological 3D fields at each time step and to consider the feedback of air pollution (e.g. aerosols) on meteorological processes and climate forcing and further on the chemical composition (as a chain of dependent processes). This promising way for future atmospheric simulation systems (as a part of and a step to ESMs) is considered in PEEX (PEEX, Citation2015). Moreover, this has led to a new generation of models for climatic, meteorological, environmental and chemical weather forecasting (COST Action EuMetChem, 2011–2015: http://www.eumetchem.info).
3.4. Socioeconomic modelling
The socioeconomic development of the Pan-Eurasian region depends upon several global and macro-economic processes, such as the future development of the world’s energy production and consumption, the national and global demand for natural resources, specifics of the national policies in developments of the middle and northern latitude territories, and especially in the Arctic as well as considering needs of indigenous people communities. In essence, the ecological systems of the region already are also socio-economic systems. Expected climate change will play a substantial role in the overall socio-economic predictions and assessments, as well as the existing climate policy that already influences economic development. In particular, in countries of the former Soviet Union (FSU), the post-Soviet period of rapid change in the regions was characterized by many negative social tendencies and processes like substantial migration of population from the northern regions, decline of multiple taiga settlements due to the collapse of the soviet forest industry, reduction and destruction of existed transportation connections, substantial worsening/declining of social services like medicine and education, supply of first-necessity goods, destruction of the system of support of small indigenous nations etc., and especially for remote territories.
The crucial prerequisite of socioeconomic development of the region, particularly at high latitudes, is a transition to sustainable development aiming at the creation of acceptable standards of human life and maintenance of the environment and regional stability of the biosphere. In many FSU countries, this transition is declared as a starting point of national and regional policies of natural resources management. However, the reality is far from the declarations. The ecological and environmental situation in some regions of Northern Eurasia can be characterized as the ongoing severe ecological crisis initiated by unregulated anthropogenic pressure on nature and the rapid increase of production and transportation of natural resources, mostly fossil fuel, using not ecologically friendly technologies. Substantial alteration of disturbance regimes, basically in forests, with respect to extent, frequency and severity of wildfire, is observed in vast region of Northern Eurasia. Wildfires occupied over 10 million hectares annually from 2000 to 2019, resulting in forest loss of 2.7 M ha year−1 and causing direct carbon emissions of about 130 Tg C year−1 (Bondur et al., Citation2023; Shvidenko & Schepaschenko, Citation2014; Tyukavina et al., Citation2022). Altogether, this results in the decreasing quality of major components of the environment, such as air, water, soil, and vegetation, and generates many risks. This region is one of the most vulnerable regions of the globe. Already now, boreal forests are considered a tipping element (Lenton et al., Citation2008).
Considering the complexity and uncertainty associated with forecasting of the region’s socioeconomic development, PEEX is currently and will continue to utilize the integrated modelling approach as a major modelling tool for these predictions. Integrated modelling combines consideration of problems of different nature – economic, ecological and social. One of the planned ways is the use of integrated clusters like the IIASA integrated modelling framework – ESM Integrated Modelling Cluster (https://previous.iiasa.ac.at/web/home/research/researchPrograms/EcosystemsServicesandManagement/Integrated-Model-Approach.en.html). The cluster integrates different models – global economic land-use model GLOBIOM (Global Biosphere Management Model) (Havlik et al., Citation2011) connected to biophysical process-based forestry specialized model G4M (Global Forest Model) (Rametsteiner et al., Citation2007) and agriculture model EPIC (Environmental Policy Integrated Climate Model) (Izaurralde et al., Citation2006) as well as others, which are combined in a common modelling framework. The cluster could be modified and adapted for the region’s conditions and problems.
Another promising approach deals with the application of combining agent-based and stock-flow modelling methods in a participative analysis of the integrated land system. This allows the illustration of various paradigms in studying the complexity of ecological-social systems. In essence, an agent-based model is a system designing a collection of individual, heterogeneous decision-makers referred to as agents. The agents consider their options in their respective environments to form decisions based on a pre-defined set of rules working in an environment of internal and external factors and different scenarios. Taking varying perspectives of different stakeholders or decision-makers, other socioeconomic modelling and research techniques could be examined. It will be done using real options modelling for investigating the impact of uncertainty emerging from a lack of information. In questions relating to adaptation and mitigation strategies, and development, various ecological and social science methods will be employed to better understand how these political processes occur and how they can be best supported. This includes a variety of participatory methods to include relevant stakeholders. As an example of progress along this direction, we can refer to a study of optimal economic incentives in an urban area (Bergen) to reduce particulate matter pollution from households’ wood combustion (Izaurralde et al., Citation2006; Wolf et al., Citation2020; Citation2021).
3.5. High-performance computing and data infrastructure
3.5.1. High-performance computing
For most PEEX-MP models, the usage of high-performance computing (HPC) facilities and their capacities is essential. Each model involved relies, first of all, on the national level of HPC computing centres and services. For example, for European partners, the ESM EC-Earth and seamless Enviro-HIRLAM models are run in CSC (IT Center for Science Computing; https://research.csc.fi/home) HPC infrastructure. During 2019–2020, the CSC’s Taito and Sisu CRAY supercomputers were replaced by Puhti and Mahti Atos BullSequana’s supercomputers (with Intel Xenon/AMD Rome processors, respectively). These HPCs allow flexibly reserving computing and memory resources, and simulations can be run from interactive single-core data processing to medium-scale simulations spanning multiple many-core nodes. In addition, the Mahti HPC, launched in August 2020, is designed for massively parallel jobs requiring high floating-point performance and fast interconnect. The Puhti (Atos BullSequana X400 cluster) has 682 CPU nodes with a peak performance of 1.8 Pflops; 2.1 GHz base frequency, and nodes are equipped with 192 GB of memory. The Mahti (Atos BullSequana XH2000) has a total of 1404 (24) CPU (GPU) nodes with a peak performance of 7.5 (2.0) Pflops; 2.6 GHz base frequency; and CPU (GPU) nodes are equipped with 256 (512) GB of memory. Both types of nodes have two AMD Rome 7H12 CPUs with 64 cores each (total core count as 180,000). Puhti/Mahti have 4.8 (8.7) PB Lustre parallel storage system. Moreover, for long-term storage, the Allas data storage server is used. Recently, the Lumi HPC is considered one of the most powerful pan-European pre-Exascale supercomputers, and it is located in CSC’s data centre in Kajaani, Finland. After upgrading OS at Lumi, its performance is 309.1 Pflop/s. Migration of selected models to Lumi HPC is also planned in due time. On these HPCs, the architecture is designed for massively parallel applications that can effectively run on hundreds to thousands of compute cores in parallel. Obtaining this scaling level with even very tightly coupled parallel computations is possible by the extremely high-bandwidth low-latency interconnections. Another ESM CESM model is run by the University of Copenhagen in Jülich Supercomputer Center (JSC; https://www.fz-juelich.de/en/ias/jsc), Germany. This centre currently hosts the second fastest supercomputer in Europe, code-named JUQUEEN, with a peak performance of 5.9 PFlops/s and 458,752 compute cores. JUQUEEN is an IBM BlueGene/Q system (with Power BQC 16C 1.600 GHz, Custom Interconnect), which is also amongst the most energy-efficient in the world. The system is specially designed for compute-intensive, highly scalable applications, which can run in parallel on a very high number of compute cores. As part of the HPC-Europa3 Transnational Access Programme, individual grants for using HPC infrastructure and resources are possible. Such grants allow to strengthen, expand, and improve international collaboration among the PEEX partners on research and development of the PEEX Modelling Platform. In particular, CSC provided such an opportunity (access to computing infrastructure, resources, and storage) for the Enviro-HIRLAM research, development and science education within the PEEX-MP-Europe3 project (2020–2023; https://www.atm.helsinki.fi/peex/index.php/peex-mp-europa3) focused on high-Resolution integrated urban environmental modelling; online integrated atmospheric modelling: the python way; integrated modelling for assessment of potential pollution regional atmospheric transport as a result of accidental wildfires; integrated modelling and analysis of the influence of land cover changes on regional weather conditions/patterns.
In addition to daily operational numerical weather prediction modelling, a set of models (including SILAM, SALSA, CTDAS) used by the Finnish Meteorological Institute (FMI) and a set of models (including DERMA, HYCOM-ICE, HBM) used by the Danish Meteorological Institute (DMI) are also employing the FMI’s and DMI’s Cray XC50 supercomputers to perform the simulations. The University of Leeds uses the Advance Research Computing (ARC; http://arc.leeds.ac.uk) facilities to run CAM-Chem, TOMCAT-GLOMAP, HadGEM2, and other models. Other European PEEX-MP partners involved run their models at their national HPC facilities and local clusters. In addition, the ECMWF HPC facilities are used as part of the collaborative projects – Enviro-PEEX on ECMWF (2018–2020; https://www.atm.helsinki.fi/peex/index.php/enviro-peex-2018–2020) and Enviro-PEEX (Plus) (2021–2023; https://www.atm.helsinki.fi/peex/index.php/enviro-peex_plus), where more than 30 researchers from 15 institutions from many countries are involved. Until migration to the new HPC system, the ECMWF HPC system was based on 2 Cray XC40 (Intel Broadwell processor, 18/36 cores per socket/nodes, 2.1 GHz frequency, 128 GiB memory, 7020 computer nodes, 0.9 PiB memory 260,208 cores). After migration in 2022, a new facility with four self-sufficient clusters (or complexes) came into operation. It is based on Atos BullSequana XH2000 (AMD Epyc Rome processor, 64/128 cores per socket/node, 2.25 GHz frequency, 256 GiB memory, 7680 computer nodes, 2.1 PiB memory 1,040,384 cores). This new HPC service could deliver up to about five times the performance of the older system. Note that these ECMWF HPC projects focus on further PEEX-MP research and development for online coupled integrated meteorology-chemistry-aerosols feedback and interactions in weather, climate and atmospheric composition multi-scale modelling. Furthermore, supercomputing is essential for the high-resolution LES models in the PEEX-MP. Scalability, efficient MPI-Open MP hybrid parallel computing and binary identical reproducibility are still main challenges for improving computing performance of earth system models when using many-core supercomputers.
Moreover, the involved PEEX-MP partners also run models (at their national HPC facilities and local clusters): FSU partners – GEOS-Chem, SWAN, ATMES, IMDAF, EurCTM and others; and Chinese partners – CH4MOD, Agro-C, AVIM2, CNMM-DNDC, EMBE, EMSR, EMGPP, and others.
3.5.2. Data infrastructure
The data flow () for the PEEX-MP is organized from simulations to assessment products and publications with long-term archiving and delivering for potential customers. The research data from PEEX models will be deposited with CSC’s Allas (https://research.csc.fi/-/allas) and IDA (https://www.fairdata.fi/en/ida) long-term general storage service for scientific data to ensure long-term access for the research community. Moreover, Allas, as an object storage system, is one of the best options for storing, managing, sharing, and analysing data across the computing infrastructure. Allas’s advantages include possibility to handle any static data, to access stored data from anywhere using URL, to have data with different levels of access control, and others. IDA provides secure storage, description, and publication of data as research datasets. IDA’s advantages include possibility to have open data access, to utilize user-friendly interface, to have option of growing or cumulative dataset, and others. As the research environment is changing continuously, there are also changes in how research data are managed. The links to the data will be generated with CSC’s Etsin service (https://etsin.fairdata.fi; e.g. research data finder that contains detailed information for metadata and allows finding research datasets from all fields of science) and included in PEEX publications. It will utilize the research dataset description tool Qvain (https://qvain.fairdata.fi), which is used to describe and publish research data with persistent identifier, landing page and to distribute its description to other relevant services. The PEEX dataflow will be organized entirely in CSC data infrastructure (HPC, archive, Allas | IDA). Additionally, various model data, including climate-related data, will be provided for open access through the national Earth System Grid Federation (ESGF) node, which is being implemented in the CSC infrastructure. The infrastructure and services of EUDAT (EUropean DATa Infrastructure; https://www.eudat.eu) for data collaboration are used through CSC between project researchers and collaborators. For example, such service as B2DROP is used for data sync and exchange, B2SHARE – to store and share, B2FIND – to find, etc. Selected datasets of smaller size could be uploaded and became available at Zenodo (https://zenodo.org), an open repository where research papers, software, reports, etc., can also be stored.
Figure 10. PEEX Modelling Platform data flow from model runs to products and publications with long-term archiving and delivering for potential customers.

