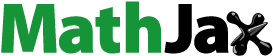
Abstract
All across the world, the construction industry is a very crucial sector for development. Cost prediction is the process of forecasting the cost of construction projects at an early stage. An effective estimation technique is necessary to reduce the effort, time, and cost. Therefore, the study aims to develop a mathematical model to predict the cost of Ethiopian Roads Authority road projects in the case of Ethiopia. The data were collected from primary and secondary sources. The method of data analysis for the study was multiple regression analysis, and it was done using SP SS and Microsoft Excel. The data were obtained from a road document review and a questionnaire survey of 62 respondents and 15 interviewers who are working in ERA. The major cost-influential factors were found to be: inflation rate, bridges, culverts, project length, width, type and scope, contract duration, and location. As a result, a multiple regression model was developed using nine significant parameters taken from 18 completed road projects. The multiple regression analysis result revealed that the model can perform at a success rate of 83.8%. The MAPE of the model was ±16.44%. The conclusion and recommendations are likely to support and reduce ineffective estimation trends.
Impact Statement
Road construction projects play a vital role in the development of infrastructure, ensuring safe and efficient transportation for communities. However, cost overruns and lack cost estimation tools in road projects have been a persistent challenge, leading to budget constraints and delays. To address this issue, we propose the development of a mathematical model that can accurately predict the cost of road projects. This innovative solution aims to enhance cost estimation accuracy, optimize resource allocation and foster responsible financial planning.
Reviewing Editor:
1. Introduction
All across the world, the construction industry is regarded as a very crucial sector for development. A country’s road infrastructure is crucial because it is the most common method of moving people, products and services from their point of origin to their intended destination. Additionally, they found that the construction cost estimate is the key component and the estimator can produce the overall expenses with reasonable accuracy given the essential project details (Akinradewo et al., Citation2020).
Velumani and Nampoothiri (Citation2019) defined that, cost prediction is the basic tasks that needs a great attention and it is the process of forecasting the total cost of construction projects. According to Kanchana and Janani (Citation2018), the early precise cost estimation of a project is crucial to its success and when project information is insufficient. The authors also stated that, construction cost estimation and prediction is the basis of cost budgeting and cost management. Kaleem et al. (Citation2014) also determined that, early and reliable cost estimates are essential inputs for decision making in the initial stages of construction projects.
According to Ravindran (Citation2018), the primary problem in the construction industry is currently dealing with is that project contracts are often completed for amounts that are significantly higher than the estimated cost; as a result, a predictive cost model that uses principal components to grasp factors affecting project cost is required. An effective estimation technique is necessary to reduce the effort, time and cost that invest for estimating.
The author also determined that, inflation rate, road structures, project location, material cost, inadequate estimation methods, incomplete data and contract duration all have an impact on the cost of road construction projects. Sonmez and Ontepeli (2019) indicated that, over the last thirty years, road projects have increased in its complexity and inaccuracy of project cost estimates also has become a larger concern. Kim et al. (Citation2008) also determined that, from the standpoint of the infrastructure providers, precise cost estimates enable efficient project budgeting and funding.
According to Andom (Citation2015), many developing nations, including Ethiopia, lack efficient methods for estimating project budgets before performing in-depth calculations. Based on the studies of Tadesse and Dinku (Citation2017), there are a numbers of major problems in Ethiopian construction, particularly in the road sectors, include the absence of preliminary data, a lack of project budget-fixing tools, inaccurate estimation trends, a lack of appropriate cost assessment techniques and the existence of numerous environmental, political, social and external uncertainties.
According to Alemayehu (Citation2014a,Citationb) even though the fact that, cost prediction is still a challenging and complex topic, experts are continually researching and testing various ways and methodologies to address it. The author also specified that, accurate cost estimation in the early project development is an essential issue where detailed project cost information is unavailable. Tayefeh Hashemi et al. (Citation2020) also stated that, If only cost prediction is a crucial step for every firm because it comes before budget prices and resource allocation during the planning and feasibility study phases of a project.
As studied by Sarode and Chandgude (Citation2020) in the world’s construction context, lack of cost, cost overrun and risk factors are the most important and challenging activities which occurs at the early stages of a project life cycle where limited information is available. Research conducted by the ERA Research Center on causes of cost overruns on Ethiopian Federal Road projects in 2016 showed that, inaccurate cost estimation practices were determined as one of the causes of cost overrun.
This indicates that measurements tools are required to improve cost estimates reliability. There is a gap in early determination of effective budgets and lack of easy, quick and effective techniques to predict the cost of Ethiopian road projects. It is very important to find a way to know the cost of road projects in a short time with reliable accuracy especially at the initial phase of road projects.
The proposed method enables decision analysts to better understand the complete evaluation process and provide a more accurate, effective and systematic decision support tool. The importance of this research paper is also to provide a reference guide for the company in general to forecast the appropriate road project cost. The study provides a significant source of secondary data for future users to get knowledge, skill and understanding of road cost estimation methods.
2. Literature review
The time, cost and quality are crucial, conflicting aspects to construction project management. Tradeoff optimization among project duration (time), project cost, and the project quality within the project scope is necessary to enhance overall construction project benefit (Luong et al., Citation2021). Dharwadkar and Arage (Citation2018) investigated the estimation of cost is the basic component of all project-related engineering fields, which greatly affects planning, bidding, design and implementation. Swei et al. (Citation2017) determined that, as project planners continue to move toward frameworks such as probabilistic life-cycle cost analysis to evaluate competing transportation investments, there is a need to enhance the current cost-estimation approaches that underlie these models to enable improved project selection.
As the definition of Akinradewo et al. (Citation2020), cost estimates used for evaluating projects, determining project feasibility, evaluating concepts and approving preliminary budgets are generally derived through four methods and the level of accuracy of these methods varies based on the project status and available information.
2.1. Cost estimating in construction projects
As the study of Alemayehu (Citation2014a) the major task of the construction industry is cost estimating. The success or failure of a construction project depends on how accurate cost estimates are throughout the project, from the early stages through tender.
According to the findings of Leung et al. (Citation2011), the majority of earlier studies primarily focused on the contractor's perspective to forecast project status to provide early warning of cost overruns and schedule delays, while in the field of highway construction, the studies emphasized on project cost estimation for budgeting proposals because a highway construction project is typically funded primarily by the government.
The author concluded that, a unit pricing contract is frequently employed in the construction of highways. If the project goes beyond budget, further funds will be required to finish it. The government official assigned to the project must regularly assess the project's financial condition, estimate the total cost and length of time required to accomplish it and provide top management with frequent project progress reports.
2.2. Types of cost estimates
As the studies of Akinradewo et al. (Citation2020), estimates inform the project team on the financial implications of inputs made to the proposed projects which allow for proper budgeting and cost control depending on the phases of construction. The major types of cost estimates are listed in .
Figure 1. Construction cost estimating methods (Jamous, Citation2013).

