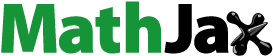
Abstract
Benin’s rapid population, economic, and industrial growth will increase energy demand. Hence, Benin’s government is keen to increase its power capacity to meet future demand. Renewable energy sources are significant options that governments could explore when developing their sustainable energy strategies. Prioritizing energy sources for a country’s sustainable development requires a decision-making process. In this study, multicriteria decision-making (MCDM) methods are used to prioritize alternatives such as solar photovoltaic (PV), concentrated solar power (CSP), wind energy, hydropower, and bioenergy suitable for sustainable electrification in Benin. The study employed eighteen (18) criteria classified under the four pillars of sustainability (technical, social, economic, and environmental) to evaluate the alternatives. Thereafter, the criteria importance through inter-criteria correlation (CRITIC) and entropy methods, which were employed to compute criteria weights and incorporated into the evaluation based on distance from average solution (EDAS) method to rank the alternatives. The findings from the EDAS rankings show that solar PV is the optimal alternative, ranking in the first position, followed by wind energy in the second position. CSP, hydropower, and bioenergy rank in the third, fourth, and fifth positions. In addition, EDAS findings correlated fully with those of other MCDM approaches evaluated on the same decision matrix. This indicates that solar PV is Benin’s optimal technology for sustainable electricity generation. The study’s findings are critical for policymakers developing Benin’s renewable energy sector.
1. Introduction
Energy is the foundation for industrialization and the key to development in every nation. Transforming the global energy sector plays a significant role in achieving the 1.5°C target of the Paris Agreement and in providing access to affordable, reliable, and sustainable energy services to communities (I. U. W. B. W. IEA, Citation2021; D. B. IRENA et al., Citation2021. Renewable energy sources (RES) are expanding beyond supplying reliable electricity and preserving the environment (Owusu et al., Citation2016). New jobs and prospects for whole industries are rising, helping economic development. At the same time, reliable, sustainable energy is essential for ensuring people access necessities like safe drinking water and healthcare (D. B. IRENA et al., Citation2021. It has therefore become necessary to cover the ever-increasing energy demand to achieve sustainable development (Güney, Citation2019).
Many countries still heavily rely on fossil fuels like coal and crude oil to meet their energy needs (IEA, Citation2019). However, the reliance on these finite resources comes at a high cost to our environment. Burning fossil fuels releases harmful greenhouse gases, contributing to global climate change and air pollution (IEA, Citation2019). Continuous large-scale exploitation of non-renewable energy sources creates grave risks to humans with rapid increases in the earth’s average temperature (Harjanne & Korhonen, Citation2019). One part of the solution is the removal of fossil fuel subsidies to curb wasteful energy consumption and utilize more reliable and environmentally friendly energy sources.
RES, comprising solar, wind, hydro, geothermal, tidal energy, and biomass, are natural energy sources that replenish at a higher rate than consumed (Al Garni et al., Citation2018; Yazdani et al., Citation2020). Renewable energy technologies are increasingly important for addressing the world’s energy challenges and mitigating the impact of climate change. RES is essential for developing and implementing various technologies in different sectors, such as transportation, the digital economy, agriculture, and public health (Eslami et al., Citation2022; Saberi-Beglar et al., Citation2023). According to the I. U. W. B. W. IEA (Citation2021), renewable energy consumption grew by 3% in 2020, while the need for other fuels decreased. This is due to the 7% growth in electricity production from RES. It is worth mentioning that the proportion of renewable energy in the world’s electricity generation mix jumped from 27% in 2019 to 29% in 2020 (IEA, Citation2021).
The use of RES has significantly increased in recent years but could not meet the overall rise in global energy demand (Hosseini, Citation2022). The intermittent nature of RES can pose challenges for grid integration. In view of this, these challenges can be addressed through the use of energy storage, backup power, and demand response programs (Golmohamadi, Citation2022; Javadi et al., Citation2022). Several techniques exist for integrating RES into the utility grid, making it more efficient and reliable (Aghdam et al., Citation2023; Ahmadi et al., Citation2022; Moafi et al., Citation2023). For example, new techniques such as artificial intelligence (Ahmad et al., Citation2022) and the Internet of Things (Mansouri et al., Citation2023) can significantly improve RES integration into the power grid, making it more efficient, reliable, and sustainable.
Benin, a West African country, is facing the challenge of providing sustainable electricity to its growing population. The country’s energy sector relies heavily on fossil fuels, making it vulnerable to price fluctuations and supply disruptions. Benin is among the West African nations that have targeted using RES to create jobs, enhance energy security, and boost the local economy. Currently, only 40% of the Beninese populace has access to electricity, and only 6% of rural and urban residents use clean cooking technologies (SEforALL, Citation2022; Stritzke et al., Citation2022). The government aspires to attain an electrification rate of 80% by 2025, with about 24.6% stemming from RES. This aims to boost its renewable energy production capacities, reduce the country’s long-standing energy shortage, and guarantee that its residents can access electricity by 2035 (MENSAH et al., Citation2022). However, implementing this target requires careful consideration of the available RES, their costs, and environmental impacts. Therefore, there is a need for a robust and effective multicriteria decision-making approach that can prioritize renewable energy resources for sustainable electricity generation in Benin.
Developing strategies to exploit RES, such as wind, solar, and hydropower, is essential for a sustainable environment (Hosseini, Citation2022; Razeghi et al., Citation2023). To address the issues of the energy-economy-environment nexus and achieve Sustainable Development Goals, several nations and institutions have adopted strategic plans that involve diversifying their energy mix and incorporating RES with other energy sources. Nearly all nations worldwide are currently promoting renewable energy programs, support policies, and targets (IEA, Citation2021). However, it is more challenging for decision-makers to select one specific RES over others when weighing all their advantages and disadvantages. As a result, choosing RES is a strategic and essential decision-making process for a nation based on various factors (Yazdani et al., Citation2020; Çelikbilek & Tüysüz, Citation2016).
Decision-makers must consider both qualitative and quantitative factors and environmental aspects to use RES safely, sustainably, and efficiently (Gülçin & Sezin, Citation2017). Relevant multicriteria decision-making (MCDM) methods have been developed to handle complicated decisions when selecting the most suitable RES in a particular country. MCDM methods are operations research-based models that address the decision-making problem when there are several competing objectives. For instance, conflicting criteria, incommensurable units, and challenges in designing and choosing alternatives are all aspects of these methods, which can handle both quantitative and qualitative criteria (Pohekar & Ramachandran, Citation2004).
