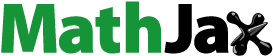
Abstract
In this work, we propose fractional-order deterministic mathematical models for human brain information dynamics, including the major effect on sensory memory. The two models are top-down and bottom-up processing. Mathematical models are analysed for different cases for long and short-term memory effects with the effect of experience and prior knowledge. Studies are conducted both qualitatively and quantitatively to analyse systems using fixed-point theory results. The impact of the global derivative, linear growth, and Lipschitz criteria are used to check the existence and uniqueness of the model for the biological feasibility of the system. Positivity and boundedness of the unique solution were also treated. The global stability of the proposed model is constructed by constructing the first and second derivatives for the Lyapunov function using wave analysis according to the equilibrium point. Numerical simulations of the proposed method in the range of fractional orders are conducted to demonstrate the implications of fractional and fractal orders. To further explore the impact of various parameters on the information processing process in the human brain, information in long-term memory, repetition, and rehearsal Chaotic modelling of the brain information dynamics model is also derived for different cases that are stable and bound to feasible regions. Results demonstrate the strong memory effect by using nonlocal and non-singular kernels at different fractional order values and fractal dimensions, which support experimental and theoretical observations.
1. Introduction
The very first step in perceiving stimuli is represented by sensory memory. It is related to the senses, and each type of sensory perception seems to play a unique role with its own set of limitations and resources. It is crucial that fresh information go swiftly to the next step of processing. Information kept in this stage of memory is temporally constrained, so if it isn’t transferred to the level after it, it starts to deteriorate quickly. For visual stimuli, this happens in as little as 1/2 seconds, whereas for auditory stimuli, it takes three seconds. In order to do this, the two main factors influencing the sensory system are attention and automatic (Huitt, Citation2000). There are numerous categories for long-term memory, and numerous hypotheses exist about the representation (Abbo Citation2002) of memory units in the mind (Abbot, Citation2002). The structure of the human brain, which has 86 billion connected neurons and almost 150 trillion synapses, allows for transmission between neurons and body parts. Brains are dynamic systems that exhibit several non-linear levels (Herculano-Houzel, Citation2009). It is essential to fully comprehend the non-linear dynamics of the brain in order to comprehend how it functions (McKenna et al., Citation1994). According to the model described in (Wei et al., Citation2012), it is strongly predicted that identical or extremely similar points on a feature dimension cannot be individually stored; instead, they would merge. In particular (Kargarnovin et al., Citation2023; Zekveld et al., Citation2006), make comments on top-down, to better comprehend the underlying principles of behavioural, cognitive, and perceptual processes, the human brain is hypothesised to be modelled as a complex system (Jeffrey et al., Citation2007). Non-linear dynamical techniques are utilised to understand the underlying brain activities in EEG signals and assess their physiological implications. The study of biological time series, such as heart rates, respiratory records, and especially EEG, is particularly adapted to the study of the ‘chaos’ attribute, one of the properties of non-linear dynamical systems (Justin et al., Citation2020; Oestreicher, Citation2007; Pritchard & Duke, Citation1995). Deterministic systems that exhibit complicated and seemingly random behaviours are addressed by the theory of non-linear dynamical systems and the chaos theory (Natarajan et al., Citation2004). Plotting the behaviour trajectories of dynamic systems has led to the development of mathematical equations that can forecast the states of the system in the future (Stam, Citation2005).
Fractional calculus (FC) is necessary to solve difficulties in the real world. Many different scientific, engineering, and financial fields make extensive use of it. Fractional integrals and derivatives of fractional order are the FC’s defining features. In recent years, researchers’ interest in fractional calculus and the different facets of that field of study has increased. This is because genetic mutations are a key method for describing the dynamic behaviour of various biological systems (Akgül, Citation2019; Atangana & Akgül, Citation2020; Toufik & Atangana, Citation2017). In the context of a double Laplace transform coupled to the Adomian approach for a fractional Caputo-Fabrizio operator in (Rahman et al., Citation2022). The mathematical modelling of the human brain’s response to external stimuli has received less attention despite certain advancements