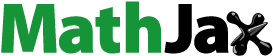
Abstract
Consumption of water contaminated with heavy metals has serious health consequences and these include hypertension, kidney disease, cancer, anaemia, brain damage, etc. However, groundwater pollution with heavy metals may occur naturally or anthropogenically as a result of water-rock interaction, dumpsite leachate, agricultural activities, or sewage percolation. Therefore, the apportionment of the specific sources of heavy metals to groundwater is vital to prevent and control its pollution to groundwater. The present work assessed thirty-four (34) groundwater samples from the vicinity of the Granvillebrook and Kingtom dumpsites, Freetown, Sierra Leone. The objective of the research was achieved by integrating geospatial, indexical, and multivariate statistical methods. The results showed that heavy metals such as Cu, Cr, Fe, and Mn exceeded the permissible levels in the majority of the groundwaters. As and Pb were below the detection level of the instrument in all the groundwaters analyzed. Seasonal variations revealed that the concentration of heavy metals is relatively higher in the dry season due to the effect of dilution. The heavy metal evaluation index and degree of contamination analyses respectively showed that 16.7 and 41.6% of the groundwaters in the Granvillebrook dumpsite are highly polluted by heavy metals. All the groundwater samples in the Kingtom dumpsites except well KW17 are unaffected by heavy metals. The principal component analysis showed that fuel combustion, brake abrasion, and discharge of municipal and industrial waste from the dumpsites are some of the anthropogenic sources that impact groundwater pollution in the study areas. The findings of this research revealed the urgent need to develop strategies to control and mitigate the occurrences of heavy metals and thus serve as a base for future research in the study areas.
1. Introduction
The contamination of groundwater with trace metals may occur naturally or as a result of industrial influence (Buragohain et al., Citation2010). Wastes from industries are the crucial causes of environmental degradation (Akbulut & Tuncer, Citation2011). Heavy metals such as chromium (Cr), lead (Pb), copper (Cu), and nickel (Ni) are used in several industrial activities. Ni is used in batteries and electroplating of metal while Tetraethyl Pb is used as an antiknock agent in petrol, and Cr is used as a pigment in paint, tanning, and steel production (Rahman et al., Citation2020). Rechargeable and household batteries, light bulbs, used motor oils, plastics, photographs, lead pipes, etc. contain heavy metals (Somani et al., Citation2019). Eventually, these metals find their way into open dumpsites and infiltrate into nearby groundwaters leading to heavy metal pollution. In previous studies conducted in both the Kingtom and Granvillebrook dumpsites, Hingston et al. (Citation2002) reported higher levels of cobalt, nickel, and zinc in soils and water at the Granvillebrook and Kingtom dumpsites. Frazer-Williams (Citation2017) also reported high concentrations of Zn, Cu, and Pb in soil samples of the Granvillebrook dumpsite. A study conducted by Egbueri (Citation2018) in Nigeria measured high concentrations of heavy metals in groundwaters closest to the Akwa and Nnewi dumpsites.
Heavy metals have serious health consequences; Pb can cause hypertension, kidney disease, anaemia, brain damage, etc. (Aboyeji & Eigbokhan, Citation2016; Raju et al., Citation2015). Das et al. (Citation2012) reported some of the adverse health effects caused by Arsenic (As). These involve cardiovascular disease, liver disease, cancer, diabetes mellitus, and skin lesions. Copper, chromium and iron can cause vomiting, nausea, diarrhea, stomach cramps, liver, and kidney diseases while nickel causes lung damage and dermatitis (Egbueri, Citation2020; Haloi & Sarma, Citation2012). High manganese (Mn) concentrations cause children’s organoleptic problems and behavioral deficits (Homoncik et al., Citation2010; Rivera-Rodríguez et al., Citation2019). Excessive intake of cadmium (Cd) and zinc (Zn) in the body can cause nausea, anemia, vomiting; diarrhea, convulsions, and low blood pressure (Raju et al., Citation2012).
The foregoing health consequences associated with high heavy metal concentrations in groundwater near dumpsites influence the appraisal of groundwater quality within the Granvillebrook and Kingtom environs. Several methods such as artificial intelligence (Alizamir & Sobhanardakani, Citation2016; Lu et al., Citation2019), numerical modeling (He et al., Citation2019; Huang et al., Citation2007), geospatial (Paolalsiam et al., Citation2021; Rizwan et al., Citation2021; Venkatesan et al., Citation2020), indexical (Egbueri & Unigwe, Citation2019; Ukah et al., Citation2019), and multivariate techniques (Baghanam et al., Citation2020; Facchinelli et al., Citation2001), etc. have been used to study the migration and contamination of heavy metals in water bodies. Artificial intelligence is often employed to predict the distribution of heavy metals (Bayatzadeh Fard et al., Citation2017; Bhagat et al., Citation2021) and to model its adsorption in water bodies (Bhagat et al., Citation2020; Hafsa et al., Citation2020) while the numerical method is used to simulate the migration of heavy metal pollutants in sediments (Ng et al., Citation2009) and water bodies (Huang et al., Citation2007). The use of the geospatial method in groundwater quality study is vital to generate spatially continuous data which will predict the heavy metal concentrations for unsampled sites. As contained in Singh et al. (Citation2013), groundwater chemistry is characterized by complex interrelationships among a range of variables. Therefore, the use of multivariate statistical analysis could be ideal to probe the possible pollution sources of heavy metals and other dissolved ions. Principal component analysis (PCA) is one of the major examples of multivariate statistical methods that have been globally used to study groundwater quality (Rezaei et al., Citation2019a). The effective assessment and monitoring of water quality requires knowledge of water quality indices (Mgbenu & Egbueri, Citation2019). The widely used indexical methods in groundwater studies are heavy metal pollution indices (HPIs). Several heavy metal pollution indices have been proffered to evaluate the extent of heavy metal contamination (Chaturvedi et al., Citation2019; Duodu et al., Citation2016; Elumalai et al., Citation2017; Zhuang et al., Citation2018). However, the degree of contamination (Cd), heavy metal evaluation index (HEI), and heavy metal pollution Index (HPI) have been more frequently used to study heavy metal pollution.
Characterizing the effect of seasonal changes in groundwater quality is critical to evaluating the factors (natural or anthropogenic) influencing groundwater pollution (Ouyang et al., Citation2006; Sappa et al., Citation2015). Seasonal variation affects the quality of water and its treatment efficiency (Etchie et al., Citation2013). Pollutants entering a groundwater system may result from seasonal-dependent pathways such as a rainstorm, leachate percolation, water runoff, and atmospheric deposition. Therefore, seasonal changes in groundwater quality must be considered when assessing the sources and extent of heavy metals contamination.
Globally, to the best of the authors’ knowledge, few or no studies have combined spatial, indexical and seasonal distribution of heavy metals in groundwaters near dumpsites. In addition, no geospatial and indexical study has been done on groundwater within the dumpsites of Sierra Leone. Therefore, the objectives of this study focused on integrating geospatial, indexical (HEI and Cd), and multivariate statistical analysis to (1) assess the seasonal variation of heavy metals (2) evaluate the heavy metal pollution indexes, and (3) identify the probable sources of heavy metals in the groundwaters of the Granvillebrook and Kingtom dumpsites. This information is vital for managers and scientists to predict future trends and potential environmental crises (Shahid et al., Citation2014).
