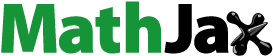
Abstract
We reported previously that the persistence of complex immune, endocrine and neurological symptoms that afflict up to one third of veterans from the 1990-91 Gulf War might be supported by a misdirected regulatory drive. Here we use a detailed model of immune signaling in concert with an overarching circuit model of known sex and stress hormone co-regulation to explore how the failure of regulatory elements may further establish a self-perpetuating imbalance that closely resembles Gulf War Illness (GWI). Defects to the model were imparted iteratively and the stable regulatory modes supported by these altered immune-endocrine circuits were identified using repeated simulation experiments. In each case the predicted homeostatic regimes were compared to experimental data collected in male GWI (n=20 ) and matched healthy veterans (n=22 ). We found that alignment of GWI with a new homeostatic regime improved significantly when cortisol's normal anti-inflammatory activity was interrupted. Alignment improved further when this cortisol insensitivity was compounded by the loss of the normal antagonistic effects of Th1 cytokines on Th2 lymphocyte activation. Together these simulation results suggest altered glucocorticoid gene regulation compounded by possible changes in IGF-1 regulation of Th1:Th2 immune balance may be key underlying features of GWI.
Introduction
Gulf War Illness (GWI) is a complex multi-symptom illness associated with deployment to the Persian Gulf between 1990-91 and presenting with symptoms that manifest across several of the body's principal regulatory systems.Citation1-3 Our work and that of others strongly suggest that pathogenesis of this complex illness includes an immunologic Citation4-7 and an endocrine component.Citation8 Based on this, we proposed an illness model whereby GWI may involve a chronic imbalance in co-regulation between the nervous, endocrine and immune systems. To investigate this further we extended a discrete logic formalism first proposed by Mendoza and XenariosCitation9 to develop a coarse-grained but overarching model describing documented regulatory signaling within and between components of the hypothalamic-pituitary-adrenal (HPA), hypothalamic-pituitary-gonadal (HPG) and immune systems for both male and female physiology.Citation10 This was followed by a more detailed fine grain model of the immune system incorporating oversight by stress hormone and male sex steroid signaling in a subsequent investigation.Citation11 In both cases logic circuits were assembled using an extension of representing normal regulatory physiology as documented in a broad survey of the medical literature.
Analysis of these logic circuit models revealed that the complexity associated with immune signaling and its oversight by sex and stress hormone regulation was such that these regulatory networks naturally supported more than one stable homeostatic regime. Comparison with a broad set of immune and endocrine markers measured in peripheral blood from GWI subjectsCitation4,5 indicated that this chronic condition is proximal to such alternate homeostatic regimes and that homeostatic drive may be involved in the perpetuation of illness.Citation10,11 Specifically, we found that that the inclusion of basic immune function and male sex hormone regulation by the HPG axis with HPA function resulted in the emergence of 5 stable equilibrium states. Steroid and cytokine levels measured experimentally in healthy and ill veterans were compared to model predictions using a theoretical approximation of Fisher's method developed by Brown that estimates the overall significance of a set of p-values obtained from multiple independent tests of the same null hypothesis.Citation12 On this basis, male veterans with GWI showed poor alignment (p=0 .82) with the predicted nominal resting state, reinforcing that GWI represents a significant departure from normal endocrine-immune function. At this level of resolution, experimental data for GWI (p=0 .30) aligned best with a predicted state supporting chronically elevated cortisol levels, low testosterone and a shift toward Th1 immune activation. These results reinforced the premise that insults to the endocrine-immune system may displace normal homeostasis toward a more classical Th1 inflammatory signature with a concurrent and perhaps stabilizing endocrine component in GWI without requiring actual damage to the regulatory circuitry or the continued presence of the original insult. Nonetheless, while the immune and endocrine signatures observed in GWI aligned much more readily with these alternate stable regimes than with normal health this alignment was only partial. In other words while homeostatic drive may play a role in perpetuating GWI, the original insult may have also imparted some damage to the normal regulatory circuitry.
In this work we attempt to identify some of the affected processes by systematically simulating defects in regulatory circuits both at the broader endocrine-immune levelCitation10 as well as at the level of detailed immune signaling.Citation11 In each case the stable regulatory regimes supported by these altered circuits were identified and compared to available experimental data describing endocrine and immune marker expression in GWI. Significant improvement in alignment between alternate homeostatic states and GWI was obtained when the normal inhibition by cortisol of innate immune inflammatory activity was interrupted. Alignment improved further when this insensitivity to cortisol was compounded by a loss of responsiveness by Th2 lymphocytes to inhibition by Th1 cytokines resulting in a loss of Th1:Th2 polarization.
Methods
Literature Based Neuro-Endocrine Immune Signaling Networks. The Course Grain Model (CGM) used in this investigation is the male HPA-HPG Immune axis presented by Craddock et al.Citation10 and has 14 biomarker nodes and 1 external influence (stress), with 40 interaction wires connecting them (). The Fine Grain Model (FGM), also for male HPA-HPG axis possesses a more detailed immune signaling network, has 21 biomarker nodes and 3 external influences (stress, infection, HPG drive), with 82 interaction wires connecting them (). Details of the FGM may also be found in previous work by our group.Citation11 The grouping of individual cytokines and hormones into the aggregate nodes is presented in Supplementary Tables S1, and S2 for the CGM and FGM respectively.
