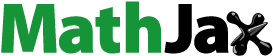
Abstract
This study looked into the quality of groundwater in Hawassa City and its periphery as a baseline survey for the ‘Assessment of Feasibility of Managed Aquifer Recharge’. Water quality was assessed using four water quality indices. Weighted arithmetic Water Quality Index (WA-WQI), Synthetic Pollution Index (SPI), and Entropy Weighted Water Quality Index (EWQI) were used for domestic water quality assessment. Whereas, Overall Irrigation Water Quality Index (IWQ), was used for irrigation water quality assessment. Accordingly, WA-WQI results showed that 6.25 and 93.75% of the water samples were very poor and poor quality status, respectively, making them unsafe for consumption. However, according to SPI, every water sample was unsuitable for usage as potable water. According to the EWQI model, the water quality status of 22.92, 58.33, and 18.75% of the water samples was average, poor, and extremely poor, respectively. Of the three models used for assessing water quality, SPI is the robust. The primary parameters in both models used to estimate the water quality statuses (68–89%) were F–, K+, BOD5, COD, and HCO3–. The Overall IWQ score indicated fair and moderate water quality levels (6.25 and 93.75%), respectively. Sodicity and toxicity hazard classes shared (47–80%) in determining the irrigation water quality status. This result indicates that the aquifer of the city is being contaminated. This requires enforcing strict standards in septic tank construction. Additionally, increasing groundwater recharge from precipitation sources during the rainy seasons to further dilute the concentration and increase aquifer volume is required.
REVIEWING EDITOR:
1. Introduction
Approximately 71% of the earth’s surface is covered by water, giving the impression that there are abundant water resources. However, freshwater, which accounts for only 3% of water resources but is essential for food production, sanitization, manufacturing, and power generation, is in short supply (Rashid et al., Citation2019). Additionally, there is an unequal distribution of the surface water resource in space (Biswas & Tortajada, Citation2019; Brhane, Citation2018).
Though surface water is mostly used for different purposes due to its increasing limitations, groundwater is increasingly being used for home, industrial, and agricultural purposes. Groundwater resource development is becoming increasingly important globally due to the advantages it has over surface water resources in terms of location, affordability, and quality (Hasan et al., Citation2018). Aquifers, however, are being depleted in quantity and/or quality as a result of population growth, industrialization, and urbanization together with better quality of life (Foster, Citation2020).
Even though soils and rocks are the primary sources of groundwater’s soluble components, human activities in areas where groundwater is present can introduce a variety of natural and man-made particulate matter, which can contaminate it (Dottridge et al., Citation2021; Jagaba et al., Citation2020; Shukla & Saxena, Citation2020). As a result, groundwater management and use are becoming critically impacted by the rising danger to its quality (Dottridge et al., Citation2021). Thus, water quality monitoring is a continuous process to assess and manage unfavorable changes in water quality that necessitate in locations with high population density and increased human activity, this is of utmost importance. In the analysis of data from 10 cities, Foster noted that there was a significant level of reliance on groundwater for urban water supply (Foster, Citation2020). Sustainable water supply in places with limited groundwater resources became essential as a result of substantial localized groundwater depletion and saline intrusion into the aquifers as well. Additionally, poorly managed urban sanitation will worsen groundwater quality; because of this fact, groundwater should be rigorously and regularly monitored in urban areas to mitigate the risk posed by pollution (Foster, Citation2020).
Water quality can be assessed with different methods. The conventional method compares each parameter value with WHO or a national standard value, which makes it hard to comprehend the values of a parameter set. Instead, a composite model called the Water Quality Index (WQI) is introduced to assess water quality. This model displays the combined effect of several water quality parameters and conveys water quality information with dimensionless numbers to the general public and legislative decision-makers clearly and understandably for action. There are many variants of WQI models, namely; started from Horton (1960) inception, NSF (1970), Dinius index (1972), Dojildo index (1994), British Colombia index (1995), Malaysian Index (2007), West Java Index (2017) and many more, varying with model structure, number of parameters used and parameter weighting, and index aggregation (Anonna et al., Citation2022; Kachroud et al., Citation2019; Matta et al., Citation2020; Son et al., Citation2020; Uddin et al., Citation2021; Wang et al., Citation2023).
Despite being close to a lake, Hawassa City primarily relies on groundwater for its water needs. Groundwater sources provide more than 80% of the city’s water supply. During the survey of water sources, it was discovered that various business centers, large public institutions, and private homes all use groundwater supplies from hand-dug wells or boreholes for needs other than potable water. Additionally, commercial irrigation farms that use a lot of groundwater are located on the eastern edge of the city.
Water wells with extremely high productivity and quality are located in the eastern and south-eastern parts of Hawassa city, which is in danger of contamination from various contaminants (JICA, Citation2012; Tadesse & Zenaw, Citation2003; Zigde & Tsegaye, Citation2019a). Due to the city’s rapid growth in this direction and the presence of unimproved sanitary facilities; in addition, a variety of industries of various sizes and types discharge their effluents into nearby streams (Berehanu et al., Citation2015; Firew et al., Citation2018; Zigde & Tsegaye, Citation2019b) thus, the groundwater in this area needs to be better protected from pollution (Lencha et al., Citation2021). Even though there are fewer wells in the southern area of the city, it is still believed that there could be contamination. The objective of this study was to investigate the quality status of the city’s groundwater to create insight into whether groundwater can be used for various planned purposes. From the authors’ knowledge, there are no comprehensive documented manuscripts about groundwater quality analysis using WQI models for Hawassa city aquifers, except some on surface water (Teshome, Citation2020).