In particular, the bulk of the ESM data consists of climate global-scale model output, which is a combination of NetCDF (network Common Data Form), HDF (Hierarchical Data Format) and GRIB (General Regularly distributed Information in Binary form) formats files. These formats are widely used for storing, exchanging, managing and archiving scientific complex multidimensional modelled data (various variables). The raw data are of Tb-scale, which is processed to a suitable size (Gb or Mb) for archiving and distribution. The observational data used for assimilation and evaluation are mostly pre-processed and harmonized data from the PEEX observation infrastructure. The climate model data in NetCDF and HDF formats support the inclusion of metadata inside the file structure. The climate model annotates relevant metadata during runtime: time of experiment, global model attributes, parameterisation details, etc. Further post-processing of NetCDF files will implement details of post-processing steps in the file as a diary. Finally, relevant data are documented as products and/or publications (e.g. in peer-reviewed manuscripts).
A selected portion of model runtime information is directed to model raw data output, including, e.g. daily and monthly accumulated variables and detailed datasets over the PEEX observation infrastructure. The generated raw model output can easily amount up to several Tb per simulated year, which is reduced to Gb-size in post-processing. A prescribed set of model output is directed to long-term archival services, where a Digital Object Identifier (DOI) is addressed to the data blocks. While a research publication contains only a minor amount of data, it will link to the DOIs of relevant datasets. Furthermore, Virtual Research Platforms directly utilize the archived datasets, see examples in Section 4.
4. Virtual research platforms
4.1. PEEX-View
Several tools have been created as online tools for visualizing and analyzing the PEEX data. The tool, PEEX-View (https://www.atm.helsinki.fi/peex_view), is based on open standards and open-source software. At first, the PEEX-View tool will be able to combine multidisciplinary datasets of varying temporal and spatial scale (Hari & Kulmala, Citation2005; Hari et al., Citation2009, Citation2016). Second, this tool will not be limited only to natural science data: even socio-economic indicators, such as for example an industrial production index, can be used in analysing changes in e.g. atmospheric observations. Currently, PEEX-View holds 2D datasets (satellite products), time series (station observations), point-data (e.g. wildfire occurrence), air mass trajectories, and others. All datasets can be complemented by relevant metadata. The tool is designed to establish fluent comparison and evaluation of modelling and observational data.
Technically, the frontend of PEEX-View is built on the OpenLayers 3. The original data are transformed into the keyhole markup language (KML) format to combine data, metadata and geographic information. The 2D maps (e.g. satellite data) are integrated as normal images. All data data-processing and KML generation are currently made with implemented Matlab – functions and Google Earth toolbox. At the OpenLayers environment, the KML-files and images are structurally placed into specific folders. They are read to the HTML and managed by JavaScript. Some HTML-elements are generated with PHP and others with JavaScript. OpenLayers reads directly certain data from KML-files. The information contains station icons at respective locations and, at least, name- and description-fields. Images require a transformation to the spherical Mercator projection. The generated KML-files can be opened also in Google-Earth – a 3D mapping software.
Online demo-version of PEEX-View visualizes (examples are shown in ) the time series for the modeled data vs. observed data, currently aerosol number concentration. The modeled data are based on the global aerosol-climate model ECHAM-HAM. Observed time-series data are based on aerosol measurements made at the SMEAR-II (Hyytiälä, Finland) field station for June 2008. Two 2D satellite products are also included: MODIS-derived aerosol optical depth and ocean chlorophyll concentration. Wildfires observed from MODIS-data are presented in the timeline with 2-day resolution. Pre-calculated trajectories (based on ECMWF data and calculated by the FLEXTRA model) for stations are acquired from NILU database. The PEEX-View generated maps demonstrate the field observation station potential across geographical areas of the Pan-Eurasian region. For these stations, contact information and a view of the site landscape are available.
Figure 11. Screenshot of the online PEEX-View tool (with locations of the PEEX stations) showing: (a) aerosol concentration and backward trajectories arriving at the SMEAR-II (Hyytiälä) station, MODIS-derived fire locations for 12 June 2008, and monthly average ocean chlorophyll concentrations from MODIS; and (b) monthly averaged (June 2008) aerosol optical depth and chlorophyll concentrations.

The online tool is being prepared to integrate new datasets from projects and partners of the PEEX program. From a modelling perspective, such software can be used for evaluation of the PEEX-MP models, including multi-scale atmospheric modelling suites consisting of Earth System Models, Chemistry Transport Models, and single-column models. Furthermore, socio-economic models are an integral part of the PEEX program, and the PEEX-View tool allows to combine socio-economic products with natural science data into same environment of the tool (Lappalainen et al., Citation2016). This tool can include both historic indicators of socio-economic development in a given region of interest and scenarios to guide future progress. While PEEX-View is an essential part of PEEX-MP, it is also used in the PEEX research training and educational activities, such as Young Scientist Schools (arranged by PEEX Partners’ Universities during 2017–2023).
In addition, together with the Smart-SMEAR online tool (https://smear.avaa.csc.fi; Junninen et al., Citation2009) used for data visualization and downloading datasets of continuous atmospheric, flux, soil, tree physiological and water quality measurements (carried out at different time intervals) at SMEAR research stations, the PEEX-View tool provides an approachable tool for handling large data volumes. In Smart-SMEAR, both data and basic metadata can be downloaded and visualized using Download and Preview pages. Moreover, in this tool, a graphical user interface allows a temporal aggregation as well as application programming interface (API) provides access to additional variables and more complete metadata.
4.2. Virtual research environment
Virtual research environment (VRE) is elaborated for regional climatic and ecological studies support. Volumes of environmental data archives are growing immensely due to increasing resolutions of recent models, high-performance computers and sensors development. It makes impossible comprehensive analysis of data archives in conventional manner on workplace using in house computing facilities, data storage and processing software at hands. One possible answer to this challenge is creation of VRE, which should provide a researcher with an integrated access to huge data resources, tools and services across disciplines and user communities and enable researchers to process structured and qualitative data in virtual workspaces (De Roure & Goble, Citation2007). Thematic VRE should integrate data, network and computing resources providing interdisciplinary climatic research community with opportunity for better understanding of ongoing and possible future climatic changes and their consequences for environment and population, sustainable development of society and economy, etc.
First steps in development of the PEEX domain VRE elements aimed at regional climatic and ecological monitoring and modelling as well as at continuous education and training support were realized in the FP6 EU Enviro-RISKS project (Baklanov & Gordov, Citation2006). The interactive web-based-system for regional climate assessment using standard meteorological data archives was developed and launched into operation (http://climate.risks.scert.ru). In particular, the so-called “Climate Platform” (http://climate.scert.ru) was developed in the project, and it is aimed at integrated analysis of heterogeneous georeferenced data (Gordov et al., Citation2013; Gordova et al., Citation2013; Okladnikov et al., Citation2013; Shulgina et al., Citation2013). It is used as an element of the PEEX VRE prototype which can include geoportal containing selected observational and modelling results and thematic data, tools for online processing, statistical analysis and visualisation, as well as integrate thematic data storages. On perspective, VRE specific tools should be aimed at high resolution rendering on-going climatic and environmental processes occurring in Northern Eurasia and reliable and found prognoses of their dynamics for selected sets of future mankind activity scenario. As any VRE, it can be also used for educational and training purposes, especially for younger generation of researchers.
Taking into account the diversity and integrated character of research intended to be completed under the PEEX initiative, it is desirable to have a solid georeferenced basis which would contain all available accumulated information about landscapes, terrestrial ecosystems, waterbodies, biological productivity of the biosphere and its interaction with the lower troposphere, etc. The utilisation of GIS tools with corresponding attributive databases and various integrated datasets at different resolutions is beneficial for VRE. In particular, it was realized in full and verified carbon account of selected Eurasian terrestrial ecosystems (Shvidenko et al., Citation2015; Shvidenko, Schepaschenko, McCallum, et al., Citation2010) for considering large-scale ecological and socio-economic tasks. The information basis was presented in the form of the Integrated Land Information System which contains available comprehensive description of terrestrial ecosystems and landscapes.
4.3. Web-based atlas
The methodology for multidisciplinary probabilistic environmental risk and vulnerability assessments elaborated in the Nordic ArcticRisk-NARP and FP6 EC Enviro-RISKS projects (Baklanov & Mahura, Citation2004; Baklanov, Sorensen, et al., Citation2008; Baklanov et al., Citation2011; Mahura & Baklanov, Citation2004; Mahura, Baklanov, & Sørensen, Citation2009; Mahura et al., Citation2005) can be refined and applied as a web-based tool for evaluation of potential impact on environment and population in the PEEX region (examples are shown in ). The GIS and Google-Earth components of such tool could provide a more valuable representation. The html-format and Java Scripting are used for building the web-based atlas. On an on-line web-request, for selected geographical locations of continuous emissions, accidental releases, planned constructions and operations, etc. the short- and long-term (ranging from a day up to several days, months, and up to one year) simulations with trajectory and dispersion modelling approaches can be used to construct various indicators of potential impact. These can include dominating atmospheric transport pathways, airflow probabilities, maximum reaching distances, fast transport, precipitation factor, time integrated air concentration, dry, wet, and total deposition patterns as well as other indicators. The results of these simulations are also applicable for integration and input for further evaluation of doses, impacts, risks, short- and long-term consequences for population and environment from potential sources. Evaluation and mapping of risks will be important for decision-making processes and for analysis of environmental, social, and economical consequences for different geographical areas and various population groups taking into account social-geophysical factors and probabilities, and using demographic and administrative databases. All these can be provided through the web-portal.
Figure 12. Web-based atlas for (a) month-to-month variability of average (AVG) and summary (SUM) time integrated air concentration (TIAC), dry (DD) and wet (WD) deposition patterns of sulphates from smelters of the Norilsk Nikel Enterprize (Krasnoyarsk Krai, Russia); and (a) individual yearly loadings for population (in kg/person) from deposited sulfates resulted from the severonickel smelters (Kola Peninsula) continuous emissions (mild scenario, appx 32 thou. tonnes of SO2).
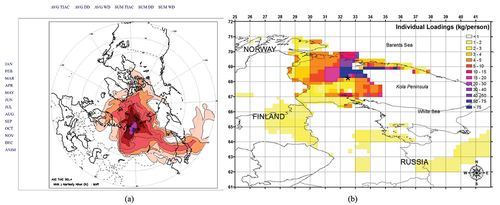
As an illustration, the web-based atlas representing monthly (from January till December) variability of the average and summary time integrated air concentration, dry and wet deposition patterns ( – on example of March) resulted from sources of continuous emissions in the Arctic regions (and in particular, on the Kola Peninsula and Krasnoyarsk Krai) is available at: http://www.atm.helsinki.fi/peex/webatlas/WEBATLAS.html. Another illustration is the results of the GIS-based integration and analysis of the pollution modelling results as potential loadings on the population with a focus on the Kola Peninsula and Scandinavian countries ( – on a yearly scale) on yearly and seasonal scales (Mahura et al., Citation2018).
Moreover, co-production and co-design with stakeholders lead us to understanding that web atlas needs expansion to web-based storytelling where factual information is assisted and extended by related narratives and societal values. Miles et al. (Citation2023) describes scientific foundation and working approaches to the environmental web-based storytelling on examples of URSA MAJOR and TURBAN projects which are developing new ways to model air quality and thermal comfort in urban areas and providing policymakers and urban planners with information needed to make informed decisions.
5. Conclusions
The Pan-Eurasian Experiment Modelling Platform (PEEX-MP) is an integral part of the PEEX research programme, and, it is one of the key blocks of the PEEX Programme. The seamless coupling multi-scale modelling concept presented here is an important and advanced step towards realization of the PEEX research agenda. It was demonstrated on a series of completed studies and achievements (with relevant references to peer-reviewed publications). The PEEX-MP includes more than 30 different models capable of helping to address urgent scientific questions and grand challenges of the Arctic-boreal and China domains, of key focus for PEEX interests. These models cover main components such as the atmosphere, hydrosphere, pedosphere and biosphere and resolve the physical-chemical-biological processes at different spatial and temporal scales and resolutions. The Earth system modelling, online coupled integrated modelling, forward and inverse modelling, socio-economical modelling, and others described in this paper are valuable and useful approaches for further development of the state-of-the-art and breaking-through research in the PEEX domain. The high-performance computing facilities and capabilities are of critical importance for the PEEX modelling activities. Currently, these are at relatively good level among the PEEX-MP partners, although further improvement in performance of the models and more efficient usage of power resources are targeted. The PEEX generated large volumes of model data to be processed and became freely available for the research, decision-makers and end-user communities. The virtual research platforms – i.e. tools such as the PEEX-View, Virtual Research Environment, Web-based Atlas – presented here will allow to improve not only visualization of modelling and observational results using modern GIS and web-based technologies but also to perform more in depth complex and sophisticated analysis of various components of the Earth’s system interactions and feedback, evaluation of processes and parameterizations for models’ improvements. In this manner, the PEEX scientific community can develop and provide novel services for the different stakeholders in the Boreal-Arctic environment.
