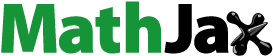
Abstract
The integrity of environmental, social and governance (ESG) data in corporate sustainability reports is of heightened importance to socially responsible investors. This study aims to define essential ESG criteria influencing investment and assess corporate ESG performance. A novel method, combining Analytic Hierarchy Process (AHP) and Weighted Aggregated Sum Product Assessment (WASPAS) in a spherical fuzzy context, is introduced to address uncertainties linked to imprecision and subjectivity in ESG evaluations. Employing Spherical Fuzzy-AHP (SF-AHP) to determine evaluation criteria for global electronics companies, followed by Spherical Fuzzy-WASPAS (SF-WASPAS) to finalize rankings, this approach was examined within the global electronics industry. The research underscores the method’s value in gauging ESG performance and guiding executives in investment decisions. Furthermore, comparative analysis (SF-WASPAS, SF-TOPSIS and Fermatean Fuzzy WASPAS) supports the framework’s reliability, suggesting its applicability in resource management for attracting investors and enhancing stakeholder interaction. The results demonstrate the effectiveness of the proposed SF-AHP-WASPAS method in assessing corporate ESG performance under uncertainty, providing executives with a robust decision-making framework for sustainable investment strategies.
Reviewing Editor:
1. Introduction
Sustainable and responsible investment practices have gained increasing prominence amid heightened awareness of environmental, social and governance (ESG) concerns (Ruan et al., Citation2021). As investors seek investments aligned with their values, integrating ESG factors into decision-making has become pivotal (Arvidsson & Dumay, Citation2022). This study explores ESG performance assessment, with a focus on the global electronics industry. The concept of ESG has risen to prominence against the backdrop of heightened global consciousness and advocacy around environmental protection, social justice and responsible governance. ESG serves as a comprehensive framework for evaluating the sustainability and societal impact of investment vehicles and corporate entities (Ahmad et al., Citation2023). The environmental aspect examines ecological impacts through metrics like energy consumption, waste generation and resource utilization. The social dimension encompasses issues, such as labor rights, community relations and consumer welfare. Governance deals with matters of transparency, accountability, regulatory compliance and ethical conduct (Eccles & Klimenko, Citation2019).
Strong ESG performance demonstrates an entity’s commitment to long-term value creation in a responsible manner that upholds stakeholder interests. Mainstreaming ESG factors in the investment process aligns financial objectives with principles of sustainable development (OECD, Citation2022). Investors increasingly recognize robust ESG practices as indicative of risk mitigation, stakeholder trust, future-readiness and resource efficiency. Hence, ESG integration and analysis has profound implications for investment decisions and profitability assessments (Economy & Forecast review, Citation2022). However, comprehensively evaluating ESG performance across its multifaceted dimensions poses considerable challenges. The complex, subjective and ambiguous nature of ESG data limits the efficacy of conventional quantitative models. Prevailing ESG rating systems exhibit inconsistencies and lack adaptability to nuanced circumstances (Hoang et al., Citation2022). These challenges have catalyzed research into innovative ESG assessment techniques equipped to handle uncertainty. The electronics sector offers a compelling avenue for investigating progressive ESG evaluation frameworks. Electronics manufacturing entails extensive utilization of hazardous materials alongside mammoth energy and water demands. The industry’s deep supply chains exacerbate challenges of environmental monitoring and ethical sourcing (Lu et al., Citation2020). With electronics bound to play an increasingly ubiquitous role across society, strategies to mitigate associated ESG risks warrant urgent attention in the context of the world always full of volatility and nontraditional security risks. However, research on ESG integration in the electronics industry remains sparse.
Notable studies have explored various aspects of ESG performance analysis, valuation and integration. Hoepner et al. (Citation2016) used ESG disclosures across multiple domains to evaluate responsible investment implications. Wang et al. (Citation2023) proposed a model to integrate ESG risks into investment decisions and estimate ESG-adjusted equity returns. Ghoul and El; Karoui (Citation2022) uncovered a positive link between corporate social responsibility and financial performance. Schiemann and Tietmeyer (Citation2022) examined investor reactions to ESG controversies, underlining reputational costs. Joof et al. (Citation2022) investigated the financial materiality of biodiversity loss risks for corporations. Cumulatively, these studies have enriched understanding of how ESG factors interface with risk metrics, valuation parameters and shareholder returns. However, gaps persist regarding methodological frameworks tailored to specific industries while accounting for informational ambiguity.
Despite the rising literature on ESG performance, previous efforts have major shortcomings and significant gaps that need to be filled. The electronics manufacturing sector is one of the world’s leading consumers of energy and resources, accounting for 2% of global greenhouse gas emissions. Due to the industry’s reliance on fossil fuels and water-intensive procedures, semiconductor manufacturing disproportionately impacts the industry’s carbon footprint (MACROFAB, Citation2023). A fresh surge of electronics production is on the horizon, propelled by fluctuating energy costs and a growing demand for environmental sustainability (Isabel Al-Dhahir, Citation2023). The lack of an ESG policy for the electronics manufacturing sector will eventually impact its proximity to capital. This may result from an absence of decision-support tools to help electronics manufacturing companies incorporate ESG into their operations. To the extent of our current understanding, there has been no research conducted to assess the various dimensions and intricate complexity of incorporating ESG considerations into the global electronics manufacturing industry.
Several previous studies have utilized various multi-criteria decision-making (MCDM) techniques to evaluate ESG performance across different contexts. For instance, Reig-Mullor et al. (Citation2022) employed a hybrid AHP-TOPSIS approach under neutrosophic logic to analyze ESG factors in the oil and gas industry. Escrig-Olmedo et al. (Citation2017) proposed a fuzzy TOPSIS model to integrate ESG preferences into sustainable investment decisions in the clothing sector. Simsek Yagli et al. (Citation2022) applied an interval-valued intuitionistic fuzzy BWM technique to determine ESG criteria weights in the banking sector. While providing valuable insights, these studies have not specifically focused on the electronics manufacturing sector. Moreover, the integration of Analytic Hierarchy Process (AHP) and Weighted Aggregated Sum Product Assessment (WASPAS) under a spherical fuzzy environment has not been applied to ESG assessment. Spherical fuzzy sets (SFS) enhance flexibility in tackling uncertainty compared to classical fuzzy sets (Kutlu Gündoğdu & Kahraman, Citation2019). SFS facilitates managerial-level people to extend an extension of fuzzy sets by creating a membership function on a spherical surface and distinctly conveying the parameters from that membership function by giving a larger domain (Kutlu Gündoğdu & Kahraman, Citation2019). SFS combines Pythagorean fuzzy sets (PFS; Radwan et al., Citation2016) and Neutrosophic fuzzy sets (Kahraman et al., Citation2019). Thus, an SFS is a preferred solution for representing expert opinion in a context with high uncertainty. The amalgamation of AHP and WASPAS leverages their complementary strengths in criteria weighting and ranking respectively (Zavadskas et al., Citation2012). However, research harnessing the combined potential of these techniques for ESG analysis in the electronics industry remains limited. This study spearheads research on ESG assessment in electronics manufacturing through an inclusive evaluation model tailored to the sectoral context. The proposed framework assimilates quantitative data, such as financial indicators with qualitative sustainability criteria extracted through expert opinions. By harmonizing AHP and WASPAS under a spherical fuzzy environment, this hybrid approach integrates the strengths of multiple techniques. SF-AHP determines influential ESG criteria and their relative weights by accommodating expert input under uncertainty. Next, SF-WASPAS aggregates scores into a nuanced ranking index, bypassing the limitations of existing rating systems. The spherical fuzzy environment aptly tackles the inherent subjectivity of ESG measures.
This research makes several notable contributions to the advancement of ESG assessment frameworks tailored to the electronics industry.
