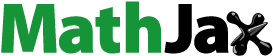
Abstract
Low energy inertia, a high penetration level of renewable energy sources, and a large ratio of power deviations in a small power transmission system have put microgrid frequency at risk of instability. Based on the close coupling between microgrid frequency and system security requirements, providing sufficient ancillary services from cost-effective and environmentally friendly resources is a major challenge in an efficient energy management system. In this article, a fuzzy approach and a new optimization technique on the PI controller are used to solve the problem of frequency stability in the AC micro-grid in the island mode by optimising the PI-fuzzy controller parameters. The suggested modified new self-organizing hierarchical PSO with jumping time-varying acceleration coefficients (MNHPSO-JTVAC) based controller’s results show that the control system has been investigated under three scenarios: load variations, RES uncertainty, white noise, and frequency correction. In terms of frequency responsiveness, the results are good when compared to traditional algorithms: The suggested design has been able to optimise the control system parameters by the proposed MNHPSO-JTVAC algorithm to solve microgrid frequency deviations caused by source uncertainty, load changes, and white noise, respectively, 0.008473, 0.01334, and 23.71, compared to classical PI control—PI, fuzzy And PI-fuzzy-GOA shows that in practical systems, this method can be considered very desirable and attractive.
1. Introduction
Today, power system operators face issues such as significant load changes, rapid demand growth, and geographical expansion of customers. On the one hand, due to environmental policies, investors do not have much desire to build fossil fuel power plants, and a new challenge has arisen in using power generation resources for power system operators. Currently, with the development of energy production technologies, increasing attention to environmental issues and an interest in improving the reliability of the electric network, the possibility and motivation to change distribution networks from inactive to active and the desire to produce renewable energy at the level of the distribution system are provided. On the other hand, the connection of scattered production sources to the current distribution networks has not met the technical and economic needs of investors, and while it was expected that the quality of electricity would improve with the increase in the penetration rate of scattered production sources due to power fluctuations caused by the difference in voltage and frequency of different renewable energy sources, the opposite results have been obtained. Therefore, the right solution is to build small networks independent of the main network or micro-network. A microgrid can be defined as a low-voltage network (such as a small urban area, a shopping center, or an industrial center) to which several small distributed energy production and storage units are connected. The microgrid can operate in two modes: connected to the grid and isolated from the main grid (Anees et al., Citation2021; Hardan et al., Citation2020; Jinglin et al., Citation2020; Khan et al., Citation2019; Sahu et al., Citation2018) In this regard, the concept of a microgrid (MG), which is a small power grid with several distributed generation sources and local loads, has been proposed. The microgrid is normally connected to the national grid, and in emergency situations, it is separated from the main grid due to the occurrence of heavy disturbances and can feed sensitive and important local loads alone (Sahu et al., Citation2020; Sahu, Chandra Prusty, Panda et al., Citation2022a, Citation2022b, Citation2021a, Citation2021b). Voltage and frequency control, in general It is one of the most important objectives of microgrid control in large disturbances, especially in sensitive feeders (Mohammadi et al., Citation2020). Among the presented control algorithms, PI controllers are the most commonly used in power systems due to their simplicity and cost-effectiveness. But the increase in the number of microgrids causes the change of the fundamental rules in these systems: the distribution of production resources; the increase in the complexity and non-linearity of the power grid; and the inadequacy of this controller. The reason for this is the offline adjustment of the coefficients of this controller based on the linear conditions and the working point of the system, which loses its optimality when the nominal operating conditions change or disturbance occurs. In order to solve this problem, adjustment of control coefficients is done depending on system changes.