TBED-2023-0046R2_SupplMaterial.docx
Download MS Word (31.9 KB)Acknowledgements
The authors are thankful to the PEEX-MP members for fruitful discussions and comments in developing of the PEEX-Modelling-Platform. The co-authors acknowledge that this work was also one of the last projects of Prof. Sergej Zilitinkevich (1936–2021), and we are thankful for his constructive comments and criticism of the paper. Since the finalization took time, the paper was actually submitted in 2023 and we could not argue that the final paper text was agreed with him. The financial support was/is provided through multiple projects related to the Pan-Eurasian EXperiment (PEEX) programme including Academy of Finland projects – ClimEco (grant #314 798/799), ACCC (grant #337549), HEATCOST (grant #334798); European Union’s Horizon 2020 Programme projects – iCUPE under ERA-PLANET (grant #689443), INTAROS (grant #727890), EXHAUSTION (grant #820655), CRiceS (grant #101003826), RI-URBANS (grant #101036245); Horizon Europe project FOCI (grant #101056783); Erasmus+ Programme projects – ECOIMPACT (grant #561975-EPP-1-2015-1-FI-EPPKA2-CBHE-JP), ClimEd (grant #619285-EPP-1-2020-1-FI-EPPKA2-CBHE-JP); The Norwegian Research Council INTPART educational and networking project (322317/H30): URban Sustainability in Action: Multi-disciplinary Approach through Jointly Organized Research schools; and the EEA project (Contract No. 2020TO01000219): Turbulent-resolving urban modelling of air quality and thermal comfort (TURBAN). The work was also supported by the Consiglio Nazionale delle Ricerche (Italy), Helsingin Yliopisto (Finland), International Institute for Applied Systems Analysis (Austria), Københavns Universitet (Denmark), Max-Planck-Institut für Chemie (Germany), University of Helsinki (Finland), Estonian University of Life Sciences (Estonia), Norwegian Institute for Air Research (Norway), Danish Meteorological Institute (Denmark), Chinese Academy of Sciences (China), Nansen Environmental and Remote Sensing Center (Norway), Finnish Meteorological Institute (Finland), School of the Earth and Environment, University of Leeds (UK), Universitetet i Tromsø (Norway), World Meteorological Organization (Switzerland).
Disclosure statement
No potential conflict of interest was reported by the authors.
Data availability statement
The data that support the findings of this study are available from authors of this paper upon reasonable request. The model outputs (from different mentioned models) are available on a case-by-case or project-by-project basis and can be accessed through communication with owners of the model results or following the references in the manuscript to corresponding publications. The observations (from different mentioned studies/campaigns/etc.) are also available on a same basis and can be accessed by contacting the owners of the observations or following the references in the manuscript to corresponding publications (or mentioned datasets/databases). The results shown in Section 4 of the manuscript (at https://www.atm.helsinki.fi/peex_view and http://www.atm.helsinki.fi/peex/webatlas/WEBATLAS.html) can be openly/publicly accessed and downloaded.
Supplementary material
Supplemental data for this article can be accessed online at https://doi.org/10.1080/20964471.2024.2325019
Additional information
Funding
Notes on contributors

Alexander Mahura
Alexander Mahura is a University Researcher at Institute for Atmospheric and Earth System Research, University of Helsinki (UH), Finland (since 2017); received BSc in 1991, MSc in 1998, PhD Phys&Math Atm.Sci. in 2002; worked in Russia, USA, Austria, France, Denmark, and Finland. His research interests include online integrated multi-scales and -processes modelling of meteorology and atmospheric composition, atmospheric chemistry, atmospheric boundary layer processes, numerical weather prediction, fine-scale road weather and birch pollen forecasting, statistics for data analysis and post-processing models output, environmental impact and risk assessment.

Alexander Baklanov
Alexander Baklanov is a Scientific Officer at Research Department, World Meteorological Organization, Switzerland (since 2014) and affiliated Professor at Niels Bohr Institute, University of Copenhagen, Denmark (since 2011); received MSc in 1979, PhD Phys&Math Geophys. in 1983, Dr.Sci. Phys&Math Atm.Sci. in 1998, Prof. Meteorology in 2008; worked in Russia, Austria, Sweden, Denmark, Switzerland; Editor-in-Chief of the Urban Climate journal. His research interests are environmental and atmospheric sciences, in particular, in developing a new generation of online coupled meteorology-chemistry models, especially for urban applications.

Risto Makkonen
Risto Makkonen is a tenure track Research Professor at Finnish Meteorological Institute (since 2018) and University Researcher at Institute for Atmospheric and Earth System Research, University of Helsinki, Finland (since 2017); received MSc in 2006, PhD in 2012; worked also in University of Oslo, Norway; Supervisor for UH’s Doctoral Programme in Atmospheric Sciences. His research interests include Earth System Modelling, atmospheric aerosol processes and aerosol-biosphere climate feedbacks.
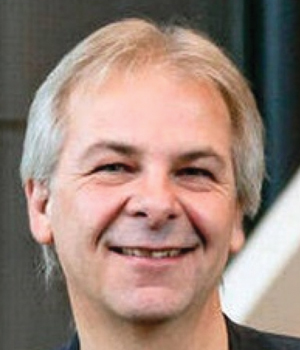
Michael Boy
Michael Boy is a Professor at Institute for Atmospheric and Earth System Research, University of Helsinki (since 2022) and at Division of Computational Engineering, Lappeenranta University of Technology, Finland (since 2022); received MSc Physical Engineering in 1992, MSc Meteorology in 1998, PhD Physics in 2003, Docent Physics in 2008, Prof. in 2022; worked in Germany, USA, Finland; Supervisor for UH’s Doctoral Programme in Atmospheric Sciences. His research interests include atmospheric chemistry, aerosol dynamics, application machine learning and artificial intelligence techniques in these areas.

Tuukka Petäjä
Tuukka Petäjä is a Professor at Institute for Atmospheric and Earth System Research, University of Helsinki; MSc in 2004, PhD in 2006, Docent in 2011, Prof. Physics (2013, experimental atmospheric sciences). His research interests include aerosol–cloud interactions, development of mass spectrometric methods for atmospheric aerosols and trace gases; measurement techniques for aerosol particles; long-term and field campaigns; aerosol-cloud-climate-biosphere interactions, air quality. He has educated 37 PhDs to completion. Highly cited scientist (since 2014); Aerosol Foundation Award for multidisciplinary research 2022, Vaisala Award 2013; Science and Technology in Society Future Leader Award, New York Academy of Sciences (Co)-Coordinating EU projects (iCUPE, ACTRIS IMP, RI-URBANS).

Markku Kulmala
Markku Kulmala is Academician, Director of Institute for Atmospheric and Earth System Research, University of Helsinki, UH (since 2017); received PhD Physics in 1988, Docent in 1993, Prof. in 1996; Academy Professor, Academy of Finland, 2004–2009, 2011–2015, 2017–; Academician, Academy of Finland, 2017; Head of Laboratory of Aerosol and Environmental Physics, UH (since 1990), Head of the Division of Atmospheric Sciences, UH (since 2001); Supervisor for UH’s Doctoral Programme in Atmospheric Sciences. His research interests include aerosols (dynamics, formation and growth mechanisms, interactions), climate, air quality, physics, chemistry, biosphere, Earth system and atmospheric sciences, science, aerosol–cloud - atmosphere/climate - biosphere/ecosystem - air quality interactions.
References
- Aagaard, K., Coachman, L. K., & Carmack, E. (1981). On the halocline of the Arctic Ocean. Deep-Sea Research, 28A(6), 529–545. https://doi.org/10.1016/0198-0149(81)90115-1
- Alekseychik, P., Lappalainen, H. K., Petäjä, T., Zaitseva, N., Heimann, M., Laurila, T., Lihavainen, H., Asmi, E., Arshinov, M., Shevchenko, V., Makshtas, A., Dubtsov, S., Mikhailov, E., Lapshina, E., Kirpotin, S., Kurbatova, Y., Ding, A., Guo, H. … Kulmala, M. (2016). Ground-Based Station Network in Arctic and Subarctic Eurasia: An overview. Geography, Environment, Sustainability, 9(2), 75–88. https://doi.org/10.15356/2071-9388_02v09_2016_06
- Arneth, A., Harrison, S. P., Zaehle, S., Tsigaridis, K., Menon, S., Bartlein, P. J., Feichter, J., Korhola, A., Kulmala, M., O’donnell, D., Schurgers, G., Sorvari, S., & Vesala, T. (2010). Terrestrial biogeochemical feedbacks in the climate system. Nature Geoscience, 3(8), 525–532. https://doi.org/10.1038/ngeo905
- Arnold, S. R., Law, K. S., Brock, C. A., Thomas, J. L., Starkweather, S. M., von Salzen, K., Stohl, A., Sharma, S., Lund, M. T., Flanner, M. G., Petäjä, T., Tanimoto, H., Gamble, J., Dibb, J. E., Melamed, M., Johnson, N., Fidel, M., Tynkkynen, V.-P. … Bozem, H. (2016). Arctic air pollution: Challenges and opportunities for the next decade. Elementa: Science of the Anthropocene, 4, 000104. https://doi.org/10.12952/journal.elementa.000104
- Auvinen, M., Järvi, L., Hellsten, A., Rannik, Ü., & Vesala, T. (2017). Numerical framework for the computation of urban flux footprints employing large-eddy simulation and Lagrangian stochastic modeling. Geoscientific Model Development, 10(11), 4187–4205. https://doi.org/10.5194/gmd-10-4187-2017
- Bai, J., & Duhl, T. (2021). A primary generalized empirical model of BVOC emissions for some typical forests in China. Atmospheric Pollution Research, 12(8), 101126. https://doi.org/10.1016/j.apr.2021.101126
- Bai, J., Duhl, T., & Hao, N. (2016). Biogenic volatile compound emissions from a temperate forest, China: model simulation. Journal of Atmospheric Chemistry, 73(1), 29–59. https://doi.org/10.1007/s10874-015-9315-3
- Bai, J., Guenther, A., Turnipseed, A., Duhl, T., & Greenberg, J. (2017). Seasonal and interannual variations in whole-ecosystem BVOC emissions from a subtropical plantation in China. Atmospheric Environment, 161, 176–190. https://doi.org/10.1016/j.atmosenv.2017.05.002
- Bai, J. H., Heikkilä, A., & Zong, X. M. (2021). Long-term variations of global solar radiation and atmospheric constituents at Sodankylä in the Arctic. Atmosphere, 12(6), 749. https://doi.org/10.3390/atmos12060749
- Bai, J. H., Yang, F. T., Wang, H. M., & Xu, M. J. (2023). An empirical model of gross primary productivity (GPP) and relations between GPP and its driving factors, biogenic volatile organic compounds in a subtropical conifer plantation in China. Atmosphere, 14(6), 1046. https://doi.org/10.3390/atmos14061046
- Bai, J. H., & Zong, X. M. (2021). Global solar radiation transfer and its loss in the atmosphere. Applied Sciences, 11(6), 2651. https://doi.org/10.3390/app11062651
- Bai, J., Zong, X., Ma, Y., Wang, B., Zhao, C., Yang, Y., Guang, J., Cong, Z., Li, K., & Song, T. (2022). Long-term variations in global solar radiation and its interaction with atmospheric substances at qomolangma. International Journal of Environmental Research and Public Health, 19(15), 8906. https://doi.org/10.3390/ijerph19158906
- Baklanov, A. (2003). Modelling of formation and dynamics of radioactive aerosols in the atmosphere. In Research on a Theory of Elementary Particles and Solid State (Vol. 4, pp. 135–148). Yaroslavl University.
- Baklanov, A. (2008). Integrated meteorological and atmospheric chemical transport modeling: Perspectives and strategy for HIRLAM/HARMONIE. HIRLAM Newsletter, 53, 68–78.
- Baklanov, A., Aloyan, A., Mahura, A., Arutyunyan, V., & Luzan, P. (2011). Evaluation of source‐receptor relationship for atmospheric pollutants using approaches of trajectory modelling, cluster, probability fields analyses and adjoint equations. Atmospheric Pollution Research, 2(4), 400‐529. https://doi.org/10.5094/APR.2011.045
- Baklanov, A., Brunner, D., Carmichael, G., Flemming, J., Freitas, S., Gauss, M., Hov, O., Mathur, R., Schlünzen, K., Seigneur, C., & Vogel, B. (2017). Key issues for seamless integrated chemistry–meteorology modeling. Bulletin of the American Meteorological Society, 98(11), 2285–2292. https://doi.org/10.1175/BAMS-D-15-00166.1
- Baklanov, A., & Gordov, E. P. (2006). Man-induced environmental risks: Monitoring, management and remediation of man-made changes in Siberia. Computational Technologies, 11, 162–171.