Figure 1. A coarse-grained model of endocrine-immune signaling. A coarse circuit logic representation of the principal signals linking the hypothalamic-pituitary-adrenal (HPA) and hypothalamic-pituitary-gonadal (HPG) endocrine axes with innate immune response (IIR) as well as T-helper 1(Th1) and 2 branches of the adaptive immune system as proposed by Craddock et al. (2014).Citation10

Figure 2. A fine-grained model of immune cell signaling. A detailed circuit logic representation of immune cell signaling including the T-helper 1 (Th-1), 2, (Th2), 17, (Th17) as well as regulatory T-cell signaling (Treg) and their interface with sex and stress hormone regulation as proposed by Fritsch et al. (2013).Citation11
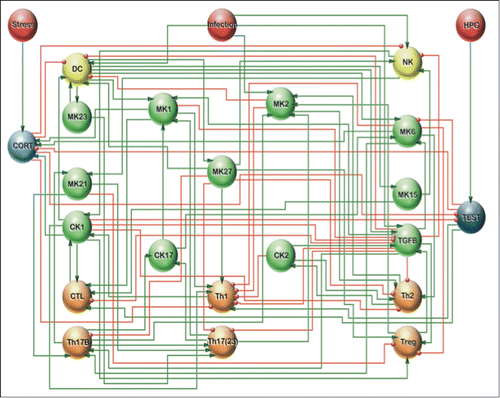
Discrete Logical Analysis. In this work we create a model of the immune system and its principal endocrine mediators by using a discrete ternary logical network analysis based on the analysis of Mendoza and XenariosCitation9 and Thomas,Citation13 and developed in our previous analysis.Citation10,11 We encode the feedback mechanisms within the neuroendocrine-immune system based only on the direction (source and target) and type (activator or inhibitor) of interaction.
In this model, signaling molecules and cell types are represented as individual variables each capable of adopting 3 discrete state: −1 (down-regulated), 0 (nominal), and 1 (up-regulated). At any point in time t, the state of a system with N variables can be represented by the vector , such that:
(1)
(1) where
is the state of the ith variable of the N variable system at time t. The image vector
describes the preferred state toward which the system evolves in the next time increment. The state value of the image vector for the ith variable is determined from its current state and a set of balanced ternary logic statements based on the current value of variable and the mode of action (i.e. activate or inhibit) of the neighboring input variables. These logic statements are expressed as follows (EquationEq. 2
(2)
(2) ):
(2)
(2) where the ∇, ∨, and ¬ symbols are ternary HIGH/LOW PASS, OR and NOT operators,
is the state of the ith variable's jth activator,
is the state of the ith variable's kth inhibitor. The ternary operators given in Equation (2) are described in further detail in supplementary tables S3- S5. The first entry in Equation (2) is used when the variable possesses X activators and Y inhibitors, the middle when the variable has only X activators and last when the activator has only Y inhibitors.
Applying Equation (2) to each variable in the model for the mth state of the system, , defines the image vector
for the mth state. With
defined, the system may be updated asynchronously (allowing only one variable to change at a time) following the generalized logical analysis of Thomas.Citation13 According to this method the ith variable of the mth state vector
is moved one step toward its preferred image
(e.g. If
= −1 and
= 1, then
is set to 0). Thus, for each current state of the system there are potentially several subsequent states toward which it may asynchronously evolve. Steady states are defined as those states for which the image vector is the same as the current state vector; in other words the state possesses an out degree of 0.
The number of molecular/cellular variables in the regulatory network model dictates the number of states the overall system is capable of possessing. Since each variable is capable of assuming one of 3 values (−1, 0, or 1), the number of states of the system is given by 3N, where N is the number of biomarker variables. The CGM contains 15 soluble mediators and cell types, yielding 314 = 4,782,969, (roughly 5 million) possible states, while the FGM has 321 = 10,460,353,203 (roughly 10.5 billion) potential states. The above logical analysis is applied to each of these states to determine which states are stable.
GWI Cohort Sample Collection. Cytokine profiles and endocrine measures were obtained as part of a larger ongoing study in n = 27 GWI, 29 health control (HC) subjects recruited from the Miami Veterans Administration Medical Center. Subjects were all male with an average age of 43 y and BMI of 28. Inclusion criteria was derived from Fukuda et al.,Citation14 and consisted in identifying veterans deployed to the theater of operations between August 8, 1990 and July 31, 1991, with one or more symptoms present after 6 months from at least 2 of the following: fatigue; mood and cognitive complaints; and musculoskeletal complaints. Subjects were in good health prior to 1990, and had no current exclusionary diagnoses.Citation15 The use of the Fukuda definition in GWI is supported by Collins et al.Citation16 Control subjects consisted of gulf war era sedentary veterans and were matched to illness subjects by age, body mass index (BMI) and ethnicity. Endocrine measures are comprised of plasma TEST and salivary CORT levels. Cytokine profiles consist of plasma levels of IL-1α, IL-1β, IL-2, IL-4, IL-5, IL-6, IL-8, IL-10, IL-12p70, IL-13, IL-17, IL-23, IFN-γ, TNF-α, and TNF-β. Summary statistics are presented in Supplementary Table S6. Additional details regarding this cohort and the laboratory assays performed are also available in Broderick et al.Citation6
Statistical Comparison against Clinical Data Using Brown's Method. Brown's theoretical approximationCitation12 of Fisher's statistics was used to calculate the significance of alignment between experimental data and a given model predicted state. Fisher's method, a meta-analysis technique, combines probabilities to obtain the overall significance of a set of p-values obtained from independent tests of the same null hypothesis. The combined χ2 statistic,(3)
(3) where N is the number of measureable variables and pi is the corresponding p-values under the null hypothesis, has a χ2 distribution with 2N degrees of freedom assuming that the performed tests are independent. As the molecular variables of the endocrine and immune system interact with one another, as evidenced by the above connectivity diagrams, they are not independent. As a result, direct application of this test statistic is invalid, since the assumption of independence is violated. BrownCitation12 suggested a method for combining non-independent tests. If the tests are not independent, then the statistic T0 has mean m = 2N and variance (σ2) given as,
(4)
(4) where pi and pj are the p-values for each test and the covariance (cov) is calculated as,
(5)
(5)
with ρij being the unadulterated correlation between variable i and variable j. Finally, the overall significance P of a set of non-independent tests is calculated using the statistic T which under the null hypothesis follows the central χ2 distribution, where T = T0/c with 2N/c degrees of freedom and c = σCitation2/4N.