2. Study area
The Granvillebrook and Kingtom dumpsites lie between latitudes 7°N and 10°N and longitudes 10°W and 13.5°W respectively. The Kingtom dumpsite is located in the western part of Freetown while the Granvillebrook is situated in the east. The Kingtom and Granvillebrook dumpsites have an area of 14.6 and 15.0 acres respectively (Sankoh et al., Citation2022a). The Kingtom dumpsite is bordered to the north by the Kingtom cemetery and to the east by Kroo Bay. The climate of the study areas is hot, humid and comprises two distinct seasons. The wet season starts in May and ends in December while the dry season starts in January and elapses in April. Figure shows the location map of the study areas.
2.1. Hydrogeology of the study area
The environments under study are characterized by a large extension of Mesozoic fractured ultrabasic igneous rocks. The igneous rocks belong to various formations and consist of fractured gabbros (secondary porosity) and are referred to as the Freetown Layered Complex and other intrusive. Figure shows the hydrogeological map of the study areas. The exposed part of the Freetown layered intrusion is crescent-shaped and consists of a 6 km thick, westward dipping sequence of gabbroic rock (Bowles et al., Citation2000; Chalokwu, Citation2001). The layering is curved and the dips increase from around 20° in the east to 40° to 50° in the west. Geophysical evidence indicates that the intrusion extends farther to the west under the Atlantic Ocean and that it is approximately circular. Five zones (zones 1–4 and an unexposed lower zone) have been identified; each showing an upward progression from troctolite through olivine, gabbro and leucogabbro to anorthosite (Bowles et al., Citation2000; Chalokwu, Citation2001). The Freetown layered complex comprises plagioclase , magnetite
, Imenite ((
, and olivine (mixed crystals of
and
) rocks (Umeji, Citation1983).
Figure 2. Simplified geological map of the Freetown Layered Complex (Cabri et al., Citation2022).

3. Materials and methods
3.1. Water sampling and analysis
Forty (40) groundwater samples consisting of boreholes, hand-dug wells, and leachates were sampled within the Granvillebrook and Kingtom dumpsites during the dry and wet seasons. Before sampling, the boreholes and hand-dug wells were purged for five minutes to eliminate stagnated waters. Groundwater samples for the trace metals analysis were collected in preconditioned high-density polyethylene (HDPE) bottles. The groundwater and leachate samples were pre-filtered through 0.45-micron cellulose filters and stored at 4°C. The trace metals samples were acidified with two (2) drops of concentrated 65% to prevent metal adsorption to the walls of the containers and to keep the ions in the solution. The geographic locations of the sampling points were determined using a hand-held Global Positioning System (GPS) (Garmin eTrex 20, U.S.A.). The electrical conductivity (EC), pH, and total dissolved solids (TDS) were measured during sample collection using WTW (3630 IDS) multi-parameter. The trace elements were analyzed using VARIAN AA240FS-fast sequential Atomic Absorption Spectrophotometer (AAS) at the Ministry of Water Resources, Sierra Leone. The accuracy of the analyses was within the range of ±5% for all samples.
3.2. Statistical analysis
The boxplots, correlation coefficients, and principal component analysis were the three major statistical analyses carried out in this study. The R-Statistical software was used to generate the boxplots and Pearson’s correlation model. Pearson’s correlation was used to quantitatively evaluate the interrelationships between various heavy metals and some physical indicators. The principal component analysis (PCA) is a quantitative and independent approach that establishes correlations between heavy metals in normally distributed datasets (Cerar & Mali, Citation2016). It significantly helps to identify the major factors impacting groundwater chemistry. The PCA was performed using Statistical Package for the Social Sciences (SPSS.22) software. The data were log-normal before analysis as elucidated in Yidana et al. (Citation2010) to ensure that all the parameters have equal or approximate variances. The Varimax rotation was employed to increase the disparity between variables under each factor (Kumar & Krishna, Citation2021). The Kaiser criterion was applied in this study to reduce the number of insignificant factors. Here, only factors with Eigenvalues greater than one were retained for analysis as the components varied significantly from one another (Yousry, Citation2011). PCA works best with larger datasets and this was ascertained by the Kaiser–Meyer–Olkin (KMO) values. In the current, only PCA results with a KMO value greater than 0.5 were analyzed.
3.3. Geospatial analysis
The heavy metal and pollution index distribution maps for both the dry and wet seasons were generated in Quantum Geographic information syste (QGIS 3.24.2) using the inverse distance weighted (IDW) method. Spatial interpolation uses points with known values to determine values at unknown points. The IDW has proven to be versatile, convenient and relatively accurate (Kumar & Krishna, Citation2021). A portable Garmin geographical positioning system (GPS) device was employed to determine the latitude and longitude of the sampling points. The GPS coordinates of the points were converted to vector data using the “add vector layer” icon to create a shape file of the study areas. The combined GPS coordinates and water quality data were introduced into the GIS environment to prepare the thematic layer maps (Raikar & Sneha, Citation2012). Buffer zones with 20 m intervals were employed in this study to assess the relationship between the sampling points and the concentration of heavy metals. The IDW interpolation method was validated using a technique referred to as ‘cross validation’. The Root Mean Square Error (RMSE) method was used to compare the observed and predicted values (Sankoh et al., Citation2022a, Citation2022b).
Where:
n = sample size, = measured value at the jth location and
= interpolated value at the jth location
3.4. Calculation of heavy metal pollution indices
The heavy metal evaluation index (HEI) and Degree of contamination (Cd) were used in this study to evaluate the extent of heavy metal contamination of groundwaters. HEI is the proportional concentration of heavy metals in the groundwater of a specific study area relative to the maximum acceptable levels in drinking water (Mokarram et al., Citation2020).
HEIi =Heavy metal evaluation index of the ith metal
MACi =Maximum allowable concentration of the ith metal m
The degree of contamination (Cd) suggested by Hakanson (Citation1980) is defined as the collective detrimental impact of heavy metals on groundwater (Karaouzas et al., Citation2021).
Where
= Contamination factor
Mi =Measured concentration of the ith metal
Si =Maximum permissible concentration of the ith
Both the HEI and Cd classifications are shown in Table while Table shows the standard values used for the computation of the heavy metal pollution indices.
Table 1. Classifications of HEI and Cd
Table 2. Permissible values of heavy metals
Figure shows the flow chart of the overall methodological approach applied in the study.