- Baklanov, A. A., Korsholm, U. S., Mahura, A. G., Nuterman, R. B., Sass, B. H., & Zakey, A. S. (2011). Physical and chemical weather forecasting as a joint problem: Two-way interacting integrated modelling, in: American Meteorological Society 91st Annual Meeting, Paper 7.1, American Meteorological Society,
- Baklanov, A., Korsholm, U., Mahura, A., Petersen, C., & Gross, A. (2008). ENVIRO-HIRLAM: On-line coupled modelling of urban meteorology and air pollution. Advances in Science and Research, 2(1), 41–46. https://doi.org/10.5194/asr-2-41-2008
- Baklanov, A., Korsholm, S. U., Nuterman, R., Mahura, A., Nielsen, K. P., Sass, B. H., Rasmussen, A., Zakey, A., Kaas, E., Kurganskiy, A., Sørensen, B., & González-Aparicio, I. (2017). Enviro-HIRLAM online integrated meteorology–chemistry modelling system: Strategy, methodology, developments and applications (v7.2). Geoscientific Model Development, 10(8), 2971–2999. https://doi.org/10.5194/gmd-10-2971-2017
- Baklanov, A., & Mahura, A. (2004). Assessment of possible airborne impact from risk sites: Methodology for probabilistic atmospheric studies. Atmospheric Chemistry and Physics, 4(2), 485–495. https://doi.org/10.5194/acp-4-485-2004
- Baklanov, A., Mahura, A., Penenko, V., Zilitinkevich, S., & Kulmala, M. (2014). Pan-Eurasian Experiment (PEEX): Modelling Platform for Earth System Observations and Forecasting. Geophysical Research Abstracts, 16, EGU2014–15751.
- Baklanov, A. A., Mahura, A., & Sokhi, R. (Eds.). (2011). Integrated Systems of Meso-Meteorological and Chemical Transport Models. Springer. https://doi.org/10.1007/978-3-642-13980-2_1
- Baklanov, A., Mestayer, P. G., Clappier, A., Zilitinkevich, S., Joffre, S., Mahura, A., & Nielsen, N. W. (2008). Towards improving the simulation of meteorological fields in urban areas through updated/advanced surface fluxes description. Atmospheric Chemistry and Physics, 8(3), 523–543. https://doi.org/10.5194/acp-8-523-2008
- Baklanov, A., Schlünzen, K. H., Suppan, P., Baldasano, J., Brunner, D., Aksoyoglu, S., Carmichael, G., Douros, J., Flemming, J., Forkel, R., Galmarini, S., Gauss, M., Grell, G., Hirtl, M., Joffre, S., Jorba, O., Kaas, E., Kaasik, M. … Zhang, Y. (2014). Online coupled regional meteorology chemistry models in Europe: Current status and prospects. Atmospheric Chemistry & Physics, 14(1), 317–398. https://doi.org/10.5194/acp-14-317-2014
- Baklanov, A., Sorensen, J. H., & Mahura, A. (2008). Methodology for Probabilistic Atmospheric Studies using Long‐Term Dispersion Modelling. Environmental Modelling and Assessment, 13(4), 541‐552. https://doi.org/10.1007/s10666-007-9124-4
- Baklanov, A., & Zhang, Y. (2020). Advances in air quality modeling and forecasting. Global Transitions, 2, 261–270. https://doi.org/10.1016/j.glt.2020.11.001
- Bergman, T., Makkonen, R., Schrödner, R., Swietlicki, E., Phillips, V. T. J., Le Sager, P., & van Noije, T. (2022). Description and evaluation of a secondary organic aerosol and new particle formation scheme within TM5-MP v1.2. Geoscientific Model Development, 15(2), 683–713. https://doi.org/10.5194/gmd-15-683-2022
- Berg, P., & Poulsen, J. W. (2012). Implementation details for HBM. DMI Technical Report No. 12-11, ISSN: 1399-1388,
- Bey, I., Jacob, D. J., Yantosca, R. M., Logan, J. A., Field, B. D., Fiore, A. M., Li, Q. B., Liu, H. G. Y., Mickley, L. J., & Schultz, M. G. (2001). Global modeling of tropospheric chemistry with assimilated meteorology: Model description and evaluation. Journal of Geophysical Research - Atmospheres, 106(D19), 23073–23095. https://doi.org/10.1029/2001JD000807
- Bondur, V. G., Gordo, K. A., Voronova, O. S., Zima, A. L., & Feoktistova, N. V. (2023). Intense wildfires in Russia over a 22-year period according to satellite data. Forests, 6(3), 99. https://doi.org/10.3390/fire6030099
- Booij, N., Ris, R., & Holthuijsen, L. A. (1999). Third-Generation Wave Model for coastal regions. 1. Model description and validation. Journal of Geophysical Research - Oceans, 104(C4), 7649–7666. https://doi.org/10.1029/98JC02622
- Boy, M., Sogachev, A., Lauros, J., Zhou, L., Guenther, A., & Smolander, S. (2011). SOSA – a new model to simulate the concentrations of organic vapours and sulphuric acid inside the ABL – part 1: Model description and initial evaluation. Atmospheric Chemistry and Physics, 11(1), 43–51. https://doi.org/10.5194/acp-11-43-2011
- Bruhwiler, L., Dlugokencky, E., Masarie, K., Ishizawa, M., Andrews, A., Miller, J., Sweeney, C., Tans, P., & Worthy, D. (2014). CarbonTracker-CH4: An assimilation system for estimating emissions of atmospheric methane. Atmospheric Chemistry and Physics, 14, 8269–8293. https://doi.org/10.5194/acp-14-8269-2014 16
- Buzzi, A., Fantini, M., Malguzzi, P., & Nerozzi, P. (1994). Validation of a limited area model in cases of mediterranean cyclogenesis: Surface fields and precipitation scores. Meteorology and Atmospheric Physics, 53(3–4), 137–153. https://doi.org/10.1007/BF01029609
- Chassignet, E. P., Hurlburt, H. E., Smedstad, O. M., Halliwel, G. R., Hogan, P. J., Wallcraft, A. J., Baraille, R., & Bleck, R. (2007). The HYCOM (hybrid coordinate ocean model) data assimilative system. Journal of Marine Systems, 65(1–4), 60–83. https://doi.org/10.1016/j.jmarsys.2005.09.016
- Chenevez, J., Baklanov, A., & Sørensen, J. H. (2004). Pollutant transport schemes integrated in a numerical weather prediction model: Model description and verification results. Meteorological Applications, 11(3), 265–275. https://doi.org/10.1017/S1350482704001343
- Chen, C., Kuhn, U., Pöschl, U., Su, H., & Cheng, Y. (2018). Elevated HONO emissions in agricultural system: Model simulation by the improved DNDC model (DNDC-HONO). Geophysical Research Abstracts, 20, EGU2018–13415–1.
- Cohen, J., Zhang, X., Francis, J., Jung, T., Kwok, R., Overland, J., Ballinger, T. J., Bhatt, U. S., Chen, H. W., Coumou, D., Feldstein, S., Gu, H., Handorf, D., Henderson, G., Ionita, M., Kretschmer, M., Laliberte, F., Lee, S., Linderholm, H. W., & Wu, Y.… Yoon, J. (2020). Divergent consensuses on Arctic amplification influence on midlatitude severe winter weather. Nature Climate Change, 10(1), 20–29. https://doi.org/10.1038/s41558-019-0662-y
- Collins, W. J., Bellouin, N., Doutriaux-Boucher, M., Gedney, N., Halloran, P., Hinton, T., Hughes, J., Jones, C. D., Joshi, M., Liddicoat, S., Martin, G., O’Connor, F., Rae, J., Senior, C., Sitch, S., Totterdell, I., Wiltshire, A., & Woodward, S. (2011). Development and evaluation of an Earth-system model – HadGEM2. Geoscientific Model Development, 4(4), 1051–1075. https://doi.org/10.5194/gmd-4-1051-2011
- Connolley, W. M., Gregory, J. M., Hunke, E. C., & McLaren, A. J. (2004). On the consistent scaling of terms in the sea ice dynamics equation. Journal of Physical Oceanography, 34(7), 1776–1780. https://doi.org/10.1175/1520-0485(2004)034<1776:OTCSOT>2.0.CO;2
- De Roure, D., & Goble, C. (2007). myExperiment – A Web 2.0 Virtual Research Environment. In, International Workshop on Virtual Research Environments and Collaborative Work Environments, Edinburgh, UK, http://eprints.soton.ac.uk/id/eprint/263961
- She J., Bethers, U., Cardin, V., Christensen, K.H., Dabrowski, T., Janssen, F., Haapala, J., Lars, A., Legrand, S., Lien, V., Lips, I., Maar, M., Mader, J., Morucci, S., Novellino, A., Orfila, A., Petihakis, G., Ruiz-Villarreal, M., Staneva, J., & Triantafyllou, G. (2021). Develop EuroGOOS marine climate service with seamless earth system approach. Proceedings of the 9th EuroGOOS International conference, Shom; Ifremer; EuroGOOS AISBL, May 2021, Brest (Virtual), France. pp. 554–561.
- Doney, S. C., Lima, I., Moore, J. K., Lindsay, K., Behrenfeld, M. J., Westberry, T. K., Mahowald, N., Glover, D. M., & Takahashi, T. (2009). Skill metrics for confronting global upper ocean ecosystem-biogeochemistry models against field and remote sensing data. Journal of Marine Systems, 76(1–2), 95–112. https://doi.org/10.1016/j.jmarsys.2008.05.015
- Döscher, R., Acosta, M., Alessandri, A., Anthoni, P., Arsouze, T., Bergman, T., Bernardello, R., Boussetta, S., Caron, L.-P., Carver, G., Castrillo, M., Catalano, F., Cvijanovic, I., Davini, P., Dekker, E., Doblas-Reyes, F. J., Docquier, D., Echevarria, P. … Zhang, Q. (2022). The EC-Earth3 earth system model for the coupled Model intercomparison project 6. Geoscientific Model Development, 15(7), 2973–3020. https://doi.org/10.5194/gmd-15-2973-2022
- Duncan, B. N., Ott, L. E., Abshire, J. B., Brucker, L., Carroll, M. L., Carton, J., Comiso, J. C., Dinnat, E. P., Forbes, B. C., Gonsamo, A., Gregg, W. W., Hall, D. K., Ialongo, I., Jandt, R., Kahn, R. A., Karpechko, A., Kawa, S. R., Kato, S., Kumpula, T., & Winker, D. M.… Wu, D. L. (2020). Space‐based observations for understanding changes in the arctic‐boreal zone. Reviews of Geophysics, 58(1), 1–95, e2019RG000652. https://doi.org/10.1029/2019RG000652
- Esau, I., Pettersson, L. H., Cancet, M., Chapron, B., Chernokulsky, A., Donlon, C., Sizov, O., Soromotin, A., & Johannesen, J. A. (2023). The arctic amplification and its impact: A synthesis through satellite observations. Remote Sensing, 15(5), 1354. https://doi.org/10.3390/rs15051354
- Galin, V. Y., Smyshlyaev, S. P., & Volodin, E. M. (2010). Combined chemistry-climate model of the atmosphere. Izvestiya Atmospheric and Oceanic Physics, 43(4), 399–412. https://doi.org/10.1134/S0001433807040020
- Gent, P., & McWilliams, J. C. (1990). Isopycnal mixing in ocean circulation models. Journal of Physical Oceanography, 20(1), 150–155. https://doi.org/10.1175/1520-0485(1990)020<0150:IMIOCM>2.0.CO;2
- Gettelman, A., Morrison, H., & Ghan, S. J. (2008). A new two-moment bulk stratiform cloud micro- physics scheme in the NCAR community atmosphere model (CAM3), Part II: Single-column and global results. Journal of Climate, 21(15), 3660–3679. https://doi.org/10.1175/2008JCLI2116.1
- Giorgetta, M. A., Jungclaus, J. H., Reick, C. H., Legutke, S., Brovkin, V., Crueger, T., Esch, M., Fieg, K., Glushak, K., Gayler, V., Haak, H., Hollweg, H.-D., Ilyina, T., Kinne, S., Kornblueh, L., Matei, D., Mauritsen, T., Mikolajewicz, U. … Stevens, B. (2013). Climate and carbon cycle changes from 1850 to 2100 in MPI-ESM simulations for the coupled model intercomparison project phase 5. Journal of Advances in Modeling Earth Systems, 5(3), 572–597. https://doi.org/10.1002/jame.20038
- Goosse, H., Kay, J. E., Armour, K. C., Bodas-Salcedo, A., Chepfer, H., Docquier, D., Jonko, A., Kushner, P. J., Lecomte, O., Massonnet, F., Park, H.-S., Pithan, F., Svensson, G., & Vancoppenolle, M. (2018). Quantifying climate feedbacks in polar regions. Nature Communications, 9(1), 1919. https://doi.org/10.1038/s41467-018-04173-0
- Gordova, Y. E., Yu, E., Genina, V. P., Gorbatenko, E. P., Gordov, I. V., Yu, K., Martynova, V., Okladnikov, I. G., Titov, A. G., Shulgina, T. M., & Barashkova, N. K. (2013). Support of the educational process in modern climatology within the web-GIS platform ”Climate”. Open and Distant Education, 1(49), 14–19.
