Abstract
Although the gut microbial metabolites exhibit potential effects on coronary heart disease (CHD), the underlying mechanism remains unclear. In this study, the active gut microbial metabolites acting on CHD and their potential mechanisms of action were explored through a network pharmacological approach. We collected a total of 208 metabolites from the gutMgene database and 726 overlapping targets from the similarity ensemble approach (SEA) and SwissTargetPrediction (STP) database, and ultimately identified 610 targets relevant to CHD. In conjunction with the gutMGene database, we identified 12 key targets. The targets of exogenous substances were removed, and 10 core targets involved in CHD were eventually retained. The microbiota–metabolites–targets–signalling pathways network analysis revealed that C-type lectin receptor signalling pathway, Lachnospiraceae, Escherichia, mitogen-activated protein kinase 1, prostaglandin-endoperoxidase synthase 2, phenylacetylglutamine and alcoholic acid are notable components of CHD and play important roles in the development of CHD. The results of molecular docking experiments demonstrated that AKT1-glycocholic acid and PTGS2-phenylacetylglutamine complexes may act on C-type lectin receptor signalling pathways. In this study, the key substances and potential mechanisms of gut microbial metabolites were analysed via network pharmacological methods, and a scientific basis and comprehensive idea were provided for the effects of gut microbial metabolites on CHD.
Introduction
Coronary heart disease (CHD) is one of the most common cardiovascular diseases, which is caused by stenosis, spasm or blockage of the coronary artery lumen, and leads to myocardial ischaemia, hypoxia or necrosis [Citation1]. Chronic ischaemia from coronary artery stenosis or rupture and myocardial infarction can lead to heart failure and even death [Citation2]. CHD, as a common cardiovascular disease, has a high incidence worldwide, and its morbidity and mortality are on the rise and the trend of younger. Modern medicine controls the incidence of CHD by actively reducing risk factors such as smoking, hyperglycaemia, hypertension and lipid metabolism disorders [Citation3]. Drugs for treating CHD usually act on individual targets, such as beta-blockers and angiotensin converting enzyme inhibitors, but causing unavoidable side effects and drug resistance [Citation4]. Therefore, a more effective and safe way for treating CHD is particularly necessary.
The gastrointestinal system of the human body is home for a large number and rich variety of microbes, including bacteria, viruses, fungi and protozoa, which are collectively known as the gut microbiota [Citation5]. Gut microbiota plays an important role in human health and disease, and bacteria can be subdivided into three categories, namely pathogenic bacteria, probiotics and neutral bacteria, according to the different benefits and disadvantages of gut microbiota to the host [Citation6]. Firmicutes and Bacteroidetes account for more than 90% of the gut microbiota community, and as the key dominant bacteria, their ratio is an important indicator of gut microbial disorder [Citation7]. The balance of microbiota structure and proportion is the basis to maintain the stability of intestinal microecology [Citation8]. The normal microbiota, after long evolution with the host, has formed an interdependent and mutually limited homeostasis system, which can produce beneficial metabolites for the treatment of diseases [Citation9]. When intestinal microecology imbalance or dysbiosis occurs, glucose and lipid metabolism disorders and systemic inflammation levels increase, which gradually promote hypertension, atherosclerosis, CHD, myocardial infarction and heart failure [Citation10].
Studies have shown that gut microbiota are associated with neurological, metabolic, liver and cardiovascular diseases. Microbial products are associated with the development and progression of cardiac complications through a variety of mechanisms, such as differences in intestinal permeability and the secretion of some secondary bile acids and short-chain fatty acids (SCFAs) [Citation11]. In particular, gut microbiota plays a key role in the maintenance of cardiovascular function by converting exogenous and endogenous compounds into metabolites [Citation12]. For instance, bile acids excretion levels have been shown to be significantly reduced in patients with CHD compared with those without CHD, and bile acids may be a useful biomarker for predicting the severity of CHD [Citation13,Citation14]. SCFAs produced by the gut microbiota regulate the immune response. Studies have shown that butyrate stimulates the formation of Nlrp3 inflammasome, which affects the involvement of inflammasome in the inflammatory response in the process of atherosclerosis [Citation15,Citation16]. Epicardial adipose tissue serves as a local storage site for metabolizing free fatty acids (FFAs) and has the ability to distribute cytokines and FFAs directly to the coronary arteries and myocardial tissue, exerting both endocrine and paracrine effects [Citation17]. Activation of FFA receptor 3 by SCFAs leads to an increase in sympathetic nervous system activity in the heart by promoting neuronal firing and the synthesis and release of norepinephrine resulting in CHD [Citation18]. Although the number of gut microbial metabolites is not fully understood, several metabolites have been tested to determine positive or negative effects on the development of CHD [Citation19]. In addition, the active gut microbial metabolites and their pharmacological mechanisms on CHD have not been reported. However, gut microbiota regulate human health through various metabolic pathways, which may be a new direction for the prevention of CHD. Network pharmacology is a new subject which studies the mechanism of disease and drug action through biological network based on the theory of systems biology [Citation20]. Due to the complex metabolites, multiple targets and wide signalling pathways of the gut microbiota, these characteristics make it challenging to further study. Therefore, in the current research on metabolites, more and more studies have been incorporated into the network pharmacology technology to provide more basic and scientific explanations for the mechanism of action.
