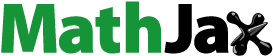
Abstract
The construction industry is the sector involved with the repair of buildings and civil engineering structures in an economy. Public building construction projects are constructed repeatedly, and also it becomes difficult to complete projects in the stated period and the estimated cost of the project. Thus, the study was focused on regression modeling for the prediction of earned value indexes in public building construction projects. The regression model was used as a data analysis method to increase the accuracy of the standard earned value analysis method for the evaluation of project performance. The regression model was built on ten sets of building projects database gathering between 2019 and 2022 through the case study method as a data collection instrument from cities, the Ministry of Construction and Housing, consultants, and contractors. The analysis method was made by using a statistical package for social science and Microsoft excels as an analysis tool. It was found that the regression model can predict earned value indexes with the excellent rank of accuracy for the correlation coefficient (R) of the cost performance index model, schedule performance index model, to complete cost performance index model, and to complete schedule performance index model was 88.80%, 95.90%, 96.60%, and 91.40%, respectively, and also for the coefficient of determination (R2) was 78.90%, 91.90%, 93.20%, and 83.40%, respectively. Finally, it can be recommended that the regression analysis method was conducted for its practicality in predicting earned value indexes.
PUBLIC INTEREST STATEMENT
The current study examined Predicting Earned Value Indexes in Public Building Construction Projects Using Regression Model. To achieve the objectives, a literature review was conducted to predict the earned value indexes through regression modeling to increase the accuracy of the standard earned value analysis results method for the evaluation of project performance.Furthermore, one of the fundamental aims of this study was to forecast the earned value indexes of public building construction projects. In this case study the projects were selected based on their data availability, and information. The findings of this study are thought to be helpful to provide a reference guide for the building construction company, academicians, and researchers, and for further exploration on the topic.
1. Introduction
The construction industry is the sector involved with the erection, repair, and demolition of buildings and civil engineering structures in an economy. It is one of the most dynamic and responsive sectors. It is also an important industry at both the global and national levels (Nyangwara & Datche, Citation2015). The construction industry has complexity in its nature because it contains a large number of parties as clients, contractors, consultants, stakeholders, shareholders, and regulators involved in the planning, execution, and evaluation of all types of civil works, such as buildings, roads, railways, water works, dams and hydropower construction (Bekr, Citation2017).
The construction industry is also one of the broad and important sectors of any country’s economy, and it is one of the main engines that governments resort to moving the economy and creating jobs, and reducing unemployment (Al-Zwainy, Citation2020).
Building construction projects are one of the physical infrastructures included in civil works and it needs appropriate planning organizing, monitoring, and controlling the whole process of the construction. Public building construction projects were constructed repeatedly; the projects faced time and cost deviation from the baseline plan (Pookot, Citation2017).
Earned value management system (EVMS) is a useful management technique available for tall buildings projects managers to monitor and control projects; it is a technique that combines the work scope, schedule, and cost elements of a project and facilitates the integrated reporting of a project’s progress and the cost status (Pookot, Citation2017). In Ethiopia, the Construction industry suffers from many problems and complex issues in performance because the industry is full of project activities, tasks, and constraints that require additional budget and cost. The performance and success of projects are measured by project cost, and time (Richard, Citation2001).
According to Aidan et al. (Citation2020), the amount of schedule slippage ranges between 61% and 80% and that of planned costs and other variables such as risk, quality, resources utilization, and safety deviates in the range of 21–40% from predetermined requirements, planned or anticipated at the beginning. Poor time and cost performance are major problems faced by today’s construction industry. According to Balali et al., public-sector construction projects are those projects financed, owned, and operated by a government for the benefit of the general public, such as infrastructure projects or public facilities.
Inaccurate estimation in building projects represents a major problem facing planners and estimators, especially when data and information about the projects are not available (Al-Zwainy, Citation2020). The current methods and techniques used to estimate earned value indexes in Ethiopia are weak and inefficient which is the traditional and standard earned value analysis. Therefore, there is a need to adopt new and advanced technologies to estimate earned value indexes that are fast, accurate and flexible to use that addresses the problem of inaccuracy of estimation arises.
A significant gap has been noticed that many public building construction projects have not been completed within the duration and cost planned and this affects the objectives of the project significantly in terms of cost, time, and quality. The using EVM will have a positive impact to mitigate that risk because the EVM will indicate the Deviation in the early stages of the project. Thus, conducting studies through regression models was interested in earned value management predicting public building construction projects, and an easy equation was developed to predict earned value indexes.
The researchers did not find any study that is the same as the current study while searching in international libraries and discreet periodicals such as Scopus, Springer, Taylor France, and others, as this current study is almost unique of its kind that deals with predicting the earned value indexes gained using regression modeling in public building construction projects.
Thus, depending on the stated problems prediction of earned value indexes in public building construction projects can be identified as the most important problem to be studied for improving the performance of building projects. The main objective of this study is to develop regression modeling for the prediction of earned value indexes in public building construction projects to reduce the percentage error of estimation.
This will facilitate the project practitioners to further use EVA for the schedule and cost control of their construction projects. To achieve this, there is a need to identify the factors that affect the performance of public building projects that can be available at early stages. Timely and targeted feedback at early stages can enable the project managers to identify problems early and make adjustments that can keep a project on time and within budget.
In this current study, this research provides a novel and extended contribution to previous research on the development of an advanced method known as the multiple regression models for the process of estimating earned value indexes. This method provides as to increase the accuracy of the standard EVA, and minimize the variance of the error.
Moreover, importance of this research paper is to provide a reference guide for the company in general to predict the project cost, and project time. The study provides a significant source of secondary data for future users to get knowledge, skills regarding project cost prediction, and time prediction by using the regression model. It can also serve to predict the earned value indexes at an early stage of public building construction projects to reduce the percentage error of estimation.
The remainder of this paper is organized as follows. Section 1 earned value management in construction, earned value management terminologies, performance indicators in earned value analysis, and regression analysis, and Section 2 presents the methodology it includes, data collection, multiple regression models, and identification of regression model variables. Section 3 numerical analysis and discussion of results. Section 4 presents managerial implications and limitations. Finally, general conclusions and remarks are then presented in Section 5.
2. Literature review
Salikuma et al. (Citation2016) stated that the construction industry plays an important role in the economy, and the activities of the industry are also vital to the achievement of national socio-economic development goals of providing shelter, infrastructure, and employment.
The construction industry is complex, and despite this complexity; the industry everywhere faces problems and challenges (Oke et al., Citation2016). Besides these challenges, the involvement of many stakeholders makes the management of cost and time difficult which consequently causes time and cost deviation. Therefore, cost and time overruns are considered one of the most critical issues during the execution of construction projects (Richard, Citation2001).
Salikuma et al. (Citation2016) noted that, the management practice in Ethiopia is even far behind those poor-performing developing countries in Africa. The level of construction project management practice in terms of adapting general project management procedures, project management functions, tools & techniques is unsatisfactory.
