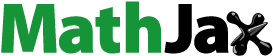
Abstract
Customer segmentation is a critical step toward appropriately differentiating services to different customers. One common way of segmenting customers is by using what is called Recency, Frequency, Monetary (RFM) approach, where customers are classified based on the recency of their transactions as well as how often they purchase goods and services and how much money they spent. However, this approach is not able to fairly differentiate customers especially when it comes to the cases where old customers have decreased or stopped their purchases and the new customers just started buying. In order to overcome this, we proposed what is called Multi Layer Recency, Frequency, and Monetary (MLRFM) approach. In this approach, we divide time periods into multiple layers and the recency, frequency, and monetary values are analyzed considering these different segments. Our numerical examples show that this multi layer approach can provide a good alternative for the companies that sell products online and customers are behaving very dynamically.
1. Introduction
The Recency, Frequency, Monetary (RFM) model was first proposed by Hughes (Citation1996) to analyze and predict customer behavior. RFM is a fairly effective and simple method that can be applied in market segmentation (Birant, Citation2011). RFM analysis is widely used to determine customer ratings based on customer purchase history information that has been recorded in the past. This method is used in various applications that involve large numbers of customers, such as online purchases, retailing, and others (Christy et al., Citation2021). In this method, customers will be grouped based on three dimensions, namely Recency (R), Frequency (F), and Monetary (M).
The recency value is measuring how recent the last transaction is. It can be measured by the number of days/periods between the last transaction made by the customer and today. The frequency value is the number of transactions made by the customer within a certain period of time. While the monetary value is the total money spent by the customer in a certain period. In the grouping, each customer is given a different score for the recency, frequency, and monetary variables. The score is given using a scale from 5 to 1, where the top quintile is given a score of 5, and the lowest quintile will be given a score of 1. The existing scores are assumed to have unique characteristics as in the example in Table .
Table 1. RFM score description
Then, all customers who have been given a score for each recency, frequency, and monetary will be grouped based on their total score (Haiying & Yu, Citation2010, May). Customers who have a score of 5, 5, 5 will be classified as potential customers for the company. On the contrary, customers who have a score of 1, 1, 1 are customers who are classified as less able to provide benefits for the company.
The problem or weakness of this RFM model is that it may not be fair in some situation. For example, let's say there is a new customer who orders continuously every day within the last three months so that it has a total number of orders (Frequency) of 90. On the other hand, there is an old customer who has been ordering very frequently in the past, but not really in the recent period. With a possibility that this large customer discontinued their business, then it should not be placed higher in the ranking compared to the new customer.
The same problem is also faced in terms of the total amount of revenue (Monetary), for example, every day in the last three months, a new customer places an order where each order contributes 10,000 to the revenue, so the total contribution to the revenue is 90 orders × 10,000 = 900,000. While old customers have a large number of orders that are collected over several years (for example, 300 orders) with each order contributing 10,000. Existing customers will have a total revenue contribution of 300 orders × 10,000 = 3,000,000. Of course, the new customers will not be able to beat the old ones. But the old customer in the last three years has never placed an order at all which could be because that old customer has switched to other companies. So, this old customer should no longer be suitable to get a high priority.
Customer segmentation based on recency, frequency, and monetary factors is very important to maintain a level of fairness towards old and new customers. This should apply in any situation, conventional or online markets. However, it should be quite obvious to note that a more accommodative approach to segmentation is needed in the era of disruption that gave birth to online businesses such as airbnb, uber, didi chuxing, grab, gojek, amazon, alibaba, airbnb, etc. The online businesses are characterized by rapid transactions and highly dynamic customers. Customers can switch to other providers easily and thus old and new customers may leave and enter quickly. With this situation, the conventional segmentation approach may no longer be very accurate in reflecting these dynamics.
To overcome these problems, this study proposes a model that divides the assessment of the frequency and monetary value in several stages/layers. These layers are used to separate transactions based on certain time periods, for example, last one week, last one month, last three months, last one year, etc. By doing this, the company can see the activity of each customer based on a certain period of time. Each layer can also be assigned a certain weight, for example, the weight for the last one week is greater than the weight for the last three months, the weight for the last three months is greater than the weight for the last one year and the weight for the last one year is greater than the weight for the last three months, and so on.
