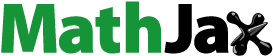
Abstract
It is critical to monitor water quality to keep water bodies ecologically healthy and facilitate the sustainable development of Kenyir Lake. Water quality differs temporally and spatially and is affected by several factors. Typically, water quality inspection systems are cost- and labour-intensive depending on water quality indicator count and sampling frequency. Optimising the frequency and location of water quality sampling is crucial. This study focused on collecting water samples from 22 locations in Kenyir Lake during different seasons (normal, dry, and wet). The study aimed to assess the spatial and temporal variations in the water quality of Kenyir Lake based on multivariate statistical methods. In this study, the following water quality parameters were selected for analysis: temperature, dissolved oxygen (DO), pH, biochemical oxygen demand (BOD), chemical oxygen demand (COD), total suspended solids (TSS), and ammoniacal nitrogen (NH3-N). In addition, a water quality index was also calculated. GIS software was used to assess water quality data, and various multivariate statistical methods like cluster analysis (CA), discriminant analysis (DA), and principal component analysis (PCA) were employed. The outcome shows minor spatial differences concerning Kenyir Lake; however, the temporal variations were noteworthy during this study duration. Cluster analysis divided the locations into 3 clusters with TSS being key parameter affecting the spatial differences in water quality. Stepwise discriminant analysis based on three parameters, pH, temperature, and TSS, produced the associated classification matrix that correctly estimated 69.7% of the input. NH3-N and TSS were found to be the two critical aspects that affect water quality during dry, wet, or normal climatic conditions.
1. Introduction
Lakes and rivers are crucial for human well-being as they offer numerous benefits, including irrigation, potable water network, transportation, hydropower production, leisure, and fishing (Jiang et al., Citation2023; Varadharajan et al., Citation2022). However, as human activities, including urbanization and industrialization, in the surrounding catchment areas of these water bodies increase, they can become contaminated by a range of pollutants (H. Zhang et al., Citation2022), which negatively impact the lake ecosystem in various ways. Lakes often experience a decline in water quality due to point-source runoff inputs, including domestic and industrial wastewater (Wang et al., Citation2016), and non-point-source runoff originating from agricultural and urban activity (Ongley et al., Citation2010). Moreover, long-range atmospheric movement of pollutants also affects water quality. Excessive human activities can have a range of negative impacts on lakes, including eutrophication (Hobæk et al., Citation2012) caused by high levels of nutrients and organic matter, siltation due to poor erosion control in agricultural, construction, logging, and mining activities, the introduction of exotic species, acidification from atmospheric sources, and contamination by toxic and organic compounds.
Water quality monitoring is crucial to handle freshwater resources. Inspecting a water body concerning water quality allows water management authorities to (i) determine if water fulfils the water quality recommendations; (ii) to ascertain contaminants and pollution sources; (iii) to observe water quality patterns, and (iv) evaluate for impairment. Typically, water quality inspection approaches comprise selecting sampling stations, identifying necessary water quality indicators, ascertaining water sampling frequency, and accounting for labour and material costs. Water quality indicators typically comprise physical, chemical, and biological properties (Alobaidy et al., Citation2010). Monitoring processes also encompass subsequent phenomena like laboratory assessment, data processing, and analysis to gather the required insights.
Water quality can be studied spatially and temporally. Pollutant levels differ based on the time scale, such as daily, seasonal and interannual variations (Arheimer & Lidén, Citation2000; Larned et al., Citation2004; Saraceno et al., Citation2009). Spatial water quality differences in a water body are typically associated with source pollutants, land use and catchment characteristics. It is critical to comprehend the spatiotemporal changes in water quality to be able to devise suitable strategies to enhance and manage stream and lake water quality.
Kenyir Lake, current case study, in Terengganu, Malaysia is famous for ecotourism. Increasing annual tourism in Kenyir has further impacted the water quality in Kenyir Lake. Hence, inspecting the lake’s water quality is critical to protect the ecosystem and ensure the sustainability of Kenyir Lake. The temporal and spatial water quality differences are typically needed for inspecting and determining the physical, chemical, and biological properties of receiving water. It creates a sophisticated data matrix having numerous physicochemical parameters (physical, nutrient, inorganic, and biological characteristics) that might be challenging to assess and comprehend owing to the latent associations between variables and monitoring locations (Koklu et al., Citation2010; Q. Zhang et al., Citation2009; Zhou et al., Citation2021). Hence, it is critical to derive useful information from such data sets without skipping vital aspects (Q. Zhang et al., Citation2009; Zhou et al., Citation2021). To build a suitable framework to determine lake water quality, this research aimed to evaluate water quality in Kenyir Lake using multivariate statistical methods to determine spatiotemporal patterns, ascertain critical parameters, and categorise pollution sources and relative quantitative contributions to water quality problems.
2. Sources of pollution
2.1. Point source
Point source pollution is referred to as pollution from a known point of discharge or fixed outlet and can be released into water bodies in pipes or man-made drainage (Gyawali et al., Citation2013). Some examples of point source pollution are pipe discharges, industrial outflows, tributaries, industrial or municipal wastewater treatment plant outflows. Since possible contaminants from a point source can be easily monitored by measuring discharge and pollutant levels from an identified discharge point, its impact is easy to define and regulate. The focus over the previous years of research work was to address point source pollution through managing the known point of discharges, such as urban wastewater effluent, as described by Citation2010) and Perona et al. (Citation1999) which was found to have been successfully under pollution control and management.