In this study, network pharmacology was utilized to analyse a multi-factorial and very complex process, including key microbiota, signalling pathways, targets and metabolites, in CHD. The metabolite-target network of gut microbiota and the protein–protein interaction (PPI) network of metabolite–CHD interaction targets were established. Signalling pathways for biological function and efficacy were analysed using Gene Ontology (GO) analysis and Kyoto Encyclopaedia of Genes and Genomes (KEGG) enrichment. Finally, molecular docking techniques were used to verify the proposed functional metabolites and main targets. Therefore, this study aims to explore the pharmacological mechanism of gut microbial metabolites, and provide a theoretical basis for subsequent studies on the influence of gut microbiota on CHD. The workflow is represented in .
Methods
Selection of gut microbial metabolites and targets
The gutMGene v1.0 database (http://bio-annotation.cn/gutmgene/) was used for searching metabolites and targets of gut microbiota, and the Simplified Molecular Input Line Entry System (SMILES) patterns of the metabolites were identified by PubChem (https://pubchem.ncbi.nlm.nih.gov/) [Citation21]. The targets associated with each metabolite of the gut microbiota were predicted in the “Homo sapiens” setting by similarity ensemble approach (SEA) [Citation22] (http://sea.bkslab.org/) and SwissTargetPrediction (STP) [Citation23] (http://www.swisstargetprediction.ch/). Further analysis of overlapping targets between SEA and STP databases was performed using the Venny 2.1.0 platform (https://bioinfogp.cnb.csic.es/tools/venny/index.html).
Seeking out the potential targets of CHD
With “coronary heart disease” as the keyword, GeneCards Database (https://www.genecards.org/), Online Mendelian Inheritance in Man Database (https://www.omim.prg/), Therapeutic Target Database (http://db.idrblab.net/ttd/) and DisGeNET Database (https://www.disgenet.org/home/) were used to search and screen the known CHD-related targets, and the repeated targets were discarded. However, there were too many targets in those databases, so targets with a target score greater than 50 in the GeneCards database and a Score greater than 0.1 in the DisGeNET database were reserved. UniProt knowledge base (https://www.uniprot.org/) was used to get the standard target’s name with the organism selected as “Homo sapiens”. Finally, the potential targets of microbial metabolites acting on CHD were obtained by using the Venny 2.1.0 platform. Then, the key targets were recognized between the potential targets and the gutMGene database. Immediately, we removed the key targets of metabolite action produced by substrate metabolism and retained the targets of metabolites produced by gut microbes as core targets.
Construction of the protein–protein interaction network
Cytoscape is a network biology visualization and analysis application that visualizes molecular connections and biological processes (BPs). Core targets were analysed using STRING (https://string-db.org/), and then ‘tsv’ file was downloaded to import Cytoscape 3.8.2 software to construct the PPI network. The organism was set to “Homo sapiens” and the minimum required interaction score was “medium confidence (0.4)”, and then the PPI network was constructed. A target with the highest degree value in the PPI network was considered a hub target.
Analysis of Gene Ontology and Kyoto Encyclopaedia of Genes and Genomes pathways of gut microbial metabolites
The core targets were imported into the DAVID platform (https://david.ncifcrf.gov/tools.jsp) for GO function enrichment analysis and KEGG pathway enrichment analysis, and the “select species” was set to “Homo sapiens”. GO enrichment analysis of the core targets involved the BP, cellular component (CC) and molecular function (MF), and the results were visualized as bar graphs. The potential signalling pathways associated with the core targets were obtained using KEGG pathway enrichment analysis. Similarly, KEGG data were uploaded to the Bioinformatics (http://www.bioinformatics.com.cn/) platform for visual analysis.
Construction of the microbiota–metabolites–targets–signalling pathways (MMTS) network
The MMTS network diagram was constructed using Cytoscape 3.8.2 software, and the gut microbiota, metabolites, targets and signalling pathways were analysed using network analyser plug-in. In the MMTS network, each component or target is represented by a node, and the relationship between the component and the target is represented by a connecting line.
The docking testing of metabolites and targets for molecular
First, we downloaded the 3D structures of the metabolites from the PubChem database and saved them in “sdf” format, and then downloaded the 3D structures of the core targets from the PDB database (https://www.rcsb.org/) and saved them in “pdb” format. We would use Chem 3D software to construct their 3D structures for some metabolites, which did not have 3D structures in the database. These metabolites and targets were prepared for ligands and receptors by using AutoDockTools software, which included operations such as dehydration, hydrogenation and deionization. Finally, these metabolite-target pairs were then docked, and the lower binding energy docking results were visualized by LigPlot and PyMOL software.