This proposed method is expected to make the division/clustering of priorities from customers into a proportional assessment in accordance with the stages of division of areas/zones that are more layered and have a weight scale according to the company’s needs. With this proposed method, new customers also get a fairly high priority when compared to old customers who have not ordered for a long time. This proposed method aims to create a fair assessment of old and new customers by considering transactions made by customers based on a certain period of time. In addition to being applicable to the customer side, this proposed method can also be applied to business service providers such as drivers, home/hostel owners, online shop owners, etc.
The rest of this paper is organized as follows. Section 2 contains review of businesses under the disruption era. Section 3 covers some of the relevant literature around RFM method. In Section 4, this paper presents the proposed method with an implementation example. Finally, in Section 5, this paper finishes by conclusion and discussing possible extensions and future works.
2. Business under disruption era
According to Cambridge Dictionary, disruptive technology is a new technology that completely changes the way things are done. This term was coined by Bower and Christensen in their article in Harvard Business Review in 1995 (Bower and Christensen, Citation1995). Disruptive technology significantly alters the way businesses, industries and even consumers operate. It significantly changes the cost or behavior of a business to either a product or a service. Disruptive technologies are radically changing the way information is gathered, the processes of business interaction, and the manufacturing of products. These disruptive technologies create impact on how individuals, businesses, and governments interact with one another to create markets, conduct business transactions, deliver services, create new business opportunities, and create new markets (International Finance Corporation, Citation2019).
The emergence of disruptive technologies provides a very drastic change in business models. For example, online transportation companies do not need to own vehicles, digital retailers do not own goods, and lodging companies do not need to own hotels or houses to rent. This creates uncertainty in business, but on the other hand it creates new business and employment opportunities (World Bank, Citation2019).
Today, many of the new business are conducted online. Merchants of goods/services, and customers interact through a software platform that has been provided by the company platformer by a third party. Under this online platform, it easy for traders and customers to join/leave. Old and new customers will be able to do business on/off easily. So that in conducting customer mapping, a mechanism is needed that is able to adopt changes in transactions that occur based on a shorter period of time.
3. RFM method
Every customer has different needs, wants, and behaviors. This is one of the challenges for the company to serve all customers in the best possible way. This causes companies to have to recognize every customer, especially in terms of recognizing potential customers in doing business. One of the methods used to identify customers is through customer segmentation. Customer segmentation is done by sorting customers into groups that have certain characteristics. These characteristics can be determined by each company according to their individual needs. For example, companies may wish to develop priority or segmentation based on profitability, loyalty, or other attributes (Bauer, Citation1988; Newell, Citation1997).
One method that is often used in segmenting customers is the RFM method. The RFM model has several basic advantages such as simplicity in the implementation process so that the method can be implemented quickly (Kahan, Citation1998). Another advantage is that its simplicity of results and processes so that the decision makers can easily interpret the results of the model obtained (Marcus, Citation1998). This model is quite capable of capturing customer characteristics using only a relatively small amount of data (Kaymak, Citation2001). Therefore, the RFM model is commonly used in customer analysis and customer segmentation.
The RFM model consists of three attributes, namely Recency (R), Frequency (F), and Monetary (M). Recency is reflecting the time of the last transaction (generally in days). This provides information about how long the last transaction made by the customer. Customers who have a small Recency value are assumed to be active customers. Frequency is the number of transactions made by customers within a certain period of time. It is considered an indication of loyalty, trust, faithfulness from customers. The higher the frequency value obtained, the higher the loyalty, the level of trust, the level of faithfulness from customers to the company. Monetary is the average amount of money that has been spent by the customer. Monetary factor is used to measure the customer’s contribution to the company’s revenue. The greater the amount spent, the more the customer’s contribution to the company’s revenue (customers who generate the most revenue for the company).