According to Richard (Citation2001), earned value is an improvement over traditional accounting progress measures. Traditional methods focus on planned expenditure and actual costs. With a clearer picture, managers can create risk mitigation plans based on the actual cost, schedule, and technical progress of the work. It is an early warning project management tool that enables managers to identify and control problems before they become insurmountable.
2.1. Earned value management in construction
According to Vyas & Birajdar (Citation2016), earned value analysis is a method of performance measurement in evaluating and forecasting construction projects. Earned value is a project control technique that provides cost and schedule performance measurements. It compares the actual accomplishment of scheduled work and associated costs against an integrated schedule and budget plan (Prasanth & Thirumalai Raja, Citation2007).
Richard (Citation2001) stated that earned value analysis allowed scope change management to keep the final budget of the project within check by providing alternatives to decide in what activities to reduce scope or reduce specifications to save money to fit cost overruns in other activities.
According to Salari et al. (Citation2014), earned value management is a powerful technique that allows program managers, project managers, and other top-level stakeholders to visualize the status of the project during the project life cycle. Earned value analysis provides the means for integrated management of schedule, progress, and cost based on majorly the three variables planned value, earned value, and actual costs, as well as the related indexes schedule performance index and cost performance index.
Salikumar & Anna Johny (Citation2016) stated that Earned value management is a project management methodology that integrates cost, time, scope, and resources to assess project performance and progress that requires a periodic measurement of cost and work performed. It is supported by a quantitative technique, earned value analysis (EVA), to evaluate the project’s performance and to forecast its final results through the monitoring of planned value, earned value, and actual cost (Almeida et al., Citation2021).
According to Aidan et al. (Citation2020), Earned Value Management (EVM) is defined as a methodology that combines scope, schedule, and resource measurements to assess project performance and progress.
Behl & Pal (Citation2020) define earned value management is a well-known approach in the project management context that can monitor schedule and cost performance indexes in projects simultaneously. Moslemi Naeni et al. (Citation2014) stated that it is a crucial technique in analyzing and controlling the performance of a project which allows a more accurate measurement of both the performance and the progress of a project.
2.2. Earned value management terminologies
EVM relies on three key data points which are planned value, earned value, and actual cost are the fundamental metrics of the earned value project management that generates variance, and performance indices for financial performance and schedules were defined as follows (Vyas & Birajdar, Citation2016).
Planned Value is the budgeted cost for the work scheduled to be completed on an activity or WBS component at a particular time. It is obtained from the cash flow diagram (The S-curve). It is also known as the Budgeted Cost of Work Performed (BCWP). The Planned Value is calculated before actually doing the work, which also serves as a baseline (Salari et al., Citation2014).
Earned Value is the budgeted amount for the work accomplished on the scheduled activity or work breakdown structure (WBS) component during a given time. It is also known as the Budgeted Cost of Work Performed (BCWP) (Jaber et al., Citation2020). It relates the original planned costs for the project or activity and the rate at which the team is completing work on the project or activity to date (See Figure ).
Figure 1. Earned Value Analysis curve (Buyse & Vandenbussche, Citation2010).

Actual Cost: according to Suresh & Ramasamy (Citation2015), actual cost is defined as the cost incurred while performing the activity. It is resolved from accounting records that keep a record of actual expenditure money, which means it is secret and actual money spent by the contractor. It is also known as the Actual Cost of Work Performed (ACWP), which is an indication of the level of resources that have been expended to achieve the actual work performed in a given period (Jaber et al., Citation2020).
Budget at Completion: this is the total approved budget when the scope of the project is completed. The project budget must reflect all planned costs incurred by the activities of which the project consists. It is the baseline cost that shows the planned cost for a task, a resource for all assigned tasks, or for work to be performed by a resource on a task (Buyse & Vandenbussche, Citation2010).
2.3. Derived performance indicators in earned value analysis
Performance indicators give the PMs an early warning signal that lets them know that corrective actions need to be implemented (Kuhl & Graciano, Citation2015). Earned value analysis involves calculating variances and indices related to the project cost and project schedule were explored as follows.
Schedule Variance (SV): a degree of schedule performance of a project, which is the difference between the planned value of the work scheduled and the value of the work accomplished for the same time phase. It indicates objectively how much the project is ahead or behind its baseline schedule (Shenoy, Citation2015).
A negative schedule variance indicates that the project is behind schedule and a positive schedule variance indicates that the project is ahead of schedule.
Cost Variance (CV): It is defined as the cost performance of a project, which is the difference between the values of the work accomplished and the actual cost incurred to perform the work, and utilizing this parameter the percentage of cost overrun or underrun can be calculated (Shenoy, Citation2015).
A negative cost variance means the project is over budget and a positive cost variance means the project is under budget.
Schedule Performance Index (SPI) is an index showing the efficiency of the time utilized on the project. It is used to predict the project’s completion time and forecast the project’s estimate at completion (EAC). SPI is defined as the ratio of EV to PV. If the value of SPI is below 1.0, it indicates that efficiency in utilizing the time allocated to the project is poor. That means the project is behind schedule. If SPI is equal to 1.0 then the project would be completed on time. When SPI is above 1.0, efficiency in utilizing the time allocated to the project is good and it shows the project is ahead of schedule (Chandrasekaran & Kumar, Citation2012).
The Cost Performance Index (CPI) is an index showing the efficiency of the utilization of the resources allocated to the project. It is used to track the project cost and predict cost overruns. It is commonly used to track cost efficiency. CPI is simply the ratio of EV to AV.
The value of CPI below 1.0 indicates that the project’s cost is over the planned cost for the work performed and efficiency in utilizing the resources allocated to the project is poor. If CPI is equal to 1, then the project would be completed at the planned cost. When CPI is above 1.0, the project’s cost is the under-planned cost for the work performed and efficiency in utilizing the resources allocated to the project is good (Chandrasekaran & Kumar, Citation2012).
Time Variance (TV): defines the amount of time the project is ahead or behind by translating the scheduled variance to time units (Kuhl & Graciano, Citation2015).
With the major components of earned value performance measures defined, one can examine the various scenarios that exist. With an unchanging baseline, some scenarios exist for a given project as illustrated in Figure . By referencing this figure, a quick determination can be made as to the budget and schedule status.
Figure 2. SPI and CPI Analysis Matrix of a Project (Suresh & Ramasamy, Citation2015).

2.4. Forecasting indicators of earned value management
Forecasting indicators predict the project’s time and cost at completion based on actual performance achieved up to a specific time of the project (Eirgash, Citation2019). During the development stage of the project, the future cost and schedule performance can be forecasted. Common forecasting indicators were discussed as follows (Shenoy, Citation2015).
2.4.1. To complete the cost performance index (TCCPI)
The TCPI is a comparative measure. It compares work completed to date with the budget required to complete the remaining work. The TCPI data can be used as the basis for a discussion that explores whether the performance required is realistically achievable. It is the ratio of remaining work to the remaining budget (Suresh & Ramasamy, Citation2015).