MCDM applications enable handling complex issues with low requirements in energy planning (Dwivedi et al., Citation2023; F. Ali et al., Citation2022). It helps to ensure transparent and objective decision-making in selecting sustainable energy alternatives by providing a structured and rigorous process for evaluating alternatives based on multiple criteria and objectively weighing each criterion’s importance (Kaya et al., Citation2019). MCDM approaches are essential for selecting RES because they provide a systematic and structured way to evaluate and compare multiple criteria and objectives. Renewable energy alternatives often have different advantages and disadvantages that need to be considered, such as cost, environmental impact, reliability, and scalability. MCDM approaches allow decision-makers to weigh and prioritize these factors based on their relative importance, leading to more informed and rational decision-making.
MCDM methods, such as the analytic hierarchy process (AHP), Shannon entropy, best and worst (BWM), and decision-making trial and evaluation laboratory (DEMATEL), are used to determine the weight of criteria (Asadabadi et al., Citation2019; Diakoulaki et al., Citation1995; Torkayesh et al., Citation2020). Likewise, measurement of alternatives and ranking according to compromise solution (MARCOS), evaluation based on distance from average solution (EDAS), the technique for order preference by similarity to ideal solution (TOPSIS), and viekriterijumsko kompromisno rangiranje (VIKOR) are among the most useful MCDM methods in ranking alternatives (Amini et al., Citation2020; Torkayesh et al., Citation2020; Yazdani et al., Citation2020).
Several authors have used MCDM methods to evaluate RES for sustainable energy planning across the globe. For instance, Kabak and Daǧdeviren (Citation2014) applied the benefits, opportunities, costs, and risks (BOCR) approach and the analytic network process (ANP) to prioritize RES in Turkey. The result showed that hydropower is Turkey’s most appropriate RES. Çelikbilek and Tüysüz (Citation2016) proposed a grey-based approach that comprises DEMATEL, ANP, and VIKOR for evaluating RES. The authors found that MCDM methods are effective and applicable worldwide and are recommended for multicriteria evaluation problems. Georgiou et al. (Citation2015) used AHP and PROMETHEE methods to evaluate five alternative energy generation topologies for desalination in the Aegean Islands. The findings indicate that the region should consider diesel generation in the case of economic priorities. Similarly, Şengül et al. (Citation2015) used the fuzzy TOPSIS method to confirm the findings of (Kabak & Daǧdeviren, Citation2014), which show that hydropower is the best RES in Turkey for long-term energy planning.
Lee and Chang (Citation2018) integrated the weighted sum model (WSM), VIKOR, TOPSIS, elimination and choice translating reality (ELECTREE), and Shannon entropy methods to prioritize RES in Taiwan. The author found hydropower is the best option, followed by solar and wind power in Taiwan. Also, Tasri and Susilawati (Citation2014) applied the fuzzy analytic method to select the most appropriate RES in Indonesia. Based on several criteria, hydropower remains Indonesia’s most suitable renewable alternative, followed by geothermal, solar, wind, and biomass. Furthermore, Garni et al. (Citation2016) evaluated renewable power generation sources in Saudi Arabia using AHP. It was found that solar photovoltaic (PV) and concentrated solar power (CSP) are the most appropriate renewable energy technologies in Saudi Arabia, followed by wind power.
Other notable studies include San Cristóbal (Citation2011), who adopted AHP and VIKOR to evaluate RES in Spain. The authors found that a biomass power plant is the best choice for the country. Also, Yazdani et al. (Citation2020) proposed a hybrid of Shannon entropy-EDAS techniques and investigated its feasibility for Saudi Arabia. The findings revealed that wind power is the region’s most suitable renewable energy choice. In addition, Streimikiene et al. (Citation2020) employed MULTIMOORA and TOPSIS to prioritize sustainable electricity production technologies. The authors recommended future energy policies prioritizing sustainable energy technologies for power production while preserving the environment. Similarly, Büyüközkan and Güleryüz (Citation2016) integrated the DEMATEL-ANP approach to select optimal RES. The authors revealed that combining economic, social, political, and technical attributes could help practitioners and investors decide best when selecting RES.
Van Thanh (Citation2022) proposed a spherical fuzzy AHP and TOPSIS approach to select RES for Vietnam’s industrial complex. The authors found that solar energy is the best RES for the industrial sector, while wind energy is the worst alternative. Pathak et al. (Citation2022) applied AHP to prioritize RES in India. The study considered nine economic, technical, and environmental criteria. The findings revealed that hydro energy ranks first, followed by solar and wind energy. Qazi et al. (Citation2023) integrated entropy and TOPSIS to select the optimum RES alternative in Malaysia. Biomass was found to be the most appropriate RES for sustainable electricity generation. T. Ali et al. (Citation2019) employed Entropy and EDAS methods to evaluate Bangladesh’s best renewable energy technology. The results revealed that the solar-wind-battery hybrid energy system is the best option. Afrane et al. (Citation2021) adopted a fuzzy TOPSIS approach to select the best RES for electrification in Ghana. It was observed that hydropower is the best RES to satisfy Ghana’s energy needs, followed by solar, wind, and biomass. Asakereh et al. (Citation2022) applied a fuzzy AHP method to determine the optimal RES for electricity generation in Iran. The results indicate that solar PV should be the primary focus for generating sustainable electricity in the Khuzestan province of Iran.
The above studies show that MDCM methods are widely used to evaluate and rank RES worldwide. The main objective of this study is to rank alternatives, comprising solar PV, CSP, wind energy, bioenergy, and hydropower, appropriate for sustainable electricity generation in Benin. This study makes several novel contributions to the literature on RES and sustainable electricity generation in Benin. Firstly, it proposes a comprehensive and systematic approach for prioritizing RES that incorporates stakeholder preferences and uncertainty. Secondly, the study identifies a set of criteria relevant and specific to the Benin context, which have not been explored in previous studies. Thirdly, the study evaluates the potential of RES, considering its technical, economic, social, and environmental aspects.
The study combines entropy, CRITIC, and EDAS MCDM methods to evaluate the suitability of different RES for sustainable electricity generation in Benin. The study findings aim to inform stakeholders, decision-makers, and policymakers on which RES could be prioritized for sustainable development. Also, it informs policymakers to consider drafting policies that would attract potential investors to venture into deploying the best renewable energy technology for the country’s socio-economic development.
2. Methodology
This section highlights the approaches used to prioritize the alternatives. Also, the criteria used for evaluating RES are explained. Additionally, the numerical algorithms attributed to the selected MCDM methods are highlighted.