2.3. Strength and weakness of the basic types of cost estimates
Gao (Citation2020) states depending on where the program is in its life cycle, many types and methods of cost estimation may be chosen. The advantages, disadvantages and uses of the three cost estimates are summarized in and .
Table 1. The strengths, weaknesses and applications of the three types of cost estimates.
Table 2. Summary of cost estimating methods (Operations, 2020).
2.4. Factors affecting cost estimation of road projects
Research conducted by Gidissa (Citation2017) reveals that, the following factors are identified as contributing factors: the cost of materials, contract modifications, location of the project, the rework of poor performance, the frequent switching of sub-contractors companies, the lack of technical expertise, the lack of experience on similar projects, the lack of experienced staff and labor, the need for high-quality work, a lack of clarity in the specifications, a delay by the owner in releasing the contractor's financial payment, an incomplete drawing, a lack of equipment, poor labor and material scheduling and poor documentation.
2.4.1. Cost influential parameters of road projects
According to Akintoye (1999), underestimating a job could lead to the organization incurring cost overruns or losing money if it wins a competitive tender without assigning enough resources to complete the work. As the idea of Akintoye the cost estimation method is crucial because it enables construction businesses to ascertain their direct expenses and to establish a ‘bottom line’ cost beyond which it would not be financially feasible to complete the task. According to Attal (2010), project length, geometric design standard, two-lane length, bridge type, bridge width, shoulders and gutters were some of the factors taken into consideration. Consequently, the major parameters in terms of highway construction cost prediction are explained as follow.
2.4.1.1. Project location
Attal (2010) point out that, location of the project is important factor correlated to populated area, ground condition and profile and environmental impacts. The distance of the project from resources supply unit is highly affects the project cost. On the other hand, the impact of the weather on the construction process is associated with the season of the year.
2.4.1.2. Length and width of the road
Alemayehu (Citation2014a) defined it as: a carriageway or roadway comprises road breadth on which a vehicle isn’t confined by any physical barriers or partition to be in motion laterally. The carriageway breadth depends upon the traffic lane breadth and number of lanes. He determines that Road width (size) and road length are the most influencing variables under parametric study.
2.4.1.3. Project type and scope
The study of Irfan et al. (Citation2011) also specified that, a project type is a project classification defined during implementation that specifies essential project attributes and determines how that project’s costs are processed. The authors investigated the estimation of highway project cost on the basis of variables known at the planning phase such as planned cost, project type and project scope. The common project types under road construction are asphalt concrete, gravel surfacing and surface treatment. As a result, the project type has a direct impact on the increasing or decreasing of the actual project cost.
2.4.1.4. Contract type
According to the study of Gofhamodimo (Citation1999), choosing the right type of contract is a very important step in contracting, be it for the client or the contractor. Each party should choose the contract basing on the long and short term interest of the project. The best and most direct way to understand the reasons for the different type of construction contracts is to examine and analyses the cost of construction. The item rate, admeasurement and lump sum are the common contract type under construction project works.
2.4.1.5. Project delivery method
Per the research conducted by Zuber et al. (2018), the selection of project delivery method is one of the factors that can influence the success of a construction project. Therefore, understanding each of the primary project delivery methods used in construction industry; Design-Bid- Build (DBB), Construction Manager at Risk (CM at Risk) and Design-Build (DB) are important before the decision-making.
2.4.1.6. Number of lanes
Vijay et al. (Citation2020) have conducted research on effect of number of lanes on highway capacity and conclude that, increasing number of lanes should bring about the changes of driver behavior, car interactions and increase the construction cost which would finally result in the decreases of average capacity per lane on highways.
2.4.1.7. Terrain type
The research conducted by Shaban et al. (Citation2017) indicated that, the geology of the terrain is the most influential as it impacts directly on the other considerations. The geological factors were given the most attention in the road hazard assessment, including topographic relief, water flow regime, properties of the surface cover and types of material. The terrain also classified under flat, rolling, mountainous and escarpment based on the nature of the topography.
2.4.1.8. Climatic condition
The investigation of Befkadu et al. (Citation2016) determined the effects of weather change are manifested around the world; it is now known that no nation including Ethiopia is threatened from its effects. The effects of weather change are evidenced in different parts of Ethiopia. A change in weather will influence the most cost-effective method of carrying out highway construction. Three main conditions are cold, warm and moderate temperature. Likewise Chakraborty et al. (Citation2016) defined that, accurate weather information is required to assess the performance of sustainable buildings. Currently such performance is assessed using Typical Meteorological Year (TMY) weather files.
2.4.1.9. Bridges and culverts
The study of Abera (Citation2016) bridges and culverts are highly costing structures that support a roadway over the water body by means of a span. Poor site selection can result in a longer, wider or higher structure than is actually necessary and can also lead to excessively high construction and maintenance costs. Mcenroe (2007) also indicated that, the first step in designing a bridge or a culvert is to determine the size of opening necessary to take the water.
2.4.1.10. Grade of the contractor
Based on the findings of Mumela et al. (Citation2017), it can be concluded that, contractor’s knowledge and experience has a direct and positive influence on cost estimation in plant infrastructure projects. The greater the levels of contractor’s knowledge and experience increases the confidence levels of estimators, consequently impacting positively on their ability to give a precise cost estimate.
2.4.1.11. Inflation rate
The report of Profile (Citation2015) specified that inflation is generally defined as the economic situation where general price level rises continuously without an accompanying rise in production of goods and services. Inflation always remains a major detrimental factor to economic progress of an individual as well as a nation. Foreign Exchange Rate also plays crucial role in inflation variations.
2.4.1.12. Contract duration
According to Williams and Songer (2018) contract duration is the time allotted to the contractor, by the owner, for the performance of all duties within a contract (Williams and Songer Citation2008). This duration is one of criteria for project performance. Unreasonably short contract durations raise the bid price, raise the actual project cost, restrict qualified bidders from submitting bids, have potential to reduce the quality of the work and increase the potential for legal disputes. Conversely, unreasonably long contract durations are a general inconvenience to the traveling public and encourage less qualified contractors to submit a bid.
2.4.1.13. Numbers of control points/villages
Based on the studies of De Bock and Van den Bergh (Citation2004), the environmental and social impacts of the project where predicted in relation to environmental and social receptors or people (e.g. residents of villages and settlements and land-use) and natural resources. The route selection of road design should consider the city, town and villages future benefited from the accessibility of road projects. This condition has positive and negative impacts on the actual project costs.
2.5. Studies on project cost estimation model
As a result of Liu et al. (Citation2019) growing complexities in the construction industry, system dynamics modeling (SDM) has been increasingly used in construction management (CM) research to explore complicated causal relationships at the various levels of construction and management processes. Hu and Skibniewski (Citation2021) also studied eight construction projects in Europe and discovered that, inaccurate cost estimations must be addressed because the cost estimation is the basis for creating a project budget and schedule. Elmousalami (Citation2019) deliberated entitled on ‘intelligent methodology for project conceptual cost prediction’ by identifying cost driver parameters and developing a reliable parametric cost model at the conceptual stage of the project is crucial for projects managers and decision makers.