1.1. Literature review
Many methods have been invented so far in order to control the frequency of micro-grids. For example, in (Solhtalab et al., Citation2022), an adaptive adjustment has been made through neural networks. Frequency adjustment in (Fathi et al., Citation2021) is based on the fuzzy algorithm and the particle cumulative optimization (PSO) method. Also in (Xiang et al., Citation2021), adaptive adjustment based on an emotional learning algorithm has been done. The authors in (Ahmadi-Nezamabad et al., Citation2019; Zand et al., Citation2020) have investigated the optimal power flow of an isolated microgrid for load capacity and related droop control constraints. However, hierarchical reserve scheduling is not considered and the frequency behaviour of the microgrid is modeled. Several multi-objective optimization methods based on the heuristic method have been reviewed in the literature. The authors in (Mohammad Zand et al., Citation2020) have presented a particle swarm optimization based on the operation scheduling of a renewable microgrid backed by battery/MT/FC to level the daily energy mismatch. In (Ghasemi et al., Citation2020), an intelligent energy management system has been investigated to optimise the forward operations of the microgrid. They have implemented a real genetic coding algorithm matrix to achieve a practical method for load management and control of energy resource distribution outputs according to different exploitation policies. Likewise, in (Nasri et al., Citation2021), a genetic algorithm is used to solve a non-linear exploitation optimization based on environmental and economic microgrids. In (Rohani et al., Citation2020), through a multi-objective optimization based on an efficient battery feeding algorithm, the author has optimised the operating costs and greenhouse gas production of a microgrid that considers the uncertainty of wind turbines. Reference (Zand et al., Citation2019), based on two-phase stochastic programming, has proposed a modified firefly adaptive algorithm to pursue optimal operation management of microgrids under various uncertainties. In (Lilia et al., Citation2020), an improved learning-inspired algorithm is used to plan the energy requirements of a microgrid with the objective of minimising greenhouse gas production and costs. Although the methods mentioned above may be effective, their solutions may be affected by the obstacles posed by heuristic algorithms in adapting to constrained problems. Also, (Zand et al.,) activates with the aim of providing optimal operation management of isolated microgrids, considering the assurance of emergency power supply services and reducing the global cost of generated power. Similarly, in (Mohammadi et al., Citation2021), using the nonlinear mixed integer programming method, the problem of energy management of an isolated microgrid is carried out in order to maximise the use of distributed energy resources with low future operating costs. However, in the sources mentioned above, the large effects of sudden load fluctuations or random changes in output power of renewable energy sources are ignored in microgrid energy planning. Also, provision of storage needs by end-user consumers is not considered.
In an interesting work (Aliyariyan et al., Citation2021), the primary and secondary frequency control loops were modeled; however, the sources of uncertainty were not modelled and the security requirements of the microgrid were not reported. In addition, no consideration has been given to load responsiveness in enhancing microgrid security. Through stochastic optimization, the future energy and storage resources of a microgrid have been managed. And also in (Zand et al., Citation2021), using some demand response providers, end-user consumers have been involved in microgrid energy and reserve planning. In fact, if DRP proposals are accepted, they will be required to participate in operational planning by regulating their energy consumption. Despite the efficiency of the proposed methods, the behaviour of frequency-dependent energy resource distribution and its effect on microgrid storage planning have not been developed. In (Sanjeevikumar et al., Citation2021), a new cost-effective frequency-dependent energy management system is proposed. The static performance of droop-controlled power supply distribution units is correctly modeled. Based on the obtained formulas, future energy reserves and reserve control are managed, which satisfy both security and economic objectives. In (Sanjeevikumar et al., Citation2021), the authors only focused on the static performance of VSI units, and no solution was proposed to deal with the low inertia stack in the microgrid. It is noteworthy that the low inertia of DG units based on VSI has a destructive effect on the dynamic security of the isolated microgrid, which should be considered by the hierarchical structure of the energy management system. Recently, under the concept of demand response in the smart grid initiative, consumers also have the opportunity to actively participate in planning the performance of power systems. Consumer participation can be done directly or through the triple DRP facility. According to the definition of DR (Gbadega & Kumar Saha, Citation2020; Shaqour et al., Citation2020; Velamuri et al., Citation2020), consumers can intervene in the energy management system, especially when microgrid reliability is discussed. Therefore, EMS can take advantage of the capacity of DR programmes in providing frequency control auxiliary services and economizing microgrid security management. For example, in (Shaqour et al., Citation2020), a linear auxiliary service demand response model is presented to combine demand response resources in energy and reserve planning of large-scale power systems. Also, the authors in (Geraee et al., Citation2018; Sahoo & Panda, Citation2018) have proposed a similar model for demand response based on gradual motivation, which can handle the system uncertainties in the functional planning of intelligent distributed power systems. In (Hayati & Karimi, Citation2020), the proposed DR response programmes are implemented through the intermediary of a DPR. In fact, DPR, as an active aggregator, is committed to the participation of small consumers in energy and reserve planning through representation between DSOs and small end-user consumers designated in DR plans. Make it possible.