To complete cost performance index: Indicates the level of cost performance that will be necessary to complete the project within budget from the report point. Like, the TCSPI is also not accurate. The reason is that, when the CPI is less than one, which indicates an unfavorable performance, the TCCPI on the other hand becomes greater than one, which is not reflective of a typical index (See Table ).
Table 1. Entered/Removed Variables for the CPI Regression model. Source: own work, 2022
The formula for deriving the TCCPI is as follows:
2.4.2. To complete the schedule performance index (TCSPI)
To Complete Schedule Performance Index, is a forecasting metric showing the efficiency at which the remaining time on the project should be utilized. This can be calculated using the following formula.
The formula mentioned above gives the efficiency at which the resources should be utilized in the “remaining time” allocated for the project.
TCSPI value of less than one indicates project team can be lenient in utilizing the remaining time allocated to the project.
TCSPI value greater than one indicates project team needs to work harder in utilizing the remaining time allocated to the project.
2.5. Regression analysis
In statistics and data analysis, it needs to establish a relationship between the various parameters in a data set for prediction and analysis. It involves fitting the right model concerning the given data set and then using that model to make further predictions (Gupta et al., Citation2017). According to Mooi (Citation2016) model of the relationship is hypothesized and estimates of the parameter values are used to develop an estimated regression equation. Various tests are then employed to determine if the model is satisfactory. If the model is deemed satisfactory, the estimated regression equation can be used to predict the value of the dependent variable given values for the independent variables (See Table ).
Table 2. Model Summary. Source: own work, 2022
2.5.1. Linear regression
Linear regression explores relationships that can be readily described by straight lines or their generalization to many dimensions. Linear regression assumed that there’s a linear relationship between variables (Boateng & Abaye, Citation2019). There are two kinds of statistical regression; simple linear regression and multi-linear regressions. When there’s quite one variable quantity multi-statistical regression is employed.
Simple Regression Model a linear association between the two variables when there is a single continuous dependent variable and a single independent variable, the analysis is called a simple linear regression analysis (Aidan et al., Citation2020).
Multiple Regressions Model: is the relationship between several independent or predictor variables and a dependent or criterion variable and it was defined as the following (Marco, Citation2022).
Independent variables: are characteristics that can be measured directly; these variables are also called predictor or explanatory variables used to predict or explain the behavior of the dependent variable (Narbaev, Citation2014).
Dependent variable: a character whose value depends on the values of independent variables (Narbaev, Citation2014).
Multiple Regression Analysis refers to a set of techniques for studying the straight-line relationships among two or more variables. Multiple regression estimates the β’s in the equation (Marco, Citation2022).
The Xs are the independent variables (IVs). Y is the dependent variable. The subscript j represents the observation (row) number. The βs are the unknown regression coefficients. Their estimates are represented by bs. Each β represents the original unknown (population) parameter, while b is an estimate of this β. The εj is the error (residual) of observation j.
3. Methodology
The study can employ a case study research approach conducted on an earned value data set comprising of ten real life projects for a total of one hundred fifty-four observations to obtain information for the objective of the study by collecting schedule and cost data of public building construction projects in Ethiopia. So the prediction model can be developed and conclusions can be made regarding the projects. The methodology of the current study was carried out according to the research aim, and the method for doing this paper is shown in a flow chart as follows (See Figure ).
Figure 3. Steps to conduct a regression analysis (Mooi, Citation2016).

3.1. Data collection
The data collection method used in this study is based on direct and indirect historical data from ten sets of public building construction company documents collected which were done between 2019 and 2022 from cities, the Ministry of Construction and Housing, consultants, and contractors. Subsequently, the data has been analyzed. Besides the company information on project cost, and project time review of the literature related to earned value management systems with forecasting techniques such as regression modeling was used as a tool for prediction and optimization in different project management knowledge areas. Thus, the previous studies are among the most important scientific foundations on which the current study is based and the study employs historic data analysis as the methodology foundation.
Additionally, using historical data helps in giving a relation among the key factors influencing the earned value parameters of the public buildings projects to create estimations for new projects used as a validation purpose.
3.2. Multiple regression model
Multiple regression models is adopted in the study because multiple linear regressions are the most widely utilized kind and employing the construction project features in a mathematical model to forecasting earned value indices.
Tables shows the results of the statistical analysis. The research used Enter method as a type of regression analysis. All requested (factors) variables were entered as shown in Table .
Table 3. Statistical significance of the model. Source: own work, 2022
Table 4. Statistical significance of the independent variables. Source: own work, 2022
Table 5. Entered/Removed Variables for the SPI Regression model. Source: own work, 2022
Table 6. Model Summary. Source: own work, 2022
Table 7. Statistical significance of the model. Source: own work, 2022
Table 8. Statistical significance of the independent variables. Source: own work, 2022
Table 9. Entered/Removed Variables for the TCCPI Regression model. Source: own work, 2022
Table 10. Model Summary. Source: own work, 2022
Table 11. Statistical significance of the model. Source: own work, 2022
Table 12. Statistical significance of the independent variables. Source: own work, 2022
Table 13. Entered/Removed Variables for the TSPI Regression model. Source: own work, 2022
Table 14. Model Summary. Source: own work, 2022
Table 15. Statistical significance of the model. Source: own work, 2022
Table 16. Statistical significance of the independent variables. Source: own work, 2022
The results of the entered variables were analyzed by using SPSS software version 23. SPSS is a statistical package for the social science version.
The correlation among input variables is tasted symbol (R), titled a linear correlation coefficient, calculating the direction and strength of the linear relationship between the dependent and independent variables. It can be considered to be one measure of the quality of the prediction of the dependent variable; in this case, it measures the quality of the cost performance index.
Also, symbol (R2) called the coefficient of determination exemplifies the amount of data that is close to the line of best fit as shown in Tables , Tables . The R2 value represents the proportion of variance in the dependent variable described by the independent variables. R-squared values range from 0 to 1 and are commonly stated as percentages from 0% to 100%. An R-squared of 100% means that all the variance of the dependent variable being studied is completely explained by the variance of the independent variable (Al-Zwainy, Citation2020).
An R-squared, between 85% and 100%, would generally be seen as showing a high level of correlation, whereas a low R-squared measure, at 70% or less, would show a low correlation. This is not a hard rule, however, and will depend on the specific analysis (Narbaev, Citation2014). R-Squared only works as intended in a simple linear regression model with one explanatory variable. With a multiple regression made up of several independent variables, the R-Squared must be adjusted.
The adjusted R-squared compares the descriptive power of regression models that include diverse numbers of predictors. If the adjusted R Square value is much lower than the R Square value, it is an indication that the regression equation may be over-fitted to the sample, and of limited generalize ability.
3.3. Identification of regression model variables
There are two types of variables that affected on the earned value indices that adopted in the regression model in public building projects are Dependent variables and Independent variables (Huang & Ho, Citation2013).