Here, we test if each experimental measure aligns with a given model predicted state. Our null hypothesis is that the experimental measures do not align. P-values for individual variables, pi, are calculated using 2-sample t-tests between ill subjects and healthy controls. Where model predictions give a variable as high (+1), ‘right-handed’ one-tailed test are used, whereas a ‘left-handed’ test was used when model predictions are low (−1), to give the probability of obtaining the predicted value when the null hypothesis is true. For the case where the model predicts normal behavior for a variable (0) a 2-tailed t-test is used. However, the p-value from the 2-tailed test, ptwo-tail, gives the probability that there is an observable difference between illness and control, which is the null hypothesis. To rectify this, when comparing to a model predicted variable of 0 we take the P-value to be pi = 1 − ptwo-tail, giving the probability of obtaining the predicted value when the null hypothesis is true. All cohort data was normalized using a Log2 transformation before T-tests and correlation calculations were performed. The unadulterated correlation values ρij between 2 variables i and j were calculated in healthy subjects as the pairwise Pearson's linear correlation coefficient between variables. Where model variables represent an aggregate set of markers each experimentally measured constituent marker was compared individually to the model predicted value.
Systematic interruption of molecular signaling. Using Brown's method, as described above, a base proximity between GWI and the stables states predicted based on the complete CGM and FGM was established. Following this each complete model was used to create new models for proximal comparison to GWI first by deleting each individual connection in turn and then by deleting connections 2 at a time. These new altered networks were then analyzed using the discrete logical analysis, described above, to find alternate homeostatic states. Once all of the alternate states for the deletion models were found, they were compared to GWI via Brown's method,Citation12 and their subsequent p-value was noted.
A network is a set of n nodes, and m edges. To create new networks, the original set A was modified using the following algorithm:
For each edge i in A,
remove edge i,
save new set as set B,
return edge i.
Creating a new set B, of n nodes, and m-1 edges.
Then using the new set B,
For each edge i in B,
remove edge i,
save new set as set C,
End
Consider a complete set A with M edge elements G defined as,(6)
(6)
removal of a single edge interaction can then be defined as,(7)
(7) and a double deletion as
(8)
(8)
and so forth.
Thus, we test the subsets of AM defined as,(9)
(9)
(10)
(10)
Ethics Statement
All subjects signed an informed consent approved by the Institutional Review Board of the University of Miami. Ethics review and approval for data analysis was also obtained by the IRB of the University of Alberta.
Results
Using Brown's combined null probability,Citation12 as described in the Methods section, a baseline statistical proximity between the observed GWI marker profile and the predicted stables states was calculated for a series of defects applied to (i) a broader but more coarse model of HPA-HPG-immune regulation () as well as to (ii) a more detailed immune signaling network model (). Defects were applied individually and 2 at a time in order to systematically create a set of altered network models. In each case the stable states associated with this new altered wiring were identified using the discrete logical analysis described above and were compared for statistical similarity to GWI via Brown's method. Note that the reference state vectors corresponding to the healthy control and Gulf War illness conditions were based on the average immune and endocrine signatures shown in Supplemental Table S6.
Assessing potential defects in endocrine-immune co-regulation. Using the coarse but inclusive model of HPA-HPG-immune regulationCitation10 () a new series of endocrine-immune networks was created by deleting in turn each individual network connection. This resulted in the creation of 40 unique networks with a single interaction removed. This was followed by a second series of networks created by deleting one additional interaction from the newly generated networks. After removal of duplicate networks, this created 818 unique new networks, each with 2 connections removed. Recall that the original HPA-HPG-immune network model with all connections intact supported 4 alternate stable steady-states in the case of male physiology. As described in the Methods section stable states were identified exhaustively from all possible systems states supported by the network logic such that the system's next allowable state remains unchanged, or constitutes a state node with a zero out degree (EquationEq. 2(2)
(2) ).