Evaluation of drug-likeness properties
The SwissADME (http://www.swissadme.ch/) and the literature were used to evaluate the drug-like properties of metabolites associated with important signalling pathways [Citation24]. Commonly, metabolites have hydrophilic properties and have low bioavailability; therefore, we identified their physicochemical properties through an in silico strategy.
Toxicological evaluation by ADMETlab
One of key reason for failure of drug development is the lack of safety caused by some adverse effects: hERG blockers obstruct potassium channels and cause human hepatotoxicity (H-HT), respiratory toxicity, rat oral acute toxicity, carcinogenicity, drug induced liver injury (DILI), skin sensitization and lethal dose 50 (LD50) of acute toxicity. ADMETlab 2.0 (https://admetmesh.scbdd.com/) is a pharmacokinetics and toxicity prediction program available online [Citation25]. It is possible to use it to forecast medication absorption, distribution, metabolism, excretion and toxicity characteristics (ADMET). Thus, we confirmed the eight parameters by using ADMETlab platform.
Results
Acquisition of potential targets and metabolites
We obtained 208 metabolites and 223 targets from the gutMGene v1.0 database, which may have important implications for gut microbiota in the progress of CHD. We imported the SMILES form of metabolites into the SEA and STP databases, and obtained 1702 and 959 targets, respectively. Finally, 726 overlapping targets were obtained ().
Figure 2. (A) The number of overlapping 726 targets between SEA and STP database. (B) The number of overlapping 98 targets between the 726 targets and CHD-related targets. (C) The number of the final overlapping 12 targets between the 98 targets and targets of gut microbiota. (D) The PPI network (10 nodes and 38 edges).
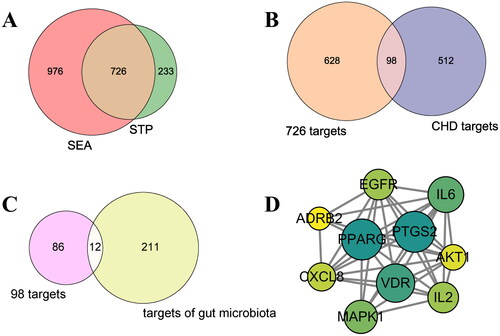
Core targets of metabolites of gut microbiota
We screened and removed duplicate values from GeneCards, OMIM, TTD and DisGeNET databases and obtained 610 CHD-related targets (). There were 98 overlapping targets between 726 targets and those directly related to CHD (610), and the final 12 key overlapping targets were identified between targets from gut microbiota (223) and 98 targets (). CASP3 and TLR4 were the targets for the post metabolic effects of exogenous substances d-fructose and chlorogenic acid, respectively. We removed two targets and left the other 10 targets as the core targets of gut microbial metabolites acting on CHD (Table S1).
PPI network and enrichment analysis
The PPI network consists of 10 nodes and 38 edges, the size of which depends on the degree of value (). In the network, node sizes are proportional to the target degree, and PPARG and CAPS3 are important hub targets in the network. The core targets have strong connections in the network, so we carry out enrichment analysis of the targets.
The 10 core targets were entered into the DAVID database for GO and KEGG enrichment analysis. The GO enrichment analysis results were reflected in three aspects: BP, CC and MF. Under the condition of p < .05, the 85, 10 and 12 items were obtained from the three aspects, respectively. According to the enrichment analysis results, MF include: enzyme binding, double-stranded DNA binding, nitric-oxide synthase regulator activity, identical protein binding, retinoid × receptor binding, RNA polymerase II transcription factor activity, protein phosphatase binding and protein binding ().
According to p value as screening condition, 10 pathways were screened through KEGG pathway analysis. Vertical axis represents enrichment pathway name and horizontal axis represents fold enrichment. A case-control study showed that the plasma levels of soluble C-type lectin-like receptor-2 (sCLEC-2) in the plasma of patients with coronary artery disease were significantly higher than those of healthy people. And CLEC-2 may be a useful biomarker for identifying high-risk patients with CHD [Citation26]. The serum high-sensitivity C-reactive protein (hsCRP) is known as an independent coronary risk factor. In subjects with low hsCRP, TLR4 expression level is higher in CAD patients than in non-CAD subjects. Therefore, serum hsCRP and peripheral blood mononuclear TLR4 expression may become a novel coronary risk marker [Citation27]. Similarly, it has been shown that annexin A3 (ANXA3) inhibits myocardial infarction and promotes myocardial tissue repair and healing through the PI3K/Akt signalling pathway [Citation28]. Therefore, the C-type lectin receptor, Toll-like receptor (TLR) and PI3K–Akt may be vital pathways affecting CHD (Figure 3(B)). In subsequent studies, we focused on the targets, metabolites and gut microbiota involved in these three pathways.