The RFM model has been widely applied in various fields, such as the retail industry (D. Chen et al., Citation2012; Y. L. Chen et al., Citation2009; Peker et al., Citation2017), banking (Firdaus & Utama, Citation2021; Nikumanesh & Albadvi, Citation2014), Hospital (Mohammadzadeh et al., Citation2017), in the field of Hotel Management (Changqiu, Citation2011), pork industry (Apichottanakul et al., Citation2021). In terms of method development, many researchers have developed RFM to meet various needs. For example, Chang and Tsay (Citation2004) developed the LRFM model by adding the Length (L) factor to the original RFM model. The addition of the L factor is used to calculate the time interval (in days) between the first transaction and the last transaction from the customer. The higher the L value, the more loyal the customer is considered.
Yeh et al. (Citation2009) proposed the RFMTC model by adding a factor of time since first purchase (T), and Churn probability (C), using Bernoulli sequence in probability theory. This model tries to predict when a customer will make the next transaction and how many times the customer will make transactions in the future. Chang and Tsai (Citation2011) has extended RFM model to GRFM model by adding product category group information. This model tries to group (group) the RFM values obtained by using the PICC (Purchased Items-Constrained Clustering) algorithm. It is hoped that with the new clustering, the purchasing characteristics of customers will be mapped more clearly. Mohammadzadeh et al. (Citation2017) initiated the LRFMP model by adding the Periodicity (P) factor to the LRFM model. Periodicity factor is intended to calculate the time interval of each purchase made by the customer. This is intended to identify the tendency of customers to conduct transactions on a regular basis. Cheng and Chen (Citation2009) used RFM method and K-Means algorithm to extract meaning rules in order to find out the characteristic of customer in order to strengthen Customer Relationship Management (CRM).
The various developments of the RFM method that have been carried out have not considered the problem of transaction factors carried out by customers based on a certain period of time. This has an impact on providing a fair assessment of priority segmentation for existing (old) and new customers. Considering that new customers who make transactions must lose to old customers from the factor of the number of transactions (frequency) and the total amount of money that has been spent (monetary). Another weakness is that the existing method has not taken into account the problem of the existence of old customers who make transactions at present but do not transact as often and as much as before (very rarely do transactions nowadays). This old customer will still have a large segmentation value (because of transactions made in the past) compared to new customers who are currently very active in transacting.
Based on these problems, a new method is proposed which aims to create a fair and detailed assessment for existing and new customers based on past and present transactions. The proposed method incorporates a period of time factor in order to perform a detailed customer segmentation analysis. The proposed method considers the assessment of customer segmentation based on a certain period of time where this time period can be divided into several time layers depending on the company’s business needs. Hence, this can be used to analyze customer activity in each period of time in detail, both from the frequency and the monetary factors. This proposed method will be very suitable in answering customer segmentation problems in online business transactions which are currently booming.
4. The proposed method
The proposed method is developed from the Recency, Frequency, Monetary (RFM) method by considering the time period factor when a business transaction is carried out by a customer. The advantage of the proposed method is that the company can consider the activity of each customer in each time period. In this proposed method, the division of time periods can be done flexibly according to the company’s business system. For example, the time periods can be divided into one week, one month, three months, one year, three years, or the whole year. The division of this time period into layers can sharpen the consideration of the active factor of each customer within a certain period of time. With this, the company can segment customers more precisely. The proposed method will also be very suitable for online transaction businesses where customers can be active or inactive at any time easily. A customer can actively conduct business transactions for a certain period of time, but then after that the customer does not make transactions for a relatively long time.
The proposed method also has flexibility in assigning weights to each layer of the time period. For example, the last one week will be given a greater weight than the last one month. This will provide flexibility for the company in determining which time period is more important for the company’s business system.
The proposed method also has the advantage of providing flexibility in assigning weights to recency, frequency, and monetary factors. Companies can consider which factors play a more important role than other factors. For example, in fast moving product companies such as fresh food products, the company will expect more frequent transactions (e.g., daily purchase transactions) even though the amount of money is not too large for each transaction. In this situation, it is possible that the frequency will be given a relatively more important weight than the monetary factor. This will be different for business companies that have products in large quantities (bulk), where companies will expect large purchases, while the frequency of purchases is relatively less important
With this proposed method, each company’s needs can be accommodated more flexibly so that each company can segment its customers in more detail and more accurately based on time period considerations. The formulation of the Multi Layer Recency, Frequency, and Monetary (MLRFM) method (Equationequation 1(1)
(1) ) that was initiated can be explained in the following.