On the other hand, although some research has been done in relation to different aspects of microgrid energy management systems, there is no work in the field of studying microgrid energy management considering both economic goals and security goals, especially with emphasis on frequency control issues. In (Jafari et al., Citation2018; Zahra et al., Citation2022), an innovative method based on multi-objective optimization for scheduling DERs in microgrids is presented. Also, in (Baldinelli et al., Citation2020), the problem of energy management of an isolated MG with the aim of maximising the use of DERs with a low operational cost has been carried out. However, in the above references, the large effects of sudden load fluctuations or random changes in RES power output have been neglected in microgrid resource planning. Also, no attention has been paid to the provision of microgrid storage requirements. In (Sánchez et al., Citation2020), using probability-based optimization, the storage and energy resources of a microgrid have been managed. However, the frequency-dependent behaviour of DERs and its effects on microgrid storage planning have not been investigated. In addition, in references (Ghanooni et al., Citation2020–Yang et al., Citation2020), the dynamic performance of frequency-based DERs has been properly modeled, but the detailed storage planning has been neglected, and the optimization process is carried out over a time horizon of several minutes and has not covered the energy requirements of the future. In addition, the large effects of RES unit uncertainties, such as wind turbines or photovoltaics, are not considered. Also, in (Mir et al., Citation2020), the primary and secondary frequency control loops are properly modeled, the corresponding uncertainty sources are not modeled, and the microgrid security requirements are not stated. By reviewing the references, a complete and detailed study vacancy can be seen in the energy management system limited to microgrid frequency security. Although detailed planning and improvement of primary and secondary storage resources considering microgrid frequency management is presented in(Abazari et al., Citation2019; Kayalvizhi & Vinod Kumar, Citation2017; Shayeghi & Younesi, Citation2019).
Although the previous methods presented for the adaptive adjustment of the secondary frequency controllers in the microgrid have been used effectively, performing the instantaneous and optimal adjustment based on more effective optimization algorithms with higher convergence speed and better performance is still It is noteworthy. On the other hand, addressing the effects of microgrid model uncertainty and noise within it is also very interesting and has not been investigated in previous research. In this article, the online intelligent technique based on more effective optimization has been used to evaluate the effects of changes in load and model parameters and the effect of white noise. In this method, PI controller parameters are automatically adjusted by a fuzzy mechanism and according to real-time measurements, and this fuzzy system is optimised instantaneously with the HPSO-TVAC algorithm in order to achieve optimal performance despite system changes. Due to the fact that this algorithm has a higher convergence speed and accuracy compared to other evolutionary algorithms, it will produce better answers compared to other common intelligent optimization methods. On the other hand, this optimization method has been used in many power systems and can have favourable results. It has not been tested for frequency control on the microgrid. Optimal adjustment of the presented control parameters provides many advantages for controlling the frequency of a microgrid with several sources of distributed generation (DG) and renewable energy (RES). The proposed design has the ability to eliminate the effects of load disturbances and the effects of the uncertainty of power system parameters and white noise. In order to show this capability, the results were tested on an AC test microgrid independent of the power grid, and in order to determine the superiority of the control plan, the results of this method were presented with the PI Ziegler-Nichols-PI. Fuzzy and PI-Fuzzy optimal control methods with GOA in research The former has been compared
2. Organization of the manuscript
The different parts of this paper are as follows: First, in Section 2, an overview of the AC micro-grid structure and its challenges is given, and in Section 3, the micro-grid test system in the island state is introduced. Section 4 summarises frequency control circuits, and Section 5 reviews conventional PI and fuzzy PI control schemes. Section 6 presents a new intelligent control strategy based on fuzzy logic and the MNHPSO-JTVAC algorithm. The simulation results are reported in Section 7. The results and summary are mentioned in Section 8.
3. The overall structure of micro-grids and their control strategies
The general structure of a micro-grid is shown in Figure (Mohammad Zand et al., Citation2020). The micro-grid and the global network are connected at a common connection point (PCP). The micro-sources used in these networks are connected to the main bus at the connection point (POC) using electronic power elements. For this reason, AC and DC sources in the micro-grid will be the path. DC sources such as solar and fuel cells with AC/DC elements to alternate DC voltage and AC sources with AC/DC/AC intermediaries to be used for frequency synchronisation in these systems. Due to the fluctuating power of distributed generation sources and the low inertia of these networks, the network’s basic parameters can be changed with the slightest disturbance. The stability of the micro-grid can be endangered . These control strategies in micro-grids must provide both connectivity and disconnection from the global network . A series of controllers are required for each of the micronutrients and for the electrical charges to apply the control methods. It is also necessary to have a central controller (MGCC) that simultaneously processes the information received from the micro-grid and the main network and decides, based on this information, on the micro-grid and micro-resource operating modes.
4. Case study
In many micro-grid control schemes, island mode is more important than network mode. Therefore, to test the proposed method, a micro-grid separate from the main power system is being considered. The test micro-grid includes a solar generator (DEG), solar cell (PV), wind turbine (WTG), fuel cell system (FC), and two storage sources: FESS and BESS. According to Figure , the micro-sources are connected to the main network through electronic power intermediaries and frequently It should be noted that, due to the focus of this paper on frequency stability, the simple frequency model of Figure of the system has been used to analyse its behaviour in the face of various disturbances. The nominal values of the frequency model parameters and DG units and times are given in Tables (Sahu et al., Citation2018). In this model D, the damping coefficient H, the inertia constant R, the drop constant Tt, the turbine time constant Tg, the TFESS generator time constant, the FESS time constant, and the TBESS time are the BESS time constants. As shown in Figure classical PI controller is designed to stabilise the micro-grid frequency.