In the first alternate state (SS1) glucocorticoid receptor expression (GRD and GR) was elevated at low levels of ACTH, while in the second state (SS2) innate and Th1 immune responses were suppressed (low ICell, IIR, T1Cell, and T1Cyt) under elevated Th2 activity (high T2Cell and T2Cyt). Combining these features, the third state (SS3) was characterized by low ACTH at high glucocorticoid receptor expression (GR and GRD) and a shift toward Th2 immune activation (T2Cell and T2Cyt) with suppressed innate and Th1 activity (ICell, IIR, T1Cell and T1Cyt). The final state (SS4) best resembled GWI with a Brown's combined null probability of p=0 .30 as reported previously.Citation10 This alternate homeostasis was characterized by hypercortisolism, low testosterone (TEST) levels and a shift toward a polarized Th1 immune response (low T2Cell, T2Cyt, GnRH, LH/FSH and TEST/EST, and high CORT, GRD, GR, T1Cyt and T1Cell). Of the 40 networks obtained by imparting a single deletion at a time to this model, one circuit supported an alternate stable state with a proximity to GWI corresponding to a combined Brown's p-value of 0.13 () (see also SS1 in supplementary Table S7). This regulatory circuit was obtained by deleting the normal inhibitory actions of cortisol (Cort) on the inflammatory response supported by innate immune cell (Icell) activation. Proximity to GWI improved slightly when 2 interactions are removed yielding 2 altered circuits, each supporting a stable state with a combined Brown's p-value of divergence from GWI of p=0 .10 (). The first of these circuits is obtained by combining the above-mentioned defect in cortisol signaling with a loss of Th1 cytokine (T1Cyt) induced inhibition of Th2 cell (T2Cell) activity ((B) top panel). The second competing circuit corresponds to loss in cortisol anti-inflammatory signaling aggravated with a defect in Th2 cytokine (T2Cyt) production by the Th2 helper cell population (T2Cell) ((B) bottom panel).
Figure 3. Single defect in HPA-HPG-immune signaling. Statistical difference between observable marker levels in GWI and the alternate steady-state produced in the coarse grained HPA-HPG-Immune model with a single defect to the signaling network. Stable states were identified exhaustively from all possible systems states supported by the network logic such that the system's next allowable state remains unchanged (i.e., x(t+1)=x(t)), or constitutes a state node with a zero out degree (EquationEq. 2(2)
(2) ). A single defect reduces Brown's combined null probability of a difference between GWI and the new modified model from a previous p-value of 0.30 to a value of 0.13 (panel A). This defect corresponds to interruption of normal cortisol-induced suppression of innate cell inflammatory activity (panel B).

Figure 4. Multiple defects in a coarse-grained signaling circuit. Interrupting two signals at a time in the coarse-grained HPA-HPG-immune network yields 2 solutions, both involving interruption of cortisol suppression of innate inflammatory response. In the first case this is accompanied by the loss of Th1 cytokine inhibition (T1Cyt) of Th2 cell activation (T2Cell) and in the second case by interruption of Th2 cytokine production (T2Cyt) by Th2 cells (T2Cell). As before, stable states were identified exhaustively from all possible systems states supported by the network logic such that the system's next allowable state remains unchanged, or constitutes a state node with a zero out degree. In both cases double interaction deletions produced stable states with a combined null p-value according to Brown's method of p=0 .10 for divergence with observed GWI marker levels.
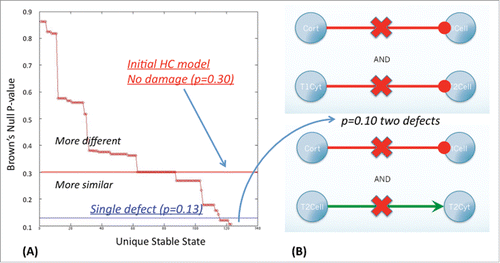
Imparting defects in an intricate immune signaling network. The same procedure was performed on the fine-grained discrete logic model (FGM) of immune signaling presented previously in Fritsch et al.Citation11 (). Imparting individual defects created 82 new immune signaling networks. Interfering with 2 immune signals at a time produced 3327 unique networks. As before stable states were identified exhaustively from all possible systems states supported by the network logic such that the system's next allowable state remains unchanged (EquationEq. 2(2)
(2) ). The original unaltered network supported 2 alternate stable attractors, one of which was separated from GWI by a combined Brown's null probability of p = 0 .13. The latter corresponded to a chronic state of Th17 immune activation in the context of elevated cortisol and low testosterone (TEST).
Of the 82 networks produced by interrupting individual immune signals 2 of these altered circuits supported alternate stable states that were more proximal to GWI ((A)). The first supported a stable state with a combined p-value for divergence from GWI of p=0 .10. The second circuit supported an alternate stable state with an even better alignment to GWI, namely a combined null probability of divergence of p=0 .05. The latter involved the loss of Th1 cytokine-induced (CK1) inhibition of Th2 helper cell activity (Th2) ((B)) (see also SS1 in supplementary Table S8). This solution reinforces the result obtained at coarser resolution with the HPA-HPG-immune multi-axis model. Removal of 2 connections at a time in this detailed immune model did not yield circuits supporting a better alignment with GWI ( and S1). Of the 3,321 dual-cut configurations created (82 x 81 x 1/2), 74 models supported 5 unique stable states that approximated GWI with a Brown's p=0 .05 (). All of these 74 circuit configurations included deletion of the inhibitory action of CK1 on the Th2 cells population. The remaining 7 of 81 available models containing a primary CK1/ Th2 deletion did not achieve the minimal Brown's p-value of 0.05. These 7 secondary defects are listed in Figure S1. Among these we find components of Th17 signaling. It is important to note that these findings do not indicate that Th17 signaling is up or downregulated but instead simply reinforce that these component mechanisms of normal Th17 signaling remain intact. Indeed their disruption decreases model fit to GWI.
Figure 5. Single compounding defect in detailed immune network circuitry. The statistical difference between observable marker levels in GWI and the alternate steady state produced with the introduction of a single defect in a detailed model of immune cell signaling. This defect reduces Brown's combined null probability of a difference between GWI and the new modified model from a previous p-value of 0.13 to a value of 0.05 (panel A). This defect corresponds to loss of Th1 cytokine (CK1) inhibition of Th2 cell activation (Th2) (panel B). Stable states were identified exhaustively from all possible systems states supported by the network logic such that the system's next allowable state remains unchanged, or constitutes a state node with a zero out degree.