First, it proposes an innovative integration of SF-AHP and SF-WASPAS to construct a robust multi-criteria decision-making model for ESG evaluation. By harnessing the complementary strengths of these methodologies within a fuzzy environment, the study pioneers a sophisticated approach to tackle uncertainty and subjectivity. Second, the research identifies a comprehensive set of ESG criteria tailored to electronics manufacturing through extensive literature reviews and expert consultations. The criteria encompass diverse factors aligned with the principles of sustainable development and responsible investment. This establishes an industry-specific evaluation framework. Additionally, the study undertakes comparative analyses to validate the proposed model against other MCDM techniques like Fuzzy WASPAS and TOPSIS. The consistency in results and company rankings substantiates the efficacy and validity of the proposed SF-AHP and SF-WASPAS integration. Finally, the research conducts an empirical analysis of real-world scenarios by applying the developed framework to assess and rank leading global electronics companies for their ESG performance. This demonstrates the practical utility of the model in enabling nuanced ESG evaluations to inform sustainable investment decisions.
Therefore, this study seeks to address three key research questions related to evaluating ESG performance in the global electronics industry: (i) What are the most significant ESG criteria for assessment in this sector, and how can their relative weights be established? (ii) How do different stakeholder groups (investors, employees, customers, etc.) prioritize ESG factors differently, and how can these diverse perspectives be incorporated into a holistic evaluation framework? (iii) How can a hybrid methodology SF-AHP and SF-WASPAS be leveraged to account for imprecision and subjectivity inherent in judging ESG rankings, providing electronics companies with a robust system for benchmarking sustainability initiatives under uncertainty? The overall goal is to develop an approach to determine the leading ESG criteria, integrate varied stakeholder priorities and quantify uncertain judgments to evaluate corporate ESG performance in the complex global electronics industry.
Section 2 covers a review of existing literature. Section 3 explains the SF-AHP and SF-WASPAS methods used. Section 4 discusses the research process, data and analysis framework, followed by outcome discussion. The last section provides a thorough conclusion and suggests areas for future research.
2. Literature review
2.1. Review of ESG criteria in investment literature
Investment strongly emphasizes inter-organizational activities, particularly those that involve collaboration, coordination and cooperation for the sake of sustainability, which is made possible by actions that are positive in attitude, conduct and consequence (Castañer & Oliveira, Citation2020). Interdisciplinary cooperation is also essential for the effective execution of any sustainability strategy. Interdisciplinary cooperation greatly impacts novel service development (Kitsios & Kamariotou, Citation2020). Similar to what was found in the preceding study, corporate sustainability, strategic management and decision making are the three main poles of any company’s business plan (Kitsios et al., Citation2020). According to Kraus et al. (Citation2021), management implications and organizational elements are crucial for improving the operative efficiencies of the service sector through various technology implementations. The key success factors for achieving sustainability in the construction industry are ambient intelligence, smart technology and technological acceptance (Marikyan et al., Citation2019). Sustainable supply chain management (SSCM) is the main objective for all companies. Organizations should use green practices, eco-design, green purchasing and environmental awareness to fulfill an organizational vision, goals and productivity toward SSCM (Patel & Desai, Citation2019). Stakeholder involvement, entrepreneurial growth and innovation management all assist firms in adopting an entrepreneurial mindset for sustainability (Shams et al., Citation2020). Through their value-generation process, digital technologies are used to identify tactical responses. Managing structural modifications and organizational obstacles will determine whether this approach has favorable or negative results.
Sustainability has added many additional criteria to investment that should be considered and addressed with this new and altered attribute. Studies on investments and issues affecting investments, particularly ESG, are discussed based on the research being conducted. The ESG ranking has an essential effect on investor decision making and the amount of fundamental information given to a company (Pedersen et al., Citation2021). Many investors employ ESG as a result of customer demand. The ESG-based decision criteria are established from a literature analysis and evaluated by experts in the field, as indicated in .
Table 1. Summary of the criteria in the previous study.
ESG data screening and analysis has become an integral part of the investment process for many investors. By examining company performance on ESG metrics, investors can identify businesses that align with their values and priorities (Jianqiang et al., Citation2022). Governance and management quality considerations are often intertwined in ESG assessments, as robust governance provides confidence in management’s ability to effectively navigate ESG issues (Ghoul & El; Karoui, Citation2022). However, comprehensively evaluating the multifaceted dimensions of ESG performance poses challenges due to the complexity and ambiguity inherent in ESG data (Eccles & Klimenko, Citation2019). To address this, MCDM techniques have gained traction, given their suitability for complex decision contexts characterized by conflicting objectives (Mohammad & Wasiuzzaman, Citation2021). Meanwhile, AHP offers systematic prioritization of criteria while WASPAS generates nuanced rankings (Mardani et al., Citation2020), making their combination well-suited for ESG assessment. This study proposes harnessing the harmonized strengths of SF-AHP and SF-WASPAS to construct a tailored ESG evaluation framework for the global electronics sector. By accounting for ambiguities and enabling calibrated criteria weighting and rankings, the hybrid SF-AHP-SF-WASPAS model constitutes an inclusive, progressive decision tool to aid electronics companies and sustainable investors. Empirically demonstrating this methodology’s ability to address complex subjectivities can enrich emerging ESG integration practices.
2.2. Literature review on spherical fuzzy MCDM models
SFSs have emerged as a progressive extension of existing fuzzy set theories like Pythagorean and intuitionistic fuzzy sets (IFSs). By specifying membership on a multidimensional spherical surface, SFSs confer an expansive preference domain suited for encapsulating uncertainty and ambiguity in decisions (Kutlu Gündoğdu & Kahraman, Citation2020a, Citation2020b, Citation2020c). The introduction of fuzzy logic by Otten and Ollier (Citation1976) allowed variables to take inclusive values between 0 and 1, enabling the representation of imprecise information. The type-1 FS has issues with mapping membership functions. A type-2 FS is proposed to address criticism of type-1 FSs and uncertainty around this membership function. While type-1 FSs have a set membership function, type-2 FSs consider a ranging membership function. Then, a large number of scholars expand the FSs and describe numerous additional sets in the literature. Type-2 FSs and IFSs were proposed by Atanassov (Citation1999). This mentioned the potential for figuring out the level of non-membership cases and expressed the DMs’ level of hesitation. Through the idea of fuzzy multi-sets, invented by Yager (Citation1986), it is conceivable for one entity to belong to the same cluster to varying degrees. This drove the development of diverse fuzzy set variants like picture fuzzy sets (PFSs; Cuong & Kreinovich, Citation2013) and neutrosophic sets (NS; Abdel-Baset et al., Citation2019) into model real-world vagueness. Neutrosophy was offered by Smarandache (Citation1999) as a resolution to the challenge of inconsistent and ambiguous data in decision making. Garibaldi and Ozen (Citation2007) presented nonstationary FSs (NFSs) to cope with the ambiguity/uncertainty in a fuzzy system’s membership functions while considering dynamic environments. The idea of hesitant FSs, which allows DMs to choose more than one membership for an element, is presented by Torra (Citation2010). Yager (Citation2013) defines a novel section of Pythagorean FSs with an aggregate of squares of membership and non-membership degrees less than or equal to one. Cuong and Kreinovich (Citation2014) presented the idea of PFSs, which incorporate the FS and IFS features (Atanassov, Citation1999). Later, Yager (Citation2017) extended the IFSs and PFSs to create a new general category known as q-rung ortho-pair FSs.
In terms of recent version of fuzzy sets, Mahmood et al. (Citation2019) proposed a departure from the conventional methodologies utilized in the representation of human opinions. Traditionally, binary yes-or-no frameworks, exemplified by FS and IFS, have been the prevailing paradigms in this arena. However, the authors introduced an innovative approach that imparts a heightened level of sophistication to this process. This advancement is realized through the application of PFSs, enabling nuanced categorizations spanning yes, abstain, no and refusal. Notably, the recent work by Gündoğdu (Citation2019) has further expanded into SFSs. SFSs address these limitations through independent assignment of membership, non-membership and hesitancy degrees adhering to the constraint that their squared sum is less than one. Thereby, SFSs comprehensively capture expert opinions under uncertainty.