In this study, two objective parameter weightage models, the Entropy Weightage Water Quality Index (EWQI) (Zhe et al., Citation2021) and the Synthetic Pollution Index (SPI) (Solangi et al., Citation2019) and one subjective parameter weightage model, the Weighted Arithmetic Water Quality Index (WA-WQI) (Solangi et al., Citation2019) were selected to evaluate water quality for domestic use. For irrigation water quality assessment, the Overall Irrigation Water Quality Index (Overall IWQI) (Zhang et al., Citation2021) was selected. These models were used in evaluating water quality in India (Kumar & Augustine, Citation2022), China (Zhang et al., Citation2021), Pakistan (Iqbal et al., Citation2021), Iran (Hosseininia & Hassanzadeh, Citation2023), and many other countries. Water samples from 48 wells were evaluated for 18 physicochemical parameters (TDS, EC, pH, BOD5, COD, K+, Na+, Ca2+, Mg2+, Fe3+, NO2−, NO3−, SO42−, Cl−, HCO3−, F−, and TH)using the above water quality indices. Using the Inverse Distance Weightage (IDW) method, the spatial distribution of water quality metrics is mapped in Arc GIS 10.5 software.
The output of this study provides important information to all stakeholders (water managers and users) (1) regarding the dangers associated with the groundwater that is currently available and (2) providing reasonable alternatives for groundwater monitoring, protection, and augmentation.
2. Materials and methods
2.1. Study area description
Hawassa City is located in the Lake Hawassa Watershed in the Central Rift Valley of Ethiopia. It is located in the region of longitudes 38°28′–38°33′ E and latitudes 6°58′–7°05′ N (). The terrain of the region is made up of flat plains and a few volcano mountains, with elevations ranging from 1680 to 2027 m a.s.l.
The wet and humid months are from April to October. The major rainy season lasts from July to September, and the average annual rainfall is around 954.9 mm (NMA, n.d.). The highest monthly temperatures (29–29.2 °C) are recorded in February and March, while they reach their lowest values (23.6–24 °C) in July and August (NMA, n.d.). 1599 mm/year is the potential evapotranspiration (PET). According to NMA (n.d.), the minimum PET is 102 mm in July and the highest PET is 173 mm in December (NMA, n.d.).
The two main aquifers in the area are the overlaying volcano-lacustrine sediments and fractured and jointed ignimbrites. Sands, tuff, and pumice are interlayered with clay aquitard in volcano lacustrine sediment aquifers. The lacustrine material is between 40 and 60 m thick. Boreholes excavated in the lacustrine sediments frequently reveal multiple aquifers divided by clay strata (Halcrow Group Limited and GIRD Consultants, 2009). The most extensive and generally high-quality groundwater aquifer in the main Ethiopian Rift is found beneath faulted tertiary ignimbrites (Halcrow Group Limited and GIRD Consultants, 2009; Hulluka et al., Citation2023).
2.2. Sampling and measurements
Before choosing sampling stations, the groundwater abstraction points and daily water abstraction rate were inventoried. With the assistance of the city water supply staff, the inventory was completed in each sub-city and kebele. Forty-eight sampling sites, hand-dug wells [with (HP) and without (HD) pumps], and boreholes (BH) were chosen for this study. The selection criteria included the quantity and kind of wells that were available, the rate of water use, and the settlement patterns of individuals in various parts of the city ().
During the inventory of water wells, it was discovered that numerous hand-dug wells were located in the town’s rural sub-city and in locations where the depth of the water table was close to the surface. Even though there were other wells nearby, taking a few samples there was thought to be sufficient. However, it was important to sample water in places with deep wells; these wells were boreholes, and samples were obtained from them. Additionally, mechanized farms, high-water-using industries, and institutions that provide public services were included as sampling places.
During the dry season of the year, from February to March 2021, water sampling was done at specific locations. GPS (GARMIN 12 h) was used to capture the coordinates and altitude of the sampling stations during the water sampling process. One liter polyethene plastic bottles were used to collect the sample, and glass bottles were utilized for the biochemical oxygen demand analysis. To prevent contamination, the bottles were first cleansed with distilled water and then rinsed three times with the sample water during collection. Following the pumping of water from a hand-dug well or borehole, sample water was collected. In the instance of a hand-dug well without a pump, samples were taken following a sizable water removal. The water samples were brought to the laboratory at Hawassa University after being securely labeled, handled, and stored in an ice box to be analyzed physico-chemically. Temperature, EC, TDS, pH, and turbidity were all monitored on-site.
The American Public Health Association’s criteria for the evaluation of water and wastewater were followed in the collection, handling, preservation, and treatment of the water samples (APHA, 2017). lists the variables affecting water quality, how they are analyzed, and the tools that were employed. The Palintest photometer test was performed using the required reagent tablets, following the instrument manufacturer’s instructions.
Table 1. Quality analysis methods and Instruments used.
2.3. Water quality indices
The current groundwater quality of Hawassa City was examined and evaluated by using the Weighted Arithmetic Water Quality Index (WA-WQI) (Chabuk et al., Citation2020), Synthetic Pollution Index (SPI) (Solangi et al., Citation2019), and Entropy Water Quality Index (EWQI) (Zhe et al., Citation2021) for domestic, and Overall Irrigation Water Quality Index (Overall IWQIndex) (Islam & Mostafa, Citation2022) for irrigation uses against WHO (WHO, Citation2018) and FAO (FAO, Citation1996) standards.
These models take into account crucial physiochemical water quality factors that affect human health when used for domestic purposes as well as effects on soil health and crop yield when used for irrigation. The main distinction between the three indices, WQI, SPI, and EWQI, in the evaluation of drinking water quality is that the weight of a particular parameter by WA-WQI is based on the consensus of experts (subjective), whereas in SPI proportionality constant is determined based on the permissible concentration levels of all parameters considered (objective). In EWQI, however, weight is based on the degree of differentiation of indicators related to the dispersion condition of observation data (objective) (Zhe et al., Citation2021). The higher discrimination degree of the parameter has a larger weight in EWQI. In addition, in SPI, all parameters considered should have permissible limits stated by a country’s or WHO standard guideline. As a result, SPI's and EWQI’s assessments of water quality are more rigorous than WA-WQI's. In assessing the quality of irrigation water, IWQidex is utilized because, unlike other irrigation water quality assessing indices, it takes into account all kinds of soil and crop hazard classes.