: Customer number {1 … m}
: Category in a certain time range {1, …, n}
: The number of points in the recency category for customer i
: The number of points in the frequency category for customer i in j time period
: The number of points in the monetary category for customer i in j time period
: Weight of recency category on customer i
: Weight of frequency category on customer i in j time period
: Weight of monetary category on customer i in j time period
EquationEquation 1(1)
(1) is the score for a particular customer, obtained from the weighted combined score of the three variables above: recency, frequency, and monetary. The recency category is obtained from the last time the customer made a transaction. For example, customer A’s last transaction was made 3 days ago. Meanwhile, customer B’s last transaction was 50 days ago. So, customer A has a higher Recency score than customer B. The length of time the customer’s last transaction was converted into Recency score according to a predetermined value threshold. For example, a customer whose last transaction was less than the last 14 days would be assigned a value of 5 (active customer), while a customer whose last transaction was more than 60 days was assigned a value of 1 (inactive customer). Each point earned in this category is multiplied by the weight of the Recency category determined by the company.
In the assessment of the Frequency category, it is obtained from how much a customer doing transactions in a certain period of time. The same thing is done in the Monetary category, only the money used the service in a certain time category is calculated. The value of the weight of each monetary category at each time duration can be distinguished in order to obtain the division of the customer category the amount of fees that have been used in transactions. The division of layers in the mathematical model that was initiated can be used to obtain detailed segmentation of the level of activity of each customer based on a certain period of time.
Through this proposed model, it is hoped that the activity of each customer based on a certain period of time can be classified more accurately and reflecting the real importance of the customers. By doing this, the company can provide more appropriate priority services for both old and new customers.
4.1. Implementation and model testing
The customer dataset that is used for testing the proposed method is divided into two categories, namely old customers and new customers. Old customers are divided into three categories, namely loyal (L1), middle (L2), and non-active (L3). Likewise, new customers are divided into three categories, namely loyal (N1), middle (N2), and non-active (N3).
L1 represents old customers who have been using the service for a long time and are still actively transacting until now. L2 represents existing customers who have been using the service for a long time but have not been actively transacting in recent times. Meanwhile, L3 represents old customers who have been using the service for a long time and have not been actively transacting for some time. The cut-off time for old and new customers can be determined subjectively but this typically depends on the nature of business. In a fast moving goods or services, this could be three months, but in a slow moving products the cut of time could be one year or more.
N1 represents new customers who have recently used the service and are actively transacting. N2 represents new customers who have recently used the service but are not actively transacting. While N3 represents new customers who have just used the service but are not active (for example, only occasionally trying to make a transaction).
The proposed method can be divided into seven steps as shown in Figure . The corresponding details are explained as follows:
Step 1: Transaction data
A collection of transaction data from customers is generated randomly (as the example shown in Table ) represents data from 20 customers with a total of 4991 transactions. This random data is used to calculate the priority level of each customer based on the proposed method. This random data reflects old customers and new customers who make transactions via online transactions. There are old customers who have been using the service for a long time and are currently still actively transacting. There are also existing customers who have been using the service for a long time but have not been actively transacting recently.
Table 2. Sample of transaction data
Step 2: Determine the period of the Multi Layer Frequency Monetary
The calculation of the proposed method is done by looking at the customer transaction data. Recency is calculated based on the day of the last transaction made by customer. Meanwhile, specifically for Frequency and Monetary, we use a multi layer approach where they are calculated by determining layer boundaries based on a certain time period. Frequency is calculated based on the number of transactions that have been made within a certain period of time. Likewise, with Monetary which is determined by calculating the total costs incurred in transactions within a certain period of time. In this experiment, the time limits used are transactions in the last one week, last one month, last three months, and the entire time.
Step 3: Calculate MLRFM summarize value
Based on the multi layer division, the summary results are obtained from each existing customer (as shown in Table ). The division of this layer is expected to increase the segmentation of existing customers.