4. Results and discussion
4.1. Seasonal geospatial distribution of physical parameters and heavy metals
Table shows the descriptive statistical results of the physical and heavy metal characteristics in the groundwaters of the study areas. The pH ranged from 5.0 to 8.4 in the groundwaters of the Granvillebrook dumpsite indicating the acidic to alkaline characteristics of the groundwaters. On the other hand, the pH of the groundwater in the Kingtom dumpsite ranged from 4.4 to 6.20 with a mean of 5.02 suggesting their acidic characteristics. The EC ranged from 363 to 2480 µS/cm and 126.0 to 462 µS/cm in the groundwaters of the Granvillebrook and Kingtom dumpsites respectively. This suggests that the groundwaters at the Granvillebrook dumpsite have been significantly polluted relative to those in the Kingtom dumpsite. The TDS follows similar trend to the EC and thus ranged from 190 to 1410 ppm and 51.8 to 270 ppm in the groundwaters of the Granvillebrook and Kingtom dumpsites respectively. Razali et al. (Citation2020) reported similar trends of these physical properties in water bodies. The greatest standard deviations were observed for Cu (0.74) and Fe (0.05) indicating significant variation of Cu and Fe in the Granvillebrook and Kingtom dumpsites respectively.
Table 3. Descriptive statistics of heavy metals in the Granvillebrook and Kingtom dumpsites
Tables and show the seasonal concentrations of heavy metals in the Granvillebrook and Kingtom dumpsites respectively. The heavy metal concentration was generally low in the groundwaters analyzed. As and Pb were below the detection levels of the instrument in all samples. Seasonal variations showed that the levels of all the heavy metals (except Zn) are relatively higher in the dry season. The lower concentrations of heavy metals during the wet season may be due to the dilution of contaminants by rain as well as surface water runoff which aids their migration (Sharma et al., Citation2021).
Table 4a. Seasonal concentration of heavy metals in groundwaters of the Granvillebrook dumpsite
Table 4b. Seasonal concentration of heavy metals in groundwaters of the Kingtom dumpsite
Figure shows the Box and Whisker plots of heavy metals (mg/L) in the groundwaters of the Granvillebrook and Kingtom dumpsites. Of all the heavy metals, Cu exhibited the highest concentrations (2.4 mg/L) in the study areas and thus ranged between 0.02 to 2.4 mg/L
Figure 4. Box and Whisker plots of heavy metals in the groundwater of the Granvillebrook and Kingtom dumpsites.

Figure shows the spatial distribution of Cu in the study areas. Four samples (GW11G, GW11J, DW01, and DW04) exceeded the national permissible limit of Cu (1.3 mg/). All the groundwaters in the Kingtom dumpsites are located upstream and thus exhibited Cu concentration below the maximum permissible limit. This confirms that the high Cu levels in the groundwaters of the Granvillebrook dumpsite are associated with anthropogenic rather than geogenic effects. The Cr values in the Granvillebrook and Kingtom dumpsites ranged from 0.0 to 0.08 mg/L and 0.02 to 0.06 mg/L respectively. The geospatial distributions of Cr in the study areas are shown in Figure . There were no consistent increasing trends in Cr relative to the dumpsites’ proximity indicating that the dumpsites could not have influenced the high Cr in the study areas. 60% of the groundwaters in the Kingtom dumpsite measured a concentration greater than the national standard i.e. 0.05 mg/L while 50% of groundwaters in the Granvillebrook dumpsite exceeded the Cr permissible level. The highest value of Cr (0.08 mg/L) was measured during the dry season in well GW31H. Massive exposure to hexavalent Cr causes acute tubular necrosis (ATN) and triggers adverse effects on the kidney (Wedeen & Qiant, Citation1991). Zinc (Zn) supports the human immune system and thus protects us from infections, and diseases. The Zn concentration ranged from 0.0 to 0.92 mg/L and 0.0 to 0.2 mg/L in the groundwaters of the Granvillebrook and Kingtom dumpsites respectively. All the groundwaters within the study areas measured low Zn concentrations below the WHO permissible limit. Seasonal variation confirms that the Zn concentration increased during the wet season. This may be due to the infiltration of rainwater from household zinc roofs into the groundwaters of the study areas. This finding is congruent with the study of Cecelia et al. (Citation2021), who reported high Zn concentrations during the wet season in the Caldwell dumpsite in Liberia. A study conducted by Naminata et al. (Citation2018) in Abidjan, Ivory Coast also reported a high concentration of Zn during the wet season. Fe concentration varied from 0.0 to 1.29 mg/L and 0.0 to 0.14 mg/L in the Granvillebrook and Kingtom dumpsites respectively. The geospatial distributions of Fe in the groundwaters of the study areas are shown in Figure . Only four wells (GW11J, DW01, DW02, and DW04) in the Granvillebrook dumpsite have Fe concentration above the WHO acceptable level (0.3 mg/L). The concentration of Fe above the acceptable limit in water may result in aesthetic problems (Adeolu et al., Citation2011) and as well influence liver damage, heart failure, diabetes, heart, and poor growth (Haloi & Sarma, Citation2012). The Fe levels in all the groundwaters of the Kingtom dumpsite are below the WHO permissible level. Relatively, the levels of Fe in this study were lower than the
concentrations measured in groundwater closest to dumpsites in Ghana (Nyame et al., Citation2012). The high levels of Fe in Groundwaters closest and farther away to the Granvillebrook dumpsite confirm the influence of both geogenic and anthropogenic pollution. The Granvillebrook dumpsite is dominated by the magnetite
, Imenite ((
and olivine (mixed crystals of
and
) rocks (Umeji, Citation1983); this could have induced the high Fe levels in well GW11J. As contained in Cumar and Nagaraja (Citation2011), the high Fe concentration in groundwaters near the Granvillebrook dumpsite may be due to the disposal of industrial iron scraps. Relative to other dumpsites elsewhere, the low concentrations of Fe in the Granvillebrook and Kingtom dumpsites are expected as most of the iron scraps deposited in the areas have been collected by scavengers. This is marked by the low mean Fe level (0.89 mg/L) in the leachates of the dumpsites. Mn concentration ranged from 0.0 to 0.90 mg/L and 0.0 to 0.13 mg/L in the Granvillebrook and Kingtom dumpsites respectively. Only two wells (GW11J and DW04) exceeded the national acceptable level of Mn (0.4 mg/L). However, exposure to Mn in water below the acceptable guideline could still cause adverse health effects with children being the most vulnerable (Bouchard et al., Citation2007). The high concentrations of Mn in the groundwaters of wells GW11J and DW04 are due to the geological formation of the study areas. Microbial activity influences both Mn and Fe concentration (Homoncik et al., Citation2010). That is why both Mn and Fe often occur concurrently in groundwater, with Fe being present at higher concentrations than Mn (Homoncik et al., Citation2010). This observation is congruent with our current study where the Fe concentrations exceeded that of Mn. Nickel (Ni) is an essential micro-nutrient for plant growth. Ni concentration ranged from 0.08 to 0.28 mg/L and 0.0 to 0.16 mg/L in the Granvillebrook and Kingtom dumpsites respectively. All the wells in the Granvillebrook dumpsite exceeded the national permissible limit of Ni (0.07 mg/L); this may lead to a range of adverse health effects such as dermatitis, lung cancer, inflammation, and irritation of the respiratory tract to persons consuming the water sources (Haloi & Sarma, Citation2012). 60% of the groundwaters in the Kingtom dumpsite exceeded the Ni permissible levels. The Ni concentration obtained in this study is higher than that observed by Abd El-Salam and Abu-Zuid (Citation2015) whose Ni values ranged fbetween 0.01 to 0.152 mg/L within the Borg El-Arab landfill site, in Egypt. Figure shows the spatial distribution of Ni in the groundwaters of the study areas. From Fig. , there was no consistent increase in Ni levels relative to the nearness of the dumpsites nor a significant difference (p-value > 0.05) of Ni between the dry and wet seasons. This suggests that the occurrence of Ni is as a result of natural influence. Umeji (Citation1983) reported high concentrations of Ni in the rocks of the Freetown Layered complex.