in studies connected to this field of brain research (Namazi & Kulish, Citation2015). In the Hodgkin-Huxley model, fractional-order derivatives have been used to simulate the dynamics of a single neuron in neuroscience (Baleanu et al., Citation2012; Coutin et al., Citation2018). Using Caputo’s fractional derivative temporal order and other operators, we develop a mathematical model of a fractional-order neural field in this study (González-Ramírez, Citation2022; Weinberg, Citation2015; Zhao & Robinson, Citation2015). The central nervous system (CNS) is commonly affected by multiple sclerosis (MS), a neurological condition that has been linked to lesions that can spread over time and space, according to research from (Khan et al., Citation2023). Studies on the use of the fractional operator in the BAM neural network, chemical reaction delays, and predator prey systems can be found in (Xu et al., Citation2023a, Citation2023b, Citation2023c).
The portions of this research article are as follows: Section 1 of the proposal model is given a thorough introduction. The model can be solved using a few fundamental fractional order derivatives from Section 2. In Section 3, you may find a generalised version of the model and an examination of the suggested model description. In Section 4 The suggested model’s qualitative analysis, including its equilibrium points, existence, and uniqueness, is examined. Lyapunov stability is examined in Section 4 investigation of the suggested model’s stability. In Section 5, we look at the Mittage-Leffler kernel’s numerical structure. In Sections 6 and 7, respectively, talk about numerical simulations and the conclusion.
2. Some important definition
In the event that there are even more complex phenomena that cannot be duplicated using fractional operators, this may provide a superior set of mathematical tools to handle these behaviours (Atangana, Citation2020). Despite the fact that it has been proven that these new operators are capable of modelling complex problems, concerns have been raised due to the operators’ inability to handle time at the origin. The modified fractal fractional operators will be introduced, discussed, and numerically approximated using tried-and-true techniques like Lagrange and Newton polynomials in this section.
Definition 2.1.
Let be a function that isn’t always differentiable. Let’s say that κ1 is a fractal dimension and α is a fractional order, and that
and
. we have
(1)
(1)
where (Atangana, Citation2020)
Integral for power law kernel is given as
(2)
(2)
Definition 2.2.
Fractional operator in the sense of exponential decay kernel is
(3)
(3)
Integral for exponential decay kernel is given as
(4)
(4)
Definition 2.3.
Fractional operator in the sense of Mittag Leffler kernel is
(5)
(5)
Integrals for Mittag Leffler kernel is given as
(6)
(6)
3. Fractional order model of sensory memory
Considering the deterministic mathematical model for sensory memory to study the information dynamics in the human brain. Models provide the motivation to explore the human brain with fractional operators having more degree of freedom to study human memory with different ways of transmission. When attention is paid to the environment, sensory registers in the sensory memory process the information and store it in short-term memory. The duration of the information’s stay in the short-term memory is dependent on mechanisms for repetition and rehearsal. By maintaining rehearsal, information that is still in short-term memory can be transferred to long-term memory. It seems naturally that these disturbances must respond instantly in the form of impulses. The two models presuppose: The brain being studied is in good physical condition. The encoding and storage systems use either bottom-up processing or top-down processing, but not both. All the compartments have unlimited storage capacity. A fractal fractional operator is used in the fractional order differential equations that represent the sensory memory model from bottom-up processing found in (Samuel et al., Citation2015).
(7)
(7)
with the initial conditions
(8)
(8)
Here, environmental data is processed into the sensory memory and causes the long-term memory (LTM) to automatically recall information of a similar kind that has been stored there. As a result, the information is given less attention and obtains a lower percentage of integration into the short-term and long-term memories, respectively. The fractional operator is used in the equation that follows to develop a top-down sensory memory model (Samuel et al., Citation2015).
(9)
(9)
with the initial conditions
(10)
(10)
a: Sensory Memory the amount of information (bottom-up processing).
b: In the Short-Term Memory the amount of information (bottom-up processing).
c: In the Long-Term Memory the amount of information at time (bottom-up processing)
x: In the Sensory Memory the amount of information at time
y: In the Short-Term Memory the amount of information at time.
z: In the Long-Term Memory the amount of information at time.
I(t): rate sensory memory time t.
e: Coefficient of forgetfulness.
d1: Attention coefficient from Bottom-up processing.