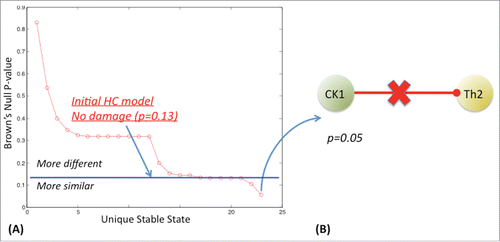
Figure 6. Multiple defects in a detailed immune signaling circuit. Interrupting two signals at a time in the detailed immune network yields 5 stable state solutions. These do not improve the fit to the GWI chronic immune profile over that obtained with a single defect. Of the 3,321 possible double interaction deletions, 74 altered circuits supported stable states with a Brown's combined null p-value of p=0 .05 for divergence with observed GWI marker levels. As before stable states were identified exhaustively from all possible systems states supported by the network logic such that the system's next allowable state remains unchanged, or constitutes a state node with a zero out degree.
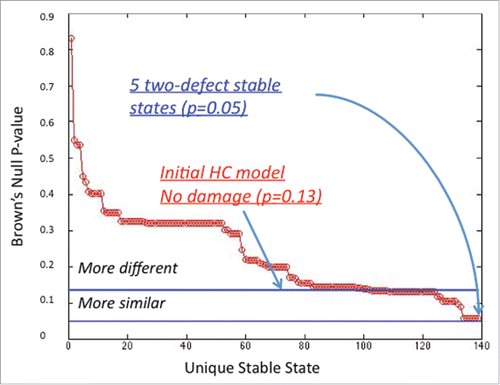
Network defects compromise robustness to challenge. In both of the damaged circuits mentioned above the defects modify the attractor landscape in a way that supports the emergence of attractors that more closely resemble GWI as a self-sustained chronic illness state. It is important to note that in each case even though a new attractor landscape emerges, this new landscape continues to support access to a stable healthy regulatory condition as shown in supplementary tables S7 and S8 (stable state SS3). This reinforces the notion that despite these alterations to the system some degree of stable remission may be possible. Indeed our simulation results suggest that it is not the outright accessibility of normal regulatory control that is compromised but rather the stability of this healthy state given these defects to the system. To explore this we simulated the response of the coarse grained model () both with and without defects to idealized impulse challenges involving a sudden increase in stress, an acute immune response to injury and a combination of both stress and injury. Acute stress was simulated as a sudden impulse increase in cortisol (Cort) concentration whereas acute response to injury was simulated as a sudden elevation in innate immune signaling (IIR). Probabilistic discrete event simulations were repeated one million times in each case. Results presented in Supplementary Figure S2 show that both altered and unaltered circuits returned to healthy homeostasis roughly 2 out of 3 times in response to a sudden stressor. Once again both altered and unaltered circuits showed similar robustness to acute injury (impulse increase in IIR) with each returning to healthy homeostasis instead of escaping to a pathological steady state in roughly 1 out of 3 cases. However when simulated stress and injury were combined the unaltered HPA-HPG-immune circuit was much more robust to challenge, basically returning to a healthy control regime 1.5 times more frequently than in the case of the defective circuit. These results suggest that these defects may compromise the robustness of normal regulatory control and facilitating relapse but may not prohibit remission outright. It is important to note that the current models capture connectivity only and that a more thorough analysis of transition states separating attractors would require formal inclusion of response dynamics. Consequently these possible differences in local stability should be interpreted in relative terms only.
Discussion
In this work we continue to explore the involvement of homeostatic regulation in the perpetuation of symptoms in the chronic complex illness known as Gulf War Illness. In our earlier work we report discrete logic models of detailed immune signalingCitation11 as well as oversight of immune activation by stress and sex hormone regulation.Citation10 In both cases logic circuits were assembled representing normal regulatory physiology as documented in a broad survey of the medical literature. As expected, we found that these regulatory networks naturally supported more than one stable homeostatic regime and that immune and endocrine signatures observed in GWI aligned much more readily with these altered stable regimes than with normal health. However this alignment was only partial suggesting that the original insult leading to GWI may have also imparted some lasting changes to the normal regulatory circuitry. To explore this we conducted an analysis of stable regulatory regimes supported when select defects were imparted to these circuit models. Using an exhaustive series of simulations based on a detailed model of immune signaling as well as a broader model of immune, stress and sex hormone regulation we found that interruption of cortisol's anti-inflammatory actions on innate immune activation produced an alternate regulatory program that more closely mimicked GWI. This similarity improved further when cortisol insensitivity was compounded with blunted inhibition of Th2 activity by the opposing Th1 immune axis.