By incorporating SFSs, Kutlu Gündoğdu and Kahraman (Citation2020a, Citation2020b, Citation2020c pioneered the spherical fuzzy AHP (SF-AHP) technique as an advancement over the conventional fuzzy AHP approach. SF-AHP determines criteria weights through spherical fuzzy pairwise comparisons, allowing ambiguity management. Similarly, SF-WASPAS emerged by harnessing SFSs within the WASPAS method Nguyen et al., Citation2022). This hybrid technique combines simple additive weighting and weighted product model under spherical fuzziness for robust rankings. Diverse studies have applied SF-AHP and SF-WASPAS across sectors like manufacturing, construction and healthcare (Kutlu Gündoğdu & Kahraman, Citation2021; Erdoğan et al., Citation2021). However, their amalgamation to evaluate ESG factors remains unexplored, especially for the electronics industry. This study postulates that the harmonization of SF-AHP and SF-WASPAS, leveraging their complementary strengths, constitutes an optimal approach for ESG assessment. By tackling uncertainty and enabling nuanced criteria prioritization and rankings, this progressive integration enriches decision-making practices for sustainable electronics investments.
2.3. Research gap
The comprehensive literature review reveals several notable knowledge gaps and opportunities for further research on investment and ESG: First, despite the recognized importance of interdisciplinary cooperation for executing sustainability strategies, there is limited research on its specific impact on new service development. Examining this relationship can uncover insights to enrich sustainable innovation. Second, while success factors for sustainability in construction are established, few studies have explored the role of technology integration in improving operational efficiency. Investigating technological solutions’ sustainability contributions can empower construction practitioners. Third, research remains scarce on cultivating an entrepreneurial mindset for sustainability through stakeholder collaboration, growth-focused ventures and innovation management. Analyzing these avenues can guide entities in embedding sustainability within business models. Fourth, research is lacking on leveraging digital technologies to manage structural transformations and overcome barriers when adopting value-generation processes. Shedding light on this can aid entities in their sustainability journeys. Finally, gaps persist in frameworks tailored to specific industries that evaluate ESG criteria, weights and rankings using robust hybrid techniques under uncertainty. The global electronics sector warrants attention here. Advancing knowledge on spherical fuzzy MCDM approaches for electronics can enrich sustainable investment practices.
3. Methodology
3.1. Preliminaries of spherical fuzzy sets
SFSs are extensions of PFSs, interval fuzzy sets (IFS), and NS that offer greater flexibility and applicability to experts in various fields. Each element in the universe of discourse is associated with a triplet degree of membership, indeterminacy and non-membership, respectively. Geometrically, a spherical fuzzy number can be represented as a point on or inside the unit sphere. The closer the point is to the surface of the sphere, the more certain the evaluation is. Points inside the sphere allow for uncertainty in the evaluation. This geometric interpretation provides an intuitive visualization for understanding SFSs.
Definition 1:
SFS is denoted as representing a SFS of the universe
as follows:
(1)
(1)
(2)
(2)
(3)
(3)
with
is known as membership function
is denoted as a non-membership function and
is a hesitant function of
to
Definition 2:
Some basic operations of SFS are presented with and
as follows:
Union:
(4)
(4)
Intersection:
(5)
(5)
Addition:
(6)
(6)
Multiplication:
(7)
(7)
Multiplication by a scalar;
(8)
(8)
Power of
(9)
(9)
Definition 3:
Spherical weighted arithmetic mean (SWAM) is calculated using EquationEquation (10)(10)
(10) :
(10)
(10)
where
);
Definition 5:
Spherical weighted geometric mean (SWGM) is calculated using EquationEquation (11)(11)
(11) :
(11)
(11)
where
Normalized spherical distance between on the surface of a sphere
Clearly, we have that and
.
3.2. SF-AHP model
The SF-AHP model (Kutlu Gündoğdu & Kahraman, Citation2020a, Citation2020b, Citation2020c) is utilized to determine criteria weights through the following five steps:
Step 1: A hierarchical structure is constructed with the overall study objective placed at level 1 and the suggested evaluation criteria positioned at level 2.
Step 2: Pairwise comparison matrices () are developed using linguistic scales to compare each pair of criteria. Score indices (SIs) for each criterion are then calculated using EquationEquations (12)(12)
(12) and Equation(13)
(13)
(13) based on these comparison matrices.
(12)
(12)
(13)
(13)
for AMI, VHI, HI, SMI, and EI.for EI, SLI, LI, VLI, and ALI.
Table 2. SF-AHP linguistic terms.
Step 3: Weights of each criterion are computed using EquationEquations (14)–(17) based on the SIs from Step 2.
(14)
(14)
where
(15)
(15)
(16)
(16)
(17)
(17)
Step 4: Consistency analysis is performed to ensure logical judgment using .
Table 3. RI values.
If the consistency test is passed, the final local and global weights for each criterion are attained. If not, the comparisons are revised until consistency is achieved.
3.3. SF-WASPAS
To surmount ambiguous and imprecise assessments, the spherical fuzzy extension of WASPAS (WASPAS-SF) employing linguistic terms was suggested in this study:
Step 1: The performance evaluations for each alternative against the criteria are expressed using linguistic terms and converted into spherical fuzzy numbers. These are then weighted based on their importance using EquationEquations (18)–(21). A defuzzification operation is applied to the spherical fuzzy numbers according to EquationEquation (24)(24)
(24) to obtain crisp values.
(18)
(18)
(19)
(19)
(20)
(20)
(21)
(21)
(22)
(22)
(23)
(23)
(24)
(24)
Step 3: The spherical fuzzy values obtained from the SAW and WPM methods are integrated using a threshold lambda (λ) that represents the relative significance assigned to each method. The aggregated value Qi for each alternative is calculated as a weighted sum of the SAW and WPM values using EquationEquations (25)–(27):
(25)
(25)
(26)
(26)
(27)
(27)
This allows the decision maker (DM) flexibility in emphasizing the relative contribution of the SAW and WPM aggregations when deriving the final rankings.
Step 4: If required, the alternatives are then ranked in decreasing order of these Qi values to determine their priority order. If any alternatives end up with the same Qi value, their accuracy degrees can be used as a tie-breaking factor. The accuracy degree is calculated as per EquationEquation (28)(28)
(28) . The alternative with the higher accuracy degree is ranked higher in case of a tie scenario.
(28)
(28)
4. Case study
4.1. Research description
This study employs spherical fuzzy MCDM techniques to establish a methodology for the assessment and integration of ESG factors into investment decision-making processes. Given the inherent complexity and subjectivity involved in ESG analysis, a multicriteria approach is deemed suitable. Input from institutional investors specializing in the electronics industry was gathered to serve as expert opinions for the SF-AHP model, thereby validating the identified ESG criteria. The analysis encompasses three primary dimensions: ESG, encompassing a total of 16 sub-criteria as presented in . The SF-AHP method is employed to determine criteria weights, utilizing spherical fuzzy pairwise comparisons to effectively address the ambiguity present in judgments. Subsequently, the SF-WASPAS method ranks electronics companies based on aggregated scores, which reflect decision-maker preferences while accounting for uncertainty.
Table 4. List of criteria and concepts of ESG factors.
The global electronics industry, valued at trillions of dollars, is undergoing rapid transformation, with major players heavily investing in cutting-edge technologies. Assessing the industry’s ‘sustainability’ poses challenges due to its immense scale, intricate supply chains, and the emergence of ESG-related risks. This investigation encourages companies to advance this evolving trend by identifying ESG criteria within the electronic product environmental evaluation tool (ecolabel) that best align with the needs of global electronics industry workers and effectively address ESG performance gaps. The primary goal of this study is to identify tailored ESG criteria that align with the specific needs of workers while simultaneously addressing performance gaps. The proposed ESG framework categorizes variables across the ESG dimensions. An empirical analysis of the top global electronics companies illustrates the application of this approach. By integrating financial and ESG factors through advanced fuzzy techniques, this study contributes to the enrichment of sustainable investment practices ().