Identification of key components in the microbiota–metabolites–targets–signalling pathways network analysis
The MMTS network analysis was performed using the Cytoscape 3.8.2 software, comprising 79 nodes (38 microbiota, 10 signalling pathways, 10 targets and 21 metabolites) and 118 edges (Figure 4). A total of nine metabolites and five targets (IL-6, MAPK1, IL-2, AKT1 and PTGS2) were connected to identify the C-type lectin receptor signalling pathway by MMST network analysis. The TLR signalling pathway connects to five metabolites and four targets (IL-6, MAPK1, CXCL8 and AKT1). The PI3K–Akt signalling pathway connects to 11 metabolites and four targets (IL-6, MAPK1, AKT1, IL-2 and EGFR) (). The core targets must be intimately associated with metabolites, and we included these targets and metabolites in subsequent analyses.
Table 1. Metabolites and core targets of major signalling pathways.
Results of the molecular docking test
Molecular docking is the docking of ligands and receptors in the active pocket, which is a process accompanied by a change in binding energy. When the docking binding energy is less than 0 kcal·mol−1, it indicates that the receptor and ligand can spontaneously bind. The lower the binding energy is, the greater the possibility of binding, the more stable the binding conformation, and the greater the possibility of reaction. If the docking binding energy is less than −5 kcal·mol−1, it indicates that there is a good binding potential between the receptor and ligand. We performed molecular docking on seven targets and 16 metabolites associated with the above three signalling pathways. The docking results showed that all 21 kinds of receptor–ligand binding conformations had binding potential, but the binding conformation of MAPK1–palmitic acid had lower binding potential, with binding energy of −4.6 kcal·mol−1 (). AKT1–glycocholic acid, MAPK1–colibactin and EGFR–indole-3-acrylic acid have strong binding potential. As shown in , glycocholic acid could interact with Gln79(A), Cys296(A), Thr82(A) and Trp80(A) through a hydrogen bond in AKT1, their binding energy is −10.1 kcal·mol−1. As shown in , colibactin could interact with Lys342(A), Phe57(A), Gly35(A), Gly32(A) and Gln15(A) through a hydrogen bond in MAPK1, their binding energy is −7.8 kcal·mol−1. As shown in , indole-3-acrylic acid could interact with Met793(A) and Lys745(A) through a hydrogen bond in EGFR, their binding energy is −7.4 kcal·mol−1. As shown in , phenylacetylglutamine could interact with Gln372(A), Lys532(A) and His122(A) through a hydrogen bond in PTGS2, their binding energy is −7.0 kcal·mol−1. These results suggest that the binding of these receptors and ligands may play an important role in the development of CHD.
Figure 5. The molecular docking test on core targets-metabolites pairs. (A) Glycocholic acid-AKT1. (B) Colibactin-MAPK1. (C) Indole-3-acrylic acid-EGFR. (D) Phenylacetylglutamine-PTGS2.

Table 2. Molecular docking results of metabolites and core targets.
Identification of drug-likeness properties in silico
The ADME parameters of 16 metabolites were identified in silico. Colibactin, which violated Lipinski’s rule, specifically the topological polar surface area (TPSA), did not possess druglikeness properties. The remaining 15 metabolites exhibited acceptable physicochemical properties. Therefore, we propose that these metabolites may have a high potential to affect CHD ().
Table 3. The physicochemical properties of the metabolites from gut microbiota.
Toxicological properties of the 15 metabolites
The physicochemical properties of metabolites cannot be used as safety evaluation indexes, and toxicological characteristics are very necessary to evaluate their safety. Therefore, the possible toxicological properties of 15 metabolites were evaluated by the ADMElab online tool. Finally, we identified phenylacetylglutamine () and glycocholic acid () as promising metabolites based on toxicity via the ADMETlab platform, which would be of great value to be further developed ().
Table 4. Toxicological properties of the metabolites from the gut microbiota.
Discussion
CHD is one of the most important cardiovascular diseases threatening human health in current world, which has great significance for its prevention and treatment. Coronary atherosclerosis and coronary spasm are recognized as the main pathogenetic mechanisms of CHD. Gut microbiota and its metabolites play an important role in the diagnosis and treatment of cardiovascular disease [Citation29]. In recent years, the significant differences of gut microbiota were observed in CHD patients from clinical studies, and the changes of gut microbiota may cause the abnormal level of inflammatory factors and the disorder of glycaemic and lipid metabolism [Citation30,Citation31]. Network-based systematic pharmacology has been applied to the diagnosis of various diseases and the identification of related substances. This study showed that relevant human gut microbial metabolites can be detected using web-based systems pharmacology to uncover important microorganisms for treating CHD.