Experimental data reported previously by this groupCitation6 indicate that GWI subjects often present with chronically elevated levels of cortisol (CORT). This same data also shows a loss of Th1:Th2 polarity as evidenced by concurrently elevated IL-13 (Th2) and TNF-β (Th1). Cortisol acts on naïve T-cells to repress both the Th1 promoting transcription factor, T-bet, and the Th2 promoting transcription factor, GATA-3. However, since the suppressive effect on T-bet is much stronger than the suppressive effect on GATA-3, a Th2 shift is ultimately favoredCitation17 under normal circumstances. The model presented here points to impaired glucocorticoid receptor (GR) signaling leading to glucocorticoid insensitivity and the persistence of elevated cortisol levels. Regulation of glucocorticoid responsiveness is complex however there is evidence that the latter is also reduced by exposure to pro-inflammatory cytokines, such as tumor necrosis factor α (TNF-α) and interleukin-1β (IL-1β),Citation18 both of which are suggested to play a role in GWI.Citation4,19 Among its broad-reaching effects GR interacts with receptor tyrosine kinase to promote BDNF-induced release of glutamate. SiRNA transfection inducing a decrease in endogenous GR has been shown to decrease BDNF-dependent binding of phospholipase C-γ (PLC-γ) to tyrosine kinase B (TrkB) resulting in the suppression of neurotransmitter release. Consistent with the proposed GR insensitivity, our previous analysis of gene expression profiles in peripheral blood mononuclear cells (PBMC) indicated a decrease in Trk pathway activity in this cohort that were linked to changes in pathways supporting neurogenesis and axonal guidance.Citation6 GR responsiveness is a known target of epigenetic control. Glucocorticoid resistance has been linked to reduced histone deacetylase-2 expression leading in turn to decreased repression of inflammatory genes.Citation18 Moreover exposure to traumatic events has been shown to modify methylation of the glucocorticoid receptor gene NR3C1 promoter region with hypermethylation found in relation to early life stressors.Citation20 Conversely Yehuda et al.Citation21 recently reported hypomethylation of NR3C1 promoter region in a cohort of male Gulf War veterans with PTSD, suggesting that epigenetic modification of the GR and associated changes in cortisol sensitivity the may depend on the timing, type and duration of exposure to adversity. Our simulation results would in principle support a hypermethylation of the glucocorticoid receptor gene promoter region leading to GR insensitivity and chronically elevated cortisol levels. This divergence in HPA status of GWI from PTSD is supported by Golier et al. (2007).Citation8
Though our data shows a broad range of values for IFN-γ levels in peripheral blood from GWI subjectsCitation7 , this Th1 cytokine tends to be elevated in this illness group (p=0 .07 in a 2-way ANOVA).Citation6 One would expect a trend toward lower expression of Th2 cytokines in GWI but these remain essentially normal in the case of IL-4 and IL-5, or increased in the case of IL-13, suggesting insensitivity to the normal IFN-γ induced down-regulation of Th2 polarization.Citation22 In T helper cells down-modulation of IFN-γR2 acts as a negative regulatory mechanism that attenuates IFN-γ/signal transducer and activator of transcription-1 (STAT-1) signaling and limits the apoptotic effect of IFN-γ. This loss of IFN-γR2 that imparts resistance to IFN-γ is induced by IFN-γ itself in Th1 fated cells. This IFN-γR2 internalization is not observed in established Th2 clones. However other factors such as hormones may play a critical role in modulating IFN-γR2 expression and IFN-γ responsiveness in human T lymphocytes. For example IGF-1 is known to signal for IFN-γR2 internalization and limit IFN-γ/STAT-1 signaling in human T cells.Citation23 Though we did not measure IGF-1 levels in our previous work, we did find high IL-13 (p<0.01) which is known to increase IGF-1 production in macrophages.Citation24 We also found genomic evidence of increased NF-kB activation in this GWI cohort which promoted by IGF-1 via phosphatidylinositol 3-kinase (PI3K) / Protein Kinase C (PkC) engagement.Citation25 While not conclusive these simulation results suggest a mechanism by which an IGF-1 rich environment may abrogate IFN-γ / STAT1 mediated apoptosis of Th2 cells leading to a joint Th1/Th2 profile observed experimentally. Moreover IGF-1 has been found to inhibit whereas IGF-2 potentiated K+-evoked ACh release from hippocampal slices in rat.Citation26,27 A leading hypothesis in GWI involves extended low-level exposure to Acetylcholine esterase inhibitorsCitation28 and elevated IGF-1 levels might be expected response to high ACh levels. This modulating action of IGF-1 was not incorporated a priori in the model and may explain why the alignment to GWI is enhanced if we provide a means for sustained Th2 activity in an environment trending toward increased IFN-γ and TNF-β.
These simulation results suggest that transient insult to the endocrine-immune regulatory network in GWI, in addition to prompting a persistent adaptive response, may have left lasting effects, perhaps through epigenomic mechanisms, to GR expression that impair HPA axis and Th1/Th2 balance. The latter may also be mediated by chronic increases in IL-13 induced IGF-1 expression. The latter response may have been prompted initially as a response to elevated ACh if exposure to AChE inhibitors was the insulting agent. In addition to suggesting possible pathogenic processes in GWI, these simulation results inform future improvements in the computational model in particular direct and preferential suppression of Th1 by GC should be modeled in greater detail, as should the immuno-modulatory effects of IGF and their relation to ACh expression. These efforts are ongoing.
It is also interesting to note that while these defects produced stable attractors presenting with immune and endocrine profiles that more closely resembled GWI, the resulting landscape still supported access to a normal homeostatic regime. Exploratory simulations suggested however that this healthy regulatory state was somewhat less robust to insult and that the defects imparted to the networks made it easier to escape health and lapse into a persistent pathological state. This result is not all that surprising when we consider the inherent properties of biological networks, which select simultaneously for adaptability and robustness.Citation29 Defects such as these might be compared to non-lethal deletions in gene regulatory networks. While the current connectivity models strictly enforce the sequence of allowable states they do not account for transition dynamics. While they remain exploratory these early findings nonetheless motivate the inclusion of more detailed transition dynamics in a future iteration of these models in order to support of a more thorough analysis of the topology in regions that lie between stable attractor states.
Disclosure of Potential Conflicts of Interest
The authors declare that they have no conflicts of interest.
Supplemental Material
Supplemental data for this article can be accessed on the publisher's website.