In this study, the top 10 electronic enterprises in the world were considered: Alphabet Inc-Cl A, Amazon. Com. Inc, Apple Inc, Flex Ltd, Hitachi Ltd, Intel Corp, Intl Business Machines Corp, Microsoft Corp, Nokia Oyj and Siemens Ag-Reg. Three main criteria, including environmental (E), social (S) and governance (G), along with 16 sub-criteria, were examined. These sub-criteria encompass environmental aspects, such as natural loss, GHG emissions, pollution and waste, resource depletion and eco-products/process strategies; social aspects including human rights, human resource management, customer satisfaction, social and political contributions, community relations and health and safety; and governance aspects comprising corporate governance, shareholder rights, risk and opportunity management, and ethics in business. The proposed hierarchy is illustrated in .
4.2. Results of SF-AHP method
A panel of experts comprising 10 members with scholarly and professional expertise was constituted to garner perspectives on the prioritization of criteria and alternative assessment approaches for ESG management in global electronics corporations (Strantzali & Aravossis, Citation2016). Input was solicited from industry professionals possessing a minimum of eight years of experience in ESG stewardship and business development. A meticulous review of extant literature aided the assembly of a comprehensive compilation of criteria for ESG management in the electronics sector, which experts validated as sufficiently exhaustive in encompassing pertinent evaluation parameters (Singh et al., Citation2012).
Adhering to the procedures outlined in Step 4, consistency examinations were conducted on the pairwise comparison matrices constructed using the linguistic terms denoted in , affirming consistency. Subsequently, the SF-AHP method was operationalized to determine the weights of the cardinal criteria - environmental, social, and social – obtained as 0.487, 0.293 and 0.221, respectively. Among these, environmental emerged as the most salient criterion with a weight of 0.487, followed by social at 0.293. Governance was construed as the least substantial criterion with an assigned weight of 0.221.
Leveraging spherical fuzzy numbers and score functions, local weights were multiplied by criteria and sub-criteria to compute the final global weights for each parameter within the electronics industry ESG management framework (Büyüközkan & Güleryüz, Citation2016). As elucidated in , pollution and waste earned recognition as the most pivotal ESG metric, succeeded by GHG emissions and eco-products/process strategies. The primacy of these top three sub-criteria within the environmental domain denotes institutional investors’ emphasis on long-term ecological factors (Biloslavo et al., Citation2018; Samanlioglu & Aya, Citation2017). Conversely, experts deemed criteria encompassing governance and social dimensions as less influential.
Table 5. Results of SF-AHP method.
As depicted in , the factors that had the biggest effects were waste and pollution, GHG emissions, natural loss, eco-products and process strategies, renewable energy, and customer satisfaction, which were found to be 12.97%, 12.32%, 9.08%, 9.08%, 8.22% and 7.46%, respectively. This suggests policymakers and electronics industry leaders orient interventions toward these six key areas for optimal enhancement of corporate ESG performance (Jovanovic et al., Citation2010). However, social and political contributions, corporate governance and business ethics displayed negligible impact. Resource depletion, shareholder rights and human resource management constituted moderately important considerations in sustainable investment decisions (Quteishat & Younis, Citation2023).
4.3. Results of SF-WASPAS method
In the study’s second phase, the SF-AHP weights obtained in Phase 1 were utilized in conjunction with the SF-WASPAS model to rank ten potential global electronic companies. The spherical fuzzy linguistic measures presented in were initially employed to gather opinions from 10 decision makers on the criteria. Subsequently, the SWAM operator was utilized to aggregate the evaluations of alternatives based on the specified criteria. After aggregating the evaluations using the SWAM and multiplication operators, the results of WSM and WPM, along with the defuzzied values, are displayed in and . The values of Q1i and Q2i represented the assessment scores of each alternative as determined by WSM and WPM, respectively. The final assessment score for each option was then calculated from Q1i and Q2i. The classification results of the SF-WASPAS model are presented in , with ‘INTEL CORP’ identified as the most effective strategy, followed by ‘SIEMENS AG-REG (EI2)’, ‘NOKIA OYJ (EI9)’, ‘MICROSOFT CORP (EI5)’ and ‘INTL BUSINESS MACHINES CORP (EI4)’.
Table 6. Calculation for WSM.
Table 7. Calculation for WPM.
Table 8. Results of SF-WASPAS.
INTEL CORP is also proactive in our efforts to reduce our environmental footprint by using natural resources and materials efficiently and responsibly. They intend to use their leadership position in the global tech ecosystem to assist their company and the industry in greater corporate responsibility advances, including environmental sustainability, social impact, and governance. Intel’s new ‘RISE’ strategy and 2030 goals seek to leverage its dominant position in the global technology environment to create a more trustworthy inclusive, and sustainable globe powered by technology and the skills and passion of its employees (Intel, Citation2023). While sustainability is fundamental to Nokia’s mission, the company’s primary objective is to develop technologies that facilitate international cooperation. The last year, Nokia has enhanced its enterprise’s comprehensive ESG approach by ensuring that sustainability is integral to its technological development and business decisions. This strategy is built on decades of successful sustainability projects and practices. This approach is the culmination of years of solid sustainability programs and practices. Nokia’s ESG strategy is built on five strategic emphasis areas in which the firm strives to differentiate itself while also generating verifiable social, environmental and cultural benefits: Environmental affairs, specifically climate and circularity; industrial design digitalization; privacy and protection; closing the digital divide; and responsible business (Nokia Takes ESG Strategy to next Level of Impact. Available online, Citation2023). Microsoft and its alliance partners enable financial organizations to achieve their ESG objectives by providing a cutting-edge, scalable and dependable cloud platform. Enable business users and institutional investors to evaluate assets and products by analyzing and visualizing ESG data (Microsoft Cloud for Sustainability Is Growing, Citation2023). IBM’s strategy for the future emphasizes not just financial success but also leadership in the areas of environmental responsibility and social responsibility. Under the advice and supervision of the board of directors and the committees that make up it, which have authority to oversee these areas, IBM’s top leadership bears responsibility for the business’s social and environmental sustainability (IBM, Citation2023).
4.4. Comparative analysis
In this segment, the ESG performance rankings of global electronics corporations derived through the integrated SF-AHP and SF-WASPAS approach are juxtaposed against the SF-TOPSIS and Fermatean fuzzy WASPAS methods (see Appendix). This comparative analysis aims to ascertain the reliability and precision of the proposed framework alongside evaluating its efficacy as a decision-making aid (Zavadskas et al., Citation2016).
Owing to its reliance on proximity to the positive ideal solution (PIS) and distance from the negative ideal solution (NIS), TOPSIS is regarded as a conventional MCDM technique (Yoon & Hwang, Citation2011). By enabling membership function mapping onto a multidimensional spherical surface, Kutlu Gündoğdu and Kahraman’s (Gündoğdu, Citation2019) introduction of SFS as an extension of IFS conferred greater flexibility. Meanwhile, the conception of Fermatean fuzzy sets by Senapati and Yager (Citation2020) proffered avenues to address ambiguity through integration of intuitionistic and PFSs while improving ambiguity-handling capabilities compared to IFS and PFS. As elucidated in and , the three models yield consistent ESG performance rankings for global electronics companies, affirming the validity of the integrated SF-AHP and SF-WASPAS approach proposed in this study. Minor divergences arose between SF-AHP-Fermatean fuzzy WASPAS and the suggested model for Microsoft Corp (EI5) and Flex Ltd. (EI10). Similarly, slight variances surfaced in the rankings of Siemens AG-Reg (EI2) and Nokia OYJ (EI9) between SF-AHP-TOPSIS and the recommended method. However, the overall concordance in results substantiates the potency and trustworthiness of the developed MCDM framework.
Table 9. Rankings’ comparisons.
In summary, the proposed integration of SF-AHP and SF-WASPAS constitutes a robust tool to assist decision makers, investors and regulatory authorities in identifying electronics industry leaders implementing exemplary ESG practices. The model’s empirical demonstration and comparative analyses reinforce its applicability across allied sectors as well (Wang et al., Citation2023). By meshing financial and ESG metrics through cutting-edge fuzzy techniques, this study meaningfully advances knowledge and practice underpinning sustainable investments globally.