Figures show the concentration trends of heavy metals pollution for the dry and wet seasons respectively. Overall, the pollution levels of heavy metals in the study areas decreased in the order of Cu>Fe>Mn>Zn>Ni>Cr>Pb>As and Cu>Fe>Zn>Mn>Ni>Cr>Pb>As for the dry and wet seasons respectively. In summary, the results of the heavy metal analysis indicate that the water quality of the study areas is vulnerable to heavy metal pollution. The mean concentration of the heavy metals in the dry season was higher than in the wet season. This demonstrated the effect of seasonal variation on heavy metals in groundwater.
4.2. Spatial distribution of heavy metal pollution indices
The heavy metal pollution indices are often used to evaluate the overall water quality with regard to heavy metals. There have been several indices used in ecological studies, but the heavy metal evaluation index (HEI) and degree of contamination (Cd) were employed in this study to determine the extent of heavy metal contamination in groundwaters. The results of the heavy metal evaluation index (HEI) and Degree of contamination (Cd) for the Granvillebrook dumpsite and Kingtom dumpsites are presented in Tables and respectively. The HEI in the Granvillebrook dumpsite ranged from 5.2 to 26.8 with a mean of 12.8 and 4.2 to 19.5 with a mean of 11.2 for the dry and wet seasons respectively. HEI is classified as low, moderate, and highly polluted with corresponding values <10, 10–20 and >20 respectively. According to the classification, 41.7%, 41.6%, and 16.7% of groundwaters fell within the range of low, moderate, and highly polluted in the Granvillebrook dumpsite for the dry season while 41.6% and 58.3% fell in the range of low and moderately polluted for the wet season. The HEI values in the Kingtom dumpsite were lower than the Granvillebrook dumpsite and thus ranged from 1.3 to 9.5 with a mean of 5.2 and 1.1 to 11.8 with a mean of 6.4 for the dry and wet seasons respectively. All the groundwaters in the Kingtom dumpsite fell within the range of low pollution for the dry season while well KW17 demonstrated moderate pollution in the wet season. The Degree of Contamination (Cd) in the Granvillebrook dumpsite ranged from -2.8 to 27.3 with a mean of 6.0 and from -3.8 to 21.2 with a mean of 4.4 for the dry and wet seasons respectively. Cd may be classified into three categories as follows: low (Cd<1), medium (Cd = 1–3), and high (Cd>3). For the Granvillebrook dumpsite, 33.3%, 16.7%, and 50.0% of the groundwaters respectively fell within the range of low, medium, and highly polluted in the dry season while 41.6%, 16.7%, and 41.6% fell in the range of low, medium and highly polluted groundwater in the wet season. Also, Cd in the Kingtom dumpsite ranged from -6.7 to 1.3 with a mean of -3.2 and -6.9 to 3.7 with a mean of -1.5 for the dry and wet seasons respectively. During the dry season, 80% and 20% of the groundwaters fell within the range of low and moderately polluted while 60% and 40% fell within the range of low and moderately polluted in the wet season. The spatial distribution of HEI in the Granvillebrook and Kingtom dumpsites are shown in Figures respectively. The highest value of HEI (26.8) was measured in a well (DW04) located immediately downgradient to the Granvillebrook dumpsite. This demonstrated the strong influence of the leachates in the Granvillebrook area. For the Kingtom dumpsite, the highest value of HEI (11.8) was measured in well KW2G. The spatial distribution of the degree of contamination (Cd) in the Granvillebrook and Kingtom dumpsites are shown in Figures respectively. The highest value of Cd (27.3) was measured in well DW04 during the dry season at the Granvillebrook dumpsite. For the Kingtom dumpsite, the highest value of Cd (3.7) was measured in well KW17. Generally, seasonal variation showed increased concentration of HEI and Cd for the dry season at the Granvillebrook dumpsite due to the pollutant dilution effect (Tiwari et al., Citation2016). This observation was contrary to the Kingtom dumpsite.
Table 5a. Computed pollution Indexes (HEI and Cd) for the Granvillebrook dumpsite
Table 5b. Computed pollution Indexes (HEI and Cd) for the Kingtom dumpsite
4.3. Correlational analysis
The interrelationships of the heavy metals in the groundwaters of the Granvillebrook and Kingtom dumpsites were studied using Pearson’s correlation matrixes. Generally, significant positive correlations between pairs of heavy metals suggest that the heavy metals are from the same origin, whereas weak or negative correlations indicate that they are of distinct origin (Egbueri, Citation2018; Rezaei et al., Citation2019b). Figures show the Pearson’s correlation matrix results of the study areas. The Pearson’s correlation results of the Granvillebrook dumpsite revealed that Cu is statistically correlated with Fe (0.60), Mn (0.60), and Ni (0.64); this indicates multiple anthropogenic and geogenic source apportionments for these heavy metals. Cr did not seem to correlate with any of the heavy metals suggesting a geogenic source of the Cr. The high positive correlation between Fe and Mn (0.84) in the groundwaters of the Granvillebrook dumpsite indicates a reducing environment confirming the influence of the dumpsite leachate. On the other hand, Pearson’s correlation result of the Kingtom dumpsite established that Fe and Mn (0.056) are weakly correlated indicating an oxic environment and thus suggesting a minimal impact on the dumpsite. Also in the Kingtom dumpsite, Cu is significantly correlated with Zn (0.76); Fe is correlated with Ni (0.61) and Mn is correlated with Ni (0.54). These various heavy metal correlations demonstrated that the groundwaters within the study areas have various pollution sources spanning from water-rock interactions (geogenic), car combustion engines, brake abrasion, dumpsites leachate, and municipal sewage runoffs.