Table 1. The values of the parameters used in the micro-grid frequency model
Table 2. Load values and rated power of scattered products
5. frequency control
In the indelible state, the power system is in equilibrium between production and consumption. If this equilibrium is lost due to a perturbation, the frequency will deviate from the nominal value. If system frequency fluctuations are not controlled, they can cause a lot of damage, even to the point of shutting down a production unit. Given the importance of this issue, the following are the control loops used to control the frequency in power systems:
5.1. Initial command
The frequency will fluctuate if there is a disturbance in the power system that upsets the balance between production and consumption. The primary control loop is the first loop to limit the frequency drop after a perturbation occurs. This control loop is based on the frequency-real power characteristic under EquationEquation 1(1)
(1) and is mounted on the generator itself.
6. Secondary control
The primary control loop limits the frequency drop but cannot return the frequency to the nominal value, so another complementary loop called the secondary frequency control should be used. This control loop often uses classical PI controllers to return the frequency to its original value. Adjusting these controllers is mainly based on classical approaches and trial and error. As mentioned earlier, classic PI controllers cannot provide the desired performance if the operating conditions change. One solution is to use fuzzy logic as an intelligent method for setting the instantaneous parameters of PI controllers. Therefore, in the following, we first express the fuzzy structure in the secondary ring setting.
7. Frequency adjustment with the conventional fuzzy PI controller
In traditional power systems, secondary frequency control is often performed using classical PI controllers, usually according to pre-set operating points. If working conditions change, this structure cannot provide the desired performance. One solution is to use fuzzy logic as an intelligent method for instantaneous adjustment of PI controller parameters (Sahu, Chandra Prusty, Panda et al., Citation2021a). The fuzzy PI controller uses frequency deviations and load perturbation inputs for adjustment and can thus compensate for classical controllers’ inability to cover complex systems with uncertainties and perturbations.
The fuzzy controller uses a set of rules to route the input variables (frequency deviations) and
load disturbance (to the output variables) KP (proportional gain) Ki (integral gain), listed in Table .
Table 3. Fuzzy rules
The membership function related to input and output variables is arranged as large negative (NL), medium negative (NM), small negative (NS), small positive (PS), medium positive (PM), and large positive (PL). Although the controller-fuzzy PI It has better performance than older methods, but its performance is highly dependent on fuzzy membership functions’ properties. Without accurate system information, it is impossible to accurately select fuzzy membership functions and achieve optimal performance over a wide range of operating conditions. Hence, the evolutionary algorithm is used to optimize the real-time membership functions.
8. Main results: Frequency control with optimal instantaneous PI-fuzzy controller with MNHPSO-JTVAC algorithm
In this section, to optimise the fuzzy structure for tuning the controller, PI uses an optimization method based on the MNHPSO-JTVAC evolutionary algorithm. It should be noted that the fuzzy rules in question are applied according to the triangular membership function, which is one of the most common functions. Also, parts of the fuzzy law are formed using the AND function with minimal interpretation, and the fuzzy inference system is used. In this paper, according to the characteristics of the micro-grid system and previous experiences with various intelligent methods, to set instantaneous functions in real-time Membership in Fuzzy-PI Control The MNHPSO-JTVAC algorithm is a flexible and powerful algorithm, which has the advantage of simplicity in execution and speed of convergence, achieving optimal results.
For the MNHPSO-JTVAC algorithm for the first time in 2020 . This algorithm is based on the novel PSO algorithm called MNHPSO-JTVAC, which is an improved version of the self-organizing hierarchical PSO with time-varying acceleration coefficients (MNHPSO-JTVAC). A modification has been made to the proposed MNHPSO-JTVAC algorithm in choosing the control parameters of MNHPSO-JTVAC.
The frequency f is usually between 0 and 100. In each iteration, one answer is selected as the best answer, and the new position of each MNHPSO-JTVAC is updated locally with a random step following the equation below:
Thus, the MNHPSO-JTVAC algorithm is summarized as follows: As mentioned earlier, the goal is to design an online controller based on the fuzzy logic of the MNHPSO-JTVAC algorithm to adjust the frequency of the AC micro-grid. The general framework of the proposed control scheme is shown in Figure . In the traditional PI method, kp and ki coefficients are set to 4.095 and 21.84, respectively.