2.2. Non-point source
Non-point source (NPS) pollution is referred to as pollution from unknown sources or its origin cannot be guaranteed from a singular source, rather a combination of sources of different natures, which are often difficult to identify at a fixed locality. Generally, NPS pollution originates from stormwater runoffs from agricultural and anthropogenic activities at urban catchment surfaces. For example, the pollutants from a nearby agricultural land use can be entered into the river by rain-runoff or infiltrate through the soil layers into the groundwater aquifers. Pollutants include but not limited to excess fertilizers from agricultural lands, toxic chemicals from urban runoff, sediment from improperly managed construction sites, and nutrients from livestock, pet wastes, and faulty septic systems. These pollutants will deteriorate the water quality of receiving waters if no control measures are taken.
Non-point source pollution is a major problem for surface waters because it is often challenging to identify the source of the pollution as its origin can be diffused from different unknown sources. Land use surveys and groundwater or surface water quality samples are the only ways of identifying possible locations of nonpoint sources. Therefore, NPS pollution has become the major cause of water quality pollution (Gyawali et al., Citation2013; Li et al., Citation2009; Zampella et al., Citation2007). Recent studies focus on determining the relationship between land use with water quality at different scales of operation and management.
3. Spatial and temporal differences in water quality
Spatial and temporal differences concerning water quality are primarily due to three key processes (1) source: pollution source within the catchment area, (2) mobilisation: the separation of these pollutants from their source due to erosion, weathering, or biogeochemical processes, and (3) deliver: the movement of separated constituents from the catchment region to receiving areas (Granger et al., Citation2010).
Typically, the aspects that influence spatial differences in water quality include human activity in catchment areas: vegetation characteristics, land use and handling, and natural aspects like climate, geology, soil type, topography, and hydrology (Onderka et al., Citation2012). In contrast, temporal water quality differences may be affected by aspects like streamflow (Mellander et al., Citation2015; Sharpley et al., Citation2002) and rainfall and air temperature (Lecce et al., Citation2006; Robson, Citation2014). Atmospheric aspects may impact the mobilisation and movement of constituents from the catchment to receiving areas. Water temperature may regulate biogeochemical processes concerning nutrients in streams (Roberts & Mulholland, Citation2007). Moreover, the levels of substances in the catchment (source) are affected by antecedent dry periods (Arheimer & Lidén, Citation2000; Lecce et al., Citation2006), vegetation cover (Kaushal et al., Citation2014; Ouyang et al., Citation2010), and seasonal differences concerning anthropogenic activities (Stutter et al., Citation2008).
4. Methodology
4.1. Study area
This study was performed at Kenyir Lake, about 40 km inland from Kuala Terengganu, Terengganu, Malaysia. Its coordinates are 5º 1‘20“North and 102º 54’ 30” East. Kelantan and Pahang are situated west and south of Kenyir Lake. The lake was formed due to hydroelectric dam built for power and flood control in 1986. Kenyir Lake has total surface and catchment areas of 369 km2 and 2,612 km2, respectively. At the Full Supply Level (FSL), the lake stores a gross volume of 13.6 billion m3. The average and maximum depths are 37 and 145 metres, respectively. The reservoir is fed by six primary tributaries, namely Sg. Terengganu (primary river), Sg. Terengan, Sg. Cacing, Sg. Petang, Sg. Tembat and Sg. Petuang. The lake supports rich biodiversity and thousands of species with over 8000 flowers, 2500 plants, 8000 orchids, 300 fungi, 370 birds, and 300 freshwater fish species (Izharuddin Shah Kamaruddin et al., Citation2011; Rouf et al., Citation2009). Kenyir Lake in Terengganu is renowned as an ecotourism hotspot, with numerous resorts and tourist activities located in its catchment area. However, the growing number of visitors to Kenyir each year has contributed to the decline in the lake’s water quality. To preserve its ecosystem and ensure its long-term sustainability, it is essential to regularly monitor the water quality of Kenyir Lake.
4.2. Water sample and field data collection
Twenty-two sampling points around Kenyir Lake were identified, and water was sampled. Figure displays the sampling location around Kenyir Lake, with their corresponding location name presented in Table . The primary datasets correspond to the three seasons: dry (August 2018), normal (April 2019) and wet (December 2018). In this study, the sample collection procedure followed the protocol described in the Environmental Protection (Water) Policy 2009 - Monitoring and Sampling Manual for surface water samples. Sampling was performed manually, utilizing 1.5-liter HDPE bottles, by collecting the water at a depth of 0.3 meters below the surface to exclude any scum or debris. In-situ evaluation of water samples was performed by employing the YSI Pro handheld multi-parameter meter. The evaluated in-situ aspects are water temperature, dissolved oxygen (DO), conductivity, salinity, total dissolved solids (TDS) and pH. Subsequently, the samples were immediately placed in an ice box at 4° C to ensure preservation. They were then moved to the environmental laboratory at the Universiti Tenaga Nasional (UNITEN), Malaysia.
Table 1. Sampling points with corresponding location name in Kenyir reservoir
4.3. Laboratory evaluation of water samples
This study evaluates several water quality aspects like Biochemical Oxygen Demand (BOD5), Chemical Oxygen Demand (COD), Total Suspended Solid (TSS), Ammonia Nitrogen (NH3-N), pH and Dissolved Oxygen (DO). These are the basic parameters for determining the water quality index (WQI) of a river/reservoir body which is adopted by The Department of Environment (DOE) in Malaysia. All laboratory evaluations were based on the standard water and wastewater examination techniques (American Public Health Association (APHA), 2017). The methods used for measurement of these parameters are presented in Table , which also contains their limiting values and units. The limiting values are approximate values obtained from the classification of different classes in Malaysian National Water Quality Standards (EQR2006) (Table ).