One of the main motivations of corporate environmental initiatives is sustainable investing (Waring & Edwards, Citation2008). To bring responsible investing into the standard operating procedures of the financial system, this is essential to raise awareness among investors, companies and society about the need to collaborate on sustainability issues and by providing investment products that can meet investors’ needs (Husted & de Sousa-Filho, Citation2017). Although the method used in this research emphasizes that there is a distinct sustainable investing market, it further demonstrates the need to incorporate ESG considerations into standard investment strategies and encourage companies to pay more attention to ESG factors. Investors and partners in business are progressively anticipating goods that promote environmental responsibility, or at least are not harmful to the natural environment, respect workplace conditions, and engage in for a long-time corporate governance. Competing companies are compelled to modify their approach as a result of these market challenges, which new entrants utilize to differentiate themselves from them. Politicians and policymakers have initiated the incorporation of sustainability problems and an ESG structure, creating new and revised legal obligations.
5. Conclusion, implications, limitation and future works
5.1. Conclusions
This study develops a model incorporating key ESG criteria synthesized from major global electronics companies to enable a meticulous analysis of each parameter’s relative significance. By operationalizing a comprehensive MCDM approach, corporate ESG performance is evaluated from multiple perspectives. The SF-AHP findings denote environmental and governance dimensions are prioritized over social factors in investment decisions. Furthermore, pollution and waste, GHG emissions, eco-products/process strategies, natural loss, renewable energy and customer satisfaction exert overarching influence as sub-criteria, as elucidated through global weight analysis. The SF-WASPAS analysis furnishes nuanced rankings, with Intel Corp, Siemens AG-Reg, Nokia OYJ, Microsoft Corp and IBM emerging as sustainability frontrunners.
By amalgamating expert opinions and literature review, the research succeeds in identifying and assessing the most decisive ESG factors within leading electronics companies, constituting a salient contribution. This progressive integration of ESG considerations within the electronics industry holds monumental potential for fostering sustainable development. SF-AHP enables a systematic prioritization framework to evaluate criteria influence, underscoring ESG’s role in mitigating ecological and social risks. The proposed approach empowers organizations to make informed decisions aligned with sustainability objectives and recalibrate strategies to address evolving ESG dynamics.
Mainstreaming this model can help electronics companies augment their social legitimacy, attract ethical investments, improve stakeholder relations and make positive societal contributions. The framework was developed by identifying common factors utilized by ten of the world’s premier electronics manufacturers. Encompassing 16 key criteria spanning ESG dimensions, the model enables a multifaceted evaluation of corporate ESG performance. By applying a robust MCDM methodology, the study selects and weighs the most influential ESG factors within global electronics leaders, delivering actionable insights of considerable scholarly and practical value.
5.2. Managerial implications
The findings highlight the growing importance of ESG factors for electronics companies to attract investors and gain a competitive edge. Managers should align sustainability initiatives to meet ESG criteria and expectations.
Environmental concerns were revealed as the most significant ESG criterion. Electronics companies must prioritize reducing hazardous waste, improving energy efficiency and mitigating ecological impacts.
Effective governance was the next critical ESG factor. Managers should address ambiguities in governance policies through collaboration with standard-setting bodies and stakeholders.
Social factors were relatively less influential but remain relevant. Fostering community engagement and addressing inequality issues is vital for managers.
The proposed MCDM framework offers managers a robust tool to benchmark ESG performance against competitors and identify areas for improvement.
5.3. Theoretical implications
The study provides empirical evidence supporting the link between ESG performance and financial competitiveness in the electronics industry.
It highlights the effectiveness of combining SF-AHP and SF-WASPAS methods to evaluate ESG criteria and rankings under uncertainty.
The framework can be extended to other industries and sustainability assessment contexts involving subjective judgments.
Analysis of criteria weights offers insights into ESG priorities for electronics companies to guide academic research.
Comparative analysis with other MCDM techniques affirms the reliability of the hybrid SF-AHP-SF-WASPAS approach.
Findings emphasize the need for clearer governance guidelines and standards for the electronics industry through multi-stakeholder collaboration.
5.4. Limitations and future works
While this study has made significant contributions to the field of MCDM in the context of ESG factors and investment decisions, several limitations should be acknowledged, paving the way for future research directions.
First, one notable limitation is the absence of a sensitivity analysis concerning the threshold parameter (δ value) on the WASPAS technique, as well as the criteria and decision-expert weights. Future research endeavors should incorporate sensitivity analyses to assess the robustness of the proposed methodologies. By systematically varying the δ value and evaluating its impact on the outcomes, researchers can gain a deeper understanding of the method’s stability and reliability.
Second, the authors limited their comparative analysis to two other MCDM methods: Fermatean fuzzy WSPAS and spherical fuzzy TOPSIS. To enhance the comprehensiveness of research in this domain, future investigations should expand the scope of comparison by incorporating a wider array of integrated MCDM techniques. By doing so, researchers can offer a more comprehensive assessment of the proposed approaches, considering their performance relative to a diverse range of existing methods.
Third, this study primarily relied on conventional SFSs for its analysis. Future research holds the potential to explore and incorporate recent extensions of SFSs, such as spherical fuzzy Dombi, T-spherical fuzzy or interval-valued T-SFSs. Investigating these extensions could further refine and advance the methodology, potentially enhancing its applicability and effectiveness in real-world decision-making scenarios. Another noteworthy limitation of this study is its exclusive focus on ESG factors in the evaluation of investment opportunities. In future research endeavors, there is an opportunity to integrate quantitative factors alongside ESG considerations. By incorporating financial and quantitative data, researchers can develop a more comprehensive model that accounts for a broader spectrum of factors influencing investment decisions. This holistic approach may provide investors with a more nuanced and accurate perspective when making investment choices.
Disclosure statement
No potential conflict of interest was reported by the author(s).
Additional information
Notes on contributors
Phi-Dinh Hoang
Phi Dinh Hoang (Assoc. Prof. Dr.) has been the Rector of Hanoi School of Business and Management (HSB) under Vietnam National University Hanoi (VNU) since 2013. He is a reputable researcher and lecturer of interdisciplinary studies in Vietnam for both schools of thoughts on IMB (Integrated Management of Business) and MNS (Management of Nontraditional Security). He is also acting as the Vice Director of the Institute of Nontraditional Security (INS).
Ly-Thi Nguyen
Thi Ly Nguyen (Ph.D) is currently a Lecturer at HSB under VNU. She has a distinguished track record in research and significant skills in Industrial Engineering and Management, Sustainable Management, TRIZ, Data Envelopment Analysis, Technology Forecasting and Multiple-Criteria Decision Making.
Binh-Quoc Tran
Binh Quoc Tran (PhD Candidate) is a visiting lecturer at HSB under VNU. His main research focuses on State Policy, Management of SOEs, Management of Nontraditional Security and Management of Sustainable Development.
References
- Abdel-Baset, M., Chang, V., Gamal, A., & Smarandache, F. (2019). An integrated neutrosophic ANP and VIKOR method for achieving sustainable supplier selection: A case study in importing field. Computers in Industry, 106, 94–110. https://doi.org/10.1016/j.compind.2018.12.017
- Ahmad, H., Yaqub, M., & Lee, S. H. (2023). Environmental-, social-, and governance-related factors for business investment and sustainability: A scientometric review of global trends. Environment, Development and Sustainability, 1–23.
- Arvidsson, S., & Dumay, J. (2022). Corporate ESG reporting quantity, quality and performance: Where to now for environmental policy and practice? Business Strategy and the Environment, 31, 1091–1110. https://doi.org/10.1002/bse.2937
- Atanassov, K. T. (1999). Intuitionistic fuzzy sets. Intuitionistic fuzzy sets (pp. 1–137). Springer.
- Ballestero, E., Perez-Gladish, B., & Garcia-Bernabeu, A. (2014). The ethical financial question and the MCDM framework. Socially responsible investment: A multi-criteria decision making approach (pp. 3–22). Springer.