9. Simulation results
In this section, to optimise the fuzzy structure for tuning the controller, PI uses an optimization method based on the MNHPSO-JTVAC evolutionary algorithm. It should be noted that the fuzzy rules in question are applied according to the triangular membership function, which is one of the most common functions. Also, parts of the fuzzy law are formed using the AND function with minimal interpretation, and the fuzzy inference system is used. In this paper, according to the characteristics of the micro-grid system and previous experience with various intelligent methods, we set instantaneous functions in real-time. Membership in Fuzzy-PI Control The MNHPSO-JTVAC algorithm is a flexible and powerful algorithm that has the advantages of simplicity in execution and speed of convergence in achieving optimal results. The convergence of the proposed algorithm is depicted in Figure . It is concluded that the proposed PI-Fuzzy-HPSO-JTVAC method can be sufficient to achieve an optimal solution.
The MNHPSO-JTVAC algorithm was first introduced in 2020 . This algorithm is based on the novel PSO algorithm called MNHPSO-JTVAC, which is an improved version of the self-organizing hierarchical PSO with time-varying acceleration coefficients (MNHPSO-JTVAC). A modification has been made to the proposed MNHPSO-JTVAC algorithm in choosing the control parameters of MNHPSO-JTVAC.
First scenario (1-S): In the first scenario, according to the first scenario (S-11), the pattern of load disturbances ΔPL in terms of p.u. It is applied according to Figure . Based on this model, the frequency response of the microgrid and its control effort are shown in Figures . As can be seen, the fuzzy PI-controller adjusts the MNHPSO-JTVAC, frequency of the system much better than other controllers and has good performance in settling time and rise rate.
In the first scenario, according to the second scenario (S-12) in Figure comparison is made between the controllers under conditions of severe load changes, which apply a large load disturbance of 0.1 p.u. It has been shown.
As is clear from the figure obtained from the application of load disturbances, the proposed method has a higher convergence speed and produces a smooth control signal, as shown in Figure .
9.1. Scenario 2: (s-2) answer to uncertainty
The second scenario shows the adaptive characteristics of the intelligent controller against uncertainty. The model parameters in the two procedures, where the first scenario (s-2) is changed according to Table , and the second scenario (s-22) according to Table . The frequency response closed-loop control efforts are shown in Figures . It can be seen that the proposed controller obtains the best response against parametric uncertainty.
Table 4. Uncertainty of MG parameters in the first scenario
Table 5. The degree of uncertainty of MG parameters in the second scenario
9.2. Scenario 3: (S-3) Noise response
In the third scenario, an attempt is made to examine the micro-grid under noisy conditions. In this scenario, white noise with a variable amplitude is applied according to Figure (b). The frequency response and the micro-grid control effort are given in Figures (a) and Figure , respectively. The results are visible. As in previous simulations, the proposed intelligent controller provides better performance than other controllers, both in terms of sitting time and amplitude of change.
Figure 15. Micro-grid frequency response under the influence of white noise—A) Micro-grid frequency response. B) White noise.

In the previous sections, the proposed intelligent controller’s performance compared to the classical PI, PI-fuzzy, and PI-fuzzy-GOA controls was observed after several testing stages. Now, to emphasise the effectiveness of the proposed intelligent controller, we consider the numerical criteria, the sum of the absolute error value (AEV) and the control signal changes (TV), based on the frequency deviation according to EquationEquations 8(8)
(8) and Equation9
(9)
(9) , respectively:
In the above equations ,
and T is the absolute magnitude of the frequency changes, the absolute magnitude of the control signal, and the simulation time. The above criteria have been calculated for different simulation scenarios, and the results are given in Tables . Scenario 1, the effects of step disturbance on load (S-11) and the effect of severe load disturbance (S-12) 0.1 p.u. on the frequency of the micro-grid system.
Table 6. Values calculated for the IAE Performance Criterion
Table 7. Values calculated for TV performance criteria
In the second scenario, the disturbance in the system parameters is investigated according to Table (S-21) and Table (S-22). Finally, in the third scenario (S-3), the effect of noise on micro-grid performance is investigated. The numerical values in Tables indicate that the proposed intelligent controller’s understanding is better than all other controllers in all simulation scenarios.