Our study shows that phenylacetylglutamine and glycocholic acid are promising metabolites that could bind stably to CXCL8, PTGS2 and AKT1 in the C-type lectin receptor, TLR and PI3K–Akt signalling pathway according to the MMTS network. Phenylacetylglutamine is produced by intestinal metabolism of Lachnospiraceae. Glycocholic acid is produced by Escherichia, Paraprevotella, Akkermansia, Carnobacterium, Ruminococcus flavefaciens, Butyricicoccus pullicaecorum, Mitsuokella multacida and Bacteroides fragilis. In addition, we confirmed that these two metabolite-target pairs have good binding activity and the metabolites are promising metabolites based on physicochemical properties and toxicity via the molecular docking and online platform.
It has been shown that CXCL8 mRNA expression was significantly increased in patients with coronary artery disease after oxidized low density lipoprotein, and CXCL8 level was associated with presentation of the disease [Citation32]. The pharmacological experiments have demonstrated that PTGS2 may increase the risk of CHD [Citation33]. Meanwhile, AKT1 is a key signalling target involved in the regulation of angiogenesis, which activates AKT1 signalling in endothelial cells to promote myocardial angiogenesis [Citation34]. Therefore, these core targets may play an important role in the occurrence and development of CHD. The CLRs, which are potent pattern recognition receptors, are crucial in maintaining the balance of the intestinal immune system [Citation35]. Inflammatory cells that are activated play a significant part in the progression of CHD. Meanwhile, suppression of the C-type lectin receptors signalling pathway, which has been implicated in inflammatory responses, may could control the development of CHD [Citation36,Citation37]. The TLR family is composed of 10 members (TLR1–TLR10) in humans. Similarly, inhibition of TLR2 blocks nuclear translocation of NF-κB and AP-1 in cardiac myocytes in response to oxidative stress in vitro [Citation38]. Activation of TLR4 induces the production of cytokines and chemokines, and induces the expression of costimulatory molecules and the production of inflammatory cytokines in dendritic cells [Citation39]. Furthermore, atorvastatin down-regulates TLR4 signalling through let-7i expression and plays a crucial role in immunity to coronary artery disease [Citation40]. Some studies have shown that triclosan may induce vascular injury by inhibiting PI3K/Akt/mTOR signalling pathway, and affect the vitality and angiogenesis of human umbilical vein endothelial cells [Citation41]. In addition, due to the activation of PI3K/Akt/eNOS signalling pathway, extracorporeal cardiac shock wave can promote the expression of Bcl-2, increase the production of NO, and inhibit the expression of Bax and Caspase3 in endothelial cells after hypoxic injury. Therefore, inhibition of PI3K/Akt/eNOS signalling pathway may be one of the pathological mechanisms that reduced endogenous vascular repair in CHD [Citation42]. Overexpression of PTEN inactivates the P13K/Akt signalling pathway, which mediates Bcl-2, Bax and Caspase-3 to promote apoptosis, thus inhibiting the occurrence of CHD [Citation43]. These pathways (C-type lectin receptors, TLR and PI3K–Akt signalling pathway) play an important role in the occurrence and development of CHD, and attention should be paid to the core targets, metabolites and gut microbiota involved in the network.
CHD is closely related to gut microbiota, and the relative abundance of unclassified Lachnospiraceae in CHD patients is significantly lower than that in healthy human [Citation44]. The abundance of Escherichia–Shigella in multivessel coronary artery disease patients’ group was significantly higher than that in normal group, and Escherichia–Shigella was positively correlated with plasma low-density lipoprotein and left atrial diameter, and negatively correlated with total bile acid [Citation45]. Paraprevotella is positively correlated with fibrinogen and negatively correlated with high-density lipoprotein cholesterol in patients with coronary artery disease [Citation46]. Therefore, Akkermansia, Carnobacterium, Ruminococcus Flavefaciens, Butyricicoccus Pullicaecorum, Mitsuokella Multacida and Bacteroides fragilis are also worthy of attention in the development of CHD.
Additionally, it was found that phenylacetylglutamine and glycocholic acid are produced by metabolism of Lachnospiraceae and Escherichia according to the MMTS network, respectively. Phenylacetic acid, converted from dietary protein, which is mediated by the por A gene of the gut microbiota, and the phenylacetic acid produced the phenylacetylglutamine by the effect of the liver enzyme. Phenylacetylglutamine may be positively correlated with cardiac inflammatory response and cardiac remodelling and a gut microbiota-dependent metabolite that promotes cardiovascular disease that signals via adrenergic receptors [Citation47,Citation48]. Regulating the production of phenylacetylglutamine by gut microbiota may be a new strategy for the treatment of CHD. Another metabolic glycocholic acid can reduce the ulcer index and microdamage of the colon, which may help gastrointestinal inflammation, and its impact on CHD should be studied, and it may be very interesting [Citation49].
In this study, crucial gut microbiota, significant signalling pathways, core targets and key metabolites against CHD were preliminary described through network pharmacology from a systematic perspective. In addition, associations and interactions using network medicine concepts can contribute to elucidate the relationship between microbiota and complex chronic diseases. In summary, we used the integration network to elucidate the potential mechanism of action in CHD. However, given the limitations of the database, this study requires further clinical trials to validate its therapeutic effect.