Recently, Son and Nguyen Dang (Citation2023) also proposed. The multi-verse optimizer (MVO) has emerged as a promising stochastic optimization algorithm in this field, as it efficiently explores and exploits the search space. The study was proposes the MVO model as a new tool to address time–cost optimization problems (TCOPs). Moreover, Adel et al. (Citation2016) similarly studied on the title of developing parametric model for conceptual cost estimate of highway projects by using neural network stated that, conceptual cost estimate is a crucial indication about the future of infrastructure projects at early stages before the completion of detailed design. It is an experience-based process, which involves determinations of complex relationships between cost and its influencing factors. Saeidlou and Ghadiminia (Citation2023) correspondingly investigated on ‘a construction cost estimation framework using DNN and validation unit’ accurate construction cost estimation is crucial to completing projects within the planned timeframe and expenditure. The estimation process depends on multiple variable relationships between themselves and the target cost.
Likewise, Tadesse and Dinku (Citation2017) was investigated a research on Conceptual Cost Estimation of Road Projects in Ethiopia Using Neural Networks with the following road parameters; Project location, project scope, wearing surface class, road length and duration, terrain classification, project delivery system and structural intensiveness. As well Son et al. (Citation2021) proposed the use of material requirements planning (MRP) to control the cost of construction materials. In other way, Son and Khoi (Citation2023) anticipated the mutation-crossover slime mold algorithm (MCSMA), which balances time, cost, quality and work continuity in a specific building project.
Additionally, Meharie and Shaik (Citation2020) was conducted a research entitled ‘Predicting Highway Construction Costs: Comparison of the Performance of Random Forest, Neural Network and Support Vector Machine Models’ by using the following road parameters; Project length, Number location, Project duration, Contract amount, Performance index and Climate of bridge, Inflation rate, Project scope, Terrain type, Project type and project location.
The study was conducted on improving cost estimation performance: on the title of An Investigation of Prediction Technique. Despite this study is derived from the context of construction industry in Malaysia, hybrid models developed and findings achieved in this study probably are useful for other industries and countries to improve project planning, implementation and outcome assessments (Xiong, Citation2016).
Williams and Songer conducted a study on the Development of Mathematical Models for Preliminary Prediction of Highway Construction Duration incorporating the duration-influential project factors and their relationship. Dharwadkar and Arage (Citation2018) was conducted a research on prediction and estimation of civil construction cost using linear regression and neural network. Thomas and Thomas (Citation2017) were developed a model to predict project cost and duration based on historical data of similar projects in Switzerland.
By classifying and integrating the construction project cost concepts, constructing the prediction index system, combining with the recurrent neural network algorithm, constructing the excitation function and calculating the initialization threshold and finally constructing the prediction model. In the experiment, the proposed method is verified for the prediction accuracy. After the experiments, it can be proved that when the proposed model is used to predict the engineering (Abera Citation2016) cost, the root mean square error of the model output is small and has more ideal prediction accuracy (Wang et al., Citation2023).
Project managers need reliable predictive analytics tools to make effective project intervention decisions throughout the project life cycle. This study uses Machine learning (ML) to enhance the reliability in project cost forecasting (Narbaev et al., Citation2023). As Velumani and Nampoothiri (Citation2019) 20% of the project's cost overruns occur during construction, while 40% occur when the contract is awarded. For the owner to commit to future project cost implications during the tender stage, accurate estimates must be provided. The development of the model is so important to address these prediction issues. Juszczyk (Citation2020) concluded that, according to the criteria of general metrics (RMSE, MAPE), the three models demonstrate similar predictive performance. MAPE values computed for the training and testing of the three developed models range between 5% and 6%.
Linear regression studies the linear relationship between the dependent variable Y and a single independent variable X. As a result, the formula obtained for the prediction of the cost of housing construction is y = 1,169,813.19 + (334,729,758) X. According to the results of Lowe et al. (Citation2006), the best regression model is the log of cost backward model which gives an R2 of 0.661 and a mean absolute percentage error (MAPE) of 19.3%.
Alemayehu (Citation2014a) was testing regression models by taking the following road parameters. Earthwork; cut, fill and topping quantities (m3), Sub base and Base coarse quantity (m3), Asphalt quantity (m2), Road width (m), Road length (m) to estimate costs of road construction projects of Ethiopia. His result shows that coefficient of determination (R2) for the developed models is ranging from 0.65 to 0.98 which indicate that the predicted values from a forecast models fit with the real-life data. The values of the mean absolute percentage error (MAPE) of the developed regression models are ranging from ±16.3% for preliminary cost estimating and to ±38.9% for conceptual method of cost estimation.
Mohammed et al. (Citation2016) concluded that seven effectiveness factors are used for cost forecasting by MLR model. They involve Security Conditions, Tower Types, Experience of Contractor, Foundation Types, Tower High, Main Cable and Site Area. It was found that Multifactor linear regression has the flexibility to foretell the cost with an excellent degree of accuracy 90.1% and mean absolute percentage error 9.891% (Mahde Abd et al., Citation2019).
2.6. Summary of the literature review and gap identification
According the explanations of different writers cost estimating is the determination of the probable cost of a project. Project cost includes: Preliminary investigation (project appraisal costs); Design and supervision (consultancy cost); Construction works (contractor’s cost); Land owning cost and Monitoring costs. According to different researchers there are a number of parameters which are influencing the cost estimating trends which are; project location, project scope, design complexity, weather condition and socio-political and environmental condition, geological characteristics, price escalation, methods of cost estimation and skill of estimator are some of the common factors. There are also different cost estimating methods which were maintained in different journals such as; parametric method, expert judgment and unit price estimate. More or less regression model and artificial neural network were some of the model mentioned as the estimation techniques.
Various approaches were investigated on prediction of project cost at the conceptual stages. However, most of the data collection methodologies of those approaches were mostly depend on historical data analysis. Thus, questionnaire survey, interview and case-studies are also mandatory to strengthen the study. Most researchers had developed a prediction model using artificial neural network. Even though ANN is one of the mechanisms to predict with more trails, but it is difficult to understand the mathematical relationship between variables. Studies on the existing trends of estimation are limited. But this issue is very important to identify the existing poor practice and to improve it with more advanced methods. Due to lack of the expert information and the mismatch of the provided solutions with the reality, recent studies still show that, errors in cost estimation have not decreased. Scholars also developed simple linear regression estimating model and such tools do not show the relationship between two or more independent variables.
Due to the gaps of the above studies, the current research meant to fill the gaps with examining the current estimating practice and improving the poor trends with multiple linear regressions estimating model through conducting structured questionnaire survey, semi-structured interview questioners and historical data. The future applicability of the model also validated with the method of predictive validity.