Step 4: Normalize MLRFM value
The existing data are then normalized to obtain a value of 0 (lowest value) to 1 (highest value). For Frequency and Monetary, high values are considered better. Especially for Recency, the opposite will apply because recency values with high values are actually considered worse. The equations for normalization of the RFM attributes are presented below:
Step 5: Determine segmentation value
In order to cluster each value, each recency, frequency, and monetary value is divided/segmented into few parts. In this experiment, five parts were used with details of the division as shown in Table . In this proposed method, the company can determine how much distribution is needed. The smaller the division, the more segmentation that will be carried out.
Table 3. MLRFM scoring threshold
Step 6: Determine weight
The next step is to assign an assessment weight (rated from 0 to 1, with a total weight value of 1) on each predetermined Multi Layer for Frequency and Monetary. For this experiment, the weights are determined by numbers as shown in Table . Each value of this weight applies to multiple layers that exist in frequency and monetary. The weights of the recency, frequency, and monetary factors are determined as in Table .
Table 4. Weight on multi layer
Table 5. Weight factor
Step 7: Calculate MLRFM scoring result
After each value of Recency, multi layer Frequency, and multi layer Monetary is obtained, then that value is entered into EquationEquation 1(1)
(1) , where each value will be multiplied by both the weight of each multi layer and the weight of each factor. Each Recency, Frequency, and Monetary factor will have its own scoring result. Then, the scoring is added so that the overall scoring results are obtained. The scoring results from this experiment can be seen in Table . Detailed examples of how to calculate the MLRFM score can be seen in Table .
Table 6. Transaction data summary
Table 7. MLRFM calculation example
4.2. Results
The results of the proposed method will be compared with the results of the RFM model values. The same transaction data (Table ) is processed using a normalization mechanism then the results are divided into five parts (Table ) but this time it does not use layer division or only takes the overall part (all) of the Recency, Frequency, and Monetary factors. Then, the results obtained are multiplied by the same weight (Table ). The final results from RFM method can be seen in Table .
It can be seen that the RFM results (Table ) of active old customers (L1) such as Customer ID 15, 4, and 1 are ranked in 1, 3, and 4, while new customers (N1) are in the lower order, for example, Customer ID 12 is ranked eighth. In actual fact (as shown in Table ) Customer ID 1 used the service (Recency) for the last 20 days, and only ordered (Frequency) one time during the last one month and only ordered 10 times in the last three months. However, because the total number of orders was 500 times, Customer ID 1 is assigned to fourth rank. Meanwhile, Customer ID 12, which is a new customer (N1) with service usage (Recency) in the last one day, ordered ten times in the last one week, 40 orders in the last one month, 130 orders in the last three months, and a total order of only 130 orders (looks like the customer just joined in the last three months) are placed in eighth rank. This is happened because the RFM method only considers the total number of orders.
This case also happened in monetary factor, where Customer ID 1 made a total transaction of 2 million IDR, while Customer ID 12 made a total transaction of 1.3 million IDR. So, the RFM method values Customer ID 1 as higher in rank than Customer ID 12, even though Customer ID 1 in the last three months is less active than Customer ID 12. Details of calculating the MLRFM score from Customer ID 1 and 12 can be seen in Table . From the data in Table , it can be seen that the last transaction from Customer ID 1 was 20 days ago. Meanwhile, the minimum value for the last transaction in the processed data was happening yesterday, and the maximum value for the transaction was happening 100 days ago. So, the result of normalization of recency factor for Customer ID 1 is 0.8. Meanwhile, the last transaction from Customer ID 12 was one day ago. This value is then processed using MLRFM threshold data (Table ). It can be seen that the value of 0.70–1.00 will have a value of five points.
From the data in Table , it can be seen that the number of transactions made by Customer ID 1 during the last week was zero. This is also the minimum value of the number of transactions on the processed data. The maximum value of the number of transactions during the last week is 14 times. So, the result of normalization of the frequency for Customer ID 1 is zero. Meanwhile, the number of transactions during the last week from Customer ID 12 was 10 times. So that when normalization is carried out the frequency factor value (one week) is 0.71. This value is processed using MLRFM threshold data (Table ). It can be seen that the value of 0.70–1.00 will have a value of 5.