10. Conclusion
Conventional power systems with secondary frequency control often use classic PI controllers, usually according to pre-set operating points. If working conditions change, this structure cannot provide the desired performance. The optimal tuning presented in this paper offers many advantages for controlling a micro-grid frequency with multiple sources of distributed generation (DG) and renewable energy (RES). The proposed design demonstrates the ability to eliminate load disturbances and the effects of uncertainty in power system parameters and white noise. In this paper, an adaptive method is used to control the secondary frequency of an independent AC micro-grid. This controller has two levels, including a PI controller and a fuzzy system that uses the MNHPSO-JTVAC algorithm to improve its parameters. The proposed method has been able to optimise the control system parameters by the proposed MNHPSO-JTVAC algorithm to solve microgrid frequency deviations caused by source uncertainty, load changes, and white noise, respectively, 0.008473, 0.01334, and 23.71, compared to classical PI control—PI, fuzzy And PI-fuzzy- GOA shows that this method can be considered very desirable and attractive in practical systems. Therefore, the proposed frequency control method is significant for the reason that the combination of the PI method with meta-innovative methods so far has very little been done. On the other hand, the proposed meta-heuristic method is an improved method and it has been able to control the frequency optimally in all possible scenarios of a microgrid compared to the traditional PI method.
Table
Disclosure statement
No potential conflict of interest was reported by the author(s).
Additional information
Funding
References
- Abazari, A., Ghazavi Dozein, M., Monsef, H., & Wu, B. (2019). Wind turbine participation in micro-grid frequency control through self-tuning, adaptive fuzzy droop in de-loaded area. IET Smart Grid, 2(2), 301–19. https://doi.org/10.1049/iet-stg.2018.0095
- Ahmadi-Nezamabad, H., Zand, M., Alizadeh, A., Vosoogh, M., & Nojavan, S. (2019). Multi-objective optimization based robust scheduling of electric vehicles aggregator. Sustainable Cities and Society, 47, 101494. https://doi.org/10.1016/j.scs.2019.101494
- Aliyariyan, M., Fathi, D., Eskandari, M., & Mohammadi, M. H. (2021). Simulation and investigation of perovskite/nano-pyramidal GeSe solar cell: Realizing high efficiency by controllable light trapping. Solar Energy, 214, 310–318. https://doi.org/10.1016/j.solener.2020.11.063
- Anees, A., Dillon, T., Wallis, S., & Chen, Y. P. P. (2021). Optimization of day-ahead and real-time prices for smart home community. International Journal of Electrical Power & Energy Systems, 124, 106403. https://doi.org/10.1016/j.ijepes.2020.106403
- Baldinelli, A., Barelli, L., Bidini, G., & Discepoli, G. (2020). Economics of innovative high capacity-to-power energy storage technologies pointing at 100% renewable micro-grids. Journal of Energy Storage, 28, 101198. https://doi.org/10.1016/j.est.2020.101198
- Fathi, D., Eskandari, M., & Fathi, D. (2021). Improving the efficiency of perovskite solar cells via embedding random plasmonic nanoparticles: Optical–electrical study on device architectures. Solar Energy, 221, 162–175. https://doi.org/10.1016/j.solener.2021.04.038
- Gbadega, P. A., & Kumar Saha, A. (2020). Impact of incorporating disturbance prediction on the performance of energy management systems in micro-Grid. IEEE Access, 8, 162855–162879. https://doi.org/10.1109/ACCESS.2020.3021598
- Geraee, S., Mohammadbagherpoor, H., Shafiei, M., Valizadeh, M., Montazeri, F., & Feyzi, M. R. (2018). Regenerative braking of electric vehicle using a modified direct torque control and adaptive control theory. Computers & Electrical Engineering, 69, 85–97. https://doi.org/10.1016/j.compeleceng.2018.05.022
- Ghanooni, P., Yazdani, A. M., Mahmoudi, A., MahmoudZadeh, S., Ahmadi Movahed, M., & Fathi, M. (2020). Robust precise trajectory tracking of hybrid stepper motor using adaptive critic-based neuro-fuzzy controller. Computers & Electrical Engineering, 81, 106535. https://doi.org/10.1016/j.compeleceng.2019.106535
- Ghasemi, M. et al. (2020). An efficient modified HPSO-TVAC-based dynamic economic dispatch of generating units. Electric Power Components and Systems, https://doi.org/10.1080/15325008.2020.1731876
- Hardan, F., Norman, R., & Leithead, W. (2020). Model-based control of a VSC-based power generator with synthetic inertia provision in an isolated micro-grid. IET Generation, Transmission & Distribution, 14(22), 5037–5046. https://doi.org/10.1049/iet-gtd.2020.0304
- Hayati, Z. Z.M., & Karimi, G., “Short-channel effects improvement of carbon nanotube field effect transistors,” 2020 28th Iranian Conference on Electrical Engineering (ICEE), Tabriz, Iran, 2020, pp. 1–6, https://doi.org/10.1109/ICEE50131.2020.9260850.