Using the Inverse Distance Weightage (IDW) method, the spatial distribution of water quality metrics and sampling point locations are mapped in Arc GIS 10.5 software. The steps taken to use the water quality indices for irrigation and home usage are discussed in the next section.
2.3.1. Water quality index for domestic use
According to Kachroud et al. (Citation2019) and Sutadian et al. (Citation2018), there are four steps in the Weighted Arithmetic Water Quality Index (WQI) model. They are as follows:
(i) Relative weight calculation (Wi):
According to the assigned weight of a parameter relevant to the total weights of all the parameters used in the quality analysis, a parameter’s relative weight is computed using (EquationEquation 1(1)
(1) ). Supplementary Table 1 presents the parameters used, the weight of each parameter, and the standard limit value.
(1)
(1)
Wi denotes a parameter’s weight, n is the number of parameters utilized in the analysis, and wi denotes the relative weight of each parameter.
(ii) Quality rating (Qi)
The current measured value of a parameter is compared to the allowable value of that parameter in the WHO standard or other chosen standards to get the quality rating of that particular parameter using (EquationEquation 2(2)
(2) ).
(2)
(2)
Where si is the limit value (mg/L) of the corresponding chemical parameter in the guidelines and ci is the concentration (mg/L) of parameters in the sample.
(iii) Determination of the sub-index of a parameter (SIi)
A parameter’s sub-index is created by adding the parameter’s relative weight and quality rating using (EquationEquation 3(3)
(3) ).
(3)
(3)
Where Wi is the relative weight, SIi is the sub-index of a parameter, and Qi is the rating based on the concentration of its parameter.
(iv) Water quality index (WQI) of a single sample
By adding up all of the parameter sub-indices used in the analysis, the water quality index of a specific sample is determined using (EquationEquation 4(4)
(4) ).
(4)
(4)
Where n is the number of parameters. A parameter’s SIi is its sub-index.
Based on the categories listed in , the water quality status was classified.
Table 2. Classification system of WQI (Sutadian et al., Citation2018).
The synthetic pollution index (SPI), which utilized three distinct processes, was the other index used to measure the water quality.
Proportionality constant (K) determination:
Using (EquationEquation 5(5)
(5) ), a constant value to be utilized for all parameters is established.
(5)
(5)
Where n is the number of parameters and Si is the allowed level for the ith parameter.
Weight coefficient calculation (Wi) for a particular parameter is calculated using (EquationEquation 6
(6)
(6) ),
(6)
(6)
Where si is the parameter’s allowable level, K is the proportionality constant.
Finally, the Synthetic Pollution Index (SPI) value for a water point is calculated using (EquationEquation 7
(7)
(7) ),
(7)
(7)
Wi is the weight coefficient of the parameter, and Ci is the observed concentration for each of the identified physicochemical water quality indicators.
Water pollution status was classified based on categorization range.
Table 3. Classification system of SPI (Solangi et al., Citation2019).
The entropy water quality index (EWQI) utilizes the following steps:
The value normalization function is done by using (EquationEquation 8
(8)
(8) )
(8)
(8)
Where yij is the normalized value of the ith sample and jth parameter, Xmax is the maximum value of a jth parameter, Xij is the concentration value of the ith sample and jth parameter and Xmin is the minimum value of the jth parameter.
Ratio of Index value calculation is done by using (EquationEquation 9
(9)
(9) )
(9)
(9)
Where Pij is the ratio of index value for the ith sample and jth parameter, yij is the normalized value of the ith sample and jth parameter, sum of the normalized value for the jth parameter.
Information entropy calculation is done by using (EquationEquation 10
(10)
(10) )
(10)
(10)
Where ej is information entropy for the jth parameter, Pij is the ratio index of sample i and parameter j, ln(Pij) is the natural logarithm for the ratio index and ln(m) is the natural logarithm of the number of sampling points.
Entropy weight calculation is done by using (EquationEquation 11
(11)
(11) )
(11)
(11)
Where wj is the entropy weight for the jth parameter, ej is the information entropy for the jth parameter.
Quality rating calculation is done by using (EquationEquation 12
(12)
(12) )
(12)
(12)
Where qij is the quality rating of a sample for a parameter, Xij is the concentration of a parameter for a sample, and Sj standard limits of a parameter.
Entropy water quality index calculation is done by using (EquationEquation 13
(13)
(13) )
(13)
(13)
Where EWQIi is the entropy water quality index for sample i, wj is the entropy weight for parameter j, and qij is the quality rating of parameter j of sample i ().
Table 4. Classification system of EWQI (Nguyen et al., Citation2021).
2.3.2. Water quality index for irrigation
In this study, the groundwater supply for irrigation in Hawassa City was assessed using the overall Irrigation Water Quality Index (IWQindex), based on Islam and Mostafa’s assumptions (Islam & Mostafa, Citation2022). This Index model was chosen because it has a clear setup for soil hazard classes with reasonable scores, parameters included in each hazard class, and their rating ( and Supplementary Table 2) (Islam & Mostafa, Citation2022). In particular, it incorporates the most popular irrigation water quality indices currently in use (Supplementary Table 3) (Islam & Mostafa, Citation2022). The following steps and practices are comparable to the arithmetic weight water quality index. Selecting parameters within each hazard class, weighting each class, sub-indexing each class, and finally averaging the sub-indices across all classes are the steps.
Table 5. Hazard classes, their score and weight with included parameters and indices (Islam & Mostafa, Citation2022).
The procedures and formulas to be used are as follows:
Calculation of the weight of ith hazard class is done by using (EquationEquation 14
(14)
(14) )
(14)
(14)
Where Wi is the weight of the ith hazard class, s is the hazard class score, and Si is the sum of all hazard class scores
Calculating the quality rating of the ith parameter is done by using (EquationEquation 15
(15)
(15) )
(15)
(15)
Where Qi is the ith parameter’s quality rating, Ci is its measured value, Cmax is its maximum value at the lowest rating (r = 1), Cmin is its minimum value at the highest rating (r = 3), and ri is its rating value.