After obtaining the results of each MLRFM value, the value is multiplied by a predetermined weight. For example, for the recency factor of Customer ID 1 and 12, a recency value of 5 is obtained multiplied by a recency weight of 0.2, so that a total recency value of 1 is obtained. Meanwhile for Customer ID 1, the one-week frequency value is one point multiplied by a weight of 0.2, plus the one-month frequency value of one point multiplied by a weight of 0.2, plus the three-month frequency value of one point multiplied by a weight of 0.3, plus the frequency value of all transactions of three points multiplied by a weight of 0.3. So that the results obtained for the total frequency value of 1.6. The same way is done for the value of the monetary factor. The results obtained from the recency, frequency, and monetary factor values are then added together to obtain the total MLRFM score. The results obtained from the recency, frequency, and monetary factors are then multiplied by the weight of each factor. The results are then summed to get the total MLRFM score. For Customer ID 1, the total recency value is one point multiplied by a weight of 0.2, plus the total frequency value of 1.6 multiplied by a weight of 0.4, plus the total monetary value of 1.6 multiplied by a weight of 0.4. So that the total MLRFM score for Customer ID 1 is 1.48. The MLRFM final result can be seen on .
Table 8. MLRFM results
In Table , the results of the RFM method are compared with the proposed method (MLRFM) with Frequency and Monetary factors divided into periods of one week, one month, three months, and total. Customer ID 1 is placed at rank 16 (down 12 ranks), while Customer ID 12 is placed at second rank (up six ranks). The changes in the RFM Level as compared to MLRFM Score in the brackets indicate that the rating has decreased by the numbers written, while numbers without brackets mean the rating has increased by the numbers written.
Table 9. RFM results
The proposed method is able to provide high ratings for existing customers who are really active both in the recent period and with high total of order frequency and value of transactions, such as Customer ID 15 at first rank (same as the results of the method RFM). The proposed method is also able to consider the active factor of the customer in placing orders in recent times. For example, Customer ID 9 (L2) ranked third and managed to beat Customer ID 4 (L1) ranked eighth. Although Customer ID 4 has a total number of orders (Frequency) of 500 and Monetary of 1.5 million IDR, while Customer ID 9 has a total frequency of 200 with Monetary of 3 million IDR. This is because Customer ID 9 ordered 50 times in the last one month and ordered 70 times in the last three months. Meanwhile, Customer ID 4 only ordered 5 times in the last one month and ordered 50 times in the last three months.
Furthermore, the advantages of the proposed method have the flexibility in terms of the use of the time period considered for special business purposes. The company can determine the division of time periods that are considered more appropriate for consideration in the company’s business, e.g., one month, two months, one year, three years, five years, etc. The company can easily determine the weights that are considered more influential in a certain period of time, for example, the weight of a period of one month is considered greater than the weight of a period of three months or vice versa.
In order to identify the cluster formed from the proposed method, the K-means clustering method is used. The results obtained are five clusters (as shown in Table ). It can be seen that clusters one and five only have one member. While clusters two, three, and four have seven, seven, and four members, respectively.
Table 10. RFM and MLRFM result comparison
To evaluate the resulting clusters, measurements of cohesion, separation, and silhouette coefficients were used. This measurement is used to identify how suitable an object belongs to a cluster. The Silhouette Coefficient value has a score range between −1 and 1. A score of 1 means clusters are well apart from each other and clearly distinguished, while a value of −1 means clusters are assigned incorrectly.
In order to calculate the dissimilarity between data, the Euclidean distance method is used. The Euclidian distance formula used is as follows:
: customer number {1 … m}
j: customer number {1 … n}
: Score in the recency category for customer i
: Score in the frequency category for customer i
: Score in the monetary category for customer i
As for the cohesion measure, the following calculation is used. For data i, the cohesion value will represent the average dissimilarity of data i to other data in the same cluster (Ci), with k number of data members.