Author Contributions
MAR, RMdR, TJAC, JZ and GB performed computational simulations and analysis of computational results. TJAC, NGK, MAF and GB, compiled physiological data and developed the immune-endocrine connectivity network. MAR, TJAC and GB performed comparisons between experimental and computational data and interpreted the results. MAF, NGK and ZB collected and analyzed the experimental data. TJAC, MAR, RMdR, and GB conceived of the discrete logical analysis algorithm. All authors contributed to the drafting of this article, and approve of its contents.
Supplemental_material.zip
Download Zip (326 KB)Acknowledgments
This research was conducted in collaboration with the High Performance Computing team at the University of Miami Center for Computational Science (CCS) (http://ccs.miami.edu). The authors would also like to thank Dr. Andrée Gruslin for many helpful discussions regarding the many regulatory effects of insulin growth factor (IGF).
Funding
Funding was provided by US Department of Defense Congressionally Directed Medical Research Program (CDMRP) awards (http://cdmrp.army.mil/) GW093042 (Broderick - PI) and GW080152 (Klimas - PI) as well as Merit Awards from the US Veterans Affairs (Klimas - PI).
References
- Unwin C, Blatchley N, Coker W, Ferry S, Hotopf M, Hull L et al. Health of UK servicemen who served in Persian Gulf War. Lancet 1999; 353:169–78; PMID:9923871; http://dx.doi.org/10.1016/S0140-6736(98)11338-7
- Bourdette DN, McCauley LA, Barkhuizen A, Johnston W, Wynn M, Joos SK, Storzbach D, Shuell T, Sticker D. Symptom factor analysis, clinical findings, and functional status in a population-based case control study of Gulf War unexplained illness. J Occup Environ Med 2001; 43(12):1026–40; PMID:11765674; http://dx.doi.org/10.1097/00043764-200112000-00005
- Kang HK, Natelson BH, Mahan CM, Lee KY, Murphy FM. Post-Traumatic Stress Disorder and Chronic Fatigue Syndrome-like Illness among Gulf War Veterans: A Population-based Survey of 30,000 Veterans. Am J Epidemiol 2003; 157:141–48; PMID:12522021; http://dx.doi.org/10.1093/aje/kwf187
- Broderick G, Kreitz A, Fuite J, Fletcher MA, Vernon SD, Klimas N. A pilot study of immune network remodeling under challenge in Gulf War Illness. Brain Behav Immun 2011 Feb; 25(2):302–13; http://dx.doi.org/10.1016/j.bbi.2010.10.011
- Broderick G, Fletcher MA, Gallagher M, Barnes Z, Vernon SD, Klimas NG. Exploring the diagnostic potential of immune biomarker coexpression in Gulf War Illness. Methods Mol Biol 2012; 934:145–64; PMID:22933145; http://dx.doi.org/10.1007/978-1-62703-071-7_8
- Broderick G, Ben-Hamo R, Vashishtha S, Efroni S, Nathanson L, Barnes Z, Fletcher MA, Klimas N. Altered immune pathway activity under exercise challenge in Gulf War Illness: an exploratory analysis. Brain Behav Immun 2013 Feb; 28:159–69; http://dx.doi.org/10.1016/j.bbi.2012.11.007
- Smylie AL, Broderick G, Fernandes H, Razdan S, Barnes Z, Collado F, Sol C, Fletcher MA, Klimas N. A comparison of sex-specific immune signatures in Gulf War illness and chronic fatigue syndrome. BMC Immunol 2013 Jun 25; 14:29; http://dx.doi.org/10.1186/1471-2172-14-29
- Golier JA, Schmeidler J, Legge J, Yehuda R. Twenty-four Hour Plasma Cortisol and Adrenocorticotropic Hormone in Gulf War Veterans: Relationships to Posttraumatic Stress Disorder and Health Symptoms. Biol Psychiatry 2007; 62(10):1175–78; PMID:17612507; http://dx.doi.org/10.1016/j.biopsych.2007.04.027
- Mendoza L, Xenarios I. A method for the generation of standardized qualitative dynamical systems of regulatory networks. Theor Biol Med Model 2006 Mar 16; 3:13; http://dx.doi.org/10.1186/1742-4682-3-13
- Craddock TJ, Fritsch P, Rice MA Jr, del Rosario RM, Miller DB, Fletcher MA, Klimas NG, Broderick G. A role for homeostatic drive in the perpetuation of complex chronic illness: Gulf War Illness and chronic fatigue syndrome. PLoS One 2014 Jan 8; 9(1):e84839; http://dx.doi.org/10.1371/journal.pone.0084839
- Fritsch P, Craddock TJ, del Rosario RM, Rice MA, Smylie A, Folcik VA, de Vries G, Fletcher MA, Klimas NG, Broderick G. Succumbing to the laws of attraction: Exploring the sometimes pathogenic versatility of discrete immune logic. Systems Biomedicine 2013; 1:179–94; http://dx.doi.org/10.4161/sysb.28948; http://dx.doi.org/10.4161/sysb.28948
- Brown M. A method for combining non-independent, one-sided tests of significance. Biometrics 1975; 31:987–92; http://dx.doi.org/10.2307/2529826
- Thomas R. Regulatory Networks Seen as Asynchronous Automata: A Logical Description. J Theor Biol 1991; 153:1–23; http://dx.doi.org/10.1016/S0022-5193(05)80350-9