Table 2. Summary of water quality parameters
Table 3. Malaysian national water quality standards (EQR2006)
4.4. Water Quality Index (WQI)
The Malaysian Department of Environment (DOE) employs Water Quality Index (WQI) to assess water quality. The fundamental aspects for computing WQI comprise DO, BOD, COD, NH3-N, TSS and pH, and it is calculated using the following expression:
where;
SIDO = Subindex of DO (% saturation)
SIBOD = Subindex of BOD
SICOD = Subindex of COD
SIAN = Subindex of NH3-N
SISS = Subindex of SS
SipH = Subindex of pH
Subindices needed for calculating WQI are ascertained based on these parameters. WQI is measured in the 0–100 range, and contamination levels are designated as: 81–100 – clean, 60–80 – Slightly Polluted, and 0–59 – Polluted. Additional WQI categorisation and other aspects into categories as specified by National Water Quality Standards are listed in Table .
4.5. GIS-based water quality mapping
A geographical information system (GIS) is a database intended to handle all varieties of geographically referenced information. This study used GIS software (ArcGIS 9) to build visual maps to depict water quality outcomes in Kenyir Lake. The coordinates of sampling locations and water quality data pertaining to the three seasons were input into the GIS dataset, including soil maps and topographic data. The results section depicts the visual map pertaining to every WQI variable.
4.6. Multivariate statistical methods
4.6.1. Cluster analysis
Cluster analysis comprises several multivariate methods with the primary objective to categorise objects using their inherent characteristics. Cluster analysis provides object classification, allowing other intra-cluster objects to be similar based on a prespecified selection rule. The resulting object clusters must demonstrate high internal (intra-cluster) homogeneity and high external (inter-cluster) heterogeneity. Hierarchical agglomerative clustering is the most widely used technique that offers intuitive similarity associations concerning one set and the complete set, usually depicted as a dendrogram. This work uses cluster analysis to categorise inspection sites using several parameters: temperature, DO, pH, BOD, COD, TSS, ammonia nitrogen and water quality index. Evaluation is conducted on the parameter means for every station. A dendrogram was prepared, consolidating the sampling sites into three statistically significant clusters. The outcomes categorise the distinct subsets (clusters) comprising the ascertained values.
4.6.2. Principal component analysis
PCA is intended to change the initial variables into fresh, uncorrelated variables (axes), referred to as principal components, representing linear combinations of the original variables. The new axes are aligned along the maximum variance direction. PCA was used with normalised data to contrast the compositional sequence comprising the assessed water samples and to ascertain the aspects affecting each sample. PCA-specific axis rotation created new factor sets comprising a subset of the initial variables categorised across groups. The raw data were treated using PCA to process all water quality variables and observations to determine the aspects associated with contamination sources. PCA offers information concerning the critical aspects representative of the entire dataset with respect to interpretation, reduction, and summarisation of the statistical association concerning water constituents with little information loss.
Due to improperly chosen variables, PCA is affected by missing data points, outliers, and inadequate linear correlation among variables. Hence, a comprehensive dataset pre-processing must be implemented to obtain an accurate image representing complex data. It is a widely used method for recognising patterns to account for the variance concerning a massive set of inter-correlated variables and reducing them into relatively small independent (uncorrelated) variables (principal components).
In practice, CA and PCA can fail to preserve the similarities within the clusters even while they successfully preserve the global data structure by creating well-separated clusters. As a result, because CA and PCA rely on the correlation of the variables for their analysis, a high sample size is typically necessary for a reliable result. It is possible to express the sample size as absolute numbers or as ratios susceptible to change. For PCA, it is advised that the absolute sample size be at least 50, or at least 10 or 5 times the number of variables. A recommended sample size scale, on the other hand, suggests that a sample size of 300 is fine and over 1000 is outstanding. As a general rule, 50 samples minimum (or more is preferable) would be sufficient for CA and PCA analysis (Jolliffe & Cadima, Citation2016).
4.6.3. Discriminant analysis
Discriminant analysis (DA) is employed for segregating observations into category-specific values, typically a dichotomy. Considering that discriminant analysis is suitable for a dataset, the produced classification of correct and incorrect predictions is expected to have a high correct percentage. This study aims to use discriminant analysis to determine the most critical variables concerning the spatial variability among the clusters. Water quality variables are predictors, while the clusters represent the predicted variable. The water quality indicators used for this analysis are temperature, DO, pH, BOD, COD, TSS, and ammonia nitrogen. DA uses several quantitative aspects to differentiate two or more naturally present datasets. Temporal changes concerning water quality are estimated using DA, which uses several quantitative indicators for differentiation. DA offers a statistical categorisation of observations and is implemented using prior information concerning the category of objects and the associated clusters. DA consolidates observations with shared properties. It creates a discriminant expression representing every group by processing raw data.
5. Results and discussion
5.1. General properties of spatial water quality in Kenyir Lake
The overall water quality outcomes were assessed using 22 sampling locations in Kenyir Lake across three seasons. depicts the mean values of temperature, pH, DO, BOD, TSS, COD, NH-N and WQI, respectively. The outcomes indicate that the observed temperature and pH levels are similar for all sampled areas in Kenyir Lake. The average water surface temperature in the lake is typically around 30.5 ºC. Further, the average DO and pH concerning the lake’s surface are 7.21 mg/L and 6.8, respectively. Considering research conducted by Kamaruddin et al. (Citation2010), the outcomes indicated average temperature, DO, and pH levels as 29.76 ºC, 6.18 mg/L and 6.91, respectively, for the Pengkalan Gawi-Pulau Dula area in Kenyir Lake. Earlier work by Yusoff and Ambak (Citation1999) concerning Kenyir Lake indicate temperature, pH, and DO ranges of 24.2 to 30.6 ºC, 6.72 to 7.61, and 3.50 to 8.90 mg/L, respectively. Moreover, Shuhaimi-Othman and Lim (Citation2006) indicated that the Chini Lake in Pahang had an average temperature, DO, and pH levels of 29.73 ± 0.44 °C, 6.08 ± 0.88 mg/L and 6.63 ± 0.24, respectively. The mean values for these variables were calculated over the sampling period. The similarity between the two lakes might be attributed to similar ecological characteristics. Azmi and Geok (Citation2016) studied the pH level of Kenyir Lake, indicating a range 6.63–6.91 to be considered as suitable because most aquatic insects can survive in water having a 5 to 8 pH range. The authors indicated that houseboat wastewater release is the primary aspect causing water quality degradation. However, the wastewater is diluted owing to the relatively high quantities of reservoir water.