- Gordov, E. P., Lykosov, V. N., Krupchatnikov, V. N., Okladnikov, I. G., Titov, A. G., & Shulgina, T. M. (2013). Computational and information technologies for monitoring and modeling of climate changes and their consequences. Nauka, Siberian branch.
- Grell, G. A., Peckham, S. E., Schmitz, R., McKeen, S. A., Frost, G., Skamarock, W. C., & Eder, B. (2005). Fully coupled “online” chemistry in the WRF model. Atmospheric Environment, 39(37), 6957–6975. https://doi.org/10.1016/j.atmosenv.2005.04.027
- Gross, A., & Baklanov, A. (2004). Modelling the influence of dimethyl sulphide on aerosol production in the marine boundary layer. International Journal of Environment and Pollution, 22(1–2), 51–71. https://doi.org/10.1504/IJEP.2004.005492
- Grote, R., Mayrhofer, S., Fischbach, R. J., Steinbrecher, R., Staudt, M., & Schnitzler, J.-P. (2006). Process-based modelling of isoprenoid emissions from evergreen leaves of Quercus ilex (L.). Atmospheric Environment, 40, 152–165. https://doi.org/10.1016/J.ATMOSENV.2005.10.071
- Guenther, A., Karl, T., Harley, P., Wiedinmyer, C., Palmer, P. I., & Geron, C. (2006). Estimates of global terrestrial isoprene emissions using MEGAN (model of emissions of gases and aerosols from nature). Atmospheric Chemistry & Physics, 6(11), 3181–3210. https://doi.org/10.5194/acp-6-3181-2006
- Hari, P., Andreae, M. O., Kabat, P., & Kulmala, M. (2009). A comprehensive network of measuring stations to monitor climate change. Boreal Environment Research, 14(4), 442–446. https://www.borenv.net/BER/archive/pdfs/ber14/ber14-442.pdf
- Hari, P., & Kulmala, M. (2005). Stations for measuring ecosystem – atmosphere relations (SMEAR II). Boreal Environment Research, 10(5), 315–322. https://www.borenv.net/BER/archive/pdfs/ber10/ber10-315.pdf
- Hari, P., Petäjä, T., Bäck, J., Kerminen, V.-M., Lappalainen, H. K., Vihma, T., Laurila, T., Viisanen, Y., Vesala, T., & Kulmala, M. (2016). Conceptual design of a measurement network of the global change. Atmospheric Chemistry and Physics, 16(2), 1017–1028. https://doi.org/10.5194/acp-16-1017-2016
- Havlik, P., Schneider, U. A., Schmid, E., Bottcher, H., Fritz, S., Skalsky, R., Aoki, K., De Cara, S., Kindermann, G., Kraxner, F., Leduc, S., McCallum, I., Mosnier, A., Sauer, T., & Obersteiner, M. (2011). Global land-use implications of first and second generation biofuel targets. Energy Policy, 39(10), 5690–5702. https://doi.org/10.1016/j.enpol.2010.03.030
- Hazeleger, W., Severijns, C., Semmler, T., Ştefănescu, S., Yang, S., Wang, X., Wyser, K., Dutra, E., Baldasano, J. M., Bintanja, R., Bougeault, P., Caballero, R., Ekman, A. M. L., Christensen, J. H., van den Hurk, B., Jimenez, P., Jones, C., Kållberg, P. … Willén, U. (2010). EC-Earth: A seamless earth-system prediction approach in action. Bulletin of American Meteorological Society, 91(10), 1357–1364. https://doi.org/10.1175/2010bams2877.1
- Hazeleger, W., Wang, X., Severijns, C., Tefanescu, S. S., Bintanja, R., Sterl, A., Wyser, K., Semmler, T., Yang, S., van den Hurk, B., van Noije, T., van der Linden, E., & van der Wiel, K. (2012). EC-Earth V2: Description and validation of a new seamless Earth system prediction model. Climate Dynamics, 39(11), 2611–2629. https://doi.org/10.1007/s00382-011-1228-5
- Huang, M., Ji, J., Li, K., Liu, Y., Yang, F., & Tao, B. (2007). The ecosystem carbon accumulation after conversion of grasslands to pine plantations in subtropical red soil of South China. Tellus B: Chemical & Physical Meteorology, 59(3), 439–448. https://doi.org/10.1111/j.1600-0889.2007.00280.x
- Huang, Y., Yu, Y., Zhang, W., Sun, W. J., Liu, S. L., Jiang, J., Wu, J. S., Yu, W. T., Wang, Y., & Yang, Z. F. (2009). Agro-C: A biogeophysical model for simulating the carbon budget of agroecosystems. Agricultural and Forest Meteorology, 149(1), 106–129. https://doi.org/10.1016/j.agrformet.2008.07.013
- Huang, Y., Zhang, W., Zheng, X., Li, J., & Yu, Y. (2004). Modeling methane emission from rice paddies with various agricultural practices. Journal of Geophysical Research – Atmospheres, 109(D8). https://doi.org/10.1029/2003JD004401
- Huijnen, V., Williams, J., van Weele, M., van Noije, T., Krol, M., Dentener, F., Segers, A., Houweling, S., Peters, W., de Laat, J., Boersma, F., Bergamaschi, P., van Velthoven, P., Le Sager, P., Eskes, H., Alkemade, F., Scheele, R., Nédélec, P., & Pätz, H.-W. (2010). The global chemistry transport model TM5: Description and evaluation of the tropospheric chemistry version 3.0. Geoscientific Model Development, 3(2), 445–473. https://doi.org/10.5194/gmd-3-445-2010
- Hunke, E. C. (2001). Viscous-plastic sea ice dynamics with the EVP model: Linearization issues. Journal of Computational Physics, 170(1), 18–38. https://doi.org/10.1006/jcph.2001.6710
- Hunke, E. C., & Dukowicz, J. K. (1997). An elastic–viscous–plastic model for sea ice dynamics. Journal of Physical Oceanography, 27(9), 1849–1867. https://doi.org/10.1175/1520-0485(1997)027<1849:AEVPMF>2.0.CO;2
- Hunke, E. C., Lipscomb, W. H., Turner, A. K., Jeffery, N., & Elliot, S. (2013). CICE: The Los Alamos Sea ice Model, documentation and software User’s manual, version 5.0. Los Alamos National Laboratory, LA-CC-06–012. https://svn-ccsm-models.cgd.ucar.edu/cesm1/alphas/branches/cesm1_5_alpha04c_timers/components/cice/src/doc/cicedoc.pdf
- Hurrell, J. W., Holland, M. M., & Gent, P. R. (2013). The community earth system Model: A framework for collaborative research. Bulletin of the American Meteorological Society, 94(9), 1339–1360. https://doi.org/10.1175/BAMS-D-12-00121.1
- Ilıcak, M., Drange, H., Wang, Q., Gerdes, R., Aksenov, Y., Bailey, D., Bentsen, M., Biastoch, A., Bozec, A., Böning, C., Cassou, C., Chassignet, E., Coward, A., Curry, B., Danabasoglu, G., Danilov, S., Fernandez, E., Fogli, P. G. … Yeager, S. (2016). An assessment of the Arctic Ocean in a suite of interannual CORE-II simulations. Part III: Hydrography and fluxes. Ocean Modelling, 100, 141–161. https://doi.org/10.1016/j.ocemod.2016.02.004
- IPCC. (2014). Climate change 2014: Synthesis report. Contribution of Working Groups I, II and III to the Fifth Assessment Report of the Intergovernmental Panel on Climate Change. (AR5)
- Ivanov, V. V., & Golovin, P. N. (2007). Observations and modelling of dense water cascading from the northwestern Laptev Sea Shelf. Journal of Geophysical Research - Oceans, 112(C9). https://doi.org/10.1029/2006JC003882
- Izaurralde, R. C., Williams, J. R., McGill, W. B., Rosenberg, N. J., & Quiroga Jakas, M. C. (2006). Simulating soil C dynamics with EPIC: Model description and testing against long-term data. Ecological Modelling, 192(3–4), 362–384. https://doi.org/10.1016/j.ecolmodel.2005.07.010
- Järvi, L., Grimmond, C. S. B., & Christen, A. (2011). The Surface urban Energy and water balance scheme (SUEWS): Evaluation in Los Angeles and Vancouver. Journal of Hydrology, 411(3–4), 219–237. https://doi.org/10.1016/j.jhydrol.2011.10.001
- Jochum, M., Jahn, A., Peacock, S., Bailey, D. A., Fasullo, J. T., Kay, J., Levis, S., & Otto-Bliesner, B. (2012). True to Milankovitch: Glacial inception in the new community climate system model. Journal of Climate, 25(7), 2226–2239. https://doi.org/10.1175/JCLI-D-11-00044.1
- Junninen, H., Lauri, A., Keronen, P., Aalto, P., Hiltunen, V., Hari, P., & Kulmala, M. (2009). Smart-SMEAR: On-line data exploration and visualization tool for SMEAR stations. Boreal Environment Research, 14(4), 447–457. https://www.borenv.net/BER/archive/pdfs/ber14/ber14-447.pdf
- Kaas, E. (2008). A simple and efficient locally mass conserving semi-Lagrangian transport scheme. Tellus A: Dynamic Meteorology and Oceanography, 60(2), 305–320. https://doi.org/10.1111/j.1600-0870.2007.00293.x
- Karsisto, P., Fortelius, C., Demuzere, M., Grimmond, C. S. B., Oleson, K. W., Kouznetsov, R. D., Masson, V., & Järvi, L. (2015). Seasonal surface urban energy balance and wintertime stability simulated using three land-surface models in the high-latitude city Helsinki. Quarterly Journal of the Royal Meteorological Society, 142(694), 401–417. https://doi.org/10.1002/qj.2659
- Kokkola, H., Korhonen, H., Lehtinen, K. E. J., Makkonen, R., Asmi, A., Järvenoja, S., Anttila, T., Partanen, A.-I., Kulmala, M., Järvinen, H., Laaksonen, A., & Kerminen, V.-M. (2008). SALSA – a Sectional Aerosol model for Large Scale Applications. Atmospheric Chemistry & Physics, 8(9), 2469–2483. https://doi.org/10.5194/acp-8-2469-2008
- Kokkola, H., Kühn, T., Laakso, A., Bergman, T., Lehtinen, K. E. J., Mielonen, T., Arola, A., Stadtler, S., Korhonen, H., Ferrachat, S., Lohmann, U., Neubauer, D., Tegen, I., Siegenthaler Le Drian, C., Schultz, M. G., Bey, I., Stier, P., Daskalakis, N., Heald, C. L., & Romakkaniemi, S. (2018). SALSA2.0: The sectional aerosol module of the aerosol–chemistry–climate model ECHAM6.3.0-HAM2.3-MOZ1.0. Geoscientific Model Development, 11(9), 3833–3863. https://doi.org/10.5194/gmd-11-3833-2018
- Korsholm, U. S. (2009). Integrated Modeling of Aerosol Indirect Effects. Ph.D. thesis, University of Copenhagen, Niels Bohr Institute and Danish Meteorological Institute,
- Korsholm, U. S., Baklanov, A., Gross, A., Mahura, A., Sass, B. H., & Kaas, E. (2008). Online coupled chemical weather forecasting based on HIRLAM – overview and prospective of enviro-HIRLAM. HIRLAM Newsletter, (54), 151–168.