Sub-index calculation of the hazard class is done by using (EquationEquation 16
(16)
(16) )
(16)
(16)
Where Qi is a parameter’s quality rating, Si is a parameter’s sub-index value, Sx is a parameter’s score, and n is the number of parameters in the specified hazard class
Aggregation of sub-index is calculated using (EquationEquation 17
(17)
(17) )
(17)
(17)
Where Si is the sub-index of the ith hazard class and Wi is the ith hazard class’s weight factor.
The category shown in was used to establish the status of the given sample.
Table 6. Irrigation water quality classification (Islam & Mostafa, Citation2022).
3. Result and discussion
3.1. Evaluation of the water quality parameters
Both natural and man-made factors have a significant impact on groundwater chemistry (Gong et al., Citation2023; Meena, Citation2022). Industrial effluents and sewer system discharges, particularly in urban areas, make up the biggest portion of the aquifer system’s contamination.
Selected parameter maps that potentially depict concentration shift direction or location are shown in Supplementary Figure 1. In this figure, pH, BOD5, and SO4 are higher in hand-dug well samples, which is an indicator of contamination. provides a summary of the parameter values. The result indicated that the groundwater is slightly alkaline, with pH values ranging from 7.65 to 8.82 and a mean value of 8.1. Of all sample wells, 83.3% meet or exceed WHO/EPA drinking water criteria. According to Kachroud et al. (Citation2019), electrical conductivity and total dissolved solids are important criteria to determine if water is suitable for irrigation and residential use. With a mean value of 858.51 mg/L and 1725.72 S/Cm, respectively, TDS and EC values varied from 175.7 to 2522 mg/L and from 395 to 5044 S/Cm. According to WHO/EPA standard values, the sampled wells had a quality of residential use at 77.1% for EC and 87.5% for TDS. The increased EC and TDS values in HD12, HD23, HD30, HP34, and HP35 could be caused by surface contamination or septic contamination from pit latrines.
Table 7. Water quality parameters.
Chemically, the main cations Na+ and K+ had mean values of 193.8 and 50.73 mg/L, respectively, with values ranging from 105 to 460 mg/L and from 11 to 172 mg/L, respectively. Ca2+ and Mg2+ had mean values of 67.58 and 17.15 mg/L, respectively, and ranged from 4 to 200 mg/L and from 0.1 to 46 mg/L, respectively. All sampling wells for Ca2+ and Mg2+ fall below WHO/EPA quality criteria, however 93.75% of K+ sampling wells and 31.25% of Na+ sampling wells did not meet those standards. The cause of this could be the localized dissolution of volcanic rock minerals or the ionic exchange of alkali metals with alkaline earth metals. Scholler CAI-I and CAI-II (Chloro-Alkaline) indices were used to validate this (Haji et al., Citation2018).
Major anions HCO3− and Cl− had average values of 357.9 and 26.39 mg/L, respectively, with values ranging from 15.2 to 640 mg/L and 13.2 to 38 mg/L, respectively. SO42− and F− had values that range from 3 to 150 mg/L and from 2.6 to 8.6 mg/L with average values of 38.83 and 5.21 mg/L, respectively. Water quality for domestic use was significantly impacted by 100% of sampling wells for F− and 91.66% for HCO3− having values over WHO/EPA requirements; however, all wells for SO42− and Cl− had values below the standards, allowing the water to be used for domestic purposes. Studies conducted in the Rift Valley locations revealed that lacustrine aquifers, in particular, have high F− continent levels of groundwater (Alcaine et al., Citation2020; Ayalew, Citation2020; Ayenew, Citation2008; Haji et al., Citation2018; Ligate et al., Citation2021; Mwiathi et al., Citation2022; Rango et al., Citation2009). Na+ and HCO3− ions were high and Ca2+ ion was low in this area which is also associated with increasing Fluoride concentration as stated by Rashid et al. (Citation2018).
Nitrate (NO3−) concentration ranged from 1.28 to 14.4 mg/L, with an average value of 3.38 mg/L. Sampling wells (2%) for NO3− had readings that are higher than the WHO/EPA standard. The Hawassa University main campus’s BH20, which is located below the chemistry and environmental engineering laboratories and other facilities, had the highest Nitrate readings.
The results showed that all sampling wells are above the WHO/EPA standard values 100% for F−, BOD5, and COD; 91.66% for HCO3− and 93.75% for K+, which unquestionably has a severe impact on the water quality for residential applications. Natural, industrial, and domestic sewage contaminations are the cause of this high value (Lencha et al., Citation2021; Zhang et al., Citation2016). In terms of water hardness 20.83, 52.08, 18.75, and 8.33% of the sampling wells contained soft, moderately hard, hard, and extremely hard water, respectively. Temporary hardness is the sort of hardness present in this area. Water hardness spatial distribution in the Hawassa area is depicted in . In the Addis Ababa and Mesrak sub-cities, as well as in the vicinity of Dato (in the Hawella Tulla sub-city), very hard water predominates. Overall, hard water dominates in the research area.
The average cation concentrations were in the following order: Na+ > Ca2+ > Mg2+, and the average anion concentrations were HCO3− > SO42− > Cl−. Scholler Indices (Ministry of Water, Irrigation and Electricity, Citation2018) CAI-I and CAI-II were used to measure the cation exchange process that frequently modifies the chemistry of groundwater. With the provided indices, all sampling points had negative values, indicating that the Ca2+ and Mg2+ ions in the water solution have been replaced by Na+ and K+ ions.
Fe3+ concentrations were higher in the town’s northeast, where the Tabor Ceramic Factory is located, as can be seen in . Iron is an essential element for health and is needed for hemoglobin and some enzymes, but a higher consumption rate can cause vomiting and diarrhea and, if worsened, has an effect on the nervous system, liver, and kidney (Rahman et al., Citation2019; Rashid et al., Citation2021). Iron is highly associated with clay minerals, and clay soil is a major input in ceramics production. Leachate from ceramic input materials and waste products could be the source of the high iron content in this area. Due to strong evaporation at shallow depths close to the lake, EC was higher along the lake’s length () and higher pH downstream of the industrial zone () and solid waste disposal facility. These locations are very vulnerable to pollution ().