The average BOD and COD levels for the Kenyir Lake across all sampling locations are recorded as 4.7 ± 1.9 mg/L and 8.0 ± 1.6 mg/L, respectively. Nevertheless, the BOD and COD values vary significantly across the sampling locations. It is attributed to wastewater disposal differences at the sampling locations. Areas supporting recreational activities pollute more than other sources, like rivers. The corresponding BOD and COD averages are between 1.1 to 8.5 mg/L and 5.3 to 12.3 mg/L, respectively. In contrast, the mean TSS and NH3-N levels aggregated across 22 sampling locations in Kenyir Lake were around 81.8 mg/L and 0.07 mg/L, respectively. TSS levels are between 53.3 mg/L and 120 mg/L, while NH3-N ranges between 0.03 mg/L and 0.40 mg/L. Sampling locations’ water quality is affected by proximal land use patterns, affecting BOD, COD, TSS and NH3-N concerning the Kenyir Lake. However, WQI levels range between 80.0 and 91.0, providing an 85.4 average WQI, classified as Class II per the NWQS.
5.2. Cluster analysis
In order to ascertain several similar inspection points and evaluate the spatial heterogeneity of water quality, cluster analysis was performed on the water quality dataset. Cluster analysis produced a dendrogram that consolidates the 22 sampling locations into three separate clusters, as listed in Table . Figure depicts the resulting dendrogram concerning sample site clustering using water quality properties. The first cluster comprises Lake land resort, Sg. Terengganu, Sg. Tembat, Suria Rakit Resort, Sg. Mandak, Taman Orkid, Butterfly Island, Sg.Petang (border) and Sg.Kenyir. The second cluster comprises Musang Kenyir Resort, Taman Tropika, Sg.Petang, Petang Island Resort, Dam (LEFT), Dam (RIGHT), Sg. Comor, Eco Snapper Farm and Herb Garden, while the third comprises the Sg. Petuang, Eco Resort, Pulau Bayas and Dam (MIDDLE) sampling locations.
Table 4. Cluster information with location and mean values
Figure depicts the water quality boxplots for every cluster. These plots are compared inter-cluster to ascertain spatial differences concerning the water quality of Kenyir Lake. Figure 4.10 depicts boxplot outcomes, suggesting cluster 2 has higher BOD, COD, TSS and NH3-N levels; however, its WQI is superior to clusters 1 and 3. It indicates that the sampled areas in cluster 2 comprise relatively more polluted water than the other two. The average observations concerning temperature, DO, pH, BOD, COD, TSS, NH3-N and WQI for cluster 1 are 30.5 ºC, 7.11 mg/L, 6.82, 4.80 mg/L, 7.85 mg/L, 59.26 mg/L, 0.05 mg/L and 86.93, respectively. Further, the cluster 2 has average temperature, DO, pH, BOD, COD, TSS, NH3-N and WQI readings of 30.5 ºC, 7.46 mg/L, 6.84, 5.03 mg/L, 8.48 mg/L, 108.57 mg/L, 0.11 mg/L and 83.24, respectively. Lastly, average readings concerning temperature, DO, pH, BOD, COD, TSS, NH3-N and WQI at cluster 3 are 30.6 ºC, 7.05 mg/L, 6.67, 4.19 mg/L, 7.50 mg/L, 84.44 mg/L, 0.06 mg/L and 85.78, respectively, for cluster 3.
To ascertain the inter-cluster significance, a one-way ANOVA analysis was performed using SPSS version 17.0. The ANOVA outcomes indicate that TSS and WQI levels have a significant difference between the three clusters. Hence, it is understood that TSS is a key parameter affecting the spatial differences concerning water quality.
TSS is a metric to categorise the sampling points into five classes: Class I, II, III, IV and V. TSS measurements have a significant difference concerning the three clusters, suggesting that human factors impact the varying TSS levels in Kenyir Lake. High TSS levels were found near recreational and agricultural land, such as Herb Garden, Fish Farms and resorts. Decaying animal and plant matter produces organic matter that increases TSS. This research established that anthropogenic activity has a noteworthy impact on TSS, which in turn impacts water quality.
All three clusters are designated class II on WQI, based on the Malaysian DOE water quality classification. Nevertheless, cluster 2 has relatively higher pollution levels than the other two clusters basis WQI outcomes.
Several recreational and agriculture parcels are located in the second cluster: Herb Garden, Tropical Garden, Eco Snapper Farm and resorts. Hence, cluster 2 WQI was higher. Typically, water quality indicators like BOD and TSS are class III for all clusters. Further, pH is the only aspect designated class II. Moreover, indicators like DO, COD and NH3-N are class I for all clusters.
5.3. General properties concerning temporal water quality in Kenyir Lake
Citation2013) and Syed Jamaludin et al. (Citation2010) suggest that May to August is the period when the southwest monsoon (SWM) is active, while the northeast monsoon (NEM) is active between November and February. Moreover, September to October and March to April are considered inter-monsoon (IM) months. S. Abdullah and Ismail (Citation2019) suggested that NEM leads to a maximum rainfall of 370 mm, leading to a 26.5 ºC minimum temperature. SWM causes overall rainfall of 117.8 mm and a temperature of 27.6 ºC. Additionally, 178.6 mm and 27.3 ºC rainfall and temperature, respectively, are attributed to the IM. The authors indicated that Kenyir Lake is relatively dry during SWM.