To measure the average distance from data points in one cluster to data in other clusters, separation measurements are used. The separation for data i in cluster Ci corresponds to the average distance to all other data (total U data) in all other clusters. for the separation measure, the following calculation is used.
The Silhouette coefficient for the clustering solution is calculated using the following calculation:
From the calculation of cluster evaluation (as can be seen in ), the average Cohesion is 1,507, Separation is 4.135, and the Silhouette Coefficient is 0.61. So, it can be concluded that the resulting cluster results are quite good (relative close to the highest score of 1).
Table 11. K-Means clustering result
Table 12. Cluster evaluation
5. Conclusion
Customer segmentation in a business is very important. Companies in general must determine which customers have priority over the other. One of the methods used in the customer segmentation process is the RFM (Recency, Frequency, and Monetary) method. This method considers when was the last time the customer made a transaction/order (Recency), how many orders have been made (Frequency), and how much the customer has spent (Monetary). This method has a weakness in considering the Frequency and Monetary factors, where the consideration factors used are the total number of Frequency and the total number of Monetary only. The general weakness of this method is that the new customers cannot rank higher than old customers, which of course generally have a larger number of Frequency and Monetary scores. This could happen even if though the old customer has not been actively placing orders in recent times. For example, the customer has moved to another supplier.
The proposed method presented in this paper tries to consider the Frequency and Monetary factors carried out by the customer within a certain period of time. We called it Multi Layer Recency, Frequency, Monetary (MLRFM) approach. In the proposed method, we develop different range of time segments, called layers, and record customer activities within those different time segments. In each situation, different time segment may be defined. Different weight to each time segment is assigned. This different weight will reflect how much more important the newer time period is considered over the older ones. With this method, it is expected that the company can result in an accurate and fair priority decisions. In the online business where customers can switch buying products to new providers very easily, this new approach is expected to provide advantages in considering the dynamic of customer behavior.
Based on the comparison between the proposed method called MLRFM with it previous version, RFM, it is obvious that the proposed method can place new consumers who have high activity at a high priority and lower the priority of the existing customers who have been less active lately. To our understanding this provides more appropriate ratings to those customers that are potentially contributing high revenue in the current and future periods.
With the testing that have been carried out, it can be concluded that the proposed MLRFM method is able to provide more detailed priorities for existing customers, both old customers and new customers. Further research is needed in implementing and testing the proposed method and to prove whether the proposed method is able to provide appropriate customer priority ratings in a variety of online transactions, such as in health services, online transportation services, and so on. With the advent of information technology and data analytics this kind of segmentation can be done at a micro level, resulting in hundreds or even thousands of different segments. Future studies may also be directed toward financial evaluation of different segmentation approaches. While operationally these different segmentation approaches may result in different technical challenges, it is important to see how much each of these different approaches result in different financial performance.
Disclosure statement
No potential conflict of interest was reported by the author(s).
Additional information
Funding
References
- Apichottanakul, A., Goto, M., Piewthongngam, K., Pathumnakul, S., & Ramirez, F. J. (2021). Customer behaviour analysis based on buying-data sparsity for multi-category products in pork industry: A hybrid approach. Cogent Engineering, 8(1), 1865598. https://doi.org/10.1080/23311916.2020.1865598
- Bauer, C. L. (1988). A direct mail customer purchase model. Journal of Direct Marketing, 2(3), 16–19. https://doi.org/10.1002/dir.4000020305
- Birant, D. (2011). Data mining using RFM analysis. In Knowledge-oriented applications in data mining. IntechOpen.
- Bower, J. L., & Christensen, C. M. (1995). Disruptive technologies: Catching the wave. Harvard Business Review, 43–53. https://doi.org/10.1016/0737-6782(96)81091-5
- Changqiu, L. (2011). An efficient method to identify customer value in tourist hotel management. International Conference on Enterprise Information Systems, 165–169
- Chang, H. C., & Tsai, H. P. (2011). Group RFM analysis as a novel framework to discover better customer consumption behavior. Expert Systems with Applications, Elsevier, 38(12), 14499–14513. https://doi.org/10.1016/j.eswa.2011.05.034
- Chang, H. H., & Tsay, S. F. (2004). Integrating of SOM and K-mean in data mining clustering: An empirical study of CRM and profitability evaluation. Journal of Information Management, 11(4), 161–203 .