2.1. Selecting alternatives
Benin’s primary energy resources include bioenergy, fossil fuels, mineral energy resources, hydro, solar energy, and wind energy (SEforALL, Citation2022). In view of this, since this study is focused on sustainable energy resources for electricity generation, only RES are considered for evaluation. These RES can significantly reduce the gap between energy demand and supply in Benin and increase electricity generation installed capacity (Odou et al., Citation2020). For example, solar energy can offer electricity to remote communities and be utilized for residential and industrial purposes. Benin’s solar radiation ranges from 3.9 to 6.1 kWh/m2/day from the south to the north, with annual productivity ranging from 1680 to 2118 kWh/m2/year. Solar energy can be harnessed using photovoltaic (PV) and concentrated solar power (CSP) technologies. A solar PV module converts sunlight into electricity. Whiles CSP generates electricity through mirrors. The mirrors concentrate and focus natural sunlight, subsequently transforming into heat. The heat produces steam, which spins a turbine to generate electricity.
Hydropower is a very efficient technology that can provide electricity for domestic and industrial applications in any country. It’s a mature technology and the most crucial energy source in many countries. Benin’s theoretical hydropower potential is estimated at 749 MW. Wind power can also provide energy for different purposes. It is also a mature technology with a relatively low cost. The wind speed at 10 m varies between 3 and 6 m/s in the coastal area of Benin. Benin has a considerable amount of agricultural waste. However, the bioenergy potential is currently untapped and mostly burned in the fields. Using 27% of its national tailings, Benin’s biomass reserve can supply its electrical capacity (Sotiman Yotto et al., Citation2021).
2.2. Selection of evaluation criteria
Criteria are required to make objective decisions about adopting the most suitable alternative. Thus, well-considered and carefully constructed selection criteria can make selection decisions transparent and acceptable. The study adopted four criteria, comprising social, economic, technical, and environmental, categorized under the “pillars of sustainability,” to evaluate the performance of the alternatives. Several criteria for each pillar were identified and selected to compare the alternatives from a specific point of view. This is the MCDM approach’s most crucial phase, which should be chosen in collaboration with the decision-makers.
The quantitative and qualitative data and information pertaining to the prospective criteria must be available before deciding how many criteria to use. The exploitation of RES possibilities is multidisciplinary, and single-criteria decision-making systems cannot handle it. A comprehensive literature review was done to compile the most common criteria used in sustainable energy planning in other countries (Büyüközkan & Güleryüz, Citation2016; Ertay et al., Citation2013; Garni et al., Citation2016; Georgiou et al., Citation2015; Kabak & Daǧdeviren, Citation2014; Lee & Chang, Citation2018; San Cristóbal, Citation2011; Streimikiene et al., Citation2020; Tasri & Susilawati, Citation2014; Torkayesh et al., Citation2020; Troldborg et al., Citation2014; Yazdani et al., Citation2020; Çelikbilek & Tüysüz, Citation2016; Şengül et al., Citation2015).
Based on the comprehensive literature review, 18 different criteria were identified and chosen for assessment. Table presents the chosen criteria and their significant impact on decision-making. Table shows that when making a decision, maximum values are better for positive attributes, and minimum values are better for negative attributes. Table shows the data obtained for each criterion. The data were retrieved from reports published by notable international organizations such as the International Renewable Energy Agency (IRENA), the National Renewable Energy Laboratory (NREL), the Environmental Protection Agency (EPA), and scientific studies. Nevertheless, 10 experts were consulted to provide quantitative data for the criteria for which there was no available data in the literature. The experts were asked to provide data for the criterion based on a scale ranging from 1 to 5, where 1 is the worst score and 5 is the best. The profile of each expert is presented in Table .
Table 1. Decision-making processes criteria
Table 2. Profile of experts
2.3. Selecting the appropriate MCDM methods
Several MCDMs exist for evaluating alternatives. This study employed two weighting methods, entropy, and CRITIC, commonly used to compute criteria weights. The CRITIC and entropy approaches compute objective criteria weights when decision-makers think differently about the value of weights for criteria. These approaches prevent decision-makers from being biased when awarding weights to criteria compared to subjective methods such as BWM and AHP (Odoi-Yorke, Abofra, et al., Citation2022; Odoi-Yorke, Atepor, et al., Citation2022). The CRITIC and entropy weights are then integrated into the EDAS to rank the alternatives. EDAS was chosen because it is a newly developed MCDM that is resilient, evaluating alternatives with several contradictory criteria (Odoi-Yorke, Abofra, et al., Citation2022).
The study further applied other MCDMs, including TOPSIS, Combinative Distance-based Assessment (CODAS), Complex proportional assessment (COPRAS), Multi-Objective Optimization By Ratio Analysis (MOORA), and VIKOR, to the decision matrix to justify the findings from EDAS. The numerical computation steps for CRITIC, entropy, and EDAS are highlighted in Sections 2.3.1–2.3.3.
2.3.1. Shannon entropy method
The entropy algorithm estimates criteria objective weights using Eq. (1) - Eq. (3): (Yazdani et al., Citation2020); Odoi-Yorke, John, et al. (Citation2022)
Step 1: Normalize the decision matrix using Eq. (2):
where, is the normalized value, and
is the decision matrix value.
Step 2: Compute the criteria entropy values as follows:
Where,
is a constant based on 0≤Nj≤1,
is the entropy value, and a is the total alternatives.
Step 3: Using Eq. (3), calculate the criterion objective weight:
where Wj is the weight for each criterion Cj.
2.3.2. CRITIC weighting technique
CRITIC estimates objective weights using the following steps: (Odoi-Yorke, Abofra, et al., Citation2022; Odoi-Yorke, John, et al., Citation2022):
Step 1: Normalizes the decision matrix using Eq. (4):
Where are the decision matrix values;
are the best values in the decision matrix that exist in the column;
are the worst values in the decision matrix that exist in the column.
Step 2: Compute the standard deviation (Sj) value as follows:
where, P is population size, α is the mean population, and is the normalized decision matrix value.
Step 3: Measure the criteria degree of conflict using Eq. (6):
where, fjk is the symmetric matrix of n x n, which is the linear correlation coefficient between the vectors xj and xk
Step 5: Compute the criterion quantity of information Cj:
Step 6: Estimate each criterion weight Wj using Eq. (8):
2.3.3. EDAS method
The EDAS approach is based on the distance from the average solution, corresponding to the best alternative. This approach presents the positive distance from the average and the negative distance from the average as the first two metrics. These metrics can demonstrate how each alternative option differs from the average solution. EDAS ranks alternatives using the following steps (Odoi-Yorke et al., Citation2023; Yazdani et al., Citation2020):
Step 1: Find the criterion average solution using Eq. (9):
Where, are the decision matrix values, and a is the total criteria.