d2: Top-down processing attention coefficient.
f: Rehearsal Coefficient.
p: Repetition Coefficient.
u: Retrieval Coefficient.
k: undetermined constant.
v: Automatically coefficient.
w: Coefficient of response.
3.1. Positiveness and boundedness of solutions
In this section, we study some important properties of the model
Lemma 3.1.
Let initial conditions be
Then if the solutions {a, b, c} exist, they are all positive t > 0
Proof.
We start with a.
which then results in
(11)
(11)
This proves that a(t) is positive. However, there is also that
Now Let the case of b(t)
Given that we assumed that every solution has the same sign, it is evident that
Therefore
which then results in
(12)
(12)
This demonstrates that for b(t) is positive. However, we also have that
Now let the case of c(t)
Given that we assumed that every solution has the same sign, it is evident that
This results in
This demonstrates that for c(t) is positive. However, we also have that
(13)
(13)
Norm is defined as
(14)
(14)
It is essential to note that due to the system’s biological correctness
(15)
(15)
Now, similarly (9) system
(16)
(16)
(17)
(17)
(18)
(18)
□
Theorem 3.1.
It is true for if a function
is continuous and bounded for
(19)
(19)
Proof.
It should be emphasised for the sake of proof that since is continuous and bounded,
there exists
such that
(20)
(20)
(21)
(21)
We now suppose that is bounded, then
(22)
(22)
We will now talk about the prerequisites with operator for uniqueness.
In order to achieve this, we will provide the definition listed below.
(23)
(23)
then
Now, a(t)
(24)
(24)
Dividing by so we have
(25)
(25)
Then,
(26)
(26)
Taking class b into consideration, we have the following
(27)
(27)
dividing by
produce
so
(28)
(28)
Consider the c class, we have the following
(29)
(29)
dividing by
produce
so
Therefore, we write
(30)
(30)
(31)
(31)
(32)
(32)
Similarly for (9) system, we write
(33)
(33)
(34)
(34)
(35)
(35)
□
Theorem 3.2.
The solutions given by models (7) and (9) are distinct and constrained in depending on the initial conditions.
Proof.
We control it by
(36)
(36)
According to EquationEq. (36)(36)
(36) , the solution cannot escape the hyper plane if
A vector field pointing towards
exists in each hyper-plane around the non-negative or positive orthant, indicating that the domain is positively invariant. Similarly, for (7) system
(37)
(37)
If
the solution cannot escape the hyperplane, according to EquationEq. (37)
(37)
(37) . □
3.2 Positive Solutions with Non-local Operators
We demonstrate the positiveness of solutions in this section with all positive initial conditions.
The following results for a power law kernel can be obtained if we follow the identical steps for fractal fractional operators (Rahman et al., Citation2022).
q is time component.
With an exponential kernel, we have
With a Mittag Leffler kernel, we have
Now, similarly for (9) system. With power law kernel
q is time component.
With an exponential kernel, we get
With a Mittag Leffler kernel, have
4. Qualitative analysis of the proposed model
4.1. Equilibrium points of models
The equilibrium points of models system (7) and (9) are obtained by setting and
The endemic equilibrium points of (7), where
(38)
(38)
The endemic equilibrium points of (9), where
(39)
(39)
4.2. Existence and uniqueness
It is necessary to show the following theorem in order to do this.
Theorem 4.1.
Assume that there are positive constants, λi, so that
(40)
(40)
(41)
(41)
Proof.
Now consider our model
(42)
(42)
We start with the function Then, we will show that
(43)
(43)
Then, we write
(44)
(44)
where
(45)
(45)
where
(46)
(46)
where
We double checked the beginning condition for each function. The second criterion of our model has now been verified.
(47)
(47)
under the condition
(48)
(48)
under the condition
(49)
(49)
under the condition
The solution to our system therefore exists and is unique given the circumstances.
(50)
(50)
We now recall our (9) model
(51)
(51)
The function serves as our starting point. Next, we’ll demonstrate this
(52)
(52)
Then, we write
(53)
(53)
where
(54)
(54)
where
(55)
(55)
where
We verified each function’s initial condition twice. The second criterion of our model has now been verified.
(56)
(56)
under the condition
(57)
(57)
under the condition
(58)
(58)
under the condition
The solution to our system therefore exists and is unique in light of the circumstances.
(59)
(59)
□
4.3. Stability analysis of the proposed models
To better understand the influence of control strategies on the dynamics, the stability of the model system in (7) and (9) is explored in order to learn more about its dynamical features. We start by looking into the stability properties of the brain models.
All independent variables in the model were created using the endemic Lyapunov function; in this case, the destructive equilibrium is represented by the equations
Lemma 4.1.
For any , suppose that
is a continuous function,
(60)
(60)
4.3.1. First derivative of Lyapunov
Theorem 4.2.
The Lyapunov function, {a, b, c}, L < 0 are endemic functions. The endemic equilibrium is . The suggested model’s endemic equilibrium points