- Fukuda K, Nisenbaum R, Stewart G, Thompson WW, Robin L, Washko RM, Noah DL, Barrett DH, Randall B, Herwaldt BL, Mawle AC, Reeves WC: Chronic multisymptom illness affecting Air Force veterans of the Gulf War. JAMA 1998; 280:981–98
- Reeves WC, Lloyd A, Vernon SD, Klimas N, Jason LA, Bleijenberg G, Evengard B, White PD, Nisenbaum R, Unger ER, International Chronic Fatigue Syndrome Study Group: Identification of ambiguities in the 1994 chronic fatigue syndrome research case definition and recommendations for resolution. BMC Health Serv Res 2003; 3(1):25; PMID:14702202; http://dx.doi.org/10.1186/1472-6963-3-25
- Collins JF, Donta ST, Engel CC, Baseman JB, Dever LL, Taylor T, Boardman KD, Martin SE, Wiseman AL: Feussner JR. The antibiotic treatment trial of Gulf War Veterans' Illnesses: issues, design, screening, and baseline characteristics. Control Clin Trials 2002; 23(3):333–53; http://dx.doi.org/10.1016/S0197-2456(02)00192-7
- Liberman AC, Druker J, Refojo D, Holsboer F, Arzt E. Glucocorticoids inhibit GATA-3 phosphorylation and activity in T cells. FASEB J 2009; 23:1558–71; PMID:19124555; http://dx.doi.org/10.1096/fj.08-121236
- Rider CF, Shah S, Miller-Larsson A, Giembycz MA, Newton R. Cytokine-induced loss of glucocorticoid function: effect of kinase inhibitors, long-acting β(2)-adrenoceptor ; corrected agonist and glucocorticoid receptor ligands. PLoS One 2015; 10(1):e0116773; PMID:25625944; http://dx.doi.org/10.1371/journal.pone.0116773
- Craddock TJ, Harvey JM, Nathanson L, Barnes ZM, Klimas NG, Fletcher MA, Broderick G. Using gene expression signatures to identify novel treatment strategies in gulf war illness. BMC Med Genomics 2015 8:36; PMID:26156520; http://dx.doi.org/10.1186/s12920-015-0111-3
- van der Knaap LJ, Riese H, Hudziak JJ, Verbiest MM, Verhulst FC, Oldehinkel AJ, van Oort FV. Glucocorticoid receptor gene (NR3C1) methylation following stressful events between birth and adolescence. The TRAILS study. Transl Psychiatry 2014 Apr 8; 4:e381; http://dx.doi.org/10.1038/tp.2014.22
- Yehuda R, Flory JD, Bierer LM, Henn-Haase C, Lehrner A, Desarnaud F, Makotkine I, Daskalakis NP, Marmar CR, Meaney MJ. Lower methylation of glucocorticoid receptor gene promoter 1F in peripheral blood of veterans with posttraumatic stress disorder. Biol Psychiatry 2015; 77(4):356–64; PMID:24661442; http://dx.doi.org/10.1016/j.biopsych.2014.02.006
- Elser B, Lohoff M, Kock S, Giaisi M, Kirchhoff S, Krammer PH, Li-Weber M. IFN-gamma represses IL-4 expression via IRF-1 and IRF-2. Immunity 2002 Dec; 17(6):703–12; http://dx.doi.org/10.1016/S1074-7613(02)00471-5
- Bernabei P, Bosticardo M, Losana G, Regis G, Di Paola F, De Angelis S, Giovarelli M, Novelli F. IGF-1 down-regulates IFN-gamma R2 chain surface expression and desensitizes IFN-gamma/STAT-1 signaling in human T lymphocytes. Blood 2003; 102(8):2933–9; PMID:12842994; http://dx.doi.org/10.1182/blood-2003-01-0100
- Wynes MW, Riches DW. Induction of macrophage insulin-like growth factor-I expression by the Th2 cytokines IL-4 and IL-13. J Immunol 2003; 171(7):3550–9; PMID:14500651; http://dx.doi.org/10.4049/jimmunol.171.7.3550
- Frey RS1, Gao X, Javaid K, Siddiqui SS, Rahman A, Malik AB. Phosphatidylinositol 3-kinase gamma signaling through protein kinase Czeta induces NADPH oxidase-mediated oxidant generation and NF-kappaB activation in endothelial cells. J Biol Chem 2006; 281(23):16128–38; PMID:16527821; http://dx.doi.org/10.1074/jbc.M508810200
- Kar S, Seto D, Doré S, Hanisch U, Quirion R. Insulin-like growth factors-I and -II differentially regulate endogenous acetylcholine release from the rat hippocampal formation. Proc Natl Acad Sci USA 1997; 94(25):14054–9; PMID:9391151; http://dx.doi.org/10.1073/pnas.94.25.14054
- Seto D, Zheng WH, McNicoll A, Collier B, Quirion R, Kar S. Insulin-like growth factor-I inhibits endogenous acetylcholine release from the rat hippocampal formation: possible involvement of GABA in mediating the effects. Neuroscience 2002; 115(2):603–12; PMID:12421625; http://dx.doi.org/10.1016/S0306-4522(02)00450-5
- Barbier L., Diserbo M., Lamproglou I., Amourette C., Peinnequin A., Fauquette W. Repeated stress in combination with pyridostigmine Part II: changes in cerebral gene expression. Behav Brain Res 2009; 197(2):292–300; PMID:18796314; http://dx.doi.org/10.1016/j.bbr.2008.08.032
- Hintze A, Adami C. Evolution of complex modular biological networks. PLoS Comput Biol 2008; 4(2):e23; PMID:18266463; http://dx.doi.org/10.1371/journal.pcbi.0040023