4.4. Multivariate statistical analysis
The principal component analysis (PCA) was used to decipher the potential sources affecting the groundwater quality in the Granvillebrook and Kingtom dumpsites. The Varimax rotation and the factor loadings and the PCA results of the heavy metals are shown in Table . Factor loadings ≥ 0.75, 0.50–0.75, and 0.30–0.50 were considered high, moderate, and weak respectively (Rahman et al., Citation2020). Figures show the Scree plots of the Granvillebrook and Kingtom dumpsites respectively. The principal component analysis results demonstrated four (4) component factors in the Granvillebrook dumpsite (Figure ). The first two factors loading of the Granvillebrook and Kingtom dumpsites accounted for 55.6% and 67.4% and are spatially distributed in space as shown in Figures respectively. The first factor (F1) of the Granvillebrook dumpsite accounted for 29.6 % of the total variance and has high positive loading for pH (0.76), (0.75); moderate loading with Cu (0.62), Zn (0.69), and Ni (0.51). The heavy metals in the F1 components are derived from either car combustion engines, brake abrasion, or dumpsite leachate. This is because Cu, Zn, and Ni are markers for diesel and lubricant oil combustion, tire, and brake abrasion (Duodu et al., Citation2016; Hulskotte et al., Citation2007; Shrivastava, Citation2009). This is obvious as well DW01, which is closer to the street measured a significant concentration of Cu and Ni above the permissible limit. The moderate Zn concentration may have been aroused from motor tire wear (Fatoki & Ayodele, Citation1991). The presence of Cu in wells downgradient to the Granvillebrook dumpsite might be due to the discharge of municipal and industrial waste containing Cu. The second factor (F2) accounted for 26.0 % of the total variance with moderate positive loading for Mn (0.70), Cu (0.52), Fe (0.53), and Ni (0.51). The Ni concentration could have been induced by either geogenic (water-rock interaction) or anthropogenic factors such as electrical appliances, machinery, and household utensils deposited in the dumpsite (Barceloux & Barceloux, Citation1999). The high Fe concentration in the groundwater could be due to geogenic (magnetite and iImenite) or anthropogenic influence i.e. the disposal of industrial scraps in the dumpsite. F3 accounted for 18.7% of the total variance with high positive loading for Cr (0.67) suggesting a geogenic source of the Cr. F4 accounted for 9.7 % of the total variance and has weak positive loading on Zn (0.48). The minute presence of Zn in the study area indicates minimal Zn pollution. PCA results depict three (3) components in the Kingtom dumpsite (Figure ). The first factor (F1) accounted for 43.0% of the total variance and 4.7 of the eigenvalue. F1 has high positive loading for EC (0.92), TDS (0.94), Fe (0.85) and moderate positive loading for As (0.57). The positive loading of EC suggests anthropogenic sources of the Fe which might be due to the leaching of iron scraps into the groundwaters. F2 accounted for 24.4% of the total variance and 2.7 of the eigenvalue; it has high positive loading for pH (0.86) and moderate loading for Cr (0.74) and Mn (0.63). This indicates waste from petroleum and agricultural practices. F3 accounted for 13.3% of the total variance and 1.5 of the eigenvalue; it has moderate positive loading with Cu (0.60) and Zn (0.68).
Figure 11. spatially distributed variables in rotated space of the groundwater in the (a) Granvillebrook and (b) Kingtom dumpsites defined by F1 vs F2 of the factor loadings.

Table 6. Varimax rotated factor loadings of heavy metals in groundwater of the study areas
5. Conclusion
No geospatial and indexical study has been done on groundwater within the dumpsites of Sierra Leone. Therefore, the groundwaters within the Granvillebrook and Kingtom dumpsites have been studied to determine the extent of heavy metal contamination. The analytical results from the two dumpsites were compared and thus revealed that the Granvillebrook dumpsite has been severely impacted by heavy metals. The majority of the heavy metals including Cu, Cr, Fe, and Mn exceeded the permissible levels in the groundwaters of the study areas. As and Pb were below the detection level of the instrument in all the groundwater samples. Seasonal variations revealed that the concentration of heavy metals is relatively higher in the dry season. The HEI and Cd results respectively showed that 16.7 and 41.6% of the groundwaters in the Granvillebrook dumpsite are highly polluted by heavy metals. All the groundwaters in the Kingtom dumpsites were unaffected by the heavy metals except for well KW17 which was moderately polluted. On a whole, the water quality analysis confirms that groundwaters in the vicinity of Granvillebrook are more polluted than those in the Kingtom. The principal component analysis (PCA) showed that fuel combustion, brake abrasion, and discharge of municipal and industrial waste from electrical appliances are some of the anthropogenic sources that impact groundwater in the study areas. The PCA also established that the prevalence of Ni and Cr in the groundwaters of the study areas was a consequence of geogenic influence.
The findings of this research revealed the urgent need to develop strategies to control and mitigate the occurrences of heavy metals in the study areas and also serve as significant information for managers and scientists to predict future trends and potential environmental crises. It is also advised that residents within the Granvillebrook dumpsites treat these waters before consumption.
Future research must focus on using stable isotope techniques to probe in-depth the exact sources that may have influenced the prevalence of heavy metals in the study areas. The major limitation of the study is associated with the lack of detailed Geochemistry data in the specific study areas; the authors had to rely on the general geochemistry of the Freetown layered complex. This causes uncertainties in apportioning the geogenic and anthropogenic sources of pollution.
Author contributions
Conceptualization, Methodology, Formal Analysis, Investigation, Writing-Original Draft Preparation: Abdul Aziz Sankoh; Water sampling and Formal Analysis: Joseph Amara; Geospatial analysis: Tamba Komba; Resources, Supervision; Reviewing and Editing: Ronnie Frazer-Williams; Supervision, Reviewing and Editing: Cynthia Laar; Conceptualization, Formal Analysis and Proofreading: Alusine Sesay; Supervision: Nana Sarfo Agyemang Derkyi.
Ethical responsibilities of Authors
All authors have read, understood, and have complied as applicable with the statement on “Ethical responsibilities of Authors” as found in the instructions for Authors and are aware that with minor exceptions, no changes can be made to authorship once the paper is submitted.
Acknowledgements
This research was funded by the Regional Centre for Energy and Environmental Sustainability (RCEES), UENR; RCEES is acknowledged. The authors would like to thank the Fourah Bay College (USL), Ministry of Water Resources (SL), and Ramsy Medical Laboratories for providing laboratory instrumentation to carry out the heavy metals and physical parameter analyses.
Disclosure statement
No potential conflict of interest was reported by the authors.
Data availability statement
The datasets generated and/or analyzed during the current study are available from the corresponding author on request.