Conclusions
The metabolites of gut microbiota in treating CHD were investigated via a network pharmacology-based study. This article provides a theoretical basis that phenylacetylglutamine and glycocholic acid act on C-type lectin receptors, TLRs and the PI3K–Akt signalling pathway, and may be used as a new strategy to treat CHD. However, the intricate progression of diseases and the pharmacodynamic activities of gut microbial metabolites are constantly changing, while computer biotechnology, which involves static network analysis and has limited simulation time, is not able to fully capture these dynamics. Therefore, additional experiments and clinical trials are necessary to validate these findings. In the future, dynamic network data analysis or simulations with extended time frames could be developed to better align with real-world results, reducing the need for unnecessary trial-and-error experiments and aiding in expediting the pharmacological research of gut microbial metabolites. The gut microbial metabolites were obtained from literature and databases without experimental verification using LC/MS technology. In addition, further pharmacological experiments are required to validate the therapeutic mechanism of gut microbial metabolites on CHD. It is necessary to assess the safety of metabolites for preclinical studies. In conclusion, our findings also help reveal the therapeutic value of metabolites derived from the gut microbiota that require further validation.
Limitations
There are limitations in current research, particularly in terms of theoretical perspectives and reliance on data-driven approaches. There are still unidentified gut microbial metabolites and their corresponding action targets, which prevents a complete understanding of the pharmacological effects of these metabolites. Additionally, we also acknowledge the limitations of public databases, as they may not contain comprehensive information on certain diseases, focusing instead on popular research topics. The use of targets from a different database may lead to false negatives due to potential bias from varying experimental conditions. We have only confirmed the metabolite’s binding ability to the target through computer simulation, as well as assessed the drug-likeness and toxicological properties of the metabolites, and the results identified in this study need to be further verified through basic science and clinical studies. These are the difficulties that we need to overcome as we continue our research.
Author contributions
Conceiving and designing the full experiments: S.-J. Y. and Y.-P. T.; methodology, H.-M. Z. and S.-J. Y.; software, X.-Y. Y. and W.-X. W.; performing the experiments: H.-M. Z.; data analysis, H.-M. Z., S.-J. Y., X.-Y. Y, W.-X. W., Q. Z., D.-Q. X., J.-J. L. and Y.-P. T.; writing and editing original draft preparation, H.-M. Z. and X.-Y. Y.; funding acquisition, S.-J. Y. and Y.-P. T. All authors have read and agreed to the published version of the manuscript.
Supplemental Material
Download MS Excel (66.6 KB)Disclosure statement
No potential conflict of interest was reported by the author(s).
Data availability statement
All data generated or analysed during this study are included in this published article (and its Supplementary Information Files).
Additional information
Funding
References
- Dalen JE, Alpert JS, Goldberg RJ, et al. The epidemic of the 20th century: coronary heart disease. Am J Med. 2014;127(9):807–812. doi: 10.1016/j.amjmed.2014.04.015.
- Berry C, Corcoran D, Hennigan B, et al. Fractional flow reserve-guided management in stable coronary disease and acute myocardial infarction: recent developments. Eur Heart J. 2015;36(45):3155–3164. doi: 10.1093/eurheartj/ehv206.
- Akhabue E, Thiboutot J, Cheng JW, et al. New and emerging risk factors for coronary heart disease. Am J Med Sci. 2014;347(2):151–158. doi: 10.1097/MAJ.0b013e31828aab45.
- Li C, Zhang WY, Yu Y, et al. Discovery of the mechanisms and major bioactive compounds responsible for the protective effects of Gualou Xiebai Decoction on coronary heart disease by Network Pharmacology Analysis. Phytomedicine. 2019;56:261–268. doi: 10.1016/j.phymed.2018.11.010.
- Temraz S, Nassar F, Nasr R, et al. Gut microbiome: a promising biomarker for immunotherapy in colorectal cancer. Int J Mol Sci. 2019;20(17):4155. doi: 10.3390/ijms20174155.
- Kolátorová L, Lapčík O, Stárka L. Phytoestrogens and the intestinal microbiome. Physiol Res. 2018;67(Suppl. 3):S401–S408. doi: 10.33549/physiolres.934022.
- Jandhyala SM, Talukdar R, Subramanyam C, et al. Role of the normal gut microbiota. World J Gastroenterol. 2015;21(29):8787–8803. doi: 10.3748/wjg.v21.i29.8787.
- Emoto T, Yamashita T, Kobayashi T, et al. Characterization of gut microbiota profiles in coronary artery disease patients using data mining analysis of terminal restriction fragment length polymorphism: gut microbiota could be a diagnostic marker of coronary artery disease. Heart Vessels. 2017;32(1):39–46. doi: 10.1007/s00380-016-0841-y.
- Adak A, Khan MR. An insight into gut microbiota and its functionalities. Cell Mol Life Sci. 2019;76(3):473–493. doi: 10.1007/s00018-018-2943-4.