Ambak and Jalal (Citation1998) asserted that Kenyir Lake typically witnesses heavy rains between November and March during NEM. The dry, hot season typically occurs between May and October. Water quality differences concerning the normal, dry, and wet seasons are evaluated using ANOVA, suggesting that water temperature, DO, pH, COD, TSS and WQI have significant differences. The temporal changes concerning overall water quality are depicted in box plots (Figure ).
5.4. Principal Component Analysis
This study used principal component analysis (PCA) on water quality datasets representing seven aspects for the 22 sampling sites. PCA aims to process the datasets, reduce dimensions, and ascertain latent factors impacting water quality. The eigenvalue criterion and scree plot were used to ascertain the count of significant principal components (PCs). Components having>1 were considered principal components. In this research, PCA determined two powerful PCs with eigenvalue exceeding one; the scree plot in Figure accounts for about 57% of the overall variance in the associated water quality datasets. The first and second PCs (Table ) account for 37.8% and 19.3% of the total variance, respectively. Varimax rotation was implemented on the determined PCs to enhance the inference of factor analysis outcomes. The first and second rotated PCs explain about 30.3% and 26.8% of the overall variance, respectively (Table ). Factor loading designations are strong for values exceeding 0.75, moderate between 0.5 and 0.75, and weak between 0.30 and 0.50. This study considered principal components with strong factor loadings (>0.70). The first rotated PC exhibits a strong correlation with DO, pH, and temperature. The second PC strongly correlates with NH3-N and TSS. The first PC may be inferred as being related to aspects affected by natural or weather factors. DO correlates positively with pH; however, these two factors have a negative correlation with temperature. This outcome helps us understand that temperature varies inversely with DO and pH. It suggests that higher temperatures reduce DO levels in Kenyir Lake. The second PC correlates strongly with NH3-N and TSS, suggesting that this contamination source is typically due to anthropogenic activities. NH3-N is produced from agricultural and domestic activities, typically fertilisers and organic or faecal matter. TSS and NH3-N levels were high in Herb Garden, Tropical Garden, Eco Snapper Farm, and resorts. Fertilisers used in Herb Garden and Tropical Garden are responsible for the NH3-N and TSS levels. PCA/FA could not produce significant data reduction because it uses five parameters (71 % of the original 7) needed to account for the 57.1 % of the overall water quality data variance. However, additional water quality indicators concerning physical, chemical, biological, nutrient, and heavy metal characteristics of Kenyir Lake should be gathered to enhance PCA outcomes concerning the better explanation of total water quality variance.
Table 5. Total variance explained by significant PCs for water quality datasets
Table 6. Total variance explained by significant rotated PCs for water quality datasets
PCA is conducted further on seasonal water quality (dry, normal, and wet seasons). The eigenvalue limits indicate two significant PCs concerning the seasonal water quality datasets, as in Table . The PCs explain 50.5%, 48.9% and 51.0% variance concerning the normal, dry, and wet seasons, respectively. The initial VF outcome concerning normal season water quality indicates that temperature and DO are strongly and positively correlated. The second VF shows a positive correlation with TSS and NH3-N. Likewise, the first VFs concerning the dry and wet seasons also correlate positively with TSS and NH3-N. The second VF concerning the dry season exhibits a strong and positive temperature-specific loading. Concerning the normal season, VF1 shares a strong positive loading with temperature and DO, suggesting that these variables are common for monthly water quality inspection concerning Kenyir Lake. NH3-N and TSS are the two significant indicators that affect water quality regardless of the season.
Table 7. Total variance explained by PCs for normal, dry, and wet seasons
The significant positive correlation concerning these two variables may account for their recreational and agricultural origin around areas near Kenyir Lake. These variables are considered outcomes of human activity close to the lake: Eco Snapper Farm, Herb Garden and Tropical Garden. Organic and inorganic material pertaining to NH3-N enters the lake through surface runoff.
5.5. Discriminant analysis
Spatial discriminant analysis was performed using water quality outcomes concerning the three clusters obtained after cluster analysis. Discriminant analysis aims to ascertain the major variables connected to the spatial variability between the three clusters. The clusters comprise the predicted variable; every other water quality indicator is a predictor. Discriminant function coefficients (DFs) and classification matrices (CMs) produced from the conventional and stepwise discriminant analysis approaches are listed in Tables . The determined categorisation function coefficients indicate that some indicators like COD and NH3-N have negative values. Conventional discriminant analysis produces the associated classification having 74.2% accuracy. Further, the stepwise approach depends on three indicators: pH, temperature and TSS; its classification matrix has a 69.7% accuracy. Discriminant analysis implemented concerning spatial water quality variables suggested that significant variables strongly affect water quality for different areas of Kenyir Lake. The outcomes offer better insights concerning water catchment management and devising better inspection approaches to safeguard Kenyir Lake’s water quality.
Table 8. Classification function coefficients
Table 9. Classification results (standard mode)
Table 10. Classification results (stepwise mode)
5.6. Geographical Information System (GIS)
GIS software helped assess the spatial variability concerning Kenyir Lake’s water quality. This section comprises the changes in water quality indicators and a map depicting spatial differences.
Low BOD levels suggest high water quality and vice versa. Figure indicates that BOD peaked at 9 mg/L, designated Class IV. This BOD peak was detected in the dry season from Sungai Petang (border) and Sungai Comor. Reduced water flow might be responsible for speedier organic matter breakdown during the dry season (P. Abdullah & Khalik, Citation2012). If the water supply comprises excessive organic waste, the bacterial presence is expected to be high, leading to faster decomposition. Consequently, oxygen requirement will be high (owing to bacterial decomposition), leading to higher BOD levels.