Step 2: Compute the positive distance from average (PAij), where Eq. (10a) is applied if the criterion type is positive and Eq. (10b) is used if the criterion type is negative:
Step 3: Estimate the negative distance from average (NAij) where Eq. (11a) is applied if the criterion type is positive and Eq. (11b) is used if the criterion type is non-negative.
Step 4: Calculate the weighted sum of PAij and NAij for the alternatives using Eqs. (12) and Eq. (13), respectively:
Where, is the weighted sum of PAij and
is the weighted sum of NAij.
Step 6: Normalize WSPi and WSNi values as follows:
Where LWPi and LWNi are the normalized values for WSPi and WSNi, respectively.
Step 7: Calculate alternatives performance score (Zi) using Eq. (16):
3.1. Criteria weights
As mentioned above, the criteria weights were computed using the CRITIC and entropy methods. Eq. (1) was used for the entropy method to normalize the decision matrix in Table . After that, the criteria entropy values were computed using Eq. (2), and the final weights for each criterion were estimated using Eq. (3). On the other hand, the CRITIC algorithm for computing criteria weights is different from entropy. The CRITIC algorithm normalizes the decision matrix (Table ) using Eq. (4). Eq. (5) was then used to calculate the criteria standard deviation. Also, the criterion quantity of information was evaluated using Eq. (6), and the criteria weights were finally estimated using Eq. (7). Different criteria influence the decision-making outcomes since each criterion’s weight affects the ranking. Despite different criteria, weights substantially determine which alternatives are preferred. The distribution of weight is based on the importance of the criteria. It considers the importance of each criterion in relation to the other criteria and is used to calculate the relative weights of the criteria for appropriate decision-making. A criterion with a higher weight greatly influences the decision-making process. Hence, estimating the weights of different criteria requires justifying the method employed. Figure presents criterion weights estimated by CRITIC and entropy methods. MCDM methods are dependent on criteria weights in ranking alternatives. It should be noted that the total weight of the criteria is 1. It can be seen in Figure that the top 5 criteria with the highest weights based on CRITIC methods are SC2 (8.5%), SC1 (6.7%), SC11 (6.4%), SC12 (6.3%), and SC5 (6.2%). Similarly, for the entropy method, the top 5 criteria with the highest weight comprise SC11 (21.4%), SC4 (19.2%), SC15 (15.2%), SC17 (9.7%), and SC3 (8.7%). This result attests that criterion weight varies among the weighting methods. However, weights determined by the CRITIC method are nearly consistent. For example, the highest and lowest weight difference is approximately 77%. In contrast, the difference between the lowest and highest weight is almost 198% for the entropy method. Furthermore, weights obtained by CRITIC and entropy have a correlation coefficient of rs = −0.086. This implies a very low negative correlation and an almost negligible relationship between the weights obtained by the two methods. To check the consistency of the criteria weight, the weights obtained from each method are compared to the no-priority approach, as shown in Figure . No-priority means that all criteria have the same weight. This was deduced by dividing the number of criteria (18) by 1. It can be observed that the weight obtained by the CRITIC method is nearly evenly distributed among the criteria compared to entropy. The CRITIC weights are roughly comparable to the no-priority weights. In fact, the highest weight obtained from the CRITIC method is just 46% higher than the no-priority weight of 0.056. Also, the lowest weight is about 33% lower than 0.056. That cannot be said for weights obtained by entropy methods. The highest weight is 116% higher than the no-priority weight of 0.056. Similarly, the lowest weight is about 190% lower than 0.056.
3.2. Alternative’s performance based on the EDAS method
The performance of the alternatives (renewable energy resources) is evaluated using the EDAS approach. With regards to the EDAS approach, the average solution was obtained using Eq. (9), and the results are presented in Table . Next, Eq. (10a) and Eq. (10b) are applied to estimate the alternatives’ positive distance from average (PA) with respect to each criterion (Table ). At the same time, Eq. (11a) and Eq. (11b) are used to compute the alternative negative distance from average (NA) for each alternative (Table ). Thus, greater PAij and lower NAij values will indicate the best option. Higher PAij and NAij values indicate that the alternative is better than the average solution. After that, the WSP and NSP for the alternatives were evaluated using Eq. (12) and Eq. (13), respectively, as shown in Table . Furthermore, Eq. (14) and Eq. (15) were applied to normalize WSP and WSN values to obtain LWP, and LWN values, which are also presented in Table . Finally, the alternative performance scores (Zi) were calculated using Eq. (16). The Zi ranks the alternatives. The alternative with a higher Zi is the best. It can be observed from Table that alternative ranking is similar when using weights obtained from the CRITIC and entropy weight approaches. However, the Zi for each approach differs due to different criterion weights. In light of this, the results show that solar PV technology, with the highest Zi, is the most promising and best alternative. Wind energy, CSP, and hydropower rank in the 2nd, 3rd, and 4th positions, respectively. As seen in Table , bioenergy technology is the worst technology, ranking in the 5th position. In summary, the rankings of the alternatives are as follows: solar PV > wind energy > CSP > hydropower > bioenergy. This result indicates that solar PV technology should be prioritized as Benin’s first sustainable electricity generation technology.
Table 3. Alternative’s performance based on the EDAS method
3.3. Comparative analysis
In order to check the performance of the proposed approach and validate EDAS rankings, other five MCDM approaches, including COPRAS-Complex Proportional Assessment (Amoozad Mahdiraji et al., Citation2018), CODAS-Combinative Distance-Based Assessment (Raheja et al., Citation2022), TOPSIS- Technique for Order Preference by Similarity to Ideal Solution (Chodha et al., Citation2022), MOORA- Multi-Objective Optimization on the Basis of Ratio Analysis (Odoi-Yorke, Abbey, et al., Citation2022), and VIKOR- Viekriterijumsko Kompromisno Rangiranje (Odoi-Yorke, John, et al., Citation2022) was tested on same decision matrix to assess and rank the alternatives. Applying multiple MCDM approaches to the same decision matrix boosts each method’s benefits because each has its limitations. Thus, multiple MCDM approaches might give better results than one. It can be observed in Figure that the positions of some alternatives are similar, while others are different in the MCDM approaches. This shows instabilities in the alternative ranks among the MCDM approaches. However, these variations could be attributed to the strengths and drawbacks of each MCDM technique. Figure shows the estimated correlation coefficient for alternative ranks obtained from other methods compared to EDAS. Correlation coefficients range from −1 to+1. Both parameters increase in a positive correlation. A negative correlation means one variable’s rank increases while the other decreases. It can be deduced from the figure that there is a perfect positive correlation between the ranks obtained from MOORA + entropy and COPRAS + entropy compared to EDAS. Also, VIKOR+entropy and TOPSIS+CRITIC exhibit a very high positive correlation (r = 0.908) and dependable relationship with EDAS. Likewise, the MOORA+CRITIC, COPRAS+CRITIC, and CODAS+entropy methods have r = 0.723. This implies a high positive correlation and a marked relationship with the EDAS approach.