Figure 4. Selected parameters map showing suitability distribution. (a) Iron, (b) pH, (c) electrical conductivity, and (d) fluoride.

To differentiate the quality status of well types, the group mean of different parameters of the well types was calculated. The relatively highest values in BOD5, COD, SO42−, NO2−, and pH were observed in hand-dug wells without pumps. Accordingly, the second higher value was in hand-dug wells with pumps and the lowest was in boreholes. These values serve as indicators for both organic and inorganic pollution. Although hand-dug wells without a pump experience the greatest quality deterioration, the findings showed that anthropogenic sources have an impact on all types of wells.
3.2. Results of WQI, SPI, and EWQI
According to the WQI study of the sampled wells in and around Hawassa city, 89.47% of hand-dug wells without pumps, 90.9% of hand-dug wells with pumps, and 100% of boreholes were of poor quality ( and ). Hand-dug wells 9.1% with pumps and 10.53% without pumps had very poor water quality. In all situations, the five parameters F−, K+, COD, BOD5, and HCO3− affected 70–90% of HD, 73–92% of HP, and 63–88% of BH water quality status. Even without taking into account the diameter of the wells, the relative depth of HD wells from the surface is shorter than that of BH; the likelihood of water contamination near the surface is higher than water at a certain depth, in this study however, pollution was displayed in BH. Most of the time, human sources, such as leaking industrial wastewater into the ground and public and private sewerage systems are to blame for these indicated excessive values in BH.
Table 8. WQI, SPI, and EWQI values of sampling wells and highest contributing parameters.
According to the Synthetic Pollution Index (SPI) results ( and ), the water quality at all sample stations was unfit for residential use. Five indicators, including F−, K+, COD, BOD5, and HCO3−, had significantly contributed to pollution, similar to WQI situations. The contribution ranged from 66 to 88% for boreholes, 71 to 89% for hand-dug wells with pumps, and 69 to 89% for hand-dug wells without pumps.
Based on the Entropy weighting Water Quality Index (EWQI) result ( and ) 21.05, 9.09, and 33.33% of water samples from HD, HP, and BH, respectively, were average in quality. Whereas, 52.63, 63.64, and 61.11% of samples from HD, HP, and BH, respectively, were poor in quality. Extremely poor quality water was found in 26.32, 27.27, and 5.56% of HD, HP and BH wells, respectively. This result showed water wells at shallow depths (HD and HP) are more contaminated than boreholes at a deeper depth. Spatially downstream of the Industry zone and solid waste disposal site, as well as part of the old city’s water sample sources, they were more contaminated than the rest of the city. This is because of contamination from anthropogenic sources like leakage from sewerage systems, septic tank leakage, and other surface contaminants. The contributions of the five parameters in EWQI status, like the other two models, were 70–83, 82, and 74–81% for HD, HP, and BH, respectively for average water quality; 74–85, 75–89, and 75–85% for HD, HP, and BH, respectively, for poor quality; and 83–89, 82–86, and 85% for HD, HP, and BH, respectively for extremely poor quality. From the applied water quality models, the SPI model was more stringent than WA-WQI and EWQI models.
3.3. Water quality index (WQI), synthetic pollution index (SPI), and entropy water quality index (EWQI) relationships
Regression analysis was used to create nine equations based on the WQI, SPI, and EWQI values. Each equation corresponds to one of the three well types: borehole (BH), hand-dug well without a pump (HD), and hand-dug well with a pump (HP). All had regression coefficients that are larger than 0.9. The relationships between models and their regression functions are presented in Supplementary Figure 2. Supplementary Table 4 showed detailed quality values for all sampling points for all used models.
3.4. Overall irrigation water quality index (overall IWQIndex)
According to the model developed by Islam and Mostafa (Hulluka et al., Citation2023), the appropriateness of the available groundwater for irrigation was predicted using 10 parameters and six well-known irrigation water quality indices. presented summarized final results, while showed the results of a separate analysis of the irrigation water quality indices. The detailed status values for the irrigation water quality models for all samples were presented in Supplementary Table 5.
Table 9. Overall irrigation water quality index values of sampling wells and highest contributing hazard classes.
makes it evident that the water quality status varies since each index uses a distinct set of evaluation criteria. To make decisions easier, it is consequently somehow necessary to integrate various indices to create one.
In this study, EC was taken into account while calculating the salinity hazard class. Only one of the two parameters should be taken into account if the correlation coefficient between EC and TDS is >0.95, according to Islam and Mostafa (Hulluka et al., Citation2023). Overall results showed that 93.75% of sample stations had a moderate water quality category, while 6.2% of sampling points fell into the fair category. All of the test sites, which fall into the fair water quality category, were hand-dug wells, two of which are in the Cheffe area and one in the Gemeto, both of which are located in the rural Hawella Tulla sub-city. The static water table in these places is close to the surface; therefore, contamination from surface water or contamination from pit latrines that are nearby in this rural settlement is the cause. In this investigation, sodicity and crop toxicity were the two most important hazard classes that affected the overall irrigation water quality index. The overall irrigation water quality index was mostly influenced by sodicity (15–41%) and toxicity (26–61%).
4. Conclusion
Water Quality Indices are becoming important water resource management tools worldwide. In this study, three models for domestic use and one model for irrigation use have been used.
According to the model results:
The Synthetic Water Quality Index (SPI) was rigorous in its evaluation of the quality, demonstrating that all water samples, regardless of their source, were inadequate.
According to the Weighted Arithmetic Water Quality Index (WA-WQI), 50% of the samples were poor and the remaining were very poor. All of the very poor water quality reports were from hand-dug wells.