- Kulmala, M. (2018). Build a global earth observatory. Nature, 553(7686), 21–23. https://doi.org/10.1038/d41586-017-08967-y
- Kulmala, M., Lappalainen, H. K., Petäjä, T., Kerminen, V., Viisanen, Y., Matvienko, G., Melnikov, V., Baklanov, A., Bondur, V., Kasimov, N., & Zilitinkevich, S. (2016). Pan-Eurasian Experiment (PEEX) program: Grand Challenges in the Arctic-Boreal Context. Geography, Environment, Sustainability, 9(2), 5–18. https://doi.org/10.15356/2071-9388_02v09_2016_01
- Kulmala, M., Lappalainen, H. K., Petäjä, T., Kurten, T., Kerminen, V.-M., Viisanen, Y., Hari, P., Baklanov, V., Matvienko, N., Bondur, V., Kasimov, G., Kotlyakov, A., Guo, H., Zilitinkevich, A., Hansson, H.-C., & Ding, S. (2015). Introduction: The Pan-Eurasian Experiment (PEEX) – multidisciplinary, multiscale and multicomponent research and capacity-building initiative. Atmospheric Chemistry and Physics, 15(22), 13085–13096. https://doi.org/10.5194/acp-15-13085-2015
- Kurppa, M., Hellsten, A., Auvinen, M., Vesala, T., Raasch, S., & Järvi, L. (2018). The influence of city-block arrangement on pollutant ventilation and air quality: A large-eddy simulation study. Atmosphere, 9(2), 65. https://doi.org/10.3390/atmos9020065
- Kurppa, M., Hellsten, A., Roldin, P., Kokkola, H., Tonttila, J., Auvinen, M., Kent, C., Kumar, P., Maronga, B., & Järvi, L. (2019). Implementation of the sectional aerosol module SALSA2.0 into the PALM model system 6.0: Model development and first evaluation. Geoscientific Model Development, 12(4), 1403–1422. https://doi.org/10.5194/gmd-12-1403-2019
- Lamarque, J.-F., Emmons, L. K., Hess, P. G., Kinnison, D. E., Tilmes, S., Vitt, F., Heald, C. L., Holland, E. A., Lauritzen, P. H., Neu, J., Orlando, J. J., Rasch, P. J., & Tyndall, G. K. (2012). CAM-chem: Description and evaluation of interactive atmospheric chemistry in the community Earth system model. Geoscientific Model Development, 5(2), 369–411. https://doi.org/10.5194/gmd-5-369-2012
- Lappalainen, H. K., Kerminen, V.-M., Petäjä, T., Kurten, T., Baklanov, A., Shvidenko, A., Bäck, J., Vihma, T., Alekseychik, P., Andreae, M. O., Arnold, S. R., Arshinov, M., Asmi, E., Belan, B., Bobylev, L., Chalov, S., Cheng, Y., Chubarova, N. … Kulmala, M. (2016). Pan-Eurasian Experiment (PEEX): Towards a holistic understanding of the feedbacks and interactions in the land–atmosphere–ocean–society continuum in the northern Eurasian region. Atmospheric Chemistry & Physics, 16(22), 14421–14461. https://doi.org/10.5194/acp-16-14421-2016
- Lappalainen, H. K., Kulmala, M., Kujansuu, J., Petäjä, T., Mahura, A., de Leeuw, G., Zilitinkevich, S., Juustila, M., Kerminen, V.-M., Bornstein, B., Jiahua, Z., Yong, X., Yubao, Q., Dong, L., Jie, L., & Huadong, G. (2018). The silk road agenda of the Pan-Eurasian Experiment (PEEX) program. Big Earth Data, 2(1), 8–35. https://doi.org/10.1080/20964471.2018.1437704
- Lappalainen, H. K., Petäjä, T., Vihma, T., Räisänen, J., Baklanov, A., Chalov, S., Esau, I., Ezhova, E., Leppäranta, M., Pozdnyakov, D., Pumpanen, J., Andreae, M. O., Arshinov, M., Asmi, E., Bai, J., Bashmachnikov, I., Belan, B., Bianchi, F. … Kulmala, M. (2022). Overview: Recent advances in the understanding of the northern Eurasian environments and of the urban air quality in China – a Pan-Eurasian Experiment (PEEX) programme perspective. Atmospheric Chemistry & Physics, 22(7), 4413–4469. https://doi.org/10.5194/acp-22-4413-2022
- Large, W., McWilliams, J., & Doney, S. (1994). Oceanic vertical mixing: A review and a model with a nonlocal boundary layer parameterization. Reviews of Geophysics, 32(4), 363–403. https://doi.org/10.1029/94RG01872
- Lenton, T. M., Held, H., Klegler, B., Hall, J. W., Lucht, W., Rahmstort, S., & Schellnhuber, H. J. (2008). Tipping elements of the Earth’s climate system. Proceedings of the National Academy of Sciences, 105(6), 1786–1793. https://doi.org/10.1073/pnas.0705414105
- Liu, X., & Ghan, S. (2010). A modal aerosol model implementation in the Community Atmosphere Model, version 5 (CAM5). Journal of Atmospheric Sciences.
- Li, S., Zhang, W., Zheng, X., Li, Y., Han, S., Wang, R., Wang, K., Yao, Z., Liu, C., & Zhang, C. (2022). Update of a biogeochemical model with process-based algorithms to predict ammonia volatilization from fertilized cultivated uplands and rice paddy fields. Biogeosciences, 19(12), 3001–3019. https://doi.org/10.5194/bg-19-3001-2022
- Li, S., Zhu, B., Zheng, X., Hu, P., Han, S., Fan, J., Wang, T., Wang, R., Wang, K., Yao, Z., Liu, C., Zhang, W., & Li, Y. (2023). Enabling a process-oriented hydro-biogeochemical model to simulate soil erosion and nutrient losses. Biogeosciences, 20(16), 3555–3572. https://doi.org/10.5194/bg-20-3555-2023
- Madec, G., & the NEMO team. (2008). NEMO ocean engine. Note du Pˆole de mod´elisation, Institut Pierre-Simon Laplace (IPSL), France, No 27. Institut Pierre-Simon Laplace.
- Madsen, K. S., Rasmussen, T. A. S., Ribergaard, M. H., & Ringgaard, M. (2016). High resolution sea-ice modelling and validation of the Arctic with focus on South Greenland waters, 2004–2013. Polarforschung, Bremerhaven, Alfred Wegener Institute for Polar and Marine Research & German Society of Polar Research, 85(2), 101–105. https://doi.org/10.2312/polfor.2016.006
- Mahura, A., Amstrup, B., Nuterman, R., Yang, X., & Baklanov, A. (2017). Multi-Scale Enviro-HIRLAM Forecasting of Weather and Atmospheric Composition over China and its Megacities. Geophysical Research Abstracts, 19, EGU2017–9564.
- Mahura, A., & Baklanov, A. (2004). Probabilistic indicators of atmospheric transport for regional monitoring and emergency preparedness systems. Environment International, 29(8), 1063‐1069. https://doi.org/10.1016/S0160-4120(03)00100-4
- Mahura, A., Baklanov, A., & Sørensen, J. H. (2009). Estimation of Potential Impact on Copenhagen, Denmark, due to Accidental Releases at Nuclear Risk Sites. International Journal of Environment and Pollution, 39(1‐2), 159‐167. https://doi.org/10.1504/IJEP.2009.027149
- Mahura, A. G., Baklanov, A., Sørensen, J. H., Parker, F. L., Novikov, V., Brown, K., & Compton, K. L. (2005). Assessment of potential atmospheric transport and deposition patterns due to Russian pacific fleet operations. Environmental Monitoring and Assessment, 101(1‐3), 261–287. https://doi.org/10.1007/s10661-005-0295-7
- Mahura, A., Gonzalez-Aparicio, I., Nuterman, R., & Baklanov, A. (2018). Seasonal impact analysis on population due to continuous sulphur emissions from severonikel smelters of the Kola Peninsula. Geography, Environment, Sustainability, 11(1), 130–144. https://doi.org/10.24057/2071-9388-2018-11-1-130-144
- Mahura, A., Petersen, C., Baklanov, A., & Amstrup, B. (2008). Evaluation of building effect parameterization module for urbanized numerical weather prediction modelling. In K. Klysika, J. Wibig & K. Fortuniaka (Eds.), Urban Climate and Bioclimate (pp. 371–380). Univesity of Lodz.
- Mahura, A., Petersen, C., Baklanov, A., Amstrup, B., Korsholm, U. S., & Sattler, K. (2008). Verification of long-term DMI-HIRLAM NWP Peninsurbanisation and building effect parameterization modules. HIRLAM Newsletter, (53), 50–60.
- Malguzzi, P., Buzzi, A., & Drofa, O. (2011). The meteorological global model GLOBO at the ISAC-CNR of Italy assessment of 1.5 yr of experimental use for medium-range weather forecasts. Weather and Forecasting, 26(6), 1045–1055. https://doi.org/10.1175/WAF-D-11-00027.1
- Maronga, B., Banzhaf, S., Burmeister, C., Esch, T., Forkel, R., Fröhlich, D., Fuka, V., Gehrke, K. F., Geletič, J., Giersch, S., Gronemeier, T., Groß, G., Heldens, W., Hellsten, A., Hoffmann, F., Inagaki, A., Kadasch, E., Kanani-Sühring, F. … Raasch, S. (2020). Overview of the PALM model system 6.0. Geoscientific Model Development, 13(3), 1335–1372. https://doi.org/10.5194/gmd-13-1335-2020
- Maronga, B., Gryschka, M., Heinze, R., Hoffmann, F., Kanani-Sühring, F., Keck, M., Ketelsen, K., Letzel, M. O., Sühring, M., & Raasch, S. (2015). The Parallelized large-eddy simulation model (PALM) version 4.0 for atmospheric and oceanic flows: Model formulation, recent developments, and future perspectives. Geoscientific Model Development, 8(8), 2515–2551. https://doi.org/10.5194/gmd-8-2515-2015
- Medvedeva, A. Y., Arkhipkin, V. S., Myslenkov, S. A., & Zilitinkevich, S. S. (2015). Wave climate of the Baltic Sea following the results of the SWAN spectral model application. Moscow University Bulletin, Series 5, Geography, 1, 12–22. https://vestnik5.geogr.msu.ru/jour/article/view/49/50
- Miles, V., Esau, I., & Pettersson, L. H. (2023). Using web GIS to promote stakeholder understanding of scientific results in sustainable urban development: A case study in Bergen, Norway. Sustainable Development. https://doi.org/10.1002/sd.2787
- Mogensen, D., Smolander, S., Sogachev, A., Zhou, L., Sinha, V., Guenther, A., Williams, J., Nieminen, T., Kajos, M. K., Rinne, J., Kulmala, M., & Boy, M. (2011). Modelling atmospheric OH-reactivity in a boreal forest ecosystem. Atmospheric Chemistry and Physics, 11(18), 9709–9719. https://doi.org/10.5194/acp-11-9709-2011
- Moore, J. K., Doney, S., Kleypas, J., Glover, D., & Fung, I. (2002). An intermediate complexity marine ecosystem model for the global domain. Deep sea research part II. Deep Sea Research Part II: Topical Studies in Oceanography, 49(1–3), 403–462. https://doi.org/10.1016/S0967-0645(01)00108-4
- Moore, J. K., Doney, S. C., & Lindsay, K. (2004). Upper ocean ecosystem dynamics and iron cycling in a global three-dimensional model. Global Biogeochemical Cycles, 18(4). https://doi.org/10.1029/2004GB002220
- Morrison, H., & Gettelman, A. (2008). A new two-moment bulk stratiform cloud microphysics scheme in the NCAR community atmosphere Model (CAM3), part I: Description and numerical tests. Journal of Climate, 21(15), 3642–3659. https://doi.org/10.1175/2008JCLI2105.1
- Myslenkov, S. A., Golubkin, P. A., & Zabolotskikh, E. V. (2016). Evaluation of wave model in the Barents Sea under winter cyclone conditions. Moscow University Bulletin, Series 5, Geography, 6, 26–32. https://vestnik5.geogr.msu.ru/jour/article/view/255/256
- Neale, R., Chen, C.-C., Gettelman, A., Lauritzen, P. H., Park, S., Williamson, D. L., Conley, A. J., Garcia, R., Kinnison, D., Lamarque, J.-F., Marsh, D., Mills, M., Smith, A. K., Tilmes, S., Vitt, F., Morrison, H., Cameron-Smith, P., Collins, W. D. … Taylor, M. (2012). Description of the NCAR community atmosphere Model (CAM5.0). NCAR/TN-486+str, NCAR Technical Note, 283.
- Nikiforov, Y., & Shpaikher, A. (1980). Features of the formation of hydrological regime large scale variations in the Arctic Ocean. Hydrometeoizdat.
- Nuterman, R., Korsholm, U., Zakey, A., Nielsen, K. P., Sørensen, B., Mahura, A., Rasmussen, A., Mažeikis, A., Gonzalez-Aparicio, I., Morozova, E., Sass, B. H., Kaas, E., & Baklanov, A. (2013). New developments in Eviro-HIRLAM online integrated modeling system. Geophysical research abstracts, 15, EGU2013–12520–1.