3.3.1. Dependent variables
The dependent variable is the variable that changes as a result of the independent variable manipulation (Chai & Draxler, Citation2014). In this study, the dependent variables were Cost Performance Index (CPI), Schedule Performance Index (SPI), and To Complete Cost Performance Indicator (TCPI), and To Complete Schedule Performance Index (TSPI) have been defined as the dependent variable, and every specific engineering project has employed as the elementary unit of the observation.
3.3.2. Independent variables
After starting the dependent variables that are projected via the regression model, it has been essential for developing independent variables to clarify any variant in earned value indexes. In this study, to predict the earned value indexes, four mathematical models have been established in this section through SPSS version 23 was used as a tool and technique for building the four models. In this study, the independent variables were Planned Value (PV), Earned Value (EV), Actual Cost (AC), and Budget at Completion (BAC) was identified as the independent variable.
3.4. Predicting earned value indexes by using a multiple regression model
The correlation between dependent and independent variables can be determined using the multiple regression method (Balali et al.,). There are four methods to enter input data into the model. These methods are the entering method (direct method), backward method, forward method, and step-wise method (Aidan et al., Citation2020). In this study, the direct entering method was selected to be exploited. The linear relationship among the variables is illustrated below in EquationEquation 8(8)
(8) :
where p is the number of predictions, bj is the value of the jth coefficient, Xij is the ith value of jth predictions, and ei is the error of the ith value.
Furthermore, the matrix form of the model is presented as Equationequation (9)(9)
(9) below.
where β is the vector of regression coefficients, is the matrix of fitting errors, Y is the vector of the dependent variable, and X is the matrix of the independent variables.
To determine and rank factors affecting the earned value of the studied projects, outputs of SPSS analyses were used. Variables with a significance of less than 0.05 were selected as effective factors. Furthermore, according to their significance value, variables were prioritized.
4. Case study: Numerical data analysis
4.1. Development of regression model for predicting earned value indexes
The development of mathematical models is divided into four parts, and a detailed description of these stages is described in the following section. Input variables have the most important influence on the model performance as the significant step in evolving regression models. The study is conducted on an EVM data set to determine the prediction of the earned value indices by multiple regression analysis to increase the accuracy of the standard earned value method of evaluation of performance and minimize the variance of the error.
In this study, four different performance indices predictor models were developed based on the historical data obtained from ten projects regarding the cost and time performance information of the projects. Even though, this study used seven real ongoing buildings projects were used for developing the regression model and it can take three new sets of projects for checking the validity of the developed regression model. This is the researchers stated that 75% of the data used for the model preparation and the remaining data that was 25% of the data was used for checking the validity of the proposed model. In carrying out this study, a total of one hundred fifty-four reported data points were recorded from the projects.
Four mathematical models have been established in this section, and the features of the project in a mathematical model were applied to estimate earned value indexes. SPSS version 23 was utilized as a tool to build up the four models, as follows:
4.1.1. First: Cost performance index regression model
In this study, the correlation coefficient (R) is equal to 88.8%, which indicates a good level of prediction. Similarly, the coefficient of determination (R2) equals 78.9%. These results indicate a positive and excellent relationship and a high correlation between the dependent variable and the independent variable. The value of R2 is 0.789, this means the independent variables involved in the model explained 78.9% of the variability of the dependent variable cost performance index variable, and 21.1% (100–78.9%) of the variation is caused by factors other than the predictors included in this model. For a precise description of the data interpretation, adjusted R2 is another important factor. The value of 0.783 indicates true 78.3% of the variation in the outcome variable is explained by the predictors in the model. The standard error of a model fit is a measure of the precision of the model. It shows how wrong one could be used the regression model to make predictions or to estimate the dependent variable or variable of interest. In this estimate of the cost performance index, the model will be wrong by 0.037 which is a small amount. The regression statistics of the Cost Performance Index Model (CPI-Model) are set in Table .
Table shows that the F-ratio tests whether the overall regression model is a good fit for the data and also shows that the independent variables statistically significantly predict the dependent variable, F (4, 149) = 139.176, and p (0.000) < 0.05, This indicates that the regression model is a good fit of the data.
As it was observed in Table that the value of p < 0.05, thus, the coefficient is statistically significant. In this regression model of the cost performance index, the significance values of all independent variables are 0.00. This indicates that all variables are statistically significant and already appear in the model. The standard error of the coefficients in regression output is also required to be as small as possible. It reflects how wrong could be while estimating its value. For instance, in this model relative to the coefficient of the variables, their standard error is 0.000. This indicates that the coefficient of the standard error of the estimate is small.
The results of linear regression showed that the predictors had significant regression values less than 0.15. Therefore, the predictors were entered into the multiple regression analysis to predict cost performance index regression model. The prediction formula was generated by multiple regression analysis with information provided in the coefficients Table . The significance value of the variable in Table was 0.000, which indicated that it was making a significant contribution to the prediction of CPI.
Statistics of regression for model CPI are set in Table , which can be formed as EquationEquation (10)(10)
(10) below:
Standardized coefficients are called beta weights that rank the predictor variables based on their contribution (irrespective of sign) in explaining the outcome variable. In this model, EV is the highest contributing (3.984) predictor to explain CPI and the next highest predictor is BAC (0.548).
The histogram shows that the residuals are normally distributed. An asymmetric bell-shaped histogram which is evenly distributed around zero indicates the normality assumption (See Figure ).
4.2. Second: Schedule performance index regression model
The value in Table shows that the model degree of predicting the dependent variable was found to be a correlation coefficient equal to 0.959. This indicates that the model has a good level of prediction.
Similarly, the model degree of explaining the variance in the dependent variable coefficient of determination (R2) was 91.9%. Looking at the results of the correlation coefficient, and coefficient of determination, it may be said that the model predicts the dependent variable very well. This shows that the independent variables involved in the model (BAC, EV, PV, and AC) explain 91.9% of the variability of the dependent variable schedule performance index, and 8.1% (100%-91.9%) of the variation is caused by factors other than the predictors included in this model.
Adjusted R Square (adj. R2) is another important factor. In this model, the value of adjusted R2 is 0.917. This indicates that 91.7% of the variation in the outcome variable is truly explained by the predictors which are to keep in the model. The standard error of a model fit is a measure of the precision of the model. It shows how wrong one could be used the regression model to make predictions or to estimate the dependent variable or variable of interest. In this estimate of the schedule performance index, the standard error of the estimate was 0.046. Hence it is a small amount.
Table shows that the F-ratio tests whether the overall regression model is a good fit for the data. Thus, the independent variables statistically significantly predict the dependent variable, F (4, 149) = 421.549, p (0.000) < 0.05, this indicates that the regression model is a good fit for the data.