On the other hand, there is a moderately negative and significant relationship between VIKOR+CRITIC and the EDAS approach. In addition, CODAS+CRITIC shows a very low negative correlation (r = −0.015). Based on this correlation test, it can be said that the correlation between EDAS rankings and the other MCDM is high, except for VIKOR+CRITIC (moderate correlation) and CODAS+CRITIC (low correlation). In view of this, it can be said that the proposed method is validated. Thus, the ranks obtained from EDAS could be accepted in prioritizing the alternatives for sustainable electricity generation. The correlation coefficient test has proven that the order of prioritizing the alternatives is as follows: solar PV (first position), wind energy (second position), CSP (third position), hydropower (fourth position), and bioenergy (fifth position). Therefore, solar PV technology is the most promising and sustainable technology. The findings are similar to those obtained for other Sub-Saharan countries, including Ghana (Afrane et al., Citation2021), Kenya (Manirambona et al., Citation2022), India (Pathak et al., Citation2022) and South Africa (Naicker & Thopil, Citation2019), where solar PV, wind energy, and CSP emerged as the top 3 alternatives for sustainable energy planning. However, it deviates from a similar study in China by Abdul et al. (Citation2022), where solar PV was considered the worst alternative for sustainable energy planning but wind energy emerged as the second optimal alternative.
Solar PV has been identified as a highly promising RES for Benin to invest in for electricity generation. Integrating solar PV into the existing energy mix in Benin can be achieved through a systematic approach. First, assessing the country’s current energy demand and supply is vital to determining the best solar PV installation locations. A feasibility study can follow this to evaluate the proposed solar PV project’s technical, financial, and environmental viability. Likewise, a comprehensive energy plan should be developed considering the existing energy infrastructure, transmission and distribution systems, and regulatory frameworks. This plan should also consider the potential impacts of solar PV integration on the grid, such as voltage stability, power quality, and reliability. Furthermore, policies and incentives can be put in place to encourage the adoption of solar PV by individuals, businesses, and industries. This can include feed-in tariffs, tax credits, and subsidies for solar PV installations.
Solar PV’s ranking as the most promising technology in Benin could be attributed to its maturity stage in the country’s electricity generation mix since the government and its stakeholders are making the necessary effort to utilize the PV technology for sustainable electrification. Recently, the government inaugurated its first utility-scale solar PV power plant. The plant has an installed capacity of 25 MW and can supply electricity to about 40,000 homes. Moreover, it would save about 23,000 metric tonnes of CO2 annually (Anyango, Citation2022). This shows that the government is committed to using PV technology to improve economic growth, provide quality electricity, and mitigate greenhouse gas emissions for sustainable development.
Furthermore, the results show that CSP and wind energy outshine conventional hydropower and bioenergy. Notwithstanding this, CSP and modern bioenergy technologies are still in the developmental stage in Benin, with no installed capacity in the country’s electricity generation mix. In light of this, extra effort is required to initiate the deployment of CSP technology, especially in the northern region where solar irradiation is around 6.1 kWh/m2/day. Likewise, a significant amount of electricity could be generated using bioenergy technologies such as direct combustion gasification, pyrolysis, and anaerobic digestion. Therefore, stakeholders may continue to embrace these technologies because they are strongly linked to sustainability.
Benin, like many other countries, can promote the development of bioenergy and maximize the use of agricultural waste for electricity generation through a combination of policies and strategies that are tailored to its specific context. Based on this, the following policies and strategies can be put in place to promote the development of bioenergy in Benin and maximize the use of agricultural waste for electricity generation, taking into consideration the economic, environmental, and social benefits: (i) Developing a comprehensive policy and regulatory framework that encourages private sector participation in the bioenergy sector. The policy should provide incentives for private investment, set clear standards for quality and sustainability, and establish a transparent system for the allocation of resources; (ii) Implement feed-in tariffs to provide a guaranteed price for electricity generated from bioenergy sources. This will help incentivize the private sector to invest and ensure a stable and predictable revenue stream; (iii) Invest in research and development to improve bioenergy production efficiency and identify new and innovative ways to use agricultural waste for electricity generation; (iv) Develop public-private partnerships to encourage the private sector to invest in bioenergy projects. This can involve partnering with local communities to establish small-scale bioenergy projects that provide electricity to remote areas (v) Develop capacity-building programs to train local communities in bioenergy production and build their technical skills. This can help create local jobs and improve the country’s overall economic development; (vi) Developing an awareness-raising campaign to educate the public about the benefits of bioenergy and encourage the adoption of sustainable practices in agriculture
4. Conclusion
This study has utilized MCDM methods comprising CRITIC, entropy, and EDAS to prioritize five RES (solar PV, CSP, bioenergy, hydropower, and wind energy). Eighteen (18) criteria that stem from technical, economic, social, and environmental indicators were used to evaluate the performance of RES for sustainable electricity generation. The study’s key findings can be summarised as follows:
Criterion weight varies among the weighting methods. Nevertheless, the CRITIC method produces nearly consistent weights. For example, the difference between the highest and lowest weights is around 77%. The difference between the lowest and highest weights is over 198% for the entropy technique.
The correlation coefficient between CRITIC and entropy weights is −0.086. This indicates a minimal relationship and a very low negative correlation between the weights obtained by the two approaches.
EDAS findings reveal that the order of priority of the renewable energy resources is as follows: solar PV > wind energy > CSP > hydropower > bioenergy. This indicates that solar PV is the most promising technology and should be considered first for sustainable energy planning. Likewise, bioenergy is the worst technology for electricity generation in Benin.
In addition, the EDAS ranking fully correlates with the ranks obtained from the other MCDM tested on the decision matrix. This result demonstrates that mixing qualitative and quantitative data improves MCDM performance by eliminating disparities and bias. Also, using many testing methods ensures precise results. The more relevant criteria chosen, the better the study’s outcomes.
With the low level of bioenergy development in Benin, it is suggested that the government develop favorable policy and regulatory frameworks that provide tax breaks and subsidies to encourage investment in bioenergy and establish regulations to set production and transportation standards and distribution. Investments are required to develop the necessary infrastructure, including processing plants and transmission lines, and to develop a public awareness plan on the benefits of bioenergy to increase support for sustainable development. Private-public participation in renewable energy technologies can become more cost-effective with the help of state support and infrastructure development. Also, funding for capacity building can boost the growth of solar and wind power in Benin. This study is vital for prioritizing RES and policy development in Benin and other developing countries willing to implement RES. Future research should increase the total criteria and apply new optimization algorithms to improve results.