Chemical oxygen demand (COD) indicates the levels at which water takes up oxygen as the organic matter breaks down and inorganic substances oxidise. Figure indicates a 13 mg/L peak COD level pertaining to the Musang Kenyir Resort and Herb Garden during the normal and dry seasons. Extensive application of organic fertiliser and sewage release impact COD concentrations. Agricultural runoff and household water are responsible for high COD. Nevertheless, the peak COD level was good (Class II) as per the National Water Quality Standards.
Figure indicates that the peak DO level was associated with the Herb Garden during the wet season. In contrast, the lowest DO level was associated with the Dam (left) during the dry season. Human activity might result in DO levels falling below the essential range. Water levels reduce during the dry period as river flow reduces. Water flow and rainfall affect DO levels, which are expected to be high with higher water discharge and velocity.
Ammonia is among the significant contaminants since it is widespread; however, it is harmful and reduces reproduction and development, or it might cause death. Unionised neutral ammonia (NH₃) affects aquatic beings adversely. Ammonia peaks during the dry season (0.9 mg/L) at the Eco Snapper Farm, resulting in a Class IV level (Figure ). Ammonia levels increase with water temperature. Ammonia levels rise due to unconsumed food breakdown (Begum et al., Citation2014). Excess food flowing into the lake reduces water quality.
Total Suspended Solids comprise different materials like silt, decaying animal and plant residue, and sewage. Figure indicates a peak TSS level of 120 mg/L at the Eco Snapper Farm during the dry season. These levels are adverse (Class IV). The Eco Snapper Farm cultures red tilapia, and excess waste from fish leads to massive areas loaded with green slime on the water’s surface.
Water acidity or basicity is determined using pH. Water having relatively high free hydrogen ions is acidic, while higher hydroxyl ion levels make water basic. Water pH is impacted by chemicals, and it is a critical indicator of chemical changes in water. Typically, this research found acceptable pH () and water quality. The average pH levels correspond to the acceptable regulatory range. The wet season leads to pH reduction, owing to the mildly acidic nature of rainwater (pH about 5.0). Hence, pH is expected to be low during the wet season (Kamaruddin et al., Citation2010).
Temperature changes trigger variations in water quality. Warm water is associated with reduced dissolved oxygen and may not have sufficient levels to support several aquatic species. Several chemicals are relatively toxic to aquatic beings at elevated temperatures (Kamaruddin et al., Citation2010). Figure depicts the spatial variability in temperature during dry, normal, and wet seasons at Kenyir Lake.
Figure indicates water quality falling in Class II for every season, indicating suitability for recreational purposes. During the dry season, domestic sewage, industrial discharge, and saltwater intrusion primarily trigger water quality variations. In contrast, water pollution during the wet season is caused by drainage from cultivated land and farms, including other contamination sources, affecting water quality.
6. Conclusion
An assessment of Kenyir Lake’s water quality indicators highlights more temporal than spatial variations for the studied period. Total suspended solid (TSS) is the single parameter that has a major difference (p < 0.05) across sampling sites. Further, water quality tests concerning normal, dry, and wet seasons based on ANOVA indicated that water temperature, DO, pH, COD, TSS and WQI had significant differences. Kenyir Lake has Class II overall water quality, indicating suitability for supply use, sensitive aquatic beings, recreational use, and human contact, based on the Malaysian National Water Quality Standards. Cluster analysis split all sampling locations into three groups. Stepwise discriminant analysis suggests pH, TSS, and temperature as the critical variables affecting spatial water quality variability concerning the 22 sampling sites. PCA count did not reduce data significantly because it generated 5 parameters (i.e., 71 % of the initial 7), accounting for about 57.1 % of the overall water quality variance. NH3-N and TSS are significant indicators that affect water quality regardless of the season.
Authors Contributions
Material preparation, data collection and analysis were performed by Poornasuthra AP Subramaniam, Chow Ming Fai, Marlinda Abdul Malek, Ali Najah Ahmed and Ahmed Elshafie. The first draft of the manuscript was written by Pavitra Kumar. Manuscript was revised by Ali Najah Ahmed, Mohsen Sherif and Ahmed Elshafie.
Availability of data and materials
This research is based on manually collected data which will be made available upon request.
Ethical approval
NA
Consent to Participate:
No human or animal participants involved in this research
Consent to Publish:
All authors have read and approved the final manuscript for publication.
Disclosure statement
No potential conflict of interest was reported by the authors.
References
- Abdullah, S., & Ismail, M. (2019). The weather and climate of tropical tasik Kenyir, Terengganu. In M. T. Abdullah, A. Mohammad, M. N. Zalipah, & M. S. Lola (Eds.), Greater Kenyir Landscapes: Social Development and environmental sustainability: From Ridge to Reef (pp. 3–29). Springer International Publishing.
- Abdullah, P., & Khalik, W. (2012). Seasonal influence on water quality status of temenggor lake, perak. Malaysian Journal of Analytical Sciences, 16(2), 163–171.
- Alobaidy, A., Abid, H., & Maulood, B. (2010). Application of water quality index for assessment of dokan lake ecosystem, Kurdistan Region, Iraq. Journal of Water Resource and Protection, 2(9), 792–798. https://doi.org/10.4236/jwarp.2010.29093
- Ambak, & Jalal. (1998). Habitat utilization by the tropical fish community in the man-made Lake Kenyir, Malaysia. Fisheries Management and Ecology, 5(2), 173–176. https://doi.org/10.1046/j.1365-2400.1998.00097.x
- Arheimer, B., & Lidén, R. (2000). Nitrogen and phosphorus concentrations from agricultural catchments—influence of spatial and temporal variables. Journal of Hydrology, 227(1), 140–159. https://doi.org/10.1016/S0022-1694(99)00177-8
- Azmi, W. A., & Geok, H. A. (2016). Aquatic insect communities in relation with water quality of selected tributaries of tasik kenyir terengganu. Journal of Sustainability Science and Management, 11(2), 11–20.