3. Methodology
The research problem, which was based on the existing situation and gaps from earlier studies, was formulated using literature reviews. The research was built upon this problem-formulation method. In light of this, main and secondary data sources were selected, and a literature review was conducted to identify the factors that enabled the achievement of the study’s objectives. After that, the questionnaires were created, given to the responders, and the documents were examined. Based on the study’s findings, extensive deliberations and conclusions were drawn in order to identify areas for improvement ().
3.1. Research type and approach
The types of research pursued are descriptive and correlational based. The main goal of descriptive research is to explain the current situation as it stands. The purpose of this study is to describe and evaluate the current cost estimation techniques and provided easy, simple and quick cost estimation tool. The correlational research type is used to link the relationship between the dependent and independent variables. Both qualitative and quantitative approaches were employed to analyze the data.
3.2. Sampling technique
Purposive sampling techniques had been utilized to determine the sample size for this research, which is purposefully limited to experts with prior experience in the road industry and mostly focuses on finished road projects. Secondary data were gathered via literature research, and primary data were gathered from questionnaire surveys and document analysis.
3.3. Data collection methods
Among the various tools utilized to gather data, questionnaire surveys, interviews and document analysis were employed to gather all the necessary information to address the research objective.
3.3.1. Questionnaire
To accomplish the goal, a questionnaire survey was conducted for professionals who are working in Ethiopian Roads Authority including Client, Contractor and Consultant in addition to the Regulatory body. The questionnaire surveys were included 62 professionals who have different work position in the institution.
3.3.2. Interview
For the study, different data collection techniques, including interviews and document reviews, were used in addition to evaluating data collected via a questionnaire survey to confirm the validity and reliability of the data. 15 employees were chosen based on their prior knowledge, experience and skill. The purpose of the interview was to identify the current practices of road cost estimation, identify influential factors of road project cost and to select the existing model development parameters.
3.3.3. Document review
A systematic approach was used to access all types of documents offered by the Ethiopian Roads Authority (ERA) with references to cost forecast parameters. All the existing documents were examined to collect the relevant model parameters. Based on the availability of data the total numbers of completed road projects were included from 2007 to 2021. According to 15 years completed reports of ERA the total numbers of the projects were 23 completed projects and the model sample size was determined based the available projects.
The main reason to oblige the population started from 2007 was the absence of final completion reports of road projects before this year. As indicated in , 18 (1–18) projects were the target populations used to develop the model, and the remaining 2 (19–20) projects were used for the predictive validity test. The remaining three projects were not included in the study due to the incompleteness of the necessary model parameters. All the model parameters were referred from those projects document.
Table 3. Lists of completed road projects taken from ERA office from 2007 to 2021.
3.4. Data analysis and interpretation methods
The Statistical Package for Social Sciences (SPSS) and Microsoft Excel were used to assist multiple regression analysis of the data. And the findings were presented as pie charts, tables, summaries of numbers and statements.
3.4.1. Descriptive analysis
Descriptive analysis is the type of analysis of data that helps to describe, show or summarize data points in a constructive way. This technique can also determine the result of the analysis through mean and standard deviation in tabular and bar forms.
3.4.2. Regression analysis
SPSS Version 23 and Excel software are particularly special in terms of data analysis due to their unique features and capabilities. Both of the software’s have their own strengths; SPSS is more specialized for advanced statistical analysis, while Excel is more versatile and widely accessible. With the use of SPSS software, the data produced by the document review was examined. As a result, it offers an adequate basis for cost and budget planning predictions. Following the conclusion of the multiple regression analysis, the variables that were deemed significant and non-significant were determined, and the model was then developed. Where, x….xk are independent variables and y is the dependent variable, the multiple regression model predicts y based on the form's xi.
The Following steps were all the procedures followed while employing the software.
All the independent and dependent variables were filled on the variable view
All the values were filled on the data view
The data analysis button was selected
click on
Regression,
Linear
the dependent variable cell was made on and the variable have taken to that cell
the independent variable cell was made on and the variable have taken to that cell
all the necessary button was on
continued
ok was clicked
the result was observed on the regression result sheet. Example:-See .
(1)
(1)
Y is the predicted value of the dependent variable (y) for any given value of the independent variable (x).
B is the intercept, the predicted value of y when the x is 0.
b1 is the regression coefficient how much expect y to change as x increases.
x is the independent variable (the variables expect influencing y).
Ɛ is error of the estimate
3.5. Model evaluation metrics
The model's correlation, performance, validity and errors calculation were determined by using the following techniques.
Correlation coefficients are used to measure the strength of the linear association between two numerical variables.
to measure how well a regression model fits the data. It determines the percentage of model performance out of 100% (Kumari & Yadav, Citation2018).
Validity Test is the process of checking the applicability of the model to perform a sample calculation. New data were collected to check the validity of the model for future applications in the road sector (Meharie & Gariy, Citation2021).
The validity and residual were tested by, Mean Absolute Percentage
Where, yi – Actual data values, ŷi– Predicted or forecasted data values of the model and, n–Total number of model samples.
4. Results and discussions
4.1. Questionnaire distribution and organizational profile
Thirty-five questions were distributed to the road organization and percentages of distributed and collected questionnaires were presented in .
Referring to , the percentage of questionnaires distributed and collected were 38.7%, 27.4%, 22.6% and 11.3% from client, consultant, contractor and regulatory body respectively. In addition knowledge, experience and personal contacts helped for reaching the study objective. The position, educational background and work experience were stated as below.
shows that, the respondent profile and their interpretations are presented in percentage as follows. The percentage values of the respondent 12.9% were highway engineer, 11.3% were senior surveyor and office engineer, 6.45% were project manager, resident engineer and general manager, 9.7% were senior engineer, quantity surveyor and site engineer and 8.06% were work inspector and team leader. Concerning educational level 56.5% of the respondents were degree holders and 43.5%, were master’s degree holders. Regarding working experience of the respondents, 16.1% were between 0 and 5 years, 35.5% were between 6 and 10 years, 37.1% were between 11 and 15 years, 9.7% were between16 and 20 and 1.6% were above 20 years.
Table 4. Respondent’s profile.
4.2. Qualitative data presentation
Qualitative data is seeks to understand a given research problem or topic from the perspectives of the local population it involves (Farr, Citation2008). Four semi-structured questions were forwarded to 15 interviewees and the summary of their responses are presented in .
Table 5. Interviewees’ responses summary table.
4.3. Developing a mathematical model for predicting the cost of road projects
The model was developed by using cost parameters and their actual data obtained from the ERA office.
4.3.1. Document review results
The documents were analyzing the most important and available factors used as parameters to predict the cost of road projects which are available in Ethiopian Roads Authority. The document review included the final (closed) project completion reports as directly obtained from Ethiopian Roads Authority road projects. The reporting formats of the completed road projects more or less are similar in documentation styles. Among those detail issues the following most important parameters were selected which were necessary to develop the model; project type, project scope, project length, project width, terrain type, numbers of bridges, number of culverts, climatic condition, inflation rate (exchange rate), completion year of the project, contract duration, contract amount, grade of the contractor and actual project cost. As explained earlier in the sample size determination part among 23 projects only 18 projects were included for the model and 2 projects were remained for validity test. The remaining 3 projects were not clearly determined the necessary parameters while the document was referred.