are globally asymptotically stable when the reproductive number
Proof.
The following is how the theorem is established using the Lyapunov function:
(61)
(61)
Now applying the lemma (4.1).
The following is how their derivative values may now be written
substituting values
and
and can be organized as follows
(62)
(62)
For clarity, we may rewrite the above equivalency as follows
where
and
It is achieved that if this yields
however when
and
(63)
(63)
The recommended model has the largest compact invariant set, as can be shown.
The model under examination has an endemic equilibrium called The Lasalles invariance principle states that if
is globally asymptotically stable in Γ, then
is also worldwide asymptotically stable in Γ. □
4.3.2. Second derivative of Lyapunov
The first derivative of the Lyapunov function is used to gauge the global stability of endemic equilibrium points. We get important information from the first derivative analysis that the second derivative analysis might improve without losing generality. For instance, the first derivative of these Lyapunov functions indicates the progression of the illness, and the second derivative, depending on its sign, indicates the curvature. From our perspective, the second derivative will provide additional information.
(64)
(64)
Now putting the second derivative values of Then we have
By substituting the appropriate formulas for and
and combining positive and negative factors, we have
Then we have
Following that, the second-order sign’s significance is explained. Similarly results are satisfied for model (9) which fulfil above drive conditions.
5. Numerical scheme
The suggested mathematical model for the brain model is used in this part to apply the new differential and integral techniques. In this case, the operator with a Mittag Leffler kernel will take the place of the differential operator. The flexible order version will also be used.
For ease of use, we have
Where
For Mittag Leffler kernel, we have
The following numerical scheme can be obtained.
(65)
(65)
(66)
(66)
(67)
(67)
The (9) mathematical model is similarly implemented using the new differential and integral operators. The numerical representation of the system is as follows.
6. Numerical simulations and discussion
The fractional-order human brain model has been studied mathematically. To determine how the fractional derivative may affect the compartments, numerical simulations are run depending on the parameter values. The end-time value of a particular parameter may be examined using a variety of numerical approaches to study how different parameters affect the dynamics of the fractional order model. In the ), graphs showing approximations of solutions are plotted against various fractional orders and parameters values given in . As fractional values decrease, a(t), b(t), c(t), x(t), y(t), and y(t) decrease, as seen in Figures (). The behaviour in all figures changes as the fractional values are decreased, indicating that the solution will be more successful if the fractional values are lower than the classical derivative. We also look into how attention, repetition, and rehearsal affect the learning process in these numerical simulations. We look into a scenario in which a person is given information under the following circumstances: Case 1: f = 0.25, p = 0.3; case 2:
f = 0.3, p = 0.25 using the bottom-up processing model shown in Figures (). We can see that only in case 2 at dimension 0.5 does the amount of information stored in each memory compartment increase over time. This indicates that to store knowledge in memory compartments, attention and rehearsal are important. Similarly, we investigate the following conditions: case 1:
f = 0.25, p = 0.75 case 2:
f = 0.75, p = 0.25 using a top-down processing model as in Figures (). Chaotic behaviour for case 1 and case 2 of the bottom-up processing model, as shown in Figures (), and top-down processing chaotic behaviour, as shown in Figures (). Here, case 1 and case 2 are examined for a person with prior knowledge, and the two results are persistent. It has also been noted that, under the same conditions of rehearsal and repetition, the information obtained by a person with new knowledge outweighs that obtained by a person with prior knowledge due to the automatic effect, which is more clear with different fractional order values. In this instance, we also want to know how rehearsal and repetition work together to create an inverted variation for a novice. The ideal combination can be seen in the graph with fractional order values and integer value α = 1 in Figures. We can observe that the solution at non integer value are more propriety and reliable which are bounded to the steady state point. This comparative study also show that benefits and memory affect of fractional operator as ordinary derivative. This indicates that repetition effectively refreshes or retains information in the short-term memory, allowing for the transfer of that information to the long-term memory.
Figure 1. Simulation of the Brain Information dynamics model with different fractional order values at dimension 1 for case 1.