By Entropy Water Quality Index (EWQI) 22.92% of water samples were average and 98.33 and 18.75% of water samples were poor and extremely poor quality, respectively. Extremely poor quality sample dominated from shallow wells.
Five parameters; F−, K+, BOD5, COD, and HCO3– hold the majority of the weight in determining the quality of all indices for domestic use.
About 93% of water samples were of moderate quality and can be used for irrigation.
The two hazard groups that contributed the most to determining the qualities in the Irrigation Water Quality Index (IWQ index) were sodicity and crop toxicity.
Currently, it is projected from a source survey that daily average water usage is around 12,956 m3/day, which is nearly twice as much as what the city’s water and sewerage systems are providing. Groundwater extraction fills the gap, demonstrating the stress on the aquifers beneath Hawassa City.
According to the Ethiopian Ministry of Water, Irrigation, and Energy (MWIE), Bahir Dar and Hawassa cities had a feasibility study and a thorough design for wastewater management system. Almost 27,000 m3/day of effluent from Hawassa city will be routed to sewerage systems leading to wastewater treatment plants. This is a significant development plan for Hawassa City, but it requires careful consideration and review because a water treatment facility is being built there, where the water quality is good and most of the city’s potable water comes from. Aquifer augmentation measures should be planned since when the sewerage system is connected, unintentional recharging of the groundwater from leaks will diminish. With such a condition and increasing water demand, the city’s aquifers will be severely depleted.
To monitor the quantity and quality of water for surface and groundwater resources, it is crucial to set up the necessary facilities and procedures. Given that the city aquifers are being polluted at a rapid rate within a constrained surface area, two supplementary options are advised based on the findings of this research: (i) enacting strict guidelines for the construction of septic tanks and sewerage systems to make them watertight, as these facilities are the primary sources of groundwater contamination; and (ii) enhancing groundwater recharge from rainwater sources during rainy seasons with gravel-packed soak pits to increase water storage and dilute pollutants.
Supplementary Tables and Figures.docx
Download MS Word (3.4 MB)Disclosure statement
No potential conflict of interest was reported by the author(s).
Additional information
Notes on contributors
Getahun Alemu
Getahun Alemu is a lecturer in the water resources and irrigation department, Hawassa University. Currently a PhD student working on water quality and ground water artificial recharge management in the southern Ethiopia.
Awdenegest Moges
Awdenegest Moges (PhD) is an Associate Professor in the department of Biosystems Engineering, Hawassa University Ethiopia. His research interest is in soil and water management. Published articles related to soil, water and conservation, landuse dynamics and environmental management aspects.
Sirak Tekleab
Sirak Tekleab (PhD) is an Assistant Professor in Addis Abeba University, Ethiopian Institute of Water Resources. His research works focused on hydrological processes and modelling, land use land cover and climate change impacts on stream flows and water resources. His research interest includes hydrological processes understanding, modelling, isotope hydrology for water resources management, and investigation of water availability under environmental changes.
References
- Alcaine, A. A., Schulz, C., Bundschuh, J., Jacks, G., Thunvik, R., Gustafsson, J. P., Mörth, C. M., Sracek, O., Ahmad, A., & Bhattacharya, P. (2020). Hydrogeochemical controls on the mobility of arsenic, fluoride and other geogenic co-contaminants in the shallow aquifers of northeastern La Pampa Province in Argentina. The Science of the Total Environment, 715, 136671. https://doi.org/10.1016/j.scitotenv.2020.136671
- Anonna, T. A., Ahmed, Z., Alam, R., Karim, M. M., Xie, Z., Kumar, P., Zhang, F., & Simal-Gandara, J. (2022). Water quality assessment for drinking and irrigation purposes in Mahananda River Basin of Bangladesh. Earth Systems and Environment, 6(1), 87–98. https://doi.org/10.1007/s41748-021-00274-x
- APHA (2017). APHA standard methods for the examination of water and wastewater (23rd ed., R. B. Baird, A. D. Eaton, E. W. Rice, & L. L. Bridgewater, Eds.). American Public Health Association (APHA), American Water Works Association (AWWA), Water Environment Federation, Water Environment Federation. ISBN9780123821652.
- Ayalew, A. A. (2020). Development of kaolin clay as a cost-effective technology for defluoridation of groundwater. International Journal of Chemical Engineering, 2020, 1–10. https://doi.org/10.1155/2020/8820727
- Ayenew, T. (2008). The distribution and hydrogeological controls of fluoride in the groundwater of central Ethiopian rift and adjacent highlands. Environmental Geology, 54(6), 1313–1324. https://doi.org/10.1007/s00254-007-0914-4
- Berehanu, B., Lemma, B., & Tekle-Giorgis, Y. (2015). Chemical composition of industrial effluents and their effect on the survival of fish and eutrophication of Lake Hawassa, Southern Ethiopia. Journal of Environmental Protection, 6(8), 792–803. https://doi.org/10.4236/jep.2015.68072
- Biswas, A. K., & Tortajada, C. (2019). Water crisis and water wars: myths and realities. International Journal of Water Resources Development, 35(5), 727–731. https://doi.org/10.1080/07900627.2019.1636502
- Brhane, G. K. (2018). Characterization of hydrochemistry and groundwater quality evaluation for drinking purposes in Adigrat area, Tigray, northern Ethiopia. Water Science, 32(2), 213–229. https://doi.org/10.1016/j.wsj.2018.09.003
- Chabuk, A., Al-Madhlom, Q., Al-Maliki, A., Al-Ansari, N., Hussain, H. M., & Laue, J. (2020). Water quality assessment along Tigris River (Iraq) using water quality index (WQI) and GIS software. Arabian Journal of Geosciences, 13(14), 1–23. https://doi.org/10.1007/s12517-020-05575-5
- Dottridge, J., Foley, A., & Walters, N. (2021). Safeguarding groundwater abstractions by enforcement of source protection zones. In Advances in Geoethics and Groundwater Management: Theory and Practice for a Sustainable Development: Proceedings of the 1st Congress on Geoethics and Groundwater Management (GEOETH&GWM'20) (pp. 515–518). Springer International Publishing.