As was observed in Table that the value of p < 0.05, the coefficients are statistically significant. The test results tell as all independent variables are statistically significant for estimating the regression model of the schedule performance index. Since the significance value of the variables is 0.000 < 0.05. This shows that the explanatory variables were useful in the model. The standard errors of the coefficients in regression output are also required to be as small as possible. It reflects how wrong could be while estimating its value, and the value of standard error is 0.000 in this model.
The results of linear regression showed that the unstandardized coefficients of the standard error of the predictors had significant regression values less than 0.15. Therefore, the predictors were entered into the multiple regression analysis to predict the schedule performance index regression model. The prediction formula was generated by multiple regression analysis with information provided in Table . The significance value of the variable in Table was 0.000, which indicated that it was making a significant contribution to the prediction of SPI.
Statistics of regression for model SPI are set in Table , which can be formed as EquationEquation (11)(11)
(11) below:
Standardized coefficients are called beta weights that rank the predictor variables based on their contribution (irrespective of sign) in explaining the outcome variable (See Figure ). In this model, EV is the highest contributing (2.046) predictor to explain SPI and the next higher predictor is AC (1.816).
The histogram shows that the residuals are normally distributed. An asymmetric bell-shaped histogram which is evenly distributed around zero indicates the normality assumption.
4.3. Third: To complete the cost performance index regression model
The value in Table shows that the correlation coefficient value for the To complete cost performance index model was equal to 0.966, which indicates a good level of prediction. Similarly, the coefficient of determination (R2) was 0.932. This indicates that the independent variables involved in the model explain 93.20% of the variability of the dependent variable (To complete performance index variable), and 6.8% (100%-93.2%) of the variation is caused by factors other than the predictors included in this model.
To accurately report the data interpretation of Adjusted R Square (adj. R2) is another important factor. A value of 0.930 indicates that 93.0% of the variation in the outcome variable is best explained by the predictors which are to keep in the model. The standard error of a model fit is a measure of the precision of the model. It shows how wrong one could be used the regression model to make predictions or to estimate the dependent variable or variable of interest. In this estimate of To complete cost performance index, the standard error of the estimate would be 0.046.
Table shows the F-ratio tests whether the overall regression model is a good fit for the data. The table shows that the independent variables statistically significantly predict the dependent variable, F (4, 149) = 512.813 and the value of p (0.000) is < 0.05. This indicates that the regression model is a good fit for the data.
As it was observed in Table that the value of p < 0.05, the coefficients are statistically significant. In this regression model of to complete cost performance index, based on the results obtained from Table , the significance level of the independent variable was good, so it adds a substantial contribution to explaining to complete cost performance index. The standard error of the coefficients in regression output is also required to be the best. It reflects how it is good at estimating its value. According to the analysis made in Table , its standard error of estimating is 0.000.
The results of linear regression showed that the unstandardized coefficients of the standard error of the predictors had significant regression values less than 0.15. Therefore, the predictors were entered into the multiple regression analysis to predict the to complete cost performance index regression model. The prediction formula was generated by multiple regression analysis with information provided in Table . The significance value of the variable in Table was 0.000, which indicated that it was making a significant contribution to the prediction of TCCPI (See Figure ).
Statistics of regression for model TCCPI are set in Table , which can be formed as EquationEquation (12)(12)
(12) below:
Standardized coefficients are called beta weights that rank the predictor variables based on their contribution (irrespective of sign) in explaining the outcome variable. In this model, AC is the highest contributing (1.486) predictor to explain TCPI and the next higher predictor is PV (0.870).
The histogram shows that the residuals are normally distributed. An asymmetric bell-shaped histogram that is evenly distributed around zero indicates the normality assumption.
4.4. Fourth: To complete the schedule performance index regression model
In this study, the correlation coefficient (R) is equal to 91.40% which indicates a good level of prediction. Similarly, the coefficient of determination (R2) equals 83.40%. These results indicate a positive and excellent relationship and a high correlation between a dependent variable and an independent variable. The value of R2 is 0.834, this indicates that the independent variables involved in the model were 83.40% of the variability of the dependent variable to complete schedule performance index variable, and 16.6% (100–83.4%) of the variation is caused by factors other than the predictors included in this model. To accurately report the data interpretation of Adjusted R Square (adj. R2) is another important factor. A value of 0.83 indicates true 83% of the variation in the outcome variable is explained by the predictors which are to keep in the model.
The standard error of a model shows how wrong one could be used the regression model to make predictions or to estimate the dependent variable or variable of interest. In this estimate of to complete schedule performance index, the model will be wrong by 0.038, which is small in amount.
Table shows that the F-ratio tests whether the overall regression model is a good fit for the data, and also the independent variables statistically significantly predict the dependent variable, F (4, 149) = 187.809, p (0.000) <0.05, this indicates that the regression model is a good fit of the data.
As it was observed in Table that the value of p < 0.05, the coefficients are statistically significant. In this regression model of to complete schedule performance index, the tests tell Earned value (EV) is not statistically significant. Since the significance value of earned value is 0.907 > 0.05. This shows that the explanatory variable earned value is no more useful in estimating the model when the three variables are already used in the model. This indicates that EV no more adds a substantial contribution to explaining to complete schedule performance index. The standard errors of the coefficients in regression output are also required to be as small as possible. Its standard error is .000.
The results of linear regression showed that the earned value had not significant contribution for the prediction of TCSPI. Therefore, the prediction formula was generated by multiple regression analysis using planned value, actual cost, and budget at compilation as predictors. Statistics of regression for the model to complete the schedule performance index are set in Table , which can be formed as Equation (13):
Standardized coefficients are called beta weights that rank the predictor variables based on their contribution (irrespective of sign) in explaining the outcome variable. In this model, PV is the highest contributing (5.734) predictor to explain TSPI (See Figure ).
The histogram shows that the residuals are normally distributed. An asymmetric bell-shaped histogram which is evenly distributed around zero indicates the normality assumption.
5. Discussions, managerial implications, and limitations
5.1. Discussion of results
In this study, the regression model is essentially helps for the performance measures, the robustness and flexibility of the model provides for the accurate performance analysis and predictions of the earned value indexes with a small sample.
The regression model can be utilized for demonstrating nonlinear and the interrelationships among them in problems of construction projects such as predicting earned value indexes. The regression model has been applied to develop a new prediction model by using SPSS software. Historical data in this technique was built up on ten sets of building projects database gathering between 2019 and 2022 through case study method as data collection instrument from cities, the Ministry of Construction and Housing, consultants, and contractors. Based the results of numerical data analysis performed in Section 3, data verification and validation were performed to validate the prediction of regression model in building projects as follows.
5.1.1. Verification and validation of the regression model
In this case study, it can take the historical data of three public building construction projects which were not included in the MLR model calibration processes were used as in independent verification examination of the proposed model in the public building construction projects. Though the most common statistical approaches have been utilized to determine the models accuracy are coefficient of determination (R2), coefficient of correlation (R), mean absolute percentage error (MAPE), and average accuracy percentage (AA%).