- Begum, A., Mondal, S., Ferdous, Z., Zafar, M. A., & Ali, M. (2014). IMPACT of WATER QUALITY PARAMETERS on MONOSEX TILAPIA (Oreochromis niloticus) PRODUCTION UNDER POND CONDITION. International Journal of Animal and Fisheries Science, 2.
- Daryabor, F., Tangang, F., & Juneng, L. (2013). Simulation of southwest monsoon current circulation and temperature in the east coast of Peninsular Malaysia. Sains Malaysiana, 43(3), 389–398.
- Granger, S. J., Hawkins, J. M. B., Bol, R.et al. (2010). High Temporal Resolution Monitoring of Multiple Pollutant Responses in Drainage from an Intensively Managed Grassland Catchment Caused by a Summer Storm. Water, Air, and Soil Pollution, 205, 377–393. https://doi.org/10.1007/s11270-009-0083-z
- Gyawali, S., Techato, K., Yuangyai, C., & Musikavong, C. (2013). Assessment of relationship between land uses of riparian zone and water quality of river for sustainable development of river basin, a case study of U-Tapao river basin. Thailand Procedia Environmental Sciences, 17, 291–297. https://doi.org/10.1016/j.proenv.2013.02.041
- Hobæk, A., Løvik, J. E., Rohrlack, T., Moe, S. J., Grung, M., Bennion, H., Clarke, G., & Piliposyan, G. T. (2012). Eutrophication, recovery and temperature in Lake Mjøsa: Detecting trends with monitoring data and sediment records. Freshwater Biology, 57, 1998–2014. https://doi.org/10.1111/j.1365-2427.2012.02832.x
- Jiang, J., Men, Y., Pang, T., Tang, S., Hou, Z., Luo, M., Sun, X., Wu, J., Yadav, S., Xiong, Y., Liu, C., & Zheng, Y. (2023). An integrated supervision framework to safeguard the urban river water quality supported by ICT and models. Journal of Environmental Management, 331, 117245. https://doi.org/10.1016/j.jenvman.2023.117245
- Jolliffe, I. T., & Cadima, J. (2016). Principal component analysis: A review and recent developments. Philosophical Transactions of the Royal Society A: Mathematical, Physical and Engineering Sciences, 374(2065), 20150202. https://doi.org/10.1098/rsta.2015.0202
- Kamaruddin, I. S., Abdul Satar, M. K., Christianus, A., Daud, S. K., & Abit, L. Y. (2011). Fish community in Pengkalan Gawi - Pulau Dula section of Kenyir Lake, Terengganu, Malaysia. Journal of Sustainability Science and Management, 6(1), 89–97.
- Kamaruddin, I. S., Kamal, A. S. M., Christianus, A., & Daud, S. K. (2010). A study on dissolved oxygen, pH and temperature of the lake water in Pengkalan Gawi – Pulau Dula Section of Kenyir Lake, Terengganu. Towards a Healthier and Sustainable Future: International Annual Symposium on Sustainability Science and Management (UMTAS 2010), 9(2), 482–486.
- Kaushal, S. S., Mayer, P. M., Vidon, P. G., Smith, R. M., Pennino, M. J., Newcomer, T. A., Duan, S., Welty, C., & Belt, K. T. (2014). Land Use and Climate Variability Amplify Carbon, Nutrient, and Contaminant Pulses: A Review with Management Implications. Journal of the American Water Resources Association (JAWRA), 50(3), 585–614. https://doi.org/10.1111/jawr.12204
- Koklu, R., Sengorur, B., & Topal, B. (2010). Water quality assessment using multivariate statistical methods—a case study: melen river system (Turkey). Water Resources Management, 24(5), 959–978. https://doi.org/10.1007/s11269-009-9481-7
- Larned, S. T., Scarsbrook, M. R., Snelder, T. H., Norton, N. J., & Biggs, B. J. F. (2004). Water quality in low‐elevation streams and rivers of New Zealand: Recent state and trends in contrasting land‐cover classes. New Zealand Journal of Marine and Freshwater Research, 38(2), 347–366. https://doi.org/10.1080/00288330.2004.9517243
- Lecce, S. A., Pease, P. P., Gares, P. A., & Wang, J. (2006). Seasonal controls on sediment delivery in a small coastal plain watershed, North Carolina, USA. Geomorphology, 73(3), 246–260. https://doi.org/10.1016/j.geomorph.2005.05.017
- Li, J., Carlson, B. E., & Lacis, A. A. (2009). A study on the temporal and spatial variability of absorbing aerosols using total ozone mapping spectrometer and ozone monitoring instrument aerosol index data. Journal of Geophysical Research, 114(D9), 114. https://doi.org/10.1029/2008JD011278
- Mellander, P. -E., Jordan, P., Shore, M., Melland, A. R., & Shortle, G. (2015). Flow paths and phosphorus transfer pathways in two agricultural streams with contrasting flow controls. Hydrological Processes, 29(16), 3504–3518. https://doi.org/10.1002/hyp.10415
- Onderka, M., Krein, A., Wrede, S., Martínez-Carreras, N., & Hoffmann, L. (2012). Dynamics of storm-driven suspended sediments in a headwater catchment described by multivariable modeling. Journal of Soils and Sediments, 12(4), 620–635. https://doi.org/10.1007/s11368-012-0480-6
- Ongley, E. D., Xiaolan, Z., & Tao, Y. (2010). Current status of agricultural and rural non-point source pollution assessment in China. Environmental Pollution, 158(5), 1159–1168. https://doi.org/10.1016/j.envpol.2009.10.047