- Jafari, M. et al. “Novel predictive fuzzy logic-based energy management system for grid-connected and off-grid operation of residential smart micro-grids.” IEEE Journal of Emerging and Selected Topics in Power Electronics (2018).
- Jinglin, L. et al. “Adaptive dynamic programming approach for micro-grid optimal energy transmission scheduling.” 2020 39th Chinese Control Conference (CCC). IEEE, 2020.
- Kayalvizhi, S., & Vinod Kumar, D. M. (2017). Load frequency control of an isolated micro grid using fuzzy adaptive model predictive control. IEEE Access, 5, 16241–16251. https://doi.org/10.1109/ACCESS.2017.2735545
- Khan, B., Haes Alhelou, H., & Mebrahtu, F. (2019). A holistic analysis of distribution system reliability assessment methods with conventional and renewable energy sources. AIMS Energy, 7(4), 413–429. https://doi.org/10.3934/energy.2019.4.413
- Lilia, T., Nasab, M. A., Yang, H., & Addeh, A. (2020). l, An intelligent system based on optimized ANFIS and association rules for power transformer fault diagnosis. ISA Transactions, 103, 63–74. https://doi.org/10.1016/j.isatra.2020.03.022
- Mir, M., Dayyani, M., Sutikno, T., Mohammadi Zanjireh, M., & Razmjooy, N. (2020). Employing a Gaussian Particle Swarm Optimization method for tuning Multi Input Multi Output‐fuzzy system as an integrated controller of a micro‐grid with stability analysis. Computational Intelligence, 36(1), 225–258. https://doi.org/10.1111/coin.12257
- Mohammadi, M. H., Fathi, D., & Eskandari, M. (2020). Nio@ gese core-shell nano-rod array as a new hole transfer layer in perovskite solar cells: A numerical study. Solar Energy, 204, 200–207. https://doi.org/10.1016/j.solener.2020.04.038
- Mohammadi, M. H., Fathi, D., & Eskandari, M. (2021). Light trapping in perovskite solar cells with plasmonic core/shell nanorod array: A numerical study. Energy Reports, 7, 1404–1415. https://doi.org/10.1016/j.egyr.2021.02.071
- Nasri, S. et al, 2021. Maximum power point tracking of photovoltaic renewable energy system using a new method based on turbulent flow of water-based optimization (TFWO) Under Partial Shading Conditions. 978-981-336-456-1
- Rohani, A. et al (2020). Three-phase amplitude adaptive notch filter control design of DSTATCOM under unbalanced/distorted utility voltage conditions. Journal of Intelligent & Fuzzy Systems, 10.3233/JIFS–201667.
- Sahoo, B. P., & Panda, S. (2018). Improved grey wolf optimization technique for fuzzy aided PID controller design for power system frequency control. Sustainable Energy, Grids and Networks, 16, 278–299. https://doi.org/10.1016/j.segan.2018.09.006
- Sahu, P. C. et al. (2020). Novel DQN optimised tilt fuzzy cascade controller for frequency stability of a tidal energy-based AC microgrid. International Journal of Ambient Energy, 1–13.
- Sahu, P. C., Chandra Prusty, R., & Panda, S. (2021a). Improved-GWO designed FO based type-II fuzzy controller for frequency awareness of an AC microgrid under plug in electric vehicle. Journal of Ambient Intelligence and Humanized Computing, 12(2), 1879–1896. https://doi.org/10.1007/s12652-020-02260-z
- Sahu, P. C., Chandra Prusty, R., & Panda, S. (2021b). Active power management in wind/solar farm integrated hybrid power system with AI based 3DOF-FOPID approach. Energy Sources, Part A: Recovery, Utilization, and Environmental Effects, 1–21.