Additional information
Funding
References
- Abd El-Salam, M. M., & Abu-Zuid, G. I. (2015). Impact of landfill leachate on the groundwater quality: A case study in Egypt. Journal of Advanced Research, 6(4), 579–30. https://doi.org/10.1016/j.jare.2014.02.003
- Aboyeji, O. S., & Eigbokhan, S. F. (2016). Evaluations of groundwater contamination by leachates around Olusosun open dumpsite in Lagos metropolis, southwest Nigeria. Journal of Environmental Management, 183, 333–341. https://doi.org/10.1016/j.jenvman.2016.09.002
- Adeolu, A. O., Ada, O. V., Gbenga, A. A., & Adebayo, O. A. (2011). Assessment of groundwater contamination by leachate near a municipal solid waste landfill. African Journal of Environmental Science and Technology, 5(11), 933–940. https://doi.org/10.5897/AJEST11.272
- Akbulut, N. E., & Tuncer, A. M. (2011). Accumulation of heavy metals with water quality parameters in KIzIlIrmak River Basin (Delice River) in Turkey. Environmental Monitoring and Assessment, 173(1–4), 387–395. https://doi.org/10.1007/s10661-010-1394-7
- Alizamir, M., & Sobhanardakani, S. (2016). Forecasting of heavy metals concentration in groundwater resources of Asadabad plain using artificial neural network approach Introduction 1. Journal of Advances in Environmental Health Research, 4(2), 68–77. http://jaehr.muk.ac.ir
- Baghanam, A. H., Nourani, V., Aslani, H., & Taghipour, H. (2020). Spatiotemporal variation of water pollution near landfill site: Application of clustering methods to assess the admissibility of LWPI. Journal of Hydrology, 591, 125581. https://doi.org/10.1016/j.jhydrol.2020.125581
- Barceloux, D. G., & Barceloux, D. (1999). Nickel. Journal of toxicology Clinical toxicology, 37(2), 239–258. https://doi.org/10.1081/CLT-100102423
- Bayatzadeh Fard, Z., Ghadimi, F., & Fattahi, H. (2017). Use of artificial intelligence techniques to predict distribution of heavy metals in groundwater of Lakan lead-zinc mine in Iran. Journal of Mining and Environment, 8(1), 35–48. https://doi.org/10.22044/jme.2016.592
- Bhagat, S. K., Tiyasha, T., Awadh, S. M., Tung, T. M., Jawad, A. H., & Yaseen, Z. M. (2021). Prediction of sediment heavy metal at the Australian Bays using newly developed hybrid artificial intelligence models. Environmental Pollution, 268, 115663. https://doi.org/10.1016/j.envpol.2020.115663
- Bhagat, S. K., Tung, T. M., & Yaseen, Z. M. (2020). Development of artificial intelligence for modeling wastewater heavy metal removal: State of the art, application assessment and possible future research. Journal of Cleaner Production, 250, 119473. https://doi.org/10.1016/j.jclepro.2019.119473
- Bouchard, M., Laforest, F., Vandelac, L., Bellinger, D., & Mergler, D. (2007). Hair manganese and hyperactive behaviors: Pilot study of school-age children exposed through tap water. Environmental Health Perspectives, 115(1), 122–127. https://doi.org/10.1289/ehp.9504
- Bowles, J. F. W., Lyon, I. C., Saxton, J. M., & Vaughan, D. J. (2000). The origin of platinum group minerals from the Freetown intrusion, Sierra Leone, inferred from osmium isotope systematics. Economic Geology, 95(3), 539–548. https://doi.org/10.2113/gsecongeo.95.3.539
- Buragohain, M., Bhuyan, B., & Sarma, H. P. (2010). Seasonal variations of lead, arsenic, cadmium and aluminium contamination of groundwater in Dhemaji district, Assam, India. Environmental Monitoring and Assessment, 170(1–4), 345–351. https://doi.org/10.1007/s10661-009-1237-6
- Cabri, L. J., Oberthür, T., & Keays, R. R. (2022). Origin and depositional history of platinum-group minerals in placers – A critical review of facts and fiction. Ore Geology Reviews, 144, 104733. https://doi.org/10.1016/j.oregeorev.2022.104733
- Cecelia F, F., Lenn G, G., Rafael S, N., Joseph F, C., & Yekeh, H. (2021). Evaluation of soil and groundwater quality at the New Georgia open dumpsite in Caldwell, Liberia. Journal of Soil and Water Science, 5(2), 197–204. https://doi.org/10.36959/624/445
- Cerar, S., & Mali, N. (2016). Assessment of presence, origin and seasonal variations of persistent organic pollutants in groundwater by means of passive sampling and multivariate statistical analysis. Journal of Geochemical Exploration, 170, 78–93. https://doi.org/10.1016/j.gexplo.2016.08.016
- Chalokwu, C. I. (2001). Petrology of the Freetown layered complex, Sierra Leone: Part II. Magma evolution and crystallisation conditions. Journal of African Earth Sciences, 32(3), 519–540. https://doi.org/10.1016/S0899-5362(01)90112-5
- Chaturvedi, A., Bhattacharjee, S., Mondal, G. C., Kumar, V., Singh, P. K., & Singh, A. K. (2019). Exploring new correlation between hazard index and heavy metal pollution index in groundwater. Ecological Indicators, 97, 239–246. https://doi.org/10.1016/j.ecolind.2018.10.023
- Cumar, S. K. M., & Nagaraja, B. (2011). Environmental impact of leachate characteristics on water quality. Environmental Monitoring and Assessment, 178(1–4), 499–505. https://doi.org/10.1007/s10661-010-1708-9
- Das, N., Paul, S., Chatterjee, D., Banerjee, N., Majumder, N. S., Sarma, N., Sau, T. J., Basu, S., Banerjee, S., Majumder, P., Bandyopadhyay, A. K., States, J. C., & Giri, A. K. (2012). Arsenic exposure through drinking water increases the risk of liver and cardiovascular diseases in the population of West Bengal, India. BMC Public Health, 12(1), 1. https://doi.org/10.1186/1471-2458-12-639
- Duodu, G. O., Goonetilleke, A., & Ayoko, G. A. (2016). Comparison of pollution indices for the assessment of heavy metal in Brisbane River sediment. Environmental Pollution, 219, 1077–1091. https://doi.org/10.1016/j.envpol.2016.09.008
- Egbueri, J. C. (2018). Assessment of the quality of groundwaters proximal to dumpsites in Awka and Nnewi metropolises: a comparative approach. International Journal of Energy and Water Resources, 2(1–4), 33–48. https://doi.org/10.1007/s42108-018-0004-1
- Egbueri, J. C. (2020). Heavy Metals Pollution Source Identification and Probabilistic Health Risk Assessment of Shallow Groundwater in Onitsha, Nigeria. Analytical Letters, 53(10), 1620–1638. https://doi.org/10.1080/00032719.2020.1712606
- Egbueri, J. C., & Unigwe, C. O. (2019). An integrated indexical investigation of selected heavy metals in drinking water resources from a coastal plain aquifer in Nigeria. SN Applied Sciences, 1(11), 11. https://doi.org/10.1007/s42452-019-1489-x
- Elumalai, V., Brindha, K., & Lakshmanan, E. (2017). Human exposure risk assessment due to heavy metals in groundwater by pollution index and multivariate statistical methods: A case study from South Africa. Water (Switzerland), 9, 4. https://doi.org/10.3390/w9040234
- Etchie, A. T., Etchie, T. O., Adewuyi, G. O., Kannan, K., Wate, S. R., Sivanesan, S., Chukwu, A. U., & Carlo, M. (2013). Influence of seasonal variation on water quality in tropical water distribution system: is the disease burden significant? Water Research, 49, 186–196. https://doi.org/10.1016/j.watres.2013.11.023
- Facchinelli, A., Sacchi, E., & Mallen, L. (2001). Multivariate statistical and GIS-based approach to identify heavy metal sources in soils. Environmental pollution, 114(3), 313–324. https://doi.org/10.1016/S0269-7491(00)00243-8
- Fatoki, O. S., & Ayodele, E. T. (1991). Zinc and copper levels in tree barks as indicators of environmental pollution. Environment International, 17(5), 455–460. https://doi.org/10.1016/0160-4120(91)90279-Y
- Frazer-Williams, R. K. (2017). Copper (Cu), lead (Pb) and Zinc (Zn) distribution in the Granvillebrook dumpsite soil, Sierra Leone. Toxicological Digestion, 1(2), 131–142.