- Ouyang, W., Hao, F., Skidmore, A. K., & Toxopeus, A. G. (2010). Soil erosion and sediment yield and their relationships with vegetation cover in upper stream of the Yellow River. The Science of the Total Environment, 409(2), 396–403. https://doi.org/10.1016/j.scitotenv.2010.10.020
- Perona, E., Bonilla, I., & Mateo, P. (1999). Spatial and temporal changes in water quality in a Spanish river. The Science of the Total Environment, 241(1–3), 75–90. https://doi.org/10.1016/S0048-9697(99)00334-4
- Roberts, B. J., & Mulholland, P. J. (2007). In-stream biotic control on nutrient biogeochemistry in a forested stream, west fork of walker branch. Journal of Geophysical Research: Biogeosciences, 112(G4), 112(G4. https://doi.org/10.1029/2007JG000422
- Robson, B. J. (2014). State of the art in modelling of phosphorus in aquatic systems: Review, criticisms and commentary. Environmental Modelling & Software, 61, 339–359. https://doi.org/10.1016/j.envsoft.2014.01.012
- Rouf, A. J. M. A., Ambak, M. A., & Phang, S. -M. (2009). The floristic composition and ecology of periphytic diatoms from the man-made tropical lake Tasik Kenyir, in Malaysia. Aquatic Ecosystem Health & Management, 12(4), 364–374. https://doi.org/10.1080/14634980903355871
- Saraceno, J. F., Pellerin, B. A., Downing, B. D., Boss, E., Bachand, P. A. M., & Bergamaschi, B. A. (2009). High-frequency in situ optical measurements during a storm event: Assessing relationships between dissolved organic matter, sediment concentrations, and hydrologic processes. Journal of Geophysical Research, 114(G4). https://doi.org/10.1029/2009JG000989
- Sharpley, A. N., Kleinman, P. J. A., McDowell, R. W., Gitau, M., & Bryant, R. B. (2002). Modeling phosphorus transport in agricultural watersheds: Processes and possibilities. Journal of Soil and Water Conservation, 57(6), 425. Retrieved from. http://www.jswconline.org/content/57/6/425.abstract
- Shuhaimi-Othman, M., & Lim, E. C. (2006). Eutrophic condition at tasik Chini. Sains Malaysiana, 35(2), 29–34.
- Stutter, M. I., Langan, S. J., & Cooper, R. J. (2008). Spatial and temporal dynamics of stream water particulate and dissolved N, P and C forms along a catchment transect, NE Scotland. Journal of Hydrology, 350(3), 187–202. https://doi.org/10.1016/j.jhydrol.2007.10.048
- Syed Jamaludin, S. S., Zin, W., Deni, S., & Jemain, A. (2010). Trends in Peninsular Malaysia rainfall data during the southwest monsoon and northeast monsoon seasons: 1975-2004. Sains Malaysiana, 39(4), 533–542.
- Varadharajan, C., Appling, A. P., Arora, B., Christianson, D. S., Hendrix, V. C., Kumar, V., Lima, A. R., Müller, J., Oliver, S., Ombadi, M., Perciano, T., Sadler, J. M., Weierbach, H., Willard, J. D., Xu, Z., & Zwart, J. (2022). Can machine learning accelerate process understanding and decision-relevant predictions of river water quality? Hydrological Processes, 36(4), e14565. https://doi.org/10.1002/hyp.14565
- Wang, W., Liu, X., Wang, Y., Guo, X., & Lu, S. (2016). Analysis of point source pollution and water environmental quality variation trends in the Nansi Lake basin from 2002 to 2012. Environmental Science and Pollution Research, 23(5), 4886–4897. https://doi.org/10.1007/s11356-015-5625-x
- Woon Lee, J., Wan Yoon, S., Woong Chung, S., & Oh, D. G. (2010). Monitoring of non-point source pollutants load from a mixed forest land use. Journal of Environmental Sciences, 22(6), 801–805. https://doi.org/10.1016/S1001-0742(09)60180-7
- Yusoff, F. M., & Ambak, M. A. (1999). Trends and fluctuations in the environmental characteristics of surfacewaters in Kenyir Reservoir. In W. L. T. V. Densen & M. J. Morris (Eds.), Fish and fisheries of lakes and reservoirs in Southeast Asia and Africa (pp. 49–58). Westbury Academic & Scientific Publishing.
- Zampella, R. A., Procopio, N. A., Lathrop, R. G., & Dow, C. L. (2007). Relationship of land-use/land-cover patterns and surface-water quality in the Mullica River Basin. Journal of the American Water Resources Association, 43(3), 594–604. https://doi.org/10.1111/j.1752-1688.2007.00045.x
- Zhang, H., Hongfei, L., Gao, D., & Haoran, Y. (2022). Source identification of surface water pollution using multivariate statistics combined with physicochemical and socioeconomic parameters. The Science of the Total Environment, 806(3), 151274. https://doi.org/10.1016/j.scitotenv.2021.151274
- Zhang, Q., Xu, Z., Shen, Z., Li, S., & Wang, S. (2009). The Han River watershed management initiative for the South-to-North Water Transfer project (Middle Route) of China. Environmental Monitoring and Assessment, 148(1), 369–377. https://doi.org/10.1007/s10661-008-0167-z
- Zhou, B., Fu, X., Wu, B., He, J., Vogt, R. D., Yu, D., Yue, F., & Chai, M. (2021). Phosphorus Release from Sediments in a Raw Water Reservoir with Reduced Allochthonous Input. Water, 13(14), 1983. https://doi.org/10.3390/w13141983