Below is the model’s summary, which has some important statistics. It provides us correlation coefficient (R) with a determination coefficient (R2) and the estimation of standard error. This statistical analysis was brought about for the regression models (CPI, SPI, TCCPI, and TCSPI) between output (earned value indexes) and input (Planned Value, Earned Value, Actual Cost, Budget at Completion).
On the other hand, the R-values for CPI, SPI, TCCPI, and TCSPI models are equal to 88.80%, 95.90%, 96.60%, and 91.40%, respectively, which is viewed as extremely aloft correlation. In addition, the determination coefficient shows the extent of the variety in input variable from an output variable, and that models have R2 values equal to 78.90%, 91.90%, 93.20%, and 83.40%, respectively.
From Figure , it can be seen that model (CPI), model (SPI), model (TCCPI), and model (TCSPI) verification have pretty performance so that offered high correlation coefficient (R) 88.80%, 95.90%, 96.60%, and 91.40%, respectively, and also the coefficient of determination (R2) is 78.90%, 91.90%, 93.20%, and 83.40%, respectively. Therefore, it can be concluded that these models show an excellent agreement with the actual measurements.
5.1.2. Validation of multiple regression model
In carrying out the validation of regression model, four main tools can be used to validating of the multiple linear regression models as following.
5.1.2.1. Using a table of critical values
The 95% critical value of the sample correlation coefficient as shown in may17 may be utilized to assign a valid idea of weather the calculated value of R is significant or not significant. Emulate (R) to the suitable critical value in Table . If (R) is not between the negative and positive critical values and then correlation coefficient is significant. If (R) is significant can be used to forecasting.
Table 17. Critical Values: Pearson Correlation (Chai et al., Citation2014)
Value (R) computed value of earned value index for Cost performance index regression model, Schedule performance index regression model, To complete the cost performance index regression model, and To complete the schedule performance index regression model were equal to 88.80%, 95.90%, 96.60%, and 91.40%, respectively, using the number of public building construction projects (n) equal to ten real life projects.
In this case study, ten sets of real-life building construction projects were taken for conducting the study. Thus, the Degree of Freedom (df=n-2 = 10–2 = 8). The correlation between degrees of freedom and critical values was presented in Table .
Based on the relationship between degrees of freedom and critical values presented in Table , the critical values correlated with degrees of freedom equal to 8 ranging between ±0.632. If value (R) is less than negative critical value or (R) greater than positive critical value, then correlation coefficient (R) is significant. Since, the correlation coefficient (R) for CPI, SPI, TCPI, and TSPI were equal to 0.888, 0.959, 0.966, and 0.914, respectively, and 0.888 > 0.632, 0. 959 > 0.632, 0.966 > 0.632, and 0.914 > 0.632. The correlation coefficient values of all earned value indexes were greater than the critical value. Therefore, the correlation coefficient is significant and the multiple linear regression models can be applied for prediction of the Earned value indexes.
5.1.2.2. “t” test
There is another test of significance of coefficient of correlation in which the value of “t” is computed by the following equation (Chai et al., Citation2014).
If the computed value of “t” is greater than the table value (t- distribution), the correlation is taken as significant. For 95% confidence and df = n-1 = 9, the t- table = 2.306 and t computed value for CPI, SPI, TCPI, and TSPI value were equal to 5.460, 9.617, 10.671, and 6.383. Therefore, t computed t-table. This indicates that the correlation coefficient is highly significant, and the multiple linear regression models can be applied for prediction of the Earned value indexes.
5.1.2.3. Mean absolute percentage error (MAPE)
The mean absolute percentage error (MAPE) is one of the most widely used measures of forecast accuracy and it can be helpful as to measure of quality for regression models. Let x, y, and n denotes the actual, predicted value, and total number of observations, respectively. Then, MAPE is represented in the formula defined by (Chai et al., Citation2014), below.
According to Chai et al. (Citation2014), for a four-point response item, Mean absolute percentage error produces a value ranging from 0 to 10 was highly accurate forecasting, 10–20 was Good forecasting, 20–50 was Reasonable forecasting, and the value of MAPE greater than 50 was considered as inaccurate forecasting.
Based on the results of the standard EVA and predicted value of dependent variables, the Mean absolute percentage error value for CPI, SPI, TCCPI, and TCSPI was presented in Table below.
Table 18. Mean Absolute Percentage Error value for earned value indexes. Source: own work, 2022
Based on the results of the mean absolute percentage error value as it was observed in Table , the projects have good forecasting regarding CPI, SPI, TCPI, and TSPI. The MAPE value of the derived performance metrics was between 10 and 20. This indicates that the predicted model was higher accuracy. Furthermore, the dependent variables are sufficiently defined and explained by the independent variables. Thus, the percentage error for the predicted model would be small and it indicates that it is valid to consider the proposed model acceptable.
5.1.2.4. Average accuracy degree
Average Accuracy degree: Statistical measures can be used to evaluate the performance of the multiple regression models as follows (Baqer & Rezouki, Citation2020).
Finally, Mean Absolute Percentage Error (MAPE) created by multiple regression models for the cost performance index model, schedule performance index model, complete cost performance index model, and to complete schedule performance index model was equal to 15.38%, 19.50%, 8.04%, and 17.82%, respectively. Therefore, Average Accuracy (AA %) for the cost performance index model, schedule performance index model, to complete cost performance index model, and to complete schedule performance index model was equal to 84.62%, 80.5%, 91.96%, and 82.18%, respectively. Thus, the predicted model can be presented as excellent with the actual data.
5.1.3. Verification of the regression model
The summary of computing CPI, SPI, TCPI, and TSPI by MLR for verification of estimating models has explained by Table . Where column two, four, six, and eight has actual Index that gotten from public building project under construction in Ethiopia, and other column represents estimate Index after applying MLR equation on them, where MLR equation has gotten through SPSS program. The comparison between the estimated and actual Index is shown in Table .
Table 19. Results of the Comparative Study. Source: own work, 2022
Table 20. CPI, SPI, TCPI, and TSPI Calculated through MLR for Verification of Estimating Model. Source: own work, 2022
Correlation coefficient between columns (Actual Index and Predicted Index by regression model equal to 88.80%, 95.90%, 96.60%, and 91.40% for Cost Performance Index (CPI), Schedule Performance Index (SPI), To Complete Cost Performance Indicator (TCPI), and To Complete Schedule Performance Indicator (TSPI), respectively; therefore, it can be concluded that this model has an excellent covenant with the actual measured results.
5.1.4. Summary of main finding
The main research findings of the proposed model can be highlighted with regard to its accuracy, precision, and from cost, and schedule performance viewpoints.