- FAO (1996). Control of water pollution from agriculture. FAO Irrigation and Drainage Paper No. 55. Food and Agriculture Organization of the United Nations.
- Firew, T., Daniel, F., & Solomon, S. S. (2018). Performance assessment of wastewater treatment plant of Hawassa St. George Brewery, Hawassa, Ethiopia. Journal of Applied Sciences and Environmental Management, 22(8), 1285–1292. https://doi.org/10.4314/jasem.v22i8.23
- Foster, S. (2020). Global policy overview of groundwater in urban development—A tale of 10 cities!. Water, 12(2), 456. https://doi.org/10.3390/w12020456
- Gong, J., Guo, X., Yan, X., & Hu, C. (2023). Review of urban drinking water contamination source identification methods. Energies, 16(2), 705. https://doi.org/10.3390/en16020705
- Haji, M., Wang, D., Li, L., Qin, D., & Guo, Y. (2018). Geochemical evolution of fluoride and implication for F− enrichment in groundwater: Example from the Bilate River Basin of Southern Main Ethiopian Rift. Water, 10(12), 1799. https://doi.org/10.3390/w10121799
- Halcrow Group Limited and Generation Integrated Rural Development Consultants (2009). Rift Valley Lakes Basin integrated resources development master plan study project. Main report. Ministry of Water Resources, The Federal Democratic Republic of Ethiopia.
- Hasan, M., Shang, Y., Akhter, G., & Jin, W. (2018). Geophysical assessment of groundwater potential: a case study from Mian Channu Area, Pakistan. Ground Water, 56(5), 783–796. https://doi.org/10.1111/gwat.12617
- Hosseininia, M., & Hassanzadeh, R. (2023). Groundwater quality assessment for domestic and agricultural purposes using GIS, hydrochemical facies and water quality indices: a case study of Rafsanjan plain, Kerman province, Iran. Applied Water Science, 13(3), 84. https://doi.org/10.1007/s13201-023-01891-9
- Hulluka, T. A., Balcha, S. K., Yohannes, B., Bantider, A., & Negatu, A. (2023). Groundwater research in the Ethiopian Rift Valley Lakes region. Frontiers in Water, 5, 819568. https://doi.org/10.3389/frwa.2023.819568
- Iqbal, J., Su, C., Rashid, A., Yang, N., Baloch, M. Y. J., Talpur, S. A., Ullah, Z., Rahman, G., Rahman, N. U., Earjh, E., & Sajjad, M. M. (2021). Hydrogeochemical assessment of groundwater and suitability analysis for domestic and agricultural utility in Southern Punjab, Pakistan. Water, 13(24), 3589. https://doi.org/10.3390/w13243589
- Islam, M. S., & Mostafa, M. G. (2022). Development of an integrated irrigation water quality index (IIWQIndex) model. Water Supply, 22(2), 2322–2337. https://doi.org/10.2166/ws.2021.378
- Jagaba, A. H., Kutty, S. R. M., Hayder, G., Baloo, L., Abubakar, S., Ghaleb, A. A. S., Lawal, I. M., Noor, A., Umaru, I., & Almahbashi, N. M. Y. (2020). Water quality hazard assessment for hand-dug wells in Rafin Zurfi, Bauchi State, Nigeria. Ain Shams Engineering Journal, 11(4), 983–999. https://doi.org/10.1016/j.asej.2020.02.004
- JICA (2012). The study of groundwater assessment in the Rift Valley Lakes Basin in the Federal Democratic Republic of Ethiopia. Final report. Japan International Cooperation Agency, Kokusai Kogyo Co. Ltd., Ministry of Water and Energy, The Federal Democratic Republic of Ethiopia.
- Kachroud, M., Trolard, F., Kefi, M., Jebari, S., & Bourrié, G. (2019). Water quality indices: Challenges and application limits in the literature. Water, 11(2), 361. https://doi.org/10.3390/w11020361
- Kumar, P. S., & Augustine, C. M. (2022). Entropy-weighted water quality index (EWQI) modelling of groundwater quality and spatial mapping in Upper Odai Sub-Basin, South India. Modeling Earth Systems and Environment, 8(1), 911–924. https://doi.org/10.1007/s40808-021-01132-5
- Lencha, S. M., Tränckner, J., & Dananto, M. (2021). Assessing the water quality of Lake Hawassa Ethiopia—Trophic state and suitability for anthropogenic uses—Applying common water quality indices. International Journal of Environmental Research and Public Health, 18(17), 8904. https://doi.org/10.3390/ijerph18178904
- Ligate, F., Ijumulana, J., Ahmad, A., Kimambo, V., Irunde, R., Mtamba, J. O., Mtalo, F., & Bhattacharya, P. (2021). Groundwater resources in the East African Rift Valley: Understanding the geogenic contamination and water quality challenges in Tanzania. Scientific African, 13, e00831. https://doi.org/10.1016/j.sciaf.2021.e00831
- Matta, G., Nayak, A., Kumar, A., & Kumar, P. (2020). Water quality assessment using NSFWQI, OIP and multivariate techniques of Ganga River system, Uttarakhand, India. Applied Water Science, 10(9), 1–12. https://doi.org/10.1007/s13201-020-01288-y
- Meena, P. L. (2022). Study on the hydrogeochemical processes regulating the groundwater chemistry in the southeast Rajasthan. Journal of the Geological Society of India, 98(10), 1455–1465. https://doi.org/10.1007/s12594-022-2193-9
- Ministry of Water, Irrigation and Electricity (2018). Feasibility study and detail design of waste water management system for Bahir Dar and Hawassa Towns. Report. Author.
- Mwiathi, N. F., Gao, X., Li, C., & Rashid, A. (2022). The occurrence of geogenic fluoride in shallow aquifers of Kenya Rift Valley and its implications in groundwater management. Ecotoxicology and Environmental Safety, 229, 113046. https://doi.org/10.1016/j.ecoenv.2021.113046
- National Meteorological Agency of Ethiopia (n.d.). Report.