- Biloslavo, R., Bagnoli, C., & Edgar, D. (2018). An eco-critical perspective on business models: The value triangle as an approach to closing the sustainability gap. Journal of Cleaner Production, 174, 746–762. https://doi.org/10.1016/j.jclepro.2017.10.281
- British Safety Council. (2023). What is occupational health and safety? British Safety Council. https://www.britsafe.org/training-and-learning/find-the-right-course-for-you/informational-resources/what-is-occupational-health-and-safety-british-safety-council/ (accessed on 5 February 2023).
- Business Ethics: Definition. (2023). Business ethics: Definition principles, why they’re important. https://www.investopedia.com/terms/b/business-ethics.asp (accessed on 5 February 2023).
- Büyüközkan, G., & Güleryüz, S. (2016). An integrated DEMATEL-ANP approach for renewable energy resources selection in Turkey. International Journal of Production Economics, 182, 435–448. https://doi.org/10.1016/j.ijpe.2016.09.015
- Castañer, X., & Oliveira, N. (2020). Collaboration, coordination, and cooperation among organizations: Establishing the distinctive meanings of these terms through a systematic literature review. Journal of Management, 46(6), 965–1001. https://doi.org/10.1177/0149206320901565
- Cuong, B. C., & Kreinovich, V. (2013). Picture fuzzy sets-a new concept for computational intelligence problems. Proceedings of the 2013 Third World Congress on Information and Communication Technologies (WICT 2013) (pp 1–6). IEEE.
- Cuong, B. C., & Kreinovich, V. (2014). Picture fuzzy sets. Journal of Computer Science and Cybernetics, 30, 409–420. https://doi.org/10.15625/1813-9663/30/4/5032
- Eccles, R. G., & Klimenko, S. (2019). Shareholders are getting serious about sustainability. Harvard Business Review, 97, 106–116.http://mithconference.com/wp-content/uploads/2019/10/MSIS_Rome-8th-Oct_Eccles_20191010.pdf
- Eliwa, Y., Aboud, A., & Saleh, A. (2021). ESG practices and the cost of debt: Evidence from EU countries. Critical Perspectives on Accounting, 79, 102097. https://doi.org/10.1016/j.cpa.2019.102097
- Erdoğan, M., Kaya, İ., Karaşan, A., & Çolak, M. (2021). Evaluation of autonomous vehicle driving systems for risk assessment based on three-dimensional uncertain linguistic variables. Applied Soft Computing, 113, 107934. https://doi.org/10.1016/j.asoc.2021.107934
- Escrig-Olmedo, E., Rivera-Lirio, J. M., Muñoz-Torres, M. J., & Fernández-Izquierdo, M. Á. (2017). Integrating multiple ESG investors’ preferences into sustainable investment: A fuzzy multicriteria methodological approach. Journal of Cleaner Production, 162, 1334–1345. https://doi.org/10.1016/j.jclepro.2017.06.143
- Economy & Forecast review. (2022). ESG Integration is an Opportunity for Companies to Refine, Protect and Create Business Value. https://kinhtevadubao.vn/tich-hop-esg-la-co-hoi-de-cac-cong-ty-tinh-chinh-bao-ve-va-tao-ra-gia-tri-kinh-doanh-22088.html (accessed on 26 December 2022)
- Garibaldi, J. M., & Ozen, T. (2007). Uncertain fuzzy reasoning: A case study in modelling expert decision making. IEEE Transactions on Fuzzy Systems, 15(1), 16–30. https://doi.org/10.1109/TFUZZ.2006.889755
- Ghoul, S., & El Karoui, A. (2022). Fund performance and social responsibility: New evidence using social active share and social tracking error. Journal of Banking & Finance, 143, 106598. https://doi.org/10.1016/j.jbankfin.2022.106598
- Gündoğdu, F. K. (2019 Principals of spherical fuzzy sets [Paper presentation]. Intelligent and Fuzzy Techniques in Big Data Analytics and Decision Making: Proceedings of the INFUS 2019 Conference (pp. 15–23). Istanbul, Turkey, July 23–25. Springer International Publishing.
- Gürbüz, T., & Albayrak, Y. E. (2014). An engineering approach to human resources performance evaluation: Hybrid MCDM application with interactions. Applied Soft Computing, 21, 365–375. https://doi.org/10.1016/j.asoc.2014.03.025
- Hoang, P. D., Nguyen, H. Q., Nguyen, K. X., & Hoang, T. A. (2022). Management of nontraditional security for Vietnam’s sustainable development: An integrated approach. Sustainability: Science, Practice, & Policy, 18(1), 696–709. https://doi.org/10.1080/15487733.2022.2111066
- Hoepner, A., Oikonomou, I., Scholtens, B., & Schröder, M. (2016). The effects of corporate and country sustainability characteristics on the cost of debt: An international investigation. Journal of Business Finance & Accounting, 43, n/a–n/a. https://doi.org/10.1111/jbfa.12183
- Husted, B. W., & de Sousa-Filho, J. M. (2017). The impact of sustainability governance, country stakeholder orientation, and country risk on environmental, social, and governance performance. Journal of Cleaner Production, 155, 93–102. https://doi.org/10.1016/j.jclepro.2016.10.025
- IBM. (2023). ESG Report. https://www.ibm.com/impact/files/reports-policies/2021/IBM_2021_ESG_Report.pdf (accessed on 6 March 2023).
- Intel. (2023). ESG - Investor Relations : Intel Corporation (INTC). Available online: https://www.intc.com/esg (accessed on 6 February 2023).
- Isabel Al-Dhahir. (2023). Decarbonizing the Electronics Industry with Sustainable Manufacturing. https://esgnews.com/decarbonizing-the-electronics-industry-with-sustainable-manufacturing/ (accessed on 6 August 2023).
- Jianqiang, G., Rong, L., & Juan, X. (2022). Data element embedding and firm performance: The influence of ESG investment. Frontiers in Environmental Science, 10, 974399. https://doi.org/10.3389/fenvs.2022.974399
- Joof, F., Samour, A., Tursoy, T., & Ali, M. (2022). Climate change, insurance market renewable energy, and biodiversity: Double-materiality concept from BRICS countries. Environmental Science and Pollution Research, 30(11), 28676–28689. https://doi.org/10.1007/s11356-022-24068-4
- Jovanovic, M., Afgan, N., & Bakic, V. (2010). An analytical method for the measurement of energy system sustainability in urban areas. Energy, 35(9), 3909–3920. https://doi.org/10.1016/j.energy.2010.06.010
- Kahraman, C., Onar, S. C., & Oztaysi, B. (2019). Customer segmentation method determination using neutrosophic sets. Proceedings of the International Conference on Intelligent and Fuzzy Systems (pp. 517–526). Springer.
- Kitsios, F., & Kamariotou, M. (2020). Mapping new service development: A review and synthesis of literature. The Service Industries Journal, 40(9–10), 682–704. https://doi.org/10.1080/02642069.2018.1561876
- Kitsios, F., Kamariotou, M., & Talias, M. A. (2020). Corporate sustainability strategies and decision support methods: A bibliometric analysis. Sustainability, 12(2), 521. https://doi.org/10.3390/su12020521
- Kraus, S., Schiavone, F., Pluzhnikova, A., & Invernizzi, A. C. (2021). Digital transformation in healthcare: Analyzing the current state-of-research. Journal of Business Research, 123, 557–567. https://doi.org/10.1016/j.jbusres.2020.10.030
- Kutlu Gündoğdu, F., & Kahraman, C. (2019). A novel fuzzy TOPSIS method using emerging interval-valued spherical fuzzy sets. Engineering Applications of Artificial Intelligence, 85, 307–323. https://doi.org/10.1016/j.engappai.2019.06.003
- Kutlu Gündoğdu, F., & Kahraman, C. (2020a). A novel spherical fuzzy analytic hierarchy process and its renewable energy application. Soft Computing, 24(6), 4607–4621. https://doi.org/10.1007/s00500-019-04222-w
- Kutlu Gündoğdu, F., & Kahraman, C. (2020b). Spherical fuzzy analytic hierarchy process (AHP) and its application to industrial robot selection. Proceedings of the Advances in Intelligent Systems and Computing (Vol. 1029, pp. 988–996). Springer.