4.3.2. Model development parameters
From the literature review, document review and current trends, a numbers factors were taken as the parameters to develop the prediction model. All of the above 17 variables were taken from the report of completed road projects ().
Table 6. Cost prediction parameters.
4.3.3. Regression analysis
Multiple regression analysis was applied to determine the relationship (correlation) between two or more variables in order to enhance the mathematical techniques utilized to estimate the cost of a road project. All of the quantitative data for the 17 attributes listed above came from the final completion reports of road projects. After copying all of the collected data to an SPSS spreadsheet, the regression analysis was carried out. There was a need to normalize the raw data, although there are various ways to change algorithms, the natural logarithmic method works better than the inverse, square root and other methods.
The raw, unstandardized data was normalized (transformed) to the necessary standards using a natural logarithmic (Ln) function. John Napier, published his discovery of logarithms in 1614. As the definition of John natural logarithm can be referred to as the power to which the base ‘e’ that has to be raised to obtain a number called its log number. The number ‘e’ is an important irrational number. It is approximately equal to 2.71828. The natural logarithmic function y = logex is abbreviated y = lnx and is the inverse of the natural exponential function y = ex. Based on the result of the regression analysis the most significant variables were selected.
4.3.4. Regression results
Regression analysis among 16 independent components revealed that three variables; project delivery method (X14), number of lanes (X15) and grade of contractor (X16) had constant values throughout all samples; therefore, they were immediately eliminated from the system. Whether parameters are introduced to the model or taken out of the system, SPSS views them as missing variables with no bearing on the outcomes. The remaining 13 independent variables and one dependent variable were considered as model parameters. The following table shows the outcomes of unstandardized and Ln transformed data.
Unstandardized and standardized mean values are shown in . The mean values of the Ln transformed data were reasonably near to each other when compared to the raw data. However, there were a lot of consequences from the raw data, and interpreting them was difficult.
Table 7. Descriptive statistics.
Dependent variable is actual project cost and predictors are (constant), inflation rate, project width, terrain type, number of bridges, project location and number of culverts, contract duration and project length and project scope. displays sum of square of regression, residual and total of regression. It also shows the degree of freedom(df) of model, the significance value, mean square value and ratio of regression with residual (F).
Table 8. ANOVA table (statistical significance of the model).
According to Ferreira and Patino (Citation2015), the p value is defined as the probability of observing the given value of the test statistic, or greater, under the null hypothesis. Commonly the cut-off value to reject the null hypothesis is 0.05, which means that when no difference exists, such an extreme value for the test statistic is expected less than 5% of the time.
Constant, project scope, project length, project width, terrain type, number of bridges, number of culverts, project location, contract duration and inflation rate are all given in as p values of .003, .046, .016, .020, .010, .015, .001, .007, .015 and .007 respectively. The variables were statistically significant and used to create a multiple linear regression model since their p values were less than 5% or (.05). The p value of project type, climate condition, number of control points and contract type are, .696, .880, .807 and .443 respectively. Because the p value for these is higher than .05, variables were not included in the model. The contributions of significant variables are also presented in the following table with Ln transformed and Ln normalized data ().
Table 9. Coefficients and statistical significance of the parameters.
Table 10. Average variable’s importance to the cost model output.
The contributions of each significance variable for the model output were determined in percentage. According to , the contribution percentage of project scope, project length, project width, terrain type, number of bridges, number of culverts, project location, contract duration and inflation rate were 2.274%, 14,101%, 7.184%, 2.887%, 6.895%, 16.689%, 19.471%, 21.721% and 8.779%; respectively. As a result contract duration, project location, number of culverts and project lengths are the variables which highly contribute as compared to others to the model. The contribution varies depending on how many input values there are for the parameters. Using the coefficient (), the model could be expressed by the following equation.
(2)
(2)
Where,
Y – Dependent variable (Actual project cost)
Xs – are project scope (x1), project length(x2), project width(x3), terrain type(x4), number of bridges(x5), number of culverts(x6), project location(x7), contract duration(x8) and inflation rate(x9) respectively and are the significance independent variables.
B – Intercept/constant
bs– b1, b2, b3, b4, b5, b6, b7, b8, b9 are the slope (coefficients of the independent variables) and,
ϵ – Residual (error)
From the results of the regression analysis, the Ln multiple regression model is developed based on the coefficients values taken from . Since the entire x values were converted to natural logarithm (Ln).
(3)
(3)
Table 11. Coefficient and probability (P) value of the variables.
Based on the result indicated in , EquationEquation (3)(3)
(3) was developed by substituting values of the constant itself and the coefficients of xs(x1, x2, x3, x4—x9) and x10, x11…x16 were removed from the system due to non-significance result.
4.3.4.1. Interpreting linear regression coefficients
Based on the coefficients (), the slope measures the strength of its relationship with the dependent variable. It is interpreted as the size of the average difference in the dependent variable that corresponds with a one-unit difference in the independent variables. The constant, or intercept term for the line of best fit, when all Xs are = 0, it is 19.506. The coefficients for; number of bridges, number of culverts, project location and inflation rate are positive: areas with more values of these variables tend to be positively associated with project cost (dependent variable). And project scope, project length, project width, terrain type and contract duration are negative: area with less value these variables tend to be negatively associated with project cost. If both the positivity and negativity coefficient values of independent variables are simultaneously increases the value of dependent variable also increase and the reverse is true. From anova (), the p value was <.05 which is 0.0008. In general where, b1, b2–-.b9 (the coefficients), are telling us the estimated change in the average value of y as at a rate of a one unit change in x.
In , the overall performance of the model is summarized with correlation coefficient (R), coefficient of determination (R2) and adjusted (R2); 0.916, 0.838 and 0.694; respectively. The average standard error of the estimate is 0.238. The significance of an R square change (F change) is 5.829. The average statistical significance value of the model is 0.0008. The Durbin-Watson (autocorrelation in the residuals from a regression analysis) is 2.123.
Table 12. Model summary.
As indicated in , the minimum, maximum, mean and std. deviation value of the Ln transformed data were determined.
Table 13. Residuals statistics.
shows that, the variations between the actual and predicted value of the model and the residual (error) value were calculated by using appropriate formula. The total value is the summation of all eighteen samples and the average values is the average of all the samples. The converted values of natural logarithm were calculated by e20.8133, e20.86309 and e2.80 for actual, predicted and residual values; respectively. The evaluations of the predicted and residual values of the model were also explained under this section.
Table 14. Predicted and residual value of the model.
4.3.4.2. Model performance evaluation metrics
Metrics are statistical measures used to evaluate the proposed prediction model. According to the study of Meharie and Gariy (Citation2021), performance evaluation metrics are employed to assess the suitability of the model to fit the data. The proposed model was evaluated and the predictive accuracy was compared based on some metrics: R2, Mean Absolute Error (MAE) and Mean Absolute Percentage Error (MAPE). New data were collected to examine whether the regression model developed from the earlier data is still applicable for other projects or not. R Square (R2) for model performance evaluation and Mean Absolute Error (MAE), Mean Absolute Percentage Error (MAPE) are used for error calculation.