- Nguyen, A. H., Pham, N. T. T., Tat, V. M. H., Truong, H. T., & Vo, P. L. (2021, February). Application of Entropy weight in groundwater quality index (EWQI) and GIS for groundwater quality zoning in the Southeastern Coastal region, Vietnam. IOP Conference Series: Earth and Environmental Science, 652(1), 012005. https://doi.org/10.1088/1755-1315/652/1/012005
- Rahman, M. A., Kumar, S., Mohana, A. A., Islam, R., Hashem, M. A., & Chuanxiu, L. (2019). Coliform Bacteria and trace metals in drinking water, southwest Bangladesh: Multivariate and human health risk assessment. International Journal of Environmental Research, 13(2), 395–408. https://doi.org/10.1007/s41742-019-00184-x
- Rango, T., Bianchini, G., Beccaluva, L., Ayenew, T., & Colombani, N. (2009). Hydrogeochemical study in the Main Ethiopian Rift: New insights to the source and enrichment mechanism of fluoride. Environmental Geology, 58(1), 109–118. https://doi.org/10.1007/s00254-008-1498-3
- Rashid, A., Ayub, M., Javed, A., Khan, S., Gao, X., Li, C., Ullah, Z., Sardar, T., Muhammad, J., & Nazneen, S. (2021). Potentially harmful metals, and health risk evaluation in groundwater of Mardan, Pakistan: Application of geostatistical approach and geographic information system. Geoscience Frontiers, 12(3), 101128. https://doi.org/10.1016/j.gsf.2020.12.009
- Rashid, A., Guan, D. X., Farooqi, A., Khan, S., Zahir, S., Jehan, S., Khattak, S. A., Khan, M. S., & Khan, R. (2018). Fluoride prevalence in groundwater around a fluorite mining area in the flood plain of the River Swat, Pakistan. The Science of the Total Environment, 635, 203–215. https://doi.org/10.1016/j.scitotenv.2018.04.064
- Rashid, A., Khattak, S. A., Ali, L., Zaib, M., Jehan, S., Ayub, M., & Ullah, S. (2019). Geochemical profile and source identification of surface and groundwater pollution of District Chitral, Northern Pakistan. Microchemical Journal, 145, 1058–1065. https://doi.org/10.1016/j.microc.2018.12.025
- Shukla, S., & Saxena, A. (2020). Groundwater quality and associated human health risk assessment in parts of Raebareli district, Uttar Pradesh, India. Groundwater for Sustainable Development, 10, 100366. https://doi.org/10.1016/j.gsd.2020.100366
- Solangi, G. S., Siyal, A. A., Babar, M. M., & Siyal, P. (2019). Application of water quality index, synthetic pollution index, and geospatial tools for the assessment of drinking water quality in the Indus Delta, Pakistan. Environmental Monitoring and Assessment, 191(12), 731. https://doi.org/10.1007/s10661-019-7861-x
- Son, C. T., Giang, N. T. H., Thao, T. P., Nui, N. H., Lam, N. T., & Cong, V. H. (2020). Assessment of Cau River water quality assessment using a combination of water quality and pollution indices. Journal of Water Supply: Research and Technology-Aqua, 69(2), 160–172. https://doi.org/10.2166/aqua.2020.122
- Sutadian, A. D., Muttil, N., Yilmaz, A. G., & Perera, B. J. C. (2018). Development of a water quality index for rivers in West Java Province, Indonesia. Ecological Indicators, 85, 966–982. https://doi.org/10.1016/j.ecolind.2017.11.049
- Tadesse, D., & Zenaw, T. (2003). Hydrogeology and engineering geology of Awassa Lake catchments. Report. Geological Survey of Ethiopia.
- Teshome, F. B. (2020). Seasonal water quality index and suitability of the water body to designated uses at the eastern catchment of Lake Hawassa. Environmental Science and Pollution Research International, 27(1), 279–290. https://doi.org/10.1007/s11356-019-06794-4
- Uddin, M. G., Nash, S., & Olbert, A. I. (2021). A review of water quality index models and their use for assessing surface water quality. Ecological Indicators, 122, 107218. https://doi.org/10.1016/j.ecolind.2020.107218
- Wang, X., Tian, Y., & Liu, C. (2023). Assessment of groundwater quality in a highly urbanized coastal city using water quality index model and Bayesian model averaging. Frontiers in Environmental Science, 11, 279. https://doi.org/10.3389/fenvs.2023.1086300
- World Health Organization (2018). Developing drinking-water quality regulations and standards: General guidance with a special focus on countries with limited resources. Author.
- Zhang, Q., Qian, H., Xu, P., Hou, K., & Yang, F. (2021). Groundwater quality assessment using a new integrated-weight water quality index (IWQI) and driver analysis in the Jiaokou Irrigation District, China. Ecotoxicology and Environmental Safety, 212, 111992. https://doi.org/10.1016/j.ecoenv.2021.111992
- Zhang, X., Qian, H., Wu, H., Chen, J., & Qiao, L. (2016). Multivariate analysis of confined groundwater hydrochemistry of a long-exploited sedimentary basin in northwest China. Journal of Chemistry, 2016, 1–15. https://doi.org/10.1155/2016/3812125
- Zhe, W., Xigang, X., & Feng, Y. (2021). An abnormal phenomenon in the entropy weight method in the dynamic evaluation of the water quality index. Ecological Indicators, 131, 108137. https://doi.org/10.1016/j.ecolind.2021.108137
- Zigde, H., & Tsegaye, M. E. (2019a). Evaluation of the current water quality of Lake Hawassa, Ethiopia. International Journal of Water Resources and Environmental Engineering, 11(7), 120–128.
- Zigde, H., & Tsegaye, M. E. (2019b). Multivariate analysis of water quality and identification of potential pollution sources of Lake Hawasa, Ethiopia. Environmental Science, 126, 52424–52429.