- Kutlu Gündoğdu, F., & Kahraman, C. (2020c). Spherical fuzzy sets and decision making applications. Proceedings of the Advances in Intelligent Systems and Computing (Vol. 1029, pp. 979–987). Springer.
- Kutlu Gündoğdu, F., & Kahraman, C. (2021). Optimal site selection of electric vehicle charging station by using spherical fuzzy TOPSIS method. Studies in Fuzziness and Soft Computing (Vol. 392, pp. 201–216). Springer.
- Lu, M. T., Tsai, J. F., Shen, S. P., Lin, M. H., & Hu, Y. C. (2020). Estimating sustainable development performance in the electrical wire and cable industry: Applying the integrated fuzzy MADM approach. Journal of Cleaner Production, 277. https://doi.org/10.1016/j.jclepro.2020.122440
- MACROFAB. (2023). How ESG Initiatives Create Value for the PCBA Industry. https://www.macrofab.com/blog/esg-create-value-pcba-industry/ (accessed on 6 August 2023).
- Mahmood, T., Ullah, K., Khan, Q., & Jan, N. (2019). An approach toward decision-making and medical diagnosis problems using the concept of spherical fuzzy sets. Neural Computing and Applications, 31(11), 7041–7053. https://doi.org/10.1007/s00521-018-3521-2
- Mardani, A., Saraji, M. K., Mishra, A. R., & Rani, P. (2020). A novel extended approach under hesitant fuzzy sets to design a framework for assessing the key challenges of digital health interventions adoption during the COVID-19 outbreak. Applied Soft Computing, 96, 106613. https://doi.org/10.1016/j.asoc.2020.106613
- Marikyan, D., Papagiannidis, S., & Alamanos, E. (2019). A systematic review of the smart home literature: A user perspective. Technological Forecasting and Social Change, 138, 139–154. https://doi.org/10.1016/j.techfore.2018.08.015
- Microsoft. (2023). Microsoft Cloud for Sustainability is Growing. https://www.microsoft.com/en-us/sustainability/cloud (accessed on 5 March 2023).
- Mohammad, W. M. W., & Wasiuzzaman, S. (2021). Environmental, social and governance (ESG) disclosure, competitive advantage and performance of firms in Malaysia. Cleaner Environmental Systems, 2, 100015. https://doi.org/10.1016/j.cesys.2021.100015
- Nguyen, T. L., Nguyen, P. H., Pham, H. A., Nguyen, T. G., Nguyen, D. T., Tran, T. H., Le, H. C., & Phung, H. T. (2022). A novel integrating data envelopment analysis and spherical fuzzy MCDM approach for sustainable supplier selection in steel industry. Mathematics, 10(11), 1897. https://doi.org/10.3390/math10111897
- NOKIA (2023). Nokia Takes ESG Strategy to Next Level of Impact. https://www.nokia.com/about-us/news/releases/2022/10/31/nokia-takes-esg-strategy-to-next-level-of-impact/ (accessed on 5 March 2023).
- OECD. (2022). Environmental Social and Governance (ESG) Investing. https://www.oecd.org/finance/esg-investing.htm (accessed on 25 December 2022).
- Otten, A., & Ollier, C. (1976). Fuzzy sets. Books Abroad, 50(3), 610. https://doi.org/10.2307/40130726
- Park, S. R., & Jang, J. Y. (2021). The impact of ESG management on investment decision: Institutional investors’ perceptions of country-specific ESG criteria. International Journal of Financial Studies, 9(3), 48. https://doi.org/10.3390/ijfs9030048
- Patel, A. B., & Desai, T. N. (2019). A systematic review and meta-analysis of recent developments in sustainable supply chain management. International Journal of Logistics Research and Applications, 22(4), 349–370. https://doi.org/10.1080/13675567.2018.1534946
- Pedersen, L. H., Fitzgibbons, S., & Pomorski, L. (2021). Responsible investing: The ESG-efficient frontier. Journal of Financial Economics, 142(2), 572–597. https://doi.org/10.1016/j.jfineco.2020.11.001
- Quteishat, A., & Younis, M. A. A. (2023). Strategic renewable energy resource selection using a fuzzy decision-making method. Intelligent Automation & Soft Computing. https://doi.org/10.32604/iasc.2023.029419
- Radwan, N. M., Senousy, M. B., & Alaa El Din, M. R. (2016). Neutrosophic AHP multi criteria decision making method applied on the selection of learning management system. International Journal of Advancements in Computing Technology, 8, 95–105.https://fs.unm.edu/neut/NeutrosophicAHP.pdf
- Raghunandan, A., & Rajgopal, S. (2022). Do ESG funds make stakeholder-friendly investments? Review of Accounting Studies, 27(3), 822–863. https://doi.org/10.1007/s11142-022-09693-1
- Reig-Mullor, J., Garcia-Bernabeu, A., Pla-Santamaria, D., & Vercher-Ferrandiz, M. (2022). Evaluating ESG corporate performance using a new neutrosophic AHP-TOPSIS based approach. Technological and Economic Development of Economy, 28(5), 1242–1266. https://doi.org/10.3846/tede.2022.17004
- Ruan, L., & Liu, H. (2021). Environmental, Social, Governance activities and firm performance: Evidence from China. Sustain, 13(2), 767. https://doi.org/10.3390/su13020767
- Samanlioglu, F., & Aya, Z. (2017). A fuzzy AHP-PROMETHEE II approach for evaluation of solar power plant location alternatives in Turkey. Journal of Intelligent & Fuzzy Systems, 33, 1–13. https://doi.org/10.3233/JIFS-162122
- Schiemann, F., & Tietmeyer, R. (2022). ESG controversies, ESG disclosure and analyst forecast accuracy. International Review of Financial Analysis, 84, 102373. https://doi.org/10.1016/j.irfa.2022.102373
- Senapati, T., & Yager, R. R. (2020). Fermatean fuzzy sets. Journal of Ambient Intelligence and Humanized Computing, 11(2), 663–674. https://doi.org/10.1007/s12652-019-01377-0
- Shabani, A., Shabani, A., Ahmadinejad, B., & Salmasnia, A. (2022). Measuring the customer satisfaction of public transportation in Tehran during the COVID-19 pandemic using MCDM techniques. Case Studies on Transport Policy, 10(3), 1520–1530. https://doi.org/10.1016/j.cstp.2022.05.009
- Shahnazari, A., Rafiee, M., Rohani, A., Nagar, B. B., Ebrahiminik, M. A., & Aghkhani, M. H. (2020). Identification of effective factors to select energy recovery technologies from municipal solid waste using multi-criteria decision making (MCDM): A review of thermochemical technologies. Sustainable Energy Technologies and Assessments, 40, 100737. https://doi.org/10.1016/j.seta.2020.100737
- Shams, S. M. R., Vrontis, D., Chaudhuri, R., Chavan, G., & Czinkota, M. R. (2020). Stakeholder engagement for innovation management and entrepreneurial development: A meta-analysis. Journal of Business Research, 119, 67–86. https://doi.org/10.1016/j.jbusres.2020.08.036
- Simsek Yagli, B., Dogan, N. O., & Yagli, I. (2022). Weighting ESG criteria of banks by using interval valued intuitionistic fuzzy best worst method. Proceedings of the International Conference on Intelligent and Fuzzy Systems (pp. 598–605). Springer.
- Singh, R. K., Murty, H. R., Gupta, S. K., & Dikshit, A. K. (2012). An overview of sustainability assessment methodologies. Ecological Indicators, 15(1), 281–299.
- Smarandache, F. (1999). A unifying field in logics. Neutrosophic Probability, Set and Logic.
- Strantzali, E., & Aravossis, K. (2016). Decision making in renewable energy investments: A review. Renewable and Sustainable Energy Reviews, 55, 885–898. https://doi.org/10.1016/j.rser.2015.11.021
- Torra, V. (2010). Hesitant fuzzy sets. International Journal of Intelligent Systems, 25(6), 529–539. https://doi.org/10.1002/int.20418
- Vargas, Z. (2013). Labor rights are civil rights. Labor rights are civil rights. Princeton University Press. ISBN 1400849284.