The most common statistical approaches have been utilized to determine the models accuracy are mean absolute percentage error (MAPE), average accuracy percentage (AA%), coefficient of determination (R2), coefficient of correlation (R). Results outputs of the comparative study have been clear in Table that the mean absolute percentage error (MAPE) is 15.38% for CPI, 19.50% for SPI, 8.04% for TCPI, and 17.82% for TSPI. The value of average accuracy is 84.62% for CPI, 80.5% for SPI, 91.96% for TCPI, and for 82.18% TSPI. The value of coefficient of determination is 78.90% for CPI, 91.90% for SPI, 93.20% for TCPI, and 83.40% for TSPI. Similarly, the value of coefficient of correlation is 88.80% for CPI, 95.90% for SPI, 96.60% for TCPI, and 91.40% for TSPI.
Thus, based on the results of average accuracy, coefficient of correlation the regression model to maintain more accurate and precise estimates in all the four models compared to the traditional earned value approach, as it means reliability in the accurate forecasting of the regression model.
Second, the proposed model provides more precise estimates. Precision refers to the narrowness of the forecasting error. The mean absolute percentage error value for the predicted model was 15.38% for CPI, 19.50% for SPI, 8.04% for TCPI, and 17.82% for TSPI. Therefore, the developed regression model gives more accurate and precise estimates.
In standard earned value analysis integrates only the planned value, actual cost, and earned value of the project for determining the performance indicators to evaluate their performance. Unlike the standard earned value analysis the developed model provides to incorporate the budget at compilation variables concepts in calculation of earned value indexes. Thus, the demonstration of these variables in to the forecasting formula reflects to increase the accuracy, and reliability of the prediction model through minimizing the standard errors associated with the earned value index.
Furthermore, the finding of the study revealed that the application of new methods are suggested to assist the building construction projects managers in the task of selecting and implementing the appropriate prediction method, and finally to prove the value that a more accurate forecasting model enables project teams to better control tight budgets and schedules through minimizing error, and more accuracy.
5.2. Managerial implications
The outcomes of this research provide some significant implications to predict the earned value indices in public building construction projects. The study provides a real-time approach to estimating project cost and duration by using regression modeling in public building construction projects. It would also help us to understand how managers could use certain techniques to better the project cost time in building construction projects by using regression modeling. The consequences of this research prove that the outcome has realized a realistic schedule with budgeted cost and duration that can incorporate lessons learned from similar history projects.
The use of regression model provides an opportunity to analyze a broader spectrum of data in the form of probability distribution of the project cost. Further, in order to minimize possible error related to wrong probability variables, distribution were defined through application of variables to enhance a more accurate prediction of the final cost.
Additionally, this paper helps us to carry out an integrated methodology of project cost, and schedule metrics into a single measurement system. It is widely applied for measuring and analyzing project actual status against its baseline, and for providing estimates of earned value indices.
Moreover, carrying out this study helps us to derive an estimate of the resources needed to complete the project, and also helps us to provide the basis to assess work progress against a baseline plan, relates technical, time, and cost performance, provides data for pro-active management action and provides managers with a summary of effective decision making. Furthermore, the importance of this study provides to identify future opportunities and analyze the actual performance of public building construction projects, not only focusing on planned expenditure and actual cost. Thus, the project managers can keep track of the possible areas that are prone to risk. Hence, it provides us to create plans accordingly.
5.3. Limitations
In the course of conducting this research, some knowledge was gained, which reveals the limitation of the techniques or methodologies in this research article. With this insight, it is worthwhile to outline additional methods that could be pursued in the future to extend the frontier of knowledge concerning regression modeling for the prediction of earned value indices in public building construction projects.
The main deficiency of the published paper is the unavailability of adequate documents for getting additional information on for prediction of earned value indices in public building construction projects. The second concern associated with the traditional approach is that it provides unreliable cost forecasts early into a project life because of few available earned value management data in public building construction projects.
In addition, within the earned value management methodology, computing earned value indices do not consider schedule progress in cost estimates. To fill these gaps, a multiple linear regression methodology is proposed to provide a more reliable prediction model that overcomes the limitations inherent to traditional earned value approaches.
6. Conclusions and remarks
Public building construction projects are among the most important projects today in Ethiopia, and for this the idea of this study came to predict the earned value index by using multiple linear regression methods. The main conclusions are that:
The multiple linear regression methods can provide an important contribution in the prediction of earned value indexes in the public building construction projects in Ethiopia.
In this study, four models was developed using four variables as inputs which are the planned value, Earned value, actual cost, and budget at compilation were self-assertively assigned and agreeably depicted for public building construction projects.
The four forecasting models were developed to foresee Schedule Performance Index (SPI) as the first model, Cost Performance Index (CPI) as the second model, To Complete Cost Performance Indicator as the third model and the fourth model is To Complete Cost Performance Indicator (TCPI) in Ethiopia was chosen as dependent variables for predicting earned value indexes in public building construction projects through using regression modeling a case study.
An equation was found that the Mathematical accuracy of 84.62%, 80.5%, 91.96%, and 82.18% to predict the CPI, SPI, TCCPI, and, TCSPI, respectively, of public building construction projects in Ethiopia.
It was found that the regression model shows excellent results in prediction.
The results of this study provide an important indicator and an additional predictive guide for predicting the performance of public building construction projects.
It has been noticed that the regression model showed good results of estimation in terms of correlation coefficient (R) generated by multiple linear regression models for CPI, SPI, TCCPI, and, TCSPI was equal to 88.80%, 95.90%, 96.60%, and 91.40%.
Similarly, the result of the coefficient of determination (R2) generated by multiple linear regression models for CPI, SPI, TCCPI, and, TCSPI was equal to 78.90%, 91.90%, 93.20%, and 83.40%, respectively.
At long last, a result tends to be presumed that the relationship between the dependent variable and independent variables of the built model was excellent and it shows a brilliant concurrence with the genuine estimations.
The regression model developed in this study is practical and predicts reliable figures in the building construction projects which help to make better decisions.
This research is an important addition in the world of knowledge, which aims to improve the appreciation of project objectives and increase confidence in expectations, which can be verified statistically.
This developed model lies in its importance for future forecasts for other projects that have realized a realistic earned value indexes that can incorporate lessons learnt from similar history projects.
Finally, the findings of this study provide academicians and practitioners with insightful information on the prediction of earned value indexes in public building construction projects to demonstrate its practicality would be the best area of exploration.
Data availability statement
The source of data used to support the findings of this study is available from the corresponding author upon request.
Disclosure statement
No potential conflict of interest was reported by the authors.
Additional information
Funding
Notes on contributors

Genet Melkamu Ayalew
Genet Melkamu is a lecturer at the Gondar Institute of Technology, University of Gondar, P.O. Box 196, Gondar, Ethiopia. She received her MSc degree in Construction Engineering and Management from the University of Gondar. Her research interest includes EVA, Project Management, and Regression Modeling.
Meseret Getnet Meharie
Meseret Getnet (Ph.D.) is a senior lecturer at Adama Science and Technology University, Adama, Ethiopia. He is also an Assistant professor at Adama Science and Technology University. His research interest includes BIM, EVA, AHP, Regression Modeling, and Simulation.