- Hafsa, N., Rushd, S., Al-Yaari, M., & Rahman, M. (2020). A generalized method for modeling the adsorption of heavy metals with machine learning algorithms. Water (Switzerland), 12(12), 1–22. https://doi.org/10.3390/w12123490
- Hakanson, L. (1980). An ecological risk index for aquatic pollution control.a sedimentological approach. Water Research, 14(8), 975–1001. https://doi.org/10.1016/0043-1354(80)90143-8
- Haloi, N., & Sarma, H. P. (2012). Heavy metal contaminations in the groundwater of Brahmaputra flood plain: An assessment of water quality in Barpeta District, Assam (India). Environmental Monitoring and Assessment, 184(10), 6229–6237. https://doi.org/10.1007/s10661-011-2415-x
- He, Y., Li, B. B., Zhang, K. N., Li, Z., Chen, Y. G., & Ye, W. M. (2019). Experimental and numerical study on heavy metal contaminant migration and retention behavior of engineered barrier in tailings pond. Environmental Pollution, 252, 1010–1018. https://doi.org/10.1016/j.envpol.2019.06.072
- Hingston, E. D. C., Frazer-Williams, R. A. D., Latiff, R. S. A., & Fode, D. V. A. (2002). Waste disposal in the Granville Brook dumpsite and its influence on the environment (Freetown, Sierra Leone). In Proceedings of 9th Congress of the International Assessment for Engineering Geology and, Environment, Durban, South Africa (pp. 16–20).
- Homoncik, S. C., MacDonald, A. M., Heal, K. V., Ó Dochartaigh, B. É., & Ngwenya, B. T. (2010). Manganese concentrations in Scottish groundwater. Science of the Total Environment, 408(12), 2467–2473. https://doi.org/10.1016/j.scitotenv.2010.02.017
- Huang, S. L., Wan, Z. H., & Smith, P. (2007). Numerical modeling of heavy metal pollutant transport-transformation in fluvial rivers. Journal of Hydraulic Research, 45(4), 451–461. https://doi.org/10.1080/00221686.2007.9521779
- Hulskotte, J. H. J., van der Gon, H. A. C. D., Visschedijk, A. J. H., & Schaap, M. (2007). Brake wear from vehicles as an important source of diffuse copper pollution. Water Science and Technology, 56(1), 223–231. https://doi.org/10.2166/wst.2007.456
- Karaouzas, I., Kapetanaki, N., Mentzafou, A., Kanellopoulos, T. D., & Skoulikidis, N. (2021). Heavy metal contamination status in Greek surface waters: A review with application and evaluation of pollution indices. Chemosphere, 263, 128192. https://doi.org/10.1016/j.chemosphere.2020.128192
- Kumar, A., & Krishna, A. P. (2021). Groundwater quality assessment using geospatial technique based water quality index (WQI) approach in a coal mining region of India. Arabian Journal of Geosciences, 14(12), 1–26. https://doi.org/10.1007/s12517-021-07474-9
- Lu, H., Li, H., Liu, T., Fan, Y., Yuan, Y., Xie, M., & Qian, X. (2019). Simulating heavy metal concentrations in an aquatic environment using artificial intelligence models and physicochemical indexes. Science of the Total Environment, 694, 133591. https://doi.org/10.1016/j.scitotenv.2019.133591
- Mgbenu, C. N., & Egbueri, J. C. (2019). The hydrogeochemical signatures, quality indices and health risk assessment of water resources in Umunya district, southeast Nigeria. Applied Water Science, 9(1), 1–19. https://doi.org/10.1007/s13201-019-0900-5
- Mokarram, M., Saber, A., & Sheykhi, V. (2020). Effects of heavy metal contamination on river water quality due to release of industrial effluents. Journal of Cleaner Production, 277, 123380. https://doi.org/10.1016/j.jclepro.2020.123380
- Naminata, S., Kwa-Koffi, K. E., Marcel, K. A., & Marcellin, Y. K. (2018). Assessment and Impact of Leachate Generated by the Landfill City in Abidjan on the Quality of Ground Water and Surface Water (M’Badon Bay, Côte d’Ivoire). Journal of Water Resource and Protection, 10(1), 145–165.
- Ng, S. M. Y., Wai, O. W. H., Li, Y. S., Li, Z. L., & Jiang, Y. (2009). Integration of a GIS and a complex three-dimensional hydrodynamic, sediment and heavy metal transport numerical model. Advances in Engineering Software, 40(6), 391–401. https://doi.org/10.1016/j.advengsoft.2008.09.001
- Nyame, F. K., Tigme, J., Kutu, J. M., & Armah, T. K. (2012). Environmental Implications of the Discharge of Municipal Landfill Leachate into the Densu River and Surrounding Ramsar Wetland in the Accra Metropolis, Ghana. Journal of Water Resource and Protection, 4(8), 622. http://dx.doi.org/10.4236/jwarp.2012.48072
- Ouyang, Y., Nkedi-kizza, P., Wu, Q. T., Shinde, D., & Huang, C. H. (2006). Assessment of seasonal variations in surface water quality. Water research, 40(20), 3800–3810. https://doi.org/10.1016/j.watres.2006.08.030
- Paolalsiam, S., Rama, V., & Kurakalva, M. (2021). Comprehensive assessment of groundwater quality using heavy metal pollution indices and geospatial technique: a case study from Wanaparthy watershed of upper Krishna River basin, Telangana. Environmental Earth Sciences, 80(17), 1–16. https://doi.org/10.1007/s12665-021-09794-1
- Pejman, A., Nabi Bidhendi, G., Ardestani, M., Saeedi, M., & Baghvand, A. (2015). A new index for assessing heavy metals contamination in sediments: A case study. Ecological Indicators, 58, 365–373. https://doi.org/10.1016/j.ecolind.2015.06.012
- Rahman, M. A. T. M. T., Paul, M., Bhoumik, N., Hassan, M., & Alam, K. (2020). Heavy metal pollution assessment in the groundwater of the Meghna Ghat industrial area, Bangladesh, by using water pollution indices approach. Applied Water Science, 1–15. https://doi.org/10.1007/s13201-020-01266-4
- Raikar, R. V., & Sneha, M. K. (2012). Water quality analysis of Bhadravathi taluk using GIS - a case study. International Journal Of Environmental Science, 2(4), 2443–2453. https://doi.org/10.6088/ijes.00202030125
- Raju, N. J., Gossel, W., & Sudhakar, M. (2015). Management of natural resources in a changing environment. Management of Natural Resources in a Changing Environment. 1–301. https://doi.org/10.1007/978-3-319-12559-6
- Raju, V. K., Somashekar, R. K., & Prakash, K. L. (2012). Heavy metal status of sediment in river Cauvery, Karnataka. Environmental Monitoring and Assessment, 184(1), 361–373. https://doi.org/10.1007/s10661-011-1973-2