- Cheng, C. H., & Chen, Y. S. (2009). Classifying the segmentation of customer value via RFM model and RS theory. Expert Systems with Applications, 36(3), 4176–4184. https://doi.org/10.1016/j.eswa.2008.04.003
- Chen, Y. L., Kuo, M. H., Wu, S. Y., & Tang, K. (2009). Discovering recency, frequency, and monetary (RFM) sequential patterns from customers’ purchasing data. Electronic Commerce Research and Applications, 8(5), 241–251. https://doi.org/10.1016/j.elerap.2009.03.002
- Chen, D., Sain, S. L., & Guo, K. (2012). Data mining for the online retail industry: A case study of RFM model-based customer segmentation using data mining. Journal of Database Marketing & Customer Strategy Management, 19(3), 197–208. https://doi.org/10.1057/dbm.2012.17
- Christy, A. J., Umamakeswari, A., Priyatharsini, L., & Neyaa, A. (2021). RFM ranking–An effective approach to customer segmentation. Journal of King Saud University-Computer and Information Sciences, 33(10), 1251–1257. https://doi.org/10.1016/j.jksuci.2018.09.004
- Firdaus, U., & Utama, D. (2021). Development of bank’s customer segmentation model based on RFM+B approach. Int. J. Innov. Comput. Inf. Cont, 12, 17–26. https://doi.org/10.24507/icicelb.12.01.17
- Haiying, M., & Yu, G. (2010, May). Customer segmentation study of college students based on the RFM. In 2010 International Conference on E-Business and E-Government (pp. 3860–3863). IEEE. https://doi.org/10.1109/ICEE.2010.968.
- Hughes, A. M. (1996). Boosting response with RFM. Marketing Tools, 5(1) , 4–8.
- International Finance Corporation. (2019). Reinventing business through disruptive technologies, sector trends and investment opportunities for firms in emerging markets (pp. 19). World Bank Group.
- Kahan, R. (1998). Using database marketing techniques to enhance your one‐to‐one marketing initiatives. Journal of Consumer Marketing, 15(5), 491–493. https://doi.org/10.1108/07363769810235965
- Kaymak, U. (2001). Fuzzy target selection using RFM variables. In Proceedings Joint 9th IFSA World Congress and 20th NAFIPS International Conference (Cat. No. 01TH8569) (Vol. 2, pp. 1038–1043). IEEE.
- Marcus, C. (1998). A practical yet meaningful approach to customer segmentation. Journal of Consumer Marketing, 15(5), 494–504. https://doi.org/10.1108/07363769810235974
- Mohammadzadeh, M., Hoseini, Z. Z., & Derafshi, H. (2017). A data mining approach for modeling churn behavior via RFM model in specialized clinics case study: A public sector hospital in Tehran. Procedia Computer Science, 120, 23–30. https://doi.org/10.1016/j.procs.2017.11.206
- Newell, F. (1997). The new rules of marketing: How to use one-to-one relationship marketing to be the leader in your industry. Irwin Professional Publishing.
- Nikumanesh, E., & Albadvi, A. (2014). Customer’s life–time value using the RFM model in the banking industry: A case study. International Journal of Electronic Customer Relationship Management, 8(1), 15–30. 2. https://doi.org/10.1504/IJECRM.2014.066876.
- Peker, S., Kocyigit, A., & Eren, P. E. (2017). LRFMP model for customer segmentation in the grocery retail industry: A case study. Marketing Intelligence & Planning, 35(4), 544–559. https://doi.org/10.1108/MIP-11-2016-0210
- World Bank. (2019). World development report 2019: The changing nature of work. https://doi.org/10.1596/978-1-4648-1328-3
- Yeh, I. C., Yang, K. J., & Ting, T. M. (2009). Knowledge discovery on RFM model using Bernoulli sequence. Expert Systems with Applications, 36(3), 5866–5871. https://doi.org/10.1016/j.eswa.2008.07.018