4.3.4.3. R square (R2)
As explained by Kumari and Yadav (Citation2018), R Square measures how much variability in dependent variable can be explained by the model. It is the square of the Correlation Coefficient(R) and that is why it is called R Square. R Square value is between 0 and 1 of which bigger value indicates a better fit between prediction and actual value. If the R2 value <0.5 weak fit, if it is within 0.5≤ R2 ≤0.8 moderately fit and if R2≥0.8 it is strongly fit.
(4)
(4)
The R2value of this model is ≥0.8 so it is strongly fit. Therefore the model has explained 83.8% of the original variability.
4.3.4.4. Validity test of the model
The following formulas were selected to determine the percentage of errors or validity of the model. MAE and MAPE are commonly used to calculate the differences between the actual and predicted value of the proposed model (Meharie & Gariy, Citation2021). MAE and MAPE were applied to determine the error of the developed model. MAE is the average of the absolute errors between the actual and the predicted values. It is taken from the sum of the absolute value of error and the formula is given below.
(5)
(5)
Value taken from .
Therefore, the MAE cannot be compared regression model’s performance for distinct categories of data. It is not suitable for applications where one wants to determine the error in percentage but it is simply the average value. The Mean Absolute Percentage Error (MAPE) is one of the most common metrics used to measure the forecasting accuracy of a model as given by the following formula.
(6)
(6)
Value taken from .
Where;
N - Sample size, (yi) - The actual data value and (ŷi) - The predicted data value. And the value 16.44% indicates the average errors of the model, but as the result of predictive validity test the error has a minimum value when the model is applied to a single project.
4.3.4.4.1. Predictive validity
As specified by Taherdoost (Citation2017), the capacity of one assessment tool to predict future performance in a particular activity or in another evaluation of the same construct is known as predictive validity. Project no. 19 & 20 were taken to test the validity of the model and the difference between the forecasted value and the actual value were predicted. The value of X and Y were taken from the new actual data (). The projects are indicated in .
Table 15. New actual data.
As indicated in , new Ln(xs) and Ln(y) value were substituted to EquationEquation (3)(3)
(3) and the results were determined in .
Table 16. Lnconverted values.
Table 17. Predictive validity test results summary table.
Based on the results of the above table the predictive performance of the model was tested. In average the results tell us that, 88.706% of the project cost is explained by the developed model with the average mean absolute percentage error (MAPE) of 11.294%. This result indicates that, the model may have an error value of ±11.294%; the maximum accept range of error is ±20% then, this result is below the limit which ensures its acceptability and the model can perform for future projects better within the minimum residual value.
5. Discussions, managerial implications and limitations
5.1. Discussion of results
The finding of the study discovered that; Since probability values of inflation rate, number of bridges, length and width of the road, contract duration, project type and scope, number of culverts, terrain type and project location were less than 5% (0.05) and selected as the most significance factors. Those factors were also identified as the most significant parameters that were needed for the development of the model. As indicated in , the contribution percentage of project scope, project length, project width, terrain type, number of bridges, number of culverts, project location, contract duration and inflation rate were 2.274%, 14,101%, 7.184%, 2.887%, 6.895%, 16.689%, 19.471%, 21.721% and 8.779%; respectively.
As a result contract duration, project location, number of culverts and project lengths are the variables which highly contribute as compared to others to the model. The contribution varies depending on how many input values there are for the parameters. The result of multiple regression analysis indicates that; the performance of the model was 83.8% with the mean absolute percentage error value of 16.44%. This finding can be supported by Lowe et al. (Citation2006) the best regression model is the log of cost backward model which gives an R2 of 0.661 and a mean absolute percentage error (MAPE) of 19.3%.
These results compare favorably with past research of Alemayehu (2014a) which has shown that, the coefficient of determination (R2) for the developed models is ranging from 0.65 to 0.98 which indicate that the predicted values from a forecast models fit with the real-life data. The values of the mean absolute percentage error (MAPE) of the developed regression models are ranging from ±16.3% for preliminary cost estimating and to ±38.9% for conceptual method of cost estimation.
5.2. Managerial implications
The study's conclusions have important implications for both project cost managers and the industry as a whole. The road project's cost estimators should be aware of the different budget sizes and how they affect the project's ability to meet its forecasting objectives. They should be aware that using proper and scientific prediction methods for cost assessment can greatly improve their budgeting choices and lower the likelihood of over- or underestimating key decision variables.
Once more, the research offers numerous significant practical implications for developing forecasting methods and policies for the business. The results of this study demonstrate that, while systematic project cost prediction is required. These models have been found to be the most effective method for the upkeep of the road by considering basic road parameters.
5.3. Limitations
In the course of conducting this research, some knowledge was gained, which reveals the limitation some information was discovered throughout the research process, which highlights the limitations of the approaches or procedures used in this study. Given this realization, it makes sense to list some approaches that could be taken in the future to broaden our understanding of road cost estimation. The lack of sufficient paperwork to obtain further information on road developments is the first shortcoming of the published study. Second, most of the time, when choosing the model's parameters, which were required a lot of processing.
In terms of the development of the historical database, this also increases the complexity and time required for the established model. Regression set theory is a popular research topic in the literature because there aren't many studies on the applied methodology of multiple regression approaches. As a result, the method is not limited to the knowledge subject. Another drawback of the suggested methodology is that it only employs a small number of variables and finished road projects to conduct the analysis.
6. Conclusions and remarks
This research aims to construct a prediction model for predicting the cost of Ethiopian Roads Authority Road projects. As the results show among sixteen cost influential factors of road project; inflation rate, project type, project scope, number of bridges and length are extremely affect the actual project cost. Width of road, contract duration, number of lanes and number of culverts were also identified as highly cost influential factors.
The objective of the study was focused on the development of mathematical model to predict the cost of road projects. According to the study's findings, the multiple regression results show that the model can execute at an efficiency of 83.8%. The average residual (error) of the model was 16.44% which is below 20%; which is acceptable. The validity of the model was also checked by predictive validity test with new data. In average the results tell us that, 88.706% of the project cost is explained by the developed model with the average mean absolute percentage error (MAPE) of 11.294%. Moreover, this approach provides a more accurate, systematic and rational decision-support tool. Finally, the findings of this study provide academicians and practitioners with insightful information on the introduction of estimation techniques in the construction industry to demonstrate its practicality would be the best area of investigation.
Disclosure statement
No potential conflict of interest was reported by the author(s).