Girmay Getawa Ayalew
Girmay Getawa is former a lecturer at the Gondar Institute of Technology, University of Gondar. Now he is a lecturer at the Woldia Institute of Technology, Woldia University, P.O. Box 400, Woldia, Ethiopia. He received his MSc degree in Construction Engineering and Management from the University of Gondar. His research interest includes Road Maintenance, FMDMM, Regression modeling, and EVA.
References
- Aidan, I. A., Jaber, F. K., Al-Jeznawi, D., & Al-Zwainy, F. M. S. (2020). “Predicting earned value indexes in residential complexes ’ construction projects using artificial neural network model,” no. March, 1–30. https://doi.org/10.20944/preprints202003.0363.v1
- Almeida, R., Abrantes, R., Romão, M., & Proença, I. (2021). ScienceDirect ScienceDirect the Impact of uncertainty in the measurement of progress in earned value analysis earned value analysis. Procedia computer science, 181(2019), 457–467. https://doi.org/10.1016/j.procs.2021.01.191
- Al-Zwainy, F. M. S. (2020). Forecasting techniques in construction industry: Earned value indicators and performance models. Scientific Review Engineering and Environmental Studies (SREES), 29(2), 234–243. https://doi.org/10.22630/PNIKS.2020.29.2.20
- Baqer, S. M., & Rezouki, S. E. (2020). Modeling of Estimate at Completion in Earned Value Modeling of Estimate at Completion in Earned Value. https://doi.org/10.1088/1757-899X/901/1/012022
- Behl, A., & Pal, A. (2020). Interpretive Structural Modeling. 26(6), 1–27. https://doi.org/10.4018/978-1-7998-2216-5.ch001
- Bekr, G. A. (2017). Factors affecting performance of construction projects in unstable political and economic situations. ARPN J Eng Appl Sci, 12(19), 5384–5395.
- Boateng, E. Y., & Abaye, D. A. (2019). A Review of the Logistic Regression Model with Emphasis on Medical Research. https://doi.org/10.4236/jdaip.2019.74012
- Buyse, P., & Vandenbussche, T. (2010). Performance analysis of earned value management in the construction industry.
- Chai, T., & Draxler, R. R. (2014). Root mean square error (RMSE) or mean absolute error (MAE)? – Arguments against avoiding RMSE in the literature. Geoscientific Model Development, 7(3), 1247–1250.
- Chai, T., Draxler, R. R., & Prediction, C. (2014). Root mean square error (RMSE) or mean absolute error (MAE)? – Arguments against avoiding RMSE in the literature. Geoscientific Model Development, 7(3), 1247–1250. https://doi.org/10.5194/gmd-7-1247-2014
- Chandrasekaran, R., & Kumar, R. V. (2012). Application of logistic regression to predict over target baseline of software projects. International Journal of Computer Applications, 44(April), 1–6. https://doi.org/10.5120/6296-8493
- Eirgash, M. A. (2019). “Earned value analysis for construction projects using project management scheduling engine,” no. American Journal of Civil Engineering, 7(5), 121. https://doi.org/10.11648/j.ajce.20190705.11
- Gupta, A., Sharma, A., & Goel, A. (2017). Review of Regression Analysis Models. International Journal of Engineering Research And, 6(8), 58–61. https://doi.org/10.17577/IJERTV6IS080060
- Huang, H., & Ho, C. Applying the fuzzy analytic hierarchy process to consumer decision-making regarding home stays. International Journal of Advancements in Computing Technology, February. 2013, 2016 5(4), 981–990. https://doi.org/10.4156/ijact.vol5.issue4.119
- Jaber, F. K., Jasim, N. A., & Al-Zwainy, F. M. S. (2020). Forecasting techniques in construction industry: Earned value indicators and performance models. Scientific Review Engineering and Environmental Studies (SREES), 29(2), 234–243.
- Kuhl, M. E. and Graciano, M. K. P., “Project planning and predictive Earned Value analysis via simulation,” Proc. - Winter Simul. Conf., pp. 3377–3387, 2015, https://doi.org/10.1109/WSC.2014.7020171.
- Marco, D. (2022). ScienceDirect ScienceDirect multiple linear regression regression model for project cost multiple linear forecasting forecasting. Procedia computer science, 196(2021), 808–815. https://doi.org/10.1016/j.procs.2021.12.079
- Mooi, E. (2016). Regression Analysis, no. July.
- Moslemi Naeni, L., Shadrokh, S., and Salehipour, A., “Erratum to a fuzzy approach for the earned value management [International Journal of Project Management, 32, (2014), 709–716 https://doi.org/10.1016/j.ijproman.2013.02.002.
- Narbaev, T. (2014). “An Earned Schedule-based regression model to improve cost estimate at completion timur narbaev, alberto de marco international journal of project management - Elsevier - Pre,” no. International Journal of Project Management, 32(6), 1007–1018. January 2019. https://doi.org/10.1016/j.ijproman.2013.12.005
- Nyangwara, P. O., & Datche, E. (2015). Factors affecting the performance of construction projects: a survey of construction projects in the coastal region of Kenya. International Journal of Scientific and Research Publications, 5(10), 1–43.
- Oke, A., Ogungbile, A., Oyewobi, L., & Tengan, C. (2016). Economic Development as a Function of Construction Project Performance. Journal of Construction Project Management and Innovation, 6(2), 1447–1459.
- Pookot, N. (2017). International journal of engineering sciences & research technology a comparison of different cost forecasting methods using earned value metrics. 6(6), 302–319.
- Prasanth, A., & Thirumalai Raja, K. (2007). Project performance evaluation by earned value method. International Journal of Innovative Research Science Engineering Technology An ISO Certify Organisation, 3297(1).
- Richard, D. (2001). Analysis and application of earned value management to the Naval Construction Force.
- Salari, M., Bagherpour, M., & Wang, J. (2014). A novel earned value management model using Z-number. International Journal of Applied Decision Sciences, 7(1), 97–119. https://doi.org/10.1504/IJADS.2014.058037
- Salikuma, S., Minu, M., & Johny, A. (2016). Application of earned value analysis in analysing project performance. International Journal of Engineering Research And, 5(9), 459–464. https://doi.org/10.17577/IJERTV5IS090341
- Salikumar, S., & Anna Johny, M. (2016). Application of earned value analysis in analysing project performance. International Journal of Engineering Research And, V5(9), 459–464. https://doi.org/10.17577/ijertv5is090341
- Shenoy, S. (2015). Project performance evaluation by earned value analysis. 2(5), 1675–1684.
- Suresh, S., & Ramasamy, G. (2015). Analysis of project performance using earned value analysis. International Journal of Scientific & Technology Research, 4(4), 1080–1085. [Online]. Available. https://doi.org/10.25077/jrs.15.1.12-20.2019.
- Vyas, A. B., & Birajdar, B. V. (2016). Tracking of construction projects by earned value management. International Journal Technology Research, V5(3), 829–831. https://doi.org/10.17577/ijertv5is031318