- Nuterman, R., Mahura, A., Baklanov, A., Kurganskiy, A., Amstrup, B., & Kass, E. (2015). Enviro-HIRLAM applicability for black carbon studies in Arctic. Geophysical Research Abstracts, 17, EGU2015-1571.
- Okladnikov, I. G., Titov, A. G., Shulgina, T. M., Gordov, E. P., Yu, V., BogomolovYu, V. M., Suschenko, S. P., & Skvortsov, A. V. (2013). Software for analysis and visualization of climate change monitoring and forecasting data. Journal Numerical Methods and Programming, 14, 123–131 https://en.num-meth.ru/index.php/journal.
- Overland, J., Francis, J. A., Hall, R., Hanna, E., Kim, S., & Vihma, T. (2015). The melting arctic and midlatitude weather patterns: Are they connected? Journal of Climate, 28(20), 7917–7932. https://doi.org/10.1175/JCLI-D-14-00822.1
- PEEX. (2015). Pan-Eurasian (PEEX) science plan. H. K. Lappalainen, M. Kulmala, S. Zilitinkevich. Eds., University of Helsinki, Helsinki.
- Penenko, V., Baklanov, A., Tsvetova, E., & Mahura, A. (2012). Direct and inverse problems in a variational concept of environmental modelling. Pure and Applied Geophysics, 169(3), 447–465. https://doi.org/10.1007/s00024-011-0380-5
- Penenko, A. V., Penenko, V. V., & Tsvetova, E. A. (2016). Sequential data assimilation algorithms for air quality monitoring models based on a weak-constraint variational principle. Numerical Analysis & Applications, 9(4), 312–325. https://doi.org/10.1134/s1995423916040054
- Penenko, V. V., Penenko, A. V., & Tsvetova, E. A. (2017). Variational approach to the study of processes of geophysical hydro-thermodynamics with assimilation of observation data. Journal of Applied Mechanics and Technical Physics, 58(5), 771–778. https://doi.org/10.1134/S0021894417050029
- Penenko, A., Penenko, V., Tsvetova, E., Grishina, A., & Antokhin, P. (2018). Sequential variational data assimilation algorithms at the splitting stages of a numerical atmospheric chemistry Model. Large-Scale Scientific Computing, Springer International Publishing. https://doi.org/10.1007/978-3-319-73441-5_59
- Penenko, V., & Tsvetova, E. (2008). Orthogonal decomposition methods for inclusion of climatic data into environmental studies. Ecological Modelling, 217(3–4), 279–291. https://doi.org/10.1016/j.ecolmodel.2008.06.004
- Penenko, V. V., Tsvetova, E. A., & Penenko, A. V. (2015a). Development of variational approach for direct and inverse problems of atmospheric hydrodynamics and chemistry. Izvestiya, Atmospheric and Oceanic Physics, 51(3), 311–319. https://doi.org/10.1134/S0001433815030093
- Penenko, V. V., Tsvetova, E. A., & Penenko, A. V. (2015b). Methods based on the joint use of models and observational data in the framework of variational approach to forecasting weather and atmospheric composition quality. Russian Meteorology and Hydrology, 40(6), 365–373. https://doi.org/10.3103/S1068373915060023
- Petäjä, T., Ganzei, K. S., Lappalainen, H. K., Tabakova, K., Makkonen, R., Räisänen, J., Chalov, S., Kulmala, M., Zilitinkevich, S., Ya, P., Baklanov, R. B. S., Mishina, N. V., Egidarev, E. G., & Kondrat’ev, I. I. (2021). Research agenda for the Russian Far East and utilization of multi-platform comprehensive environmental observations. International Journal of Digital Earth, 14(3), 311–337. https://doi.org/10.1080/17538947.2020.1826589
- Peters, W., Krol, M. C., van der Werf, G. R., Houweling, S., Jones, C. D., Hughes, J., Schaefer, K., Masarie, K. A., Jacobson, A. R., Miller, J. B., Cho, C. H., Ramonet, M., Schmidt, M., Ciattaglia, L., Apadula, F., Heltai, D., Meinhardt, F., DiSarra, A. G. … Tans, P. P. (2010). Seven years of recent European net terrestrial carbon dioxide exchange constrained by atmospheric observations. Global Change Biology, 16(4), 1317–1337. https://doi.org/10.1111/j.1365-2486.2009.02078.x
- Ponsoni, L., Ribergaard, M. H., Nielsen-Englyst, P., Wulf, T., Buus-Hinkler, J., Kreiner, M. B., & Rasmussen, T. A. S. (2023). Greenlandic sea ice products with a focus on an updated operational forecast system. Frontiers in Marine Science, 10. https://doi.org/10.3389/fmars.2023.979782
- Poulsen, M. B., Jochum, M., & Nuterman, R. (2018). Parameterized and resolved Southern ocean eddy compensation. Ocean Modelling, 124, 1–15. https://doi.org/10.1016/j.ocemod.2018.01.008
- Previdi, M., Smith, K. L., & Polvani, L. M. (2021). Arctic amplification of climate change: A review of underlying mechanisms. Environmental Research Letters, 16(9), 093003. https://doi.org/10.1088/1748-9326/ac1c29
- Pulliainen, J., Aurela, M., Laurila, T., Aalto, T., Takala, M., Salminen, M., Kulmala, M., Barr, A., Heimann, M., Lindroth, A., Laaksonen, A., Derksen, C., Mäkelä, A., Markkanen, T., Lemmetyinen, J., Susiluoto, J., Dengel, S., Mammarella, I., Tuovinen, J.-P., & Vesala, T. (2017). Early snowmelt significantly enhances boreal springtime carbon uptake. Proceedings of the National Academy of Sciences, 114(42), 11081–11086. https://doi.org/10.1073/pnas.1707889114
- Pyanova, E. A., Penenko, V. V., & Faleychik, L. M. (2014). Simulation of atmospheric dynamics and air quality in the Baikal region, Proceedings SPIE 9292, 20th International Symposium on Atmospheric and Ocean Optics. Atmospheric Physics, 929247 25 Nov (2014); https://doi.org/10.1117/12.2074998
- Rametsteiner, E., Nilsson, S., Bottcher, H., Havlik, P., Kraxner, F., Leduc, S., Obersteiner, M., Rydzak, F., Schneider, U., Schwab, D., & Willmore, L. (2007). Study of the effects of globalization on the economic viability of EU Forestry. Final Report of the AGRI Tender Project: AGRI-G4-2006-06, IIASA, 16p.
- Rantanen, M., Karpechko, A. Y., Lipponen, A., Nordling, K., Hyvärinen, O., Ruosteenoja, K., Vihma, T., & Laaksonen, A. (2022). The Arctic has warmed nearly four times faster than the globe since 1979. Communications Earth & Environment, 3(1), 168. https://doi.org/10.1038/s43247-022-00498-3
- Redler, R., Valcke, S., & Ritzdorf, H. (2010). OASIS4 – a coupling software for next generation earth system modelling. Geoscientific Model Development, 3(1), 87–104. https://doi.org/10.5194/gmd-3-87-2010
- Richards, N. A. D., Arnold, S. R., Chipperfield, M. P., Miles, G., Rap, A., Siddans, R., Monks, S. A., & Hollaway, M. J. (2013). The Mediterranean summertime ozone maximum: Global emission sensitivities and radiative impacts. Atmospheric Chemistry and Physics, 13(5), 2331–2345. https://doi.org/10.5194/acp-13-2331-2013
- Rousset, C., Vancoppenolle, M., Madec, G., Fichefet, T., Flavoni, S., Barthélemy, A., Benshila, R., Chanut, J., Levy, C., Masson, S., & Vivier, F. (2015). The Louvain-La-Neuve sea ice model LIM3.6: global and regional capabilities. Geoscientific Model Development, 8(10), 2991–3005. https://doi.org/10.5194/gmd-8-2991-2015
- Sand, M., Berntsen, T. K., von Salzen, K., Flanner, M. G., Langner, J., & Victor, D. G. (2016). Response of Arctic temperature to changes in emissions of short-lived climate forcers. Nature Climate Change, 6(3), 286–289. https://doi.org/10.1038/nclimate2880
- Scott, C. E., Arnold, S. R., Monks, S. A., Asmi, A., Paasonen, P., & Spracklen, D. V. (2018). Substantial large-scale feedbacks between natural aerosols and climate. Nature Geoscience, 11(1), 44–48. https://doi.org/10.1038/s41561-017-0020-5
- She, J., Berg, P., & Berg, J. (2007). Bathymetry impacts on water exchange modelling through the Danish straits. Journal of Marine Systems, 65(1–4), 450–459. https://doi.org/10.1016/j.jmarsys.2006.01.017
- She, J., Christensen, A., Garaventa, F., Lips, U., Murawski, J., Ntoumas, M., & Tsiaras, K. (2023). Developing realistic models for assessing marine plastic pollution in semi-enclosed seas. In Eds. E. S. Kappel, V. Cullen, M. J. Costello, L. Galgani, C. Gordó-Vilaseca, A. Govindarajan, S. Kouhi, C. Lavin, L. McCartin, J. D. Müller, B. Pirenne, T. Tanhua, Q. Zhao, & S. Zhao. Frontiers in ocean observing: Emerging technologies for understanding and managing a changing ocean (pp. 54–57). Oceanography, 36 (Supplement 1) https://doi.org/10.5670/oceanog.2023.s1.17
- She, J., & Murawski, J. (2018). Towards seamless modelling for the Baltic Sea. In Operational Oceanography serving Sustainable Marine Development. Proceedings of the Eight EuroGOOS International Conference, 3-5 October 2017, N. E. B. Bergen, V. Fernández, D. Eparkhina, P. Gorringe, & G. Nolan (Eds.) EuroGOOS. Brussels, Belgium. ISBN 978-2-9601883-3-2. 516 pp. p233–241
- Shtabkin Yu, A., Moiseenko, K. B., Skorokhod, A. I., Vasileva, A. V., & Heimann, M. (2016). Sources of and variations in tropospheric CO in central Siberia: Numerical experiments and observations at the zotino tall tower observatory. Izvestiya, Atmospheric and Oceanic Physics, 52(1), 45–56. https://doi.org/10.1134/S0001433816010096
- Shulgina, T. M., Gordov, E. P., Okladnikov, I. G., Titov, A. G., Yu, E., Genina, N. P., Kuzhevskaya, G. I. V., & Akhmetshina, A. S. (2013). Software complex for a regional climate change analysis. Vestnik NGU, Series: Information Technologies, 11(1), 124–131.