Additional information
Notes on contributors

Sisay Birhanu Belete
Sisay Birhanu Belete is a lecturer at Assosa College of Engineering, Assosa University, P.O. Box 18, Assosa, Ethiopia. He received his MSc degree in Construction Engineering and Management from the University of Gondar, Ethiopia. His research interest includes Regression Modeling, Cost Prediction and Project Management
Meseret Getnet Meharie
Meseret Getnet Meharie, PhD, is a senior lecturer at Adama Science and Technology University, Adama, Ethiopia. He is an Assistant professor at Adama Science and Technology University, Adama, Ethiopia. His research interest includes Cost Estimation of Construction Projects, Maintenance management, Fuzzy AHP and Machine learning algorithm in predicting the cost of highway projects.
Girmay Getawa Ayalew
Girmay Getawa Ayalew is currently a lecturer at Woldia Institute of Technology, Woldia University, P.O. Box 400, Woldia, Ethiopia. He is former lecturer at Gondar Institute of Technology, University of Gondar, P.O. Box 196, Gondar, Ethiopia. He received his MSc degree in Construction Engineering and Management from the University of Gondar, Ethiopia. His research interest includes Fuzzy AHP, Performance Management and Maintenance management.
References
- Abera, M. A. B. (2016). Bridge and culvert hydraulics (HENG – 5203) lecture-01 : Introduction. U.S. Department of Transportation Federal Highway Administration.
- Adel, K., Elyamany, A., Belal, A. M., & Kotb, A. S. (2016). Developing parametric model for conceptual cost estimate of highway projects. International Journal of Engineering Science, 6(7), 1728–1734.
- Akinradewo, O., Aigbavboa, C., Oke, A. E., & Data, B. (2020). Road projects estimation: A review road projects estimation: A review. Proceedings of the International Conference on Industrial Engineering and Operations Management Dubai, UAE, 2108-2113.
- Akintoye, A. S. (1999, September 8). Factors influencing the project cost estimating decision [paper presentation]. CIB W55 & W65 Joint Triennial Symposium Customer Satisfaction : A Focus for Research & Practice. United Kingdom.
- Alemayehu, S. (2014a). Testing regression models to estimate costs of road construction projects. Jesuit Historical Institute In Africa(Jhia).
- Alemayehu, S. (2014b). Testing regression models to estimate cost of road construction projects of science in civil engineering (construction technology and management). Jesuit Historical Institute In Africa(Jhia).
- Andom, D. (2015). A thesis submitted to the School of Graduate Studies, Addis Ababa University Institute of Technology in partial fulfillment of the requirements for the degree of evaluation of the cost estimating system of building contractors in Addis Ababa. Institute of Technology.
- Attal, A. (2010, August). Development of neural network models for prediction of highway construction cost and project duration. Proceeding of the joint Conference on computing in Construction (JC3).
- Befkadu, A., Quezon, P. E. T., & Busier, M. (2016). Assessment on the effects of weather change on road construction planning at some selected projects in Addis Ababa and Oromia. International Journal of Scientific and Engineering Research.
- Chakraborty, D., Elzarka, H., & Bhatnagar, R. (2016). Generation of accurate weather files using a hybrid machine learning methodology for design and analysis of sustainable and resilient buildings. Sustainable Cities and Society, 24, 33–41. https://doi.org/10.1016/j.scs.2016.04.009
- De Bock, L. P., & Van den Bergh, H. (2004). Stainless steel slags in hydraulic bound mixtures for road construction, 2 case studies in Belgium. In International RILEM Conference on the Use of Recycled Materials in Building and Structures (pp. 1095–1104). Belgian Road Research Centre (BRRC).
- Dharwadkar, N. V, & Arage, S. S. (2018). Prediction and estimation of civil construction cost using linear regression and neural network. International Journal of Intelligent Systems Design and Computing, 2(1), 28–44. https://doi.org/10.1504/IJISDC.2018.092554
- Elmousalami, H. H. (2019). Intelligent methodology for project conceptual cost prediction. Heliyon, 5(5), e01625. https://doi.org/10.1016/j.heliyon.2019.e01625
- Farr, B. C. (2008). Designing qualitative research. Transformation: An International Journal of Holistic Mission Studies, 25(2–3), 165–166. https://doi.org/10.1177/026537880802500310
- Ferreira, J. C., & Patino, C. M. (2015). The p value. Jornal Brasileiro de Pneumologia, 41(5), 485. https://doi.org/10.1590/S1806-37132015000000215
- Gao, U. (2020, March). Cost estimating and assessment guide. U.S Government Accountability Office.
- Gidissa, D. (2017). Factors affecting cost and time performance of construction projects in Ethiopia (case of: public building projects in Addis Ababa). St. Mary’s University Institutional Repository.
- Gofhamodimo, C. M. (1999). Construction contracts. Procurement methods in Botswana. Sustainable Design and Construction in Africa.
- Hu, M., & Skibniewski, M. J. (2021). A review of building construction cost research: Current status, gaps and green buildings. Green Building & Construction Economics, 2, 1–17. https://doi.org/10.37256/gbce.212021768
- Irfan, M., Bilal, M., & Anastasopoulos, P. (2011). anticipated project cost, project type, and contract type planning-stage estimation of highway project duration on the basis of anticipated project cost, project type, and contract type. International Journal of Project Management, 29(1), 78–92. https://doi.org/10.1016/j.ijproman.2010.01.001
- Jamous, E. H. A. (2013). Parametric cost estimation of road projects using artificial neural networks. National Library of Canada.
- Juszczyk, M. (2020). Development of cost estimation models based on ANN ensembles and the SVM method. Civil and Environmental Engineering Reports, 30(3), 48–67. https://doi.org/10.2478/ceer-2020-0033
- Kaleem, S., Irfan, M., & Gabriel, H. F. (2014). Estimation of highway project duration at the planning stage and analysis of risk factors leading to time overrun. Second Transportation & Development Congress. https://doi.org/10.1061/9780784413586.059
- Kanchana, S., & Janani, S. (2018). A study on factors affecting estimation of construction project. International Research Journal of Engineering and Technology (IRJET), 05(11), 1154–1157.
- Kim, D. Y., Kim, B., & Han, S. H. (2008). Two-staged early cost estimation for highway construction projects. In ISARC 2008 - Proceedings from the 25th International Symposium on Automation and Robotics in Construction (pp. 490–495). Institute of Internet and Intelligent Technologies. https://doi.org/10.3846/isarc.20080626.490
- Kumari, K., & Yadav, S. (2018). Linear regression analysis study. Journal of the Practice of Cardiovascular Sciences, 4(1), 33. https://doi.org/10.4103/jpcs.jpcs_8_18
- Leung, M., Shan, Y., Chan, I., & Dongyu, C. (2011). Engineering, construction and architectural management article information. Engineering, Construction and Architectural Management, 18(3), 312–328. https://doi.org/10.1108/09699981111126205
- Liu, M., Le, Y., Hu, Y., Xia, B., Skitmore, M., & Gao, X. (2019). System dynamics modeling for construction management research: Critical review and future trends. Journal of Civil Engineering and Management, 25(8), 730–741. https://doi.org/10.3846/jcem.2019.10518
- Lowe, D. J., Emsley, M. W., & Harding, A. (2006). Predicting construction cost using multiple regression techniques. Journal of Construction Engineering and Management, 132(7), 750–758. https://doi.org/10.1061/(asce)0733-9364(2006)132:7(750)
- Luong, D. L., Tran, D. H., & Nguyen, P. T. (2021). Optimizing multi-mode time-cost-quality trade-off of construction project using opposition multiple objective difference evolution. International Journal of Construction Management, 21(3), 271–283. https://doi.org/10.1080/15623599.2018.1526630
- Mahde Abd, A., Adnan Jasim, N., & Saleh Naseef, F. (2019). Developing a prediction model to predict the construction project cost by using multiple linear regression technique. Indian Journal of Science and Technology, 12(7), 1–6. https://doi.org/10.17485/ijst/2019/v12i7/138685
- Mcenroe, B. M. (2007, February). Final report sizing of highway culverts and bridges: A historical review of methods and criteria. Kansas Department of Transportation.