Acknowledgments
The authors acknowledge Mr. John Eshun Davies for assisting them conduct desk literature review.
Disclosure statement
No potential conflict of interest was reported by the authors.
Additional information
Funding
References
- Abdul, D., Wenqi, J., & Tanveer, A. (2022). Prioritization of renewable energy source for electricity generation through AHP-VIKOR integrated methodology. Renewable Energy, 184, 1018–24. https://doi.org/10.1016/j.renene.2021.10.082
- Afrane, S., Ampah, J. D., Yusuf, A. A., Mensah, E. A., Oti, J. B., Asante, D., Dankwa, D. A., Engineering, A., Resources, N., & Carli, G. (2021). Prioritizing renewable energy sources for electrification in Ghana: A decision support framework using fuzzy theory. SSRN Electronic Journal. https://doi.org/10.2139/ssrn.3941689
- Aghdam, F. H., Mudiyanselage, M. W., Mohammadi-Ivatloo, B., & Marzband, M. (2023). Optimal scheduling of multi-energy type virtual energy storage system in reconfigurable distribution networks for congestion management. Applied Energy, 333, 120569. November 2022. https://doi.org/10.1016/j.apenergy.2022.120569
- Ahmad, F., Ashraf, I., Iqbal, A., Marzband, M., & Khan, I. (2022). A novel AI approach for optimal deployment of EV fast charging station and reliability analysis with solar based DGs in distribution network. Energy Reports, 8, 11646–11660. https://doi.org/10.1016/j.egyr.2022.09.058
- Ahmadi, S. E., Marzband, M., Ikpehai, A., & Abusorrah, A. (2022). Optimal stochastic scheduling of plug-in electric vehicles as mobile energy storage systems for resilience enhancement of multi-agent multi-energy networked microgrids. Journal of Energy Storage, 55(PB), 105566. https://doi.org/10.1016/j.est.2022.105566
- Al Garni, H. Z., Awasthi, A., & Ramli, M. A. M. (2018). Optimal design and analysis of grid-connected photovoltaic under different tracking systems using HOMER. Energy Conversion and Management, 155, 42–57. July 2017. https://doi.org/10.1016/j.enconman.2017.10.090
- Ali, F., Srisuwan, C., Techato, K., & Bennui, A. (2022). Assessment of small hydropower in Songkhla Lake Basin, Thailand using GIS-MCDM. Sustainable Water Resources Management, 9(1), 25. https://doi.org/10.1007/s40899-022-00788-w
- Ali, T., Ma, H., & Nahian, A. J. (2019). An analysis of the renewable energy technology selection in the southern region of Bangladesh using a hybrid multi-criteria decision making (MCDM) method. International Journal of Renewable Energy Research, 9(4), 1838–1848.
- Amini, M., Chang, S., & Malmir, B. (2020). A fuzzy MADM method for uncertain attributes using ranking distribution. Proceedings of the 2016 Industrial and Systems Engineering Research Conference, ISERC 2016, May, 759–764.
- Amoozad Mahdiraji, H., Arzaghi, S., Stauskis, G., & Zavadskas, E. K. (2018). A hybrid fuzzy BWM-COPRAS method for analyzing key factors of sustainable architecture. Sustainability, 10(5), 1626. https://doi.org/10.3390/su10051626
- Anyango, A. (2022). Benin inaugurates first large-scale solar photovoltaic power plant. https://pumps-africa.com/benin-inaugurates-first-large-scale-solar-photovoltaic-power-plant/
- Asadabadi, M. R., Chang, E., & Saberi, M. (2019). Are MCDM methods useful? A critical review of Analytic Hierarchy Process (AHP) and Analytic Network Process (ANP). Cogent Engineering, 6(1). https://doi.org/10.1080/23311916.2019.1623153
- Asakereh, A., Soleymani, M., & Safieddin Ardebili, S. M. (2022). Multi-criteria evaluation of renewable energy technologies for electricity generation: A case study in Khuzestan province, Iran. Sustainable Energy Technologies and Assessments, 52, 52. https://doi.org/10.1016/j.seta.2022.102220
- Büyüközkan, G., & Güleryüz, S. (2016). An integrated DEMATEL-ANP approach for renewable energy resources selection in Turkey $. International Journal of Production Economics, 182, 435–448. https://doi.org/10.1016/j.ijpe.2016.09.015
- Çelikbilek, Y., & Tüysüz, F. (2016). An integrated grey based multi-criteria decision making approach for the evaluation of renewable energy sources. Energy, 115, 1246–1258. https://doi.org/10.1016/j.energy.2016.09.091
- Center for Sustainable Systems, U. of M. (2022). Photovoltaic Energy Factsheet.: Vol. Pub. No. C.
- Chodha, V., Dubey, R., Kumar, R., Singh, S., & Kaur, S. (2022). Selection of industrial arc welding robot with TOPSIS and Entropy MCDM techniques. Materials Today: Proceedings, 50, 709–715. https://doi.org/10.1016/j.matpr.2021.04.487
- Diakoulaki, G., Mavrotas, D., & Papayannakis, L. (1995). Determining objective weights in multiple criteria problems: The critic method. Computers & Operations Research, 22(7), 763–770. https://doi.org/10.1016/0305-0548(94)00059-H
- Dwivedi, A., Goel, V., Kumar Pathak, S., & Kumar, A. (2023). Prioritization of potential barriers to the implementation of solar drying techniques using MCDM tools: A case study and mapping in INDIA. Solar Energy, 253, 199–218. https://doi.org/10.1016/j.solener.2023.02.030
- Ertay, T., Kahraman, C., & Kaya, I. (2013). Evaluation of renewable energy alternatives using MACBETH and fuzzy AHP multicriteria methods: The case of Turkey. Technological and Economic Development of Economy, 19(1), 38–62. https://doi.org/10.3846/20294913.2012.762950
- Eslami, S., Noorollahi, Y., Marzband, M., & Anvari-Moghaddam, A. (2022). District heating planning with focus on solar energy and heat pump using GIS and the supervised learning method: Case study of Gaziantep, Turkey. Energy Conversion and Management, 269(May), 116131. https://doi.org/10.1016/j.enconman.2022.116131
- Garni, H., Kassem, A., Awasthi, A., Komljenovic, D., & Al-Haddad, K. (2016). A multicriteria decision making approach for evaluating renewable power generation sources in Saudi Arabia. Sustainable Energy Technologies and Assessments, 16, 137–150. https://doi.org/10.1016/j.seta.2016.05.006
- Georgiou, D., Mohammed, E. S., & Rozakis, S. (2015). Multi-criteria decision making on the energy supply configuration of autonomous desalination units. Renewable Energy, 75, 459–467. https://doi.org/10.1016/j.renene.2014.09.036
- Golmohamadi, H. (2022). Demand-side management in industrial sector: A review of heavy industries. Renewable and Sustainable Energy Reviews, 156, 111963. https://doi.org/10.1016/j.rser.2021.111963
- Gülçin, B., & Sezin, G. (2017). Evaluation of renewable energy resources in turkey using an integrated MCDM approach with linguistic interval fuzzy preference relations. https://doi.org/10.1016/j.energy.2017.01.137
- Güney, T. (2019). Renewable energy, non-renewable energy and sustainable development. International Journal of Sustainable Development and World Ecology, 26(5), 389–397. https://doi.org/10.1080/13504509.2019.1595214
- Harjanne, A., & Korhonen, J. M. (2019). Abandoning the concept of renewable energy. Energy Policy, 127, 330–340. September 2018. https://doi.org/10.1016/j.enpol.2018.12.029
- Hosseini, S. E. (2022). Transition away from fossil fuels toward renewables: Lessons from Russia-Ukraine crisis. Future Energy, 1(1), 2–5. https://doi.org/10.55670/fpll.fuen.1.1.8
- IEA. (2019). World energy outlook 2017. International Energy Agency, 290.