- Sahu, P. C., Chandra Prusty, R., & Panda, S. (2022a). Frequency regulation of an electric vehicle-operated micro-grid under WOA-tuned fuzzy cascade controller. International Journal of Ambient Energy, 43(1), 2900–2911. https://doi.org/10.1080/01430750.2020.1783358
- Sahu, P. C., Chandra Prusty, R., & Panda, S. (2022b). Optimal design of a robust FO-Multistage controller for the frequency awareness of an islanded AC microgrid under i-SCA algorithm. International Journal of Ambient Energy, 43(1), 2681–2693. https://doi.org/10.1080/01430750.2020.1758783
- Sahu, P. C., Mishra, S., Prusty, R. C., & Panda, S. (2018). Improved-salp swarm optimized type-II fuzzy controller in load frequency control of multi area islanded AC microgrid. Sustainable Energy, Grids and Networks, 16, 380–392. https://doi.org/10.1016/j.segan.2018.10.003
- Sánchez, D., Melin, P., & Castillo, O. (2020). Comparison of particle swarm optimization variants with fuzzy dynamic parameter adaptation for modular granular neural networks for human recognition. Journal of Intelligence Fuzzy System, 38(3), 3229–3252. https://doi.org/10.3233/JIFS-191198
- Sanjeevikumar, P., Zand, M., Nasab, M. A., Hanif, M. A., & Bhaskar, M. S., “Spider community optimization algorithm to determine upfc optimal size and location for improve dynamic stability,” 2021 IEEE 12th Energy Conversion Congress & Exposition - Asia (ECCE-Asia), 2021, pp. 2318–2323, https://doi.org/10.1109/ECCE-Asia49820.2021.9479149
- Shaqour, A., Farzaneh, H., Yoshida, Y., & Hinokuma, T. (2020). Power control and simulation of a building integrated stand-alone hybrid PV-wind-battery system in Kasuga City, Japan. Energy Reports, 6, 1528–1544. https://doi.org/10.1016/j.egyr.2020.06.003
- Shayeghi, H., & Younesi, A. (2019). Mini/Micro-Grid Adaptive Voltage and Frequency Stability Enhancement. Journal of Operation and Automation in Power Engineering, 7(1), 107.
- Solhtalab, N., Mohammadi, M. H., Eskandari, M., & Fathi, D. (2022). Efficiency improvement of half-tandem CIGS/perovskite solar cell by designing nano-prism nanostructure as the controllable light trapping. Energy Reports, 8, 1298–1308. https://doi.org/10.1016/j.egyr.2021.12.038
- Velamuri, S., Pachauri, N., & Vigneysh, T. (2020). Decentralized control strategy for fuel cell/PV/BESS based microgrid using modified fractional order PI controller. International Journal of Hydrogen Energy.
- Xiang, Q., Liao, Z., & Tinghui, L. (2021). A novel control strategy of the seamless transitions between grid-connected and islanding operation modes for the multiple complementary power microgrid. International Journal of Electronics, 108(8), 1381–1400. https://doi.org/10.1080/00207217.2020.1870726
- Yang, H., Zhu, Z., Li, C., & Li, R. (2020). A novel combined forecasting system for air pollutants concentration based on fuzzy theory and optimization of aggregation weight. Applied Soft Computing, 87, 105972. https://doi.org/10.1016/j.asoc.2019.105972
- Zahra, Z., Saba, K., & Hayati, M. (2022, December). Design of GaAs-thin film solar cell using TiO2 hemispherical nanoparticles array. Optics & Laser Technology, 156, 108608. https://doi.org/10.1016/j.optlastec.2022.108608
- Zand, M., Azimi Nasab, M., Khoobani, M., Jahangiri, A., Hossein Hosseinian, S., & Hossein Kimiai, A. (2021). Robust speed control for induction motor drives using STSM control. In 2021 12th Power Electronics, Drive Systems, and Technologies Conference (PEDSTC) (pp. 1-6). (IEEE). https://doi.org/10.1109/PEDSTC52094.2021.9405912
- Zand, M., Nasab, M. A., Hatami, A., Kargar, M., & Chamorro, H. R., 2020 “Using Adaptive Fuzzy Logic for Intelligent Energy Management in Hybrid Vehicles,” 2020 28th ICEE, pp. 1–7, https://doi.org/10.1109/ICEE50131.2020.9260941.IEEE Index
- Zand, M., Nasab, M. A., Neghabi, O., Khalili, M., & Goli, A. (2019). “Fault locating transmission lines with thyristor-controlled series capacitors By fuzzy logic method,” In 2020 14th International Conference on Protection and Automation of Power Systems (IPAPS), Tehran, Iran, 2019, pp. 62–70. IEEE. https://doi.org/10.1109/IPAPS49326.2019.9069389
- Zand, M., Nasab, M. A., Sanjeevikumar, P., Maroti, P. K., & Holm-Nielsen, J. B. (2020). Energy management strategy for solid‐state transformer‐based solar charging station for electric vehicles in smart grids. IET renewable power generation, 14(18), 3843–3852. https://doi.org/10.1049/iet-rpg.2020.0399
- Zand, M., Neghabi, O., Nasab, M.A., Eskandari, M., & Abedini, M. (2020, December). A hybrid scheme for fault locating in transmission lines compensated by the TCSC. In 2020 15th International Conference on Protection and Automation of Power Systems (IPAPS), (pp. 130–135). IEEE. https://doi.org/10.1109/IPAPS52181.2020.9375626