- Meharie, M. G., & Gariy, Z. A. (2021). Application of stacking ensemble machine learning algorithm in predicting the cost of highway construction projects. Engineering, Construction and Architectural Management, 29(7), pp. 2836–2853. https://doi.org/10.1108/ECAM-02-2020-0128
- Meharie, M. G., & Shaik, N. (2020). Predicting highway construction costs : Comparison of the performance of random forest, neural network and support vector machine models. Journal of Soft Computing in Civil Engineering, 2, 103–112.
- Mohammed, F., Al-Zwainy, S., & Hadhal, N. T. (2016). Building a mathematical model for predicting the cost of the communication towers projects using multifactor linear regression technique. International Journal of Construction Engineering and Management, 5(1), 25–29. http://journal.sapub.org/ijcem
- Mumela, M. E., Christine, N., Limited, R. E., & Box, P. O. (2017). Effect of contractor knowledge and experience on cost estimation in plant infrastructure projects in Kenya. International Journal of Science and Research (IJSR), 6(6), 2460–2466. https://doi.org/10.21275/ART20174864
- Narbaev, T., Hazir, Ö., Khamitova, B., & Talgat, S. (2023). A machine learning study to improve the reliability of project cost estimates. International Journal of Production Research, 61, 1–18. https://doi.org/10.1080/00207543.2023.2262051
- Operations, R. (2020, December). Cost estimating manual for projects. Washington State Department of Transportation’s (WSDOT).
- Profile, S. E. E. (2015). Manipulation theory of inflation: A research study on components of general price rise manipulation theory of inflation. PRAGATI: Journal of Indian Economy, 1(2), 66–82. https://doi.org/10.17492/pragati.v1i2.2507
- Ravindran, H. (2018). Cost prediction model for building construction projects. International Journal of Innovative Science and Research Technology, 3(4), 448–449.
- Saeidlou, S., & Ghadiminia, N. (2023). A construction cost estimation framework using DNN and validation unit. Building Research and Information, 0(0), 1–11. https://doi.org/10.1080/09613218.2023.2196388
- Sarode, G. C., & Chandgude, S. E. (2020). A review on cost prediction analysis of construction project using ANN model. International Journal of Innovative Research in Science, Engineering and Technology (IJIRSET), 9(4), 1516–1524.
- Shaban, A., Khawlie, M., Kheir, R. B., & Abdallah, C. (2017). Assessment of road instability along a typical mountainous road using GIS and aerial photos, Lebanon ± eastern Mediterranean. Bulletin of the International Association of Engineering Geology,60, 93–101. https://doi.org/10.1007/BF02595036
- Son, P. V. H., Duy, N. H. C., & Dat, P. T. (2021). Optimization of construction material cost through logistics planning model of dragonfly algorithm—Particle swarm optimization. KSCE Journal of Civil Engineering, 25(7), 2350–2359. https://doi.org/10.1007/s12205-021-1427-5
- Son, P. V. H., & Khoi, L. N. Q. (2023a). Multi-modal optimization for time–cost–quality–work continuity trade-off in construction projects using a hybrid based on SMA and M&C. Asian Journal of Civil Engineering, 147, 110–775. https://doi.org/10.1007/s42107-023-00880-2
- Son, P. V. H., & Nguyen Dang, N. T. (2023). Optimizing time and cost simultaneously in projects with multi-verse optimizer. Asian Journal of Civil Engineering, 24(7), 2443–2449. https://doi.org/10.1007/s42107-023-00652-y
- Sonmez, R., & Ontepeli, B. (2010). Predesign cost estimation of urban railway projects with parametric modeling. Journal of Civil Engineering and Management, 15(4), 405–409. https://doi.org/10.3846/1392-3730.2009.15.405-409
- Swei, O., Gregory, J., & Kirchain, R. (2017). Construction cost estimation: A parametric approach for better estimates of expected cost and variation. Transportation Research Part B: Methodological, 101, 295–305. https://doi.org/10.1016/j.trb.2017.04.013
- Tadesse, N., & Dinku, A. (2017). Conceptual cost estimation of road projects in Ethiopia using neural networks. Journal of the European Economic Association, 35, 17–29.
- Taherdoost, H. (2017). Validity and reliability of the research instrument; how to test the validation of a questionnaire/survey in a research. International Journal of Academic Research in Management (IJARM). https://doi.org/10.2139/ssrn.3205040
- Tayefeh Hashemi, S., Ebadati, O. M., & Kaur, H. (2020). Cost estimation and prediction in construction projects: A systematic review on machine learning techniques. SN Applied Sciences, 2(10), 1–27. https://doi.org/10.1007/s42452-020-03497-1
- Thomas, N., & Thomas, A. V. (2017). Regression modelling for prediction of construction cost and duration. Applied Mechanics and Materials, 857, 195–199. https://doi.org/10.4028/www.scientific.net/AMM.857.195
- Velumani, P., & Nampoothiri, N. V. N. (2019). Analysis of construction cost prediction studies-global perspective. International Review of Applied Sciences and Engineering, 10(3), 275–281. https://doi.org/10.1556/1848.2019.0032
- Vijay, B. G., Rudraswamy, C. K., & Khatawkar, R. (2020). Study on impact of gradient on capacity of two lane undivided national highways. Bannerghatta Biological Park.
- Wang, Y., Ning, X., Zhen, D., Yong, W., & Zhang, H. (2023). Research on construction project cost prediction model based on recurrent neural network. SHS Web of Conferences, 170, 02009. https://doi.org/10.1051/shsconf/202317002009
- Williams, R. C., & Songer, A. D. (2008). The development of mathematical models for preliminary prediction of highway construction duration. (Doctoral dissertation, Virginia Tech).
- Xiong, B. (2016). Improving cost estimation performance: An investigation of prediction technique and person - environment interaction (Doctoral dissertation, Queensland University of Technology).
- Zuber, S., Nawi, M., & Abdul Nifa, B. (2018, December). An overview of project delivery methods in construction industry. International Journal of Supply Chain Management (IJSCM).