- IEA. (2021). Global energy review, assessing the effects of economic recoveries on global energy demand and CO2 emissions in 2021. Global Energy Review. https://doi.org/10.1787/90c8c125-en.
- IEA, I. U. W. B. W. (2021). Tracking SDG7: The energy progress report. License: creative commons attribution—NonCommercial 3.0 IGO (CC BY- NC 3.0 IGO). World Bank.
- IRENA. (2018). Planification et perspectives pour les énergies renouvelables: Afrique de l’Ouest 2018.
- IRENA. (2022). Renewable power generation costs in 2021. International Renewable Energy Agency. https://www.irena.org/-/media/Files/IRENA/Agency/Publication/2018/Jan/IRENA_2017_Power_Costs_2018.pdf
- IRENA, D. B., Zusammenarbeit, D., & Für, G. (2021). The renewable energy transition in Africa powering access, resilience and prosperity on behalf of the.
- Javadi, M. S., Gough, M., Mansouri, S. A., Ahmarinejad, A., Nematbakhsh, E., Santos, S. F., & Catalão, J. P. S. (2022). A two-stage joint operation and planning model for sizing and siting of electrical energy storage devices considering demand response programs. International Journal of Electrical Power & Energy Systems, 138, 107912. https://doi.org/10.1016/j.ijepes.2021.107912
- Kabak, M., & Daǧdeviren, M. (2014). Prioritization of renewable energy sources for Turkey by using a hybrid MCDM methodology. Energy Conversion and Management, 79, 25–33. https://doi.org/10.1016/j.enconman.2013.11.036
- Kaya, İ., Çolak, M., & Terzi, F. (2019). A comprehensive review of fuzzy multi criteria decision making methodologies for energy policy making. Energy Strategy Reviews, 24, 207–228. https://doi.org/10.1016/j.esr.2019.03.003
- Lee, H. C., & Chang, C. T. (2018). Comparative analysis of MCDM methods for ranking renewable energy sources in Taiwan. Renewable and Sustainable Energy Reviews, 92(May), 883–896. https://doi.org/10.1016/j.rser.2018.05.007
- Manirambona, E., Talai, S. M., & Kimutai, S. K. (2022). Sustainability evaluation of power generation technologies using multi-criteria decision making: The Kenyan case. Energy Reports, 8, 14901–14914. https://doi.org/10.1016/j.egyr.2022.11.055
- Mansouri, S. A., Rezaee Jordehi, A., Marzband, M., Tostado-Véliz, M., Jurado, F., & Aguado, J. A. (2023). An IoT-enabled hierarchical decentralized framework for multi-energy microgrids market management in the presence of smart prosumers using a deep learning-based forecaster. Applied Energy, 333, 120560. November 2022. https://doi.org/10.1016/j.apenergy.2022.120560
- MENSAH, J. H. R., dos Santos, I. F. S., & Tiago Filho, G. L. (2022). A Critical analysis of the energy situation in the Benin Republic and its evolution in last decade. SSRN Electronic Journal, 55(35). https://doi.org/10.2139/ssrn.4074515
- Moafi, M., Ardeshiri, R. R., Mudiyanselage, M. W., Marzband, M., Abusorrah, A., Rawa, M., & Guerrero, J. M. (2023). Optimal coalition formation and maximum profit allocation for distributed energy resources in smart grids based on cooperative game theory. International Journal of Electrical Power & Energy Systems, 144, 108492. July 2022. https://doi.org/10.1016/j.ijepes.2022.108492
- Naicker, P., & Thopil, G. A. (2019). A framework for sustainable utility scale renewable energy selection in South Africa. Journal of Cleaner Production, 224, 637–650. https://doi.org/10.1016/j.jclepro.2019.03.257
- Odoi-Yorke, F., Abbey, A. A., Atepor, L., & Sarkodie, W. O. (2022). A Decision Support System for Evaluating Cooking Fuels for Sustainable Development in Ghana BT - Sustainable Education and Development – Making Cities and Human Settlements Inclusive, Safe, Resilient, and Sustainable J. N. Mojekwu, W. Thwala, C. Aigbavboa, E. Bamfo-Agyei, L. Atepor, & R. A. Oppong, (Eds.). Springer International Publishing. https://doi.org/10.1007/978-3-030-90973-4_38
- Odoi-Yorke, F., Abofra, N., & Kemausuor, F. (2022). Decision-making approach for evaluating suitable hybrid renewable energy system for SMEs in Ghana. International Journal of Ambient Energy, 43(1), 1–45. https://doi.org/10.1080/01430750.2022.2068068
- Odoi-Yorke, F., Atepor, L., & Abbey, A. A. (2022). A multicriteria decision making approach for evaluating crop residues for sustainable briquette production in Ghana. In J. N. Mojekwu, W. Thwala, C. Aigbavboa, E. Bamfo-Agyei, L. Atepor, & R. A. Oppong (Eds.), Sustainable education and development – making cities and human settlements inclusive, safe, resilient, and sustainable (pp. 193–205). Springer International Publishing. https://doi.org/10.1007/978-3-030-90973-4_16
- Odoi-Yorke, F., Frimpong, A. T., Ampimah, C. B., & Atepor, L. (2023). Techno-economic assessment of a utility-scale wind power plant in Ghana. Energy Conversion and Management, X, 100375. https://doi.org/10.1016/j.ecmx.2023.100375
- Odoi-Yorke, F., John, J., & Atepor, L. (2022). Composite decision-making algorithms for optimisation of hybrid renewable energy systems: Port of Takoradi as a case study. Energy Reports, 8(November), 2131–2150. https://doi.org/10.1016/j.egyr.2022.01.118
- Odou, O. D. T., Bhandari, R., & Adamou, R. (2020). Hybrid off-grid renewable power system for sustainable rural electrification in Benin. Renewable Energy, 145, 1266–1279. https://doi.org/10.1016/j.renene.2019.06.032