- Poli A. What connection is there between intestinal microbiota and heart disease? Eur Heart J Suppl. 2020;22(Suppl. L):L117–L120. doi: 10.1093/eurheartj/suaa149.
- Wang YL, Cheng YL, Shang LH. Research advance in gut microbiota in the pathogenesis of coronary artery disease. Chin Res Hosp. 2022;10(1):59–62.
- Groen RN, de Clercq NC, Nieuwdorp M, et al. Gut microbiota, metabolism and psychopathology: a critical review and novel perspectives. Crit Rev Clin Lab Sci. 2018;55(4):283–293. doi: 10.1080/10408363.2018.1463507.
- Li W, Shu S, Cheng L, et al. Fasting serum total bile acid level is associated with coronary artery disease, myocardial infarction and severity of coronary lesions. Atherosclerosis. 2020;292:193–200. doi: 10.1016/j.atherosclerosis.2019.11.026.
- Liu H, Tian R, Wang H, et al. Gut microbiota from coronary artery disease patients contributes to vascular dysfunction in mice by regulating bile acid metabolism and immune activation. J Transl Med. 2020;18(1):382. doi: 10.1186/s12967-020-02539-x.
- Marques FZ, Mackay CR, Kaye DM. Beyond gut feelings: how the gut microbiota regulates blood pressure. Nat Rev Cardiol. 2018;15(1):20–32. doi: 10.1038/nrcardio.2017.120.
- Yuan X, Wang L, Bhat OM, et al. Differential effects of short chain fatty acids on endothelial Nlrp3 inflammasome activation and neointima formation: antioxidant action of butyrate. Redox Biol. 2018;16:21–31. doi: 10.1016/j.redox.2018.02.007.
- Quarta S, Santarpino G, Carluccio MA, et al. Exploring the significance of epicardial adipose tissue in aortic valve stenosis and left ventricular remodeling: unveiling novel therapeutic and prognostic markers of disease. Vascul Pharmacol. 2023;152:107210. doi: 10.1016/j.vph.2023.107210.
- Carbone AM, Del Calvo G, Nagliya D, et al. Autonomic nervous system regulation of epicardial adipose tissue: potential roles for regulator of G protein signaling-4. Curr Issues Mol Biol. 2022;44(12):6093–6103. doi: 10.3390/cimb44120415.
- Zhang H, Jing L, Zhai C, et al. The intestinal flora metabolite trimethylamine oxide is inextricably linked to coronary heart disease. J Cardiovasc Pharm. 2023;81(3):175–182. doi: 10.1097/FJC.0000000000001387.
- Hopkins AL. Network pharmacology: the next paradigm in drug discovery. Nat Chem Biol. 2008;4(11):682–690. doi: 10.1038/nchembio.118.
- Oh KK, Gupta H, Min BH, et al. The identification of metabolites from gut microbiota in NAFLD via network pharmacology. Sci Rep. 2023;13(1):724. doi: 10.1038/s41598-023-27885-w.
- Keiser MJ, Roth BL, Armbruster BN, et al. Relating protein pharmacology by ligand chemistry. Nat Biotechnol. 2007;25(2):197–206.
- Gfeller D, Michielin O, Zoete V. Shaping the interaction landscape of bioactive molecules. Bioinformatics. 2013;29(23):3073–3079.
- Daina A, Zoete V. A boiled-egg to predict gastrointestinal absorption and brain penetration of small molecules. Chem Med Chem. 2016;11(11):1117–1121. doi: 10.1002/cmdc.201600182.
- Dong J, Wang NN, Yao ZJ, et al. ADMETlab: a platform for systematic ADMET evaluation based on a comprehensively collected ADMET database. J Cheminform. 2018;10(1):1–11. doi: 10.1186/s13321-018-0283-x.
- Fei M, Xiang L, Chai X, et al. Plasma soluble C-type lectin-like receptor-2 is associated with the risk of coronary artery disease. Front Med. 2020;14(1):81–90. doi: 10.1007/s11684-019-0692-x.
- Shiraki R, Inoue N, Kobayashi S, et al. Toll-like receptor 4 expressions on peripheral blood monocytes were enhanced in coronary artery disease even in patients with low C-reactive protein. Life Sci. 2006;80(1):59–66. doi: 10.1016/j.lfs.2006.08.027.
- Meng H, Zhang Y, An ST, et al. Annexin A3 gene silencing promotes myocardial cell repair through activation of the PI3K/Akt signaling pathway in rats with acute myocardial infarction. J Cell Physiol. 2019;234(7):10535–10546. doi: 10.1002/jcp.27717.
- Ascher S, Reinhardt C. The gut microbiota: an emerging risk factor for cardiovascular and cerebrovascular disease. Eur J Immunol. 2018;48(4):564–575. doi: 10.1002/eji.201646879.