- Shvidenko, A., & Schepaschenko, D. (2014). Carbon Budget of Russian forests. Siberian Journal of Forest Science, 1, 69–92. https://pure.iiasa.ac.at/10782/1/0fd7fb4421fd068bc0f1b9b578ad5d55.pdf
- Shvidenko, A., Schepaschenko, D., McCallum, I., & Nilsson, S. (2010). Can the uncertainty of full carbon accounting of forest ecosystems be made acceptable to policy makers? Climatic Change, 103(1–2), 137–157. https://doi.org/10.1007/s10584-010-9918-2
- Shvidenko, A., Shepasenko, D., Kraxner, F., & Fritz, S. (2015). Full verified carbon account of forest ecosytems as a fuzzy system: An attempt to assess uncertainty. In Proceedings of 4th International Workshop on Uncertainty in Atmospheric Emissions, 7-9 October, Krakow, Poland, рр.1–8
- Small, R. J., Bacmeister, J., Bailey, D., Baker, A., Bishop, S., Bryan, F., Caron, J., Dennis, J., Gent, P., Hsu, H.-M., Jochum, M., Lawrence, D., Muñoz, E., diNezio, P., Scheitlin, T., Tomas, R., Tribbia, J., Tseng, Y.-H., & Vertenstein, M. (2014). A new synoptic scale resolving global climate simulation using the community earth system Model. Journal of Advances in Modeling Earth Systems, 6(4), 1065–1094. https://doi.org/10.1002/2014MS000363
- Smith, S. L., O’Neill, H. B., Isaksen, K., Noetzli, J., & Romanovsky, V. E. (2022). The changing thermal state of permafrost. Nature Reviews Earth and Environment, 3(1), 10–23. https://doi.org/10.1038/s43017-021-00240-1
- Smith, B., Prentice, I. C., & Sykes, M. T. (2001). Representation of vegetation dynamics in the modelling of terrestrial ecosystems: Comparing two contrasting approaches within European climate space. Global Ecology and Biogeography, 10(6), 621–637. https://doi.org/10.1046/j.1466-822X.2001.t01-1-00256.x
- Smolander, S., He, Q., Mogensen, D., Zhou, L., Bäck, J., Ruuskanen, T., Noe, S., Guenther, A., Aaltonen, H., Kulmala, M., & Boy, M. (2014). Comparing three vegetation monoterpene emission models to measured gas concentrations with a Model of meteorology. Air Chemistry and Chemical Transport Biogeosciences, 11(19), 5425–5443. https://doi.org/10.5194/bg-11-5425-2014
- Smyshlyaev, S. P., Galin, V. Y., Shaariibuu, G., & Motsakov, M. A. (2010). Modeling the variability of gas and Aerosol Components in the stratosphere of polar regions. Izvestiya, Atmospheric and Oceanic Physics, 46(3), 265–280. https://doi.org/10.1134/S0001433810030011
- Smyshlyaev, S. P., Mareev, E. A., & Galin, V. Y. (2010). Simulation of the impact of thunderstorm activity on atmospheric gas composition. Izvestiya, Atmospheric and Oceanic Physics, 46(4), 451–467. https://doi.org/10.1134/S0001433810040043
- Sofiev, M., Vira, J., Kouznetsov, R., Prank, M., Soares, J., & Genikhovich, E. (2015). Construction of the SILAM Eulerian atmospheric dispersion model based on the advection algorithm of Michael Galperin. Geoscientific Model Development, 8(11), 3497–3522. https://doi.org/10.5194/gmd-8-3497-2015
- Sørensen, H. S., Baklanov, A., & Hoe, S. (2007). The Danish emergency response Model of the atmosphere (DERMA). Journal of Environmental Radioactivity, 96(1–3), 122–129. https://doi.org/10.1016/j.jenvrad.2007.01.030
- Sørensen, B., Kaas, E., & Korsholm, U. S. (2013). A mass-conserving and multi-tracer efficient transport scheme in the online integrated enviro-HIRLAM model. Geoscientific Model Development, 6(4), 1029–1042. https://doi.org/10.5194/gmd-6-1029-2013
- Spracklen, D. V., Pringle, K. J., Carslaw, K. S., Chipperfield, M. P., & Mann, G. W. (2005). A global offline model of size-resolved aerosol microphysics: I. Model development and prediction of aerosol properties. Atmospheric Chemistry and Physics, 5(8), 2227–2252. https://doi.org/10.5194/acp-5-2227-2005
- Stohl, A., Forster, C., Frank, A., Seibert, P., & Wotawa, G. (2005). Technical note: The Lagrangian particle dispersion model FLEXPART version 6.2. Atmospheric Chemistry and Physics, 5(9), 2461–2474. https://doi.org/10.5194/acp-5-2461-2005
- Taylor, P. C., Boeke, R. C., Boisvert, L. N., Feldl, N., Henry, M., Huang, Y., Langen, P. L., Liu, W., Pithan, F., Sejas, S. A., & Tan, I. (2022). Process drivers, inter-model spread, and the path forward: A review of amplified arctic warming. Frontiers in Earth Science, 9. https://doi.org/10.3389/feart.2021.758361
- Tettamanti, R., Malguzzi, P., & Zardi, D. (2002). Numerical simulation of katabatic winds with a non-hydrostatic meteorological model. Polar Atmospheres. 1, 1–95. ISSN 1591-3902.
- Tonttila, J., Maalick, Z., Raatikainen, T., Kokkola, H., Kühn, T., & Romakkaniemi, S. (2017). UCLALES–SALSA v1.0: A large-eddy model with interactive sectional microphysics for aerosol, clouds and precipitation. Geoscientific Model Development, 10(1), 169–188. https://doi.org/10.5194/gmd-10-169-2017
- Tsamados, M., Feltham, D. L., & Wilchinsky, A. V. (2013). Impact of a new anisotropic rheology on simulations of arctic sea ice. Journal Geophysical Research - Oceans, 118(1), 91–107. https://doi.org/10.1029/2012JC007990
- Tsuruta, A., Aalto, T., Backman, L., Hakkarainen, J., van der Laan-Luijkx, I. T., Krol, M. C., Spahni, R., Houweling, S., Laine, M., Dlugokencky, E., Gomez-Pelaez, A. J., van der Schoot, M., Langenfelds, R., Ellul, R., Arduini, J., Apadula, F., Gerbig, C., Feist, D. G. … Peters, W. (2017). Global methane emission estimates for 2000-2012 from CarbonTracker Europe-CH4 v1.0. Geoscientific Model Development, 10(3), 1261–1289. https://doi.org/10.5194/gmd-10-1261-2017
- Tyukavina, A., Potapov, P., Hansen, M. C., Pickens, A. H., Stehman, S. V., Turubanova, S., Parker, D., Zalles, V., Lima, A., Kommareddy, I., Song, X.-P., Wang, L., & Harris, N. (2022). Global trend of forest loss due to fire from 2001 to 2019. Frontiers in Remote Sensing, 3. https://doi.org/10.3389/frsen.2022.825190
- Vancoppenolle, M., Fichefet, T., Goosse, H., Bouillon, S., Madec, G., & Morales Maqueda, M. A. (2009). Simulating the mass balance and salinity of Arctic and Antarctic sea ice. 1. Model description and validation. Ocean Modelling, 27(1–2), 33–53. https://doi.org/10.1016/j.ocemod.2008.10.005
- van Noije, T., Bergman, T., Le Sager, P., O’Donnell, D., Makkonen, R., Gonçalves-Ageitos, M., Döscher, R., Fladrich, U., von Hardenberg, J., Keskinen, J.-P., Korhonen, H., Laakso, A., Myriokefalitakis, S., Ollinaho, P., Pérez García-Pando, C., Reerink, T., Schrödner, R., Wyser, K., & Yang, S. (2021). EC-Earth3-AerChem: A global climate model with interactive aerosols and atmospheric chemistry participating in CMIP6. Geoscientific Model Development, 14(9), 5637–5668. https://doi.org/10.5194/gmd-14-5637-2021
- van Noije, T. P. C., Le Sager, P., Segers, A. J., van Velthoven, P. F. J., Krol, M. C., Hazeleger, W., Williams, A. G., & Chambers, S. D. (2014). Simulation of tropospheric chemistry and aerosols with the climate model EC-Earth. Geoscientific Model Development, 7(5), 2435–2475. https://doi.org/10.5194/gmd-7-2435-2014
- Vignati, E., Wilson, J., & Stier, P. (2004). M7: An efficient size-resolved aerosol microphysics module for large-scale aerosol transport models. Journal of Geophysical Research - Atmospheres, 109(D22). https://doi.org/10.1029/2003JD004485
- Vihma, T., Uotila, P., Sandven, S., Pozdnyakov, D., Makshtas, A., Pelyasov, A., Pirazzini, R., Danielsen, F., Chalov, S., Lappalainen, H. K., Ivanov, V., Frolov, I., Albin, A., Cheng, B., Dobrolyubov, S., Arkhipkin, V., Mуslenkov, S., Petäjä, T., & Kulmala, M. (2019). Towards an advanced observation system for the marine Arctic in the framework of the Pan-Eurasian Experiment (PEEX). Atmospheric Chemistry and Physics, 19(3), 1941–1970. https://doi.org/10.5194/acp-19-1941-2019
- Ward, H., Kotthaus, S., Järvi, L., & Grimmond, C. S. B. (2016). Surface urban energy and water balance scheme (SUEWS): Development and evaluation at two UK sites. Urban Climate, 18, 1–32. https://doi.org/10.1016/j.uclim.2016.05.001
- Wilchinsky, A. V., & Feltham, D. (2006). Modelling the rheology of sea ice as a collection of diamond-shaped floes. Journal of Non-Newtonian Fluid Mechanics, 138(1), 22–32. https://doi.org/10.1016/j.jnnfm.2006.05.001
- Wolf-Grosse, T., Esau, I., & Reuder, J. (2017). Sensitivity of local air quality to the interplay between small- and large-scale circulations: A large-eddy simulation study. Atmospheric Chemistry and Physics, 17(11), 7261–7276. https://doi.org/10.5194/acp-17-7261-2017
- Wolf, T., Pettersson, L. H., & Esau, I. (2020). A very high-resolution assessment and modelling of urban air quality. Atmospheric Chemistry and Physics, 20(2), 625–647. https://doi.org/10.5194/acp-20-625-2020
- Wolf, T., Pettersson, L. H., & Esau, I. (2021). Dispersion of particulate matter (PM2.5) from wood combustion for residential heating: Optimization of mitigation actions based on large-eddy simulations. Atmospheric Chemistry and Physics, 21(16), 12463–12477. https://doi.org/10.5194/acp-21-12463-2021
- WWRP. (2015). Seamless prediction of the earth system: From minutes to months. WMO-No. 1156, 483p., ISBN 978-92-63-11156-2
- Yantosca, B., Sulprizio, M., Lundgren, L., Xu, J., & GEOS-Chem Support Team. (2016). GEOS-Chem v11-01 Online User’s Guide, http://acmg.seas.harvard.edu/geos/doc/man/;Dec2016
- Zhang, W., Li, Y., Zhu, B., Zheng, X., Liu, C., Tang, J., Su, F., Zhang, C., Ju, X., & Deng, J. (2018). A process-oriented hydro-biogeochemical model enabling simulation of gaseous carbon and nitrogen emissions and hydrologic nitrogen losses from a subtropical catchment. Science of the Total Environment, 616-617, 305–317. https://doi.org/10.1016/j.scitotenv.2017.09.261
- Zhang, Y., Mallet, C., Bocquet, M., Seigneur, V., & Baklanov, A. (2012b). Real-time air quality forecasting, part II: State of the science, current research needs, and future prospects atmospheric environment. Atmospheric Environment, 60, 656–676. https://doi.org/10.1016/j.atmosenv.2012.02.041
- Zhang, Y., Seigneur, C., Bocquet, M., Mallet, V., & Baklanov, A. (2012a). Real-time air quality forecasting, Part I: History, techniques, and current status. Atmospheric Environment, 60, 632–655. https://doi.org/10.1016/j.atmosenv.2012.06.031
- Zhang, W., Yao, Z., Li, S., Zheng, X., Zhang, H., Ma, L., Wang, K., Wang, R., Liu, C., Han, S., Deng, J., & Li, Y. (2021). An improved process-oriented hydro-biogeochemical model for simulating dynamic fluxes of methane and nitrous oxide in alpine ecosystems with seasonally frozen soils. Biogeosciences, 18(13), 4211–4225. https://doi.org/10.5194/bg-18-4211-2021
- Zhang, W., Yao, Z., Zheng, X., Liu, C., Wang, R., Wang, K., Li, S., Han, S., Zuo, Q., & Shi, J. (2020). Effects of fertilization and stand age on N2O and NO emissions from tea plantations: A site-scale study in a subtropical region using a modified biogeochemical model. Atmospheric Chemistry and Physics, 20(11), 6903–6919. https://doi.org/10.5194/acp-20-6903-2020
- Zhou, P., Ganzeveld, L., Rannik, Ü., Zhou, L., Gierens, R., Taipale, D., Mammarella, I., & Boy, M. (2017). Simulating ozone dry deposition at a boreal forest with a multi-layer canopy deposition model. Atmospheric Chemistry and Physics, 17(2), 1361–1379. https://doi.org/10.5194/acp-17-1361-2017
- Zhou, P., Ganzeveld, L., Taipale, D., Rannik, U. ̈., Rantala, P., Rissanen, M. P., Chen, D., & Boy, M. (2017). Boreal forest BVOC exchange: Emissions versus in-canopy sinks. Atmospheric Chemistry and Physics, 17(23), 14309–14332. https://doi.org/10.5194/acp-17-14309-2017
- Zhou, L., Nieminen, T., Mogensen, D., Smolander, S., Rusanen, A., Kulmala, M., & Boy, M. (2014). SOSAA — a new model to simulate the concentrations of organic vapours, sulphuric acid and aerosols inside the ABL — part 2: Aerosol dynamics and one case study at a boreal forest site. Boreal Environment Research, 19(suppl. B), 237–256.
- Zilitinkevich, S., Kulmala, M., Esau, I., & Baklanov, A. (2015). Megacities – refining models to personal environment. WMO Bulletin, 64(1), 20–22.
- Zimmer, W., Bruggemann, N., Emeis, S., Giersch, C., Lehning, A., Steinbrecher, R., & Schnitzler, J.-P. (2000). Process-based modelling of isoprene emission by oak leaves. Plant, Cell and Environment, 23(6), 585–595. https://doi.org/10.1046/j.1365-3040.2000.00578.x
- Zou, Y., Wang, Y., Zhang, Y., & Koo, J. H. (2017). Arctic sea ice, Eurasia snow, and extreme winter haze in China. Science Advances, 3(3). https://doi.org/10.1126/sciadv.1602751