- Owusu, P. A., Asumadu-Sarkodie, S., & Dubey, S. (2016). A review of renewable energy sources, sustainability issues and climate change mitigation. Cogent Engineering, 3(1), 1167990. https://doi.org/10.1080/23311916.2016.1167990
- Pathak, S. K., Sharma, V., & Chougule, S. S. (2022). Prioritization of renewable energy Alternatives by Using Analytic Hierarchy Process (AHP) model: A case study of India. Lecture Notes in Mechanical Engineering, 103–118. https://doi.org/10.1007/978-981-16-7059-6_10
- Pohekar, S. D., & Ramachandran, M. (2004). Application of multi-criteria decision making to sustainable energy planning - a review. Renewable and Sustainable Energy Reviews, 8(4), 365–381. https://doi.org/10.1016/j.rser.2003.12.007
- Qazi, A., Bhowmik, C., Hussain, F., Yang, S., Naseem, U., Adebayo, A. -A., Gumaei, A., & Al-Rakhami, M. (2023). Analyzing the public opinion as a guide for renewable-energy status in Malaysia: A case study. IEEE Transactions on Engineering Management, 70(2), 371–385. https://doi.org/10.1109/TEM.2020.3046749
- Raheja, S., Obaidat, M. S., Kumar, M., Sadoun, B., & Bhushan, S. (2022). A hybrid MCDM framework and simulation analysis for the assessment of worst polluted cities. Simulation Modelling Practice and Theory, 118, 102540. https://doi.org/10.1016/j.simpat.2022.102540
- Razeghi, M., Hajinezhad, A., Naseri, A., Noorollahi, Y., & Moosavian, S. F. (2023). An overview of renewable energy technologies for the simultaneous production of high-performance power and heat. Future Energy, 2(2), 1–11. https://doi.org/10.55670/fpll.fuen.2.2.1
- Saberi-Beglar, K., Zare, K., Seyedi, H., Marzband, M., & Nojavan, S. (2023). Risk-embedded scheduling of a CCHP integrated with electric vehicle parking lot in a residential energy hub considering flexible thermal and electrical loads. Applied Energy, 329, 120265. November 2022. https://doi.org/10.1016/j.apenergy.2022.120265
- San Cristóbal, J. R. (2011). Multi-criteria decision-making in the selection of a renewable energy project in Spain: The Vikor method. Renewable Energy, 36(2), 498–502. https://doi.org/10.1016/j.renene.2010.07.031
- SEforALL. (2022). Benin SEforALL focal point. https://www.se4all-africa.org/seforall-in-africa/country-data/benin/
- Şengül, Ü., Eren, M., Eslamian Shiraz, S., Gezder, V., & Sengül, A. B. (2015). Fuzzy TOPSIS method for ranking renewable energy supply systems in Turkey. Renewable Energy, 75, 617–625. https://doi.org/10.1016/j.renene.2014.10.045
- Sotiman Yotto, H. C., Chetangny, P. K., Aredjodoun, J., Houndedako, S., Chamagne, D., Barbier, G., & Vianou, A. (2021). The renewable energy landscape in Benin: An analysis and review of barriers, targets, policies and actions for a clean energy transition. International Conference on Electrical, Computer, Communications and Mechatronics Engineering, ICECCME 2021, October, 7–8. https://doi.org/10.1109/ICECCME52200.2021.9590886
- Streimikiene, D., Balezentis, T., & Krisciukaitien, I. (2020). Prioritizing sustainable electricity production technologies: MCDM approach. Renewable and Sustainable Energy Reviews, 16(5), 3302–3311. https://doi.org/10.1016/j.rser.2012.02.067
- Stritzke, S., Leach, M., Leary, J., Rousseau, N., Batchelor, S., Brömling, G., Fehrenbach, S., Tchenga, V. G. E., & Andres, M. M. (2022). Benin eCooking Market Assessment (Issue February). https://mecs.org.uk/wp-content/uploads/2022/02/MECS-EnDev-Benin-eCooking-Market-Assessment-presentation.pdf
- Tasri, A., & Susilawati, A. (2014). Selection among renewable energy alternatives based on a fuzzy analytic hierarchy process in Indonesia. Sustainable Energy Technologies and Assessments, 7, 34–44. https://doi.org/10.1016/j.seta.2014.02.008
- Torkayesh, S. E., Amiri, A., Iranizad, A., & Torkayesh, A. E. (2020). Entropy based edas decision making model for neighborhood selection: A case study in Istanbul. Journal of Industrial Engineering and Decision Making, 1(1), 1–11. https://doi.org/10.31181/jiedm200101001t
- Troldborg, M., Heslop, S., & Hough, R. L. (2014). Assessing the sustainability of renewable energy technologies using multi-criteria analysis: Suitability of approach for national-scale assessments and associated uncertainties. Renewable and Sustainable Energy Reviews, 39, 1173–1184. https://doi.org/10.1016/j.rser.2014.07.160
- United States Environmental Protection Agency. (2013). Renewable energy fact sheet: Wind turbines. United States Environmental Protection Agency. August.
- Van Thanh, N. (2022). Sustainable energy source selection for industrial complex in Vietnam: A Fuzzy MCDM Approach. IEEE Access, 10, 50692–50701. https://doi.org/10.1109/ACCESS.2022.3173609
- Yazdani, M., Torkayesh, A. E., Santibanez-Gonzalez, E. D., & Otaghsara, S. K. (2020). Evaluation of renewable energy resources using integrated Shannon Entropy—EDAS model. Sustainable Operations and Computers, 1(December), 35–42. https://doi.org/10.1016/j.susoc.2020.12.002
Appendix A
Table A1. Decision matrix for MCDM methods
Table A2. Criteria average solution with respect to the alternatives
Table A3. Alternatives positive distance from average with respect to each criterion
Table A4. Alternatives negative distance from average with respect to each criterion