- Cui L, Zhao T, Hu H, et al. Association study of gut flora in coronary heart disease through high-throughput sequencing. BioMed Res Int. 2017;2017:1–10. doi: 10.1155/2017/3796359.
- Peng Y, Zhang N, Li WJ, et al. Correlations of changes in inflammatory factors, glucose and lipid metabolism indicators and adiponectin with alterations in intestinal flora in rats with coronary heart disease. Eur Rev Med Pharmacol Sci. 2020;24(19):10118–10125.
- Oliveira RTD, Mamoni RL, Souza JRM, et al. Differential expression of cytokines, chemokines and chemokine receptors in patients with coronary artery disease. Int J Cardiol. 2009;136(1):17–26. doi: 10.1016/j.ijcard.2008.04.009.
- Xie X, Ma YT, Yang YN, et al. Interaction between COX-2 G-765C and smoking in relation to coronary artery disease in a Chinese Uighur population. Clin Chem Lab Med. 2011;49(1):55–60. doi: 10.1515/CCLM.2011.024.
- Schiekofer S, Belisle K, Galasso G, et al. Angiogenic-regulatory network revealed by molecular profiling heart tissue following Akt1 induction in endothelial cells. Angiogenesis. 2008;11(3):289–299. doi: 10.1007/s10456-008-9112-6.
- Li TH, Liu L, Hou YY, et al. C-type lectin receptor-mediated immune recognition and response of the microbiota in the gut. Gastroenterol Rep. 2019;7(5):312–321. doi: 10.1093/gastro/goz028.
- Ma X, Yin H, Chen K. Differential gene expression profiles in coronary heart disease patients of blood stasis syndrome in traditional Chinese medicine and clinical role of target gene. Chin J Integr Med. 2009;15(2):101–106. doi: 10.1007/s11655-009-0101-4.
- Zhou Y, Zhou H, Hua L, et al. Verification of ferroptosis and pyroptosis and identification of PTGS2 as the hub gene in human coronary artery atherosclerosis. Free Radic Biol Med. 2021;171:55–68. doi: 10.1016/j.freeradbiomed.2021.05.009.
- Frantz S, Kelly RA, Bourcier T. Role of TLR-2 in the activation of nuclear factor κB by oxidative stress in cardiac myocytes. J Biol Chem. 2001;276(7):5197–5203. doi: 10.1074/jbc.M009160200.
- Akira S, Takeda K, Kaisho T. Toll-like receptors: critical proteins linking innate and acquired immunity. Nat Immunol. 2001;2(8):675–680. doi: 10.1038/90609.
- Satoh M, Tabuchi T, Minami Y, et al. Expression of let-7i is associated with Toll-like receptor 4 signal in coronary artery disease: effect of statins on let-7i and toll-like receptor 4 signal. Immunobiology. 2012;217(5):533–539. doi: 10.1016/j.imbio.2011.08.005.
- Zhang M, Zhu R, Zhang L. Triclosan stimulates human vascular endothelial cell injury via repression of the PI3K/Akt/mTOR axis. Chemosphere. 2020;241:125077. doi: 10.1016/j.chemosphere.2019.125077.
- Wang M, Yang D, Hu Z, et al. Extracorporeal cardiac shock waves therapy improves the function of endothelial progenitor cells after hypoxia injury via activating PI3K/Akt/eNOS signal pathway. Front Cardiovasc Med. 2021;8:747497. doi: 10.3389/fcvm.2021.747497.
- Wang S, Cheng Z, Chen X. Promotion of PTEN on apoptosis through PI3K/Akt signal in vascular smooth muscle cells of mice model of coronary heart disease. J Cell Biochem. 2019;120(9):14636–14644. doi: 10.1002/jcb.28725.
- Choroszy M, Litwinowicz K, Bednarz R, et al. Human gut microbiota in coronary artery disease: a systematic review and meta-analysis. Metabolites. 2022;12(12):1165. doi: 10.3390/metabo12121165.
- Yu H, Li L, Deng Y, et al. The relationship between the number of stenotic coronary arteries and the gut microbiome in coronary heart disease patients. Front Cell Infect Microbiol. 2022;12:903828.
- Nakajima A, Mitomo S, Yuki H, et al. Gut microbiota and coronary plaque characteristics. J Am Heart Assoc. 2022;11(17):e026036.
- Wang CY, Wang M, Jiang H. The correlation between phenylacetylglutamine and atrial fibrillation. Chin J Cardiovasc Res. 2022;20(10):876–880.
- Nemet I, Saha PP, Gupta N, et al. A cardiovascular disease-linked gut microbial metabolite acts via adrenergic receptors. Cell. 2020;180(5):862–877.e22. doi: 10.1016/j.cell.2020.02.016.
- Oktar BK, Gülpınar MA, Ercan F, et al. Beneficial effects of glycocholic acid (GCA) on gut mucosal damage in bile duct ligated rats. Inflammation. 2001;25(5):311–318. doi: 10.1023/A:1012812616083.