- Razali, A., Norkhadijah, S., Ismail, S., Awang, S., Praveena, M., & Abidin, E. Z. (2020). The impact of seasonal change on river water quality and dissolved metals in mountainous agricultural areas and risk to human health. Environmental Forensics, 21(2), 195–211. https://doi.org/10.1080/15275922.2020.1728434
- Rezaei, A., Hassani, H., Fard Mousavi, S. B., & Jabbari, N. (2019a). Evaluation of Heavy Metals Concentration in Jajarm Bauxite Deposit in Northeast of Iran Using Environmental Pollution Indices. Malaysian Journal of Geosciences, 3(1), 12–20. https://doi.org/10.26480/mjg.01.2019.12.20
- Rezaei, A., Hassani, H., & Jabbari, N. (2019b). Evaluation of groundwater quality and assessment of pollution indices for heavy metals in North of Isfahan Province, Iran. Sustainable Water Resources Management, 5(2), 491–512. https://doi.org/10.1007/s40899-017-0209-1
- Rivera-Rodríguez, D. A., Beltrán-Hernández, R. I., Lucho-Constantino, C. A., Coronel-Olivares, C., Hernández-González, S., Villanueva-Ibáñez, M., Nolasco-Arizmendi, V., & Vázquez-Rodríguez, G. A. (2019). Water quality indices for groundwater impacted by geogenic background and anthropogenic pollution: case study in Hidalgo, Mexico. International Journal of Environmental Science and Technology, 16(5), 2201–2214. https://doi.org/10.1007/s13762-018-1852-2
- Rizwan, K. M., Thirukumaran, V., & Suresh, M. (2021). Assessment and source identification of heavy metal contamination of groundwater using geospatial technology in Gadilam River basin, Tamil Nadu, India. Applied Water Science, 11(6), 1–24. https://doi.org/10.1007/s13201-021-01436-y
- Sankoh, A. A., Komba, T., Laar, C., Derkyi, N. S. A., & Frazer-Williams, R. (2022a). Application of Multivariate and Geospatial Techniques to Assess Groundwater Quality of Two Major Dumpsites in Sierra Leone. Environmental Nanotechnology Monitoring and Management, 18, 100753. https://doi.org/10.1016/j.enmm.2022.100753
- Sankoh, A. A., Laar, C., Rashid, A., & Frazer-williams, R. A. D. (2022b). Coupling multivariate analysis and Bayesian isotope mixing model to assess the origin and quality of groundwater in the Freetown layered complex, Sierra Leone. Journal of African Earth Sciences, 198, 104808. https://doi.org/10.1016/j.jafrearsci.2022.104808
- Sappa, G., Ergul, S., Ferranti, F., & Sweya, L. N. (2015). Effects of seasonal change and seawater intrusion on water quality for drinking and irrigation purposes, in coastal aquifers of Dar es Salaam, Tanzania. Journal of African Earth Sciences, 105, 64–84. https://doi.org/10.1016/j.jafrearsci.2015.02.007
- Shahid, S. U., Iqbal, J., & Hasnain, G. (2014). Groundwater quality assessment and its correlation with gastroenteritis using GIS: a case study of Rawal Town, Rawalpindi, Pakistan. Environmental Monitoring and Assessment, 186(11), 7525–7537. https://doi.org/10.1007/s10661-014-3945-9
- Sharma, R., Kumar, A., Singh, N., & Sharma, K. (2021). Impact of seasonal variation on water quality of Hindon River: physicochemical and biological analysis. SN Applied Sciences, 3(1), 1–11. https://doi.org/10.1007/s42452-020-03986-3
- Shrivastava, A. K. (2009). A review on copper pollution.pdf. Indian Journal of Environmental Protection, 29(6), 552–560.
- Singh, A. K., Raj, B., Tiwari, A. K., & Mahato, M. K. (2013). Evaluation of hydrogeochemical processes and groundwater quality in the Jhansi district of Bundelkhand region, India. Environmental Earth Sciences, 70(3), 1225–1247. https://doi.org/10.1007/s12665-012-2209-7
- Somani, M., Datta, M., Gupta, S. K., Sreekrishnan, T. R., & Ramana, G. V. (2019). Comprehensive assessment of the leachate quality and its pollution potential from six municipal waste dumpsites of India. Bioresource Technology Reports, 6, 198–206. https://doi.org/10.1016/j.biteb.2019.03.003
- Tiwari, A. K., Kumar, P., Abhay, S., & Singh, K. (2016). Estimation of heavy metal contamination in groundwater and development of a heavy metal pollution index by using GIS technique. Bulletin of Environmental Contamination and Toxicology, 96(4), 508–515. https://doi.org/10.1007/s00128-016-1750-6
- Ukah, B. U., Egbueri, J. C., Unigwe, C. O., & Ubido, O. E. (2019). Extent of heavy metals pollution and health risk assessment of groundwater in a densely populated industrial area, Lagos, Nigeria. International Journal of Energy and Water Resources, 3(4), 291–303. https://doi.org/10.1007/s42108-019-00039-3
- Umeji, A. C. (1983). Geochemistry and mineralogy of the Freetown layered basic igneous complex of Sierra Leone. Chemical Geology, 39(1–2), 17–38. https://doi.org/10.1016/0009-2541(83)90070-0
- Venkatesan, G., Subramani, T., Sathya, U., & Roy, P. D. (2020). Geochemistry seasonal changes in groundwater composition in an industrial center of south India and quality evaluation for consumption and health risk using geospatial methods. Geochemistry, 80(4), 125651. https://doi.org/10.1016/j.chemer.2020.125651
- Wedeen, R. P., & Qiant, L. (1991). Chromium-induced kidney disease. Environmental health perspectives, 92, 71–74. https://doi.org/10.1289/ehp.92-1519395
- Yidana, S. M., Banoeng-yakubo, B., & Akabzaa, T. M. (2010). Analysis of groundwater quality using multivariate and spatial analyses in the Keta basin, Ghana. Journal of African Earth Sciences, 58(2), 220–234. https://doi.org/10.1016/j.jafrearsci.2010.03.003
- Yousry, M. M. (2011). Identification of pollution sources and homogenous regions in lake Nasser water: A multivariate statistical analysis. Proceedings of the 4th Global Fisheries and Aquaculture Research Conference, the Egyptian International Center for Agriculture, Giza, Egypt, 15(3), 151–163.
- Zhuang, Q., Li, G., & Liu, Z. (2018). Distribution, source and pollution level of heavy metals in river sediments from South China. Catena, 170, 386–396. https://doi.org/10.1016/j.catena.2018.06.037