- Vural-Yavaş, Ç. (2021). Economic policy uncertainty, stakeholder engagement, and environmental, social, and governance practices: The moderating effect of competition. Corporate Social Responsibility and Environmental Management, 28, 82–102. https://doi.org/10.1002/csr.2034
- Wang, L., Fan, X., & Zhuang, H. (2023). ESG disclosure facilitator: How do the multiple large shareholders affect firms’ ESG disclosure? Evidence from China. Frontiers in Environmental Science, 11, 1063501. https://doi.org/10.3389/fenvs.2023.1063501
- Waring, P., & Edwards, T. (2008). Socially Responsible Investment: Explaining Its Uneven Development and Human Resource Management Consequences. Corporate Governance: An International Review, 16(3), 135–145. https://doi.org/10.1111/j.1467-8683.2008.00676.x
- What Are Employee Rights. (2023). Employee rights are a set, are entitled to under law. https://www.quitgenius.com/hr-glossary/employee-rights#:∼:text= (accessed on 5 February 2023).
- WIKIPEDIA (2023). Resource Depletion. https://en.wikipedia.org/wiki/Resource_depletion (accessed on 5 February 2023).
- Yager, R. R. (1986). On the theory of bags. International Journal of General Systems. 13(1), 23–37. https://doi.org/10.1080/03081078608934952
- Yager, R. R. (2013). Pythagorean fuzzy subsets. Proceedings of the Proceedings of the 2013 Joint IFSA World Congress and NAFIPS Annual Meeting, IFSA/NAFIPS 2013 (pp. 57–61). IEEE.
- Yager, R. R. (2017). Generalized orthopair fuzzy sets. IEEE Transactions on Fuzzy Systems, 25(5), 1222–1230. https://doi.org/10.1109/TFUZZ.2016.2604005
- Yoon, K., & Hwang, C. L. (2011). Multiple attribute decision making (Vol. 104). Sage Publications.
- Zavadskas, E. K., Govindan, K., Antucheviciene, J., & Turskis, Z. (2016). Hybrid multiple criteria decision-making methods: A review of applications for sustainability issues. Economic Research-Ekonomska Istraživanja, 29, 857–887. https://doi.org/10.1080/1331677X.2016.1237302
- Zavadskas, E. K., Turskis, Z., Antucheviciene, J., & Zakarevicius, A. (2012). optimization of weighted aggregated sum product assessment. Elektron ir Elektrotechnika, 122, 3–6. https://doi.org/10.5755/j01.eee.122.6.1810
Appendix
A1: Fermatean Fuzzy WASPAS
Senapati and Yager (Citation2020) introduced Fermatean fuzzy sets as a method for facilitating ways to handle of ambiguous data. These three factors are described as the degree of membership the degree of non-membership
and the degree of indeterminacy
illustrates the distinctions between the membership degree spaces of IMS, PMGs and FMGs. The following are definitions relating to FFs:
Definition 1.
Let X represent the context of discourse. A Fermatean fuzzy set F in X has the following element form: where
with the condition
For any FFS
and
the indeterminacy degree of
with respect to
is described as follow (Senapati & Yager, Citation2020):
(1)
(1)
For convenience, the symbol is referred to as follows: for the FFS
The Fermatean fuzzy numbers (FFNs) were presumed to be FFS elements for the sake of simplicity.
The set operations on FFS are given in EquationEquations (2)–(5).
Definition 2.
Assuming are two FFSs, and
0, the following operations have been established:
(2)
(2)
(3)
(3)
(4)
(4)
(5)
(5)
Definition 3.
The complement function of Fermatean fuzzy set is given in EquationEquation (6)
(6)
(6)
(6)
(6)
Definition 4.
The scoreƻ function () and accuracy function (
) for this FFS are established as follows:
(7)
(7)
where
(8)
(8)
where
Definition 5.
Assuming are two FFSs, and
are the score functions, respectively, then
If
If
If
Definition 6.
Assuming are two FFSs, and
are the score functions, respectively, then
are two FFSs, and
If
If
If
If
If
Definition 7.
Suppose is the set of
FFS, and
is the weight vector for
where
FF weighted average aggregation operator FFWA is established as follows:
(9)
(9)
Definition 8.
Equation specifies the positive defuzzified value in EquationEquation (10)(10)
(10)
(10)
(10)
where
Fermatean fuzzy WASPAS
In this section, we intend to present a new effective method for evaluating ESG management in global electronics industry in an uncertain environment based on Fermatean fuzzy sets and WASPAS. The preceding section introduced the definitions and operators of Fermatean fuzzy sets, which are utilized to extend the WASPAS technique.
Step 1. Compile a list of alternatives. The decision makers evaluate the items using the linguistic terms associated with each criterion. contains a translation of linguistic terms into Fermatean fuzzy crisp numbers.
Table A1.1. The linguistic terms and FFSs.
Step 2. In this step, decision makers need to define language terms along with their respective FFSs.
Step 3. The evaluation procedure uses linguistic terms based on Fermatean fuzzy sets. Here, the assessment of the alternative by the
decision maker based on the
criterion is represented by:
Step 4. Aggregate the assessments made by decision makers. In the preceding section, we constructed an aggregation operator using EquationEquation (9)(9)
(9) . Using this equation
we should sum each DM's evaluations in Step 3. Therefore, the aggregated evaluations or decision matrix elements
are presented as follows:
(11)
(11)
Step 5. The decision matrix must be normalized. If there are non-beneficial (cost) criteria, we must make adjustments. In this study, we transform the values associated with non-beneficial criteria using the concept of the FFS complement. EquationEquation (6)(6)
(6) defines the complement of an FFS. Let BC and NC represent the beneficial and non-beneficial criteria, respectively. The normalized decision matrix components can be determined as follows:
(12)
(12)
Step 6. The WSM and WPM values need to be calculated using the defined operators of FFSs (addition, multiplication, etc.) in EquationEquations (2)–(5).
(13)
(13)
(14)
(14)
Step 7. Perform the WASPAS calculation. Combining the WSM and WPM metrics produces the WASPAS metric. At this juncture, we must establish and set the value of a combination parameter The formula that follows is utilized for calculations in this step:
(15)
(15)
Step 8. Rank the options according to the positive score values of We utilize Definition 6, to evaluate the value of
and rank the relative values of the alternatives.
A2: SF-TOPSIS method
Table A2.1. Linguistic scales.
Step 1: Let DMs to populate matrices for decisions with linguistic terms from . Then, DMs may assess the criteria to determine their relative weights.
Step 2: Aggregate the judgments of each DM using EquationEquation (16)(10)
(10) . Aggregated Spherical Fuzzy decision matrix is constructed using EquationEquation (16)
(10)
(10) .
(16)
(16)
Step 3: Normalize the accumulated weights of the criteria using EquationEquation (17)(11)
(11) . Defuzzify the aggregated weighted decision matrix by utilizing the suggested score function by using EquationEquation (18)
(12)
(12) .
(17)
(17)
(18)
(18)
Step 4: Calculate the spherical fuzzy positive ideal solution (SF-PIS) and the spherical fuzzy negative ideal solution (SF-NIS). EquationEquations (19)(13)
(13) and Equation(20)
(14)
(14) are used for the SF-PIS:
(19)
(19)
(20)
(20)
EquationEquations (21)(15)
(15) and Equation(22)
(16)
(16) are used for the SF-NIS:
(21)
(21)
(22)
(22)
Step 5: Measuring the distances of SF-NIS and SF-PIS between alternative using EquationEquations (23)
(17)
(17) and Equation(24)
(18)
(18) :
(23)
(23)
(24)
(24)
Step 6: EquationEquations (25)(19)
(19) and Equation(26)
(20)
(20) are applied to obtain maximum and minimum distances of SF-NIS and SF-PIS:
(25)
(25)
(26)
(26)
Step 7: To acquire zero or positive outcomes, EquationEquation (12)(12)
(12) is used to determine the optimal ranking order of the options and to pick the best option based on increasing values of the closeness ratio:
(27)
(27)