Figure 2. Simulation of the Brain Information dynamics model with different fractional order values at dimension 0.5 for case 1.

Figure 3. Simulation of the Brain Information dynamics model with different fractional order values at dimension 1 for case 2.

Figure 4. Simulation of the Brain Information dynamics model with different fractional order values at dimension 0.5 for case 2.

Figure 5. Simulation of the Brain Information dynamics model with different fractional order values at dimension 1 for case 1.

Figure 6. Simulation of the Brain Information dynamics model with different fractional order values at dimension 0.5 for case 1.

7. Conclusion
In this study, we created two fractional fractal generalised form deterministic mathematical models.
The first was born out of bottom-up, data-driven processing, whereas the second was born out of top-down, experience-driven processing.
In order to provide non-negative solutions, we have expanded the model using fractal fractional-order derivative operators. Additionally, we have established equilibrium points.
Furthermore, we have determined the prerequisites for the existence of a special fractional system.
The examination of the Lyapunov function for global stability is supported by the findings of the first and second derivative tests.
Numerical simulations are used to analyse the effects of the fractional operator, and two-step Lagrange polynomial solutions are used to do so. The study displays the outcomes of various scenarios where the behaviour of the model is examined for a few fractional values. As it reproduces the behaviour of the classical model for fractional values, we have come to the conclusion that the proposed system is more general than the classical model.
We examined how much attention, repetition, and rehearsal are needed for the dynamics of information processing in the human brain. To clearly assess the effectiveness of the parameters under evaluation, other factors were kept constant in each set of tests. Numerical modelling using fictitious data was used to further study the effects of attention, rehearsal, and repetition on the dynamics of information processing in the human brain. In the learning process, we discovered that information deserves adequate attention. It’s also critical to constantly practice to keep the mind informed. Additionally, repetition is advised as a powerful method of memory retention.
MANUSCRIPT.tex
Download Latex File (68.9 KB)Disclosure statement
No potential conflict of interest was reported by the author(s).
Figure 9. Chaotic modeling of Brain Information dynamics model for case 1 bounded to feasible region.

Figure 10. Chaotic modeling of Brain Information dynamics model for case 2 bounded to feasible region.

Figure 11. Chaotic modeling of Brain Information dynamics model for case 1 bounded to feasible region.

Figure 12. Chaotic modeling of Brain Information dynamics model for case 2 bounded to feasible region.

Table 1. Parameter values.
Additional information
Funding
References
- Abbot, B. (2002). Human memory (pp. 1–20). Indiana University-Purdue University at Fort Wayne, Psychology Department. Indiana.
- Akgül, E. K. (2019). Solutions of the linear and nonlinear differential equations within the generalized fractional derivatives. Chaos (Woodbury, N.Y.), 29(2), 023108. https://doi.org/10.1063/1.5084035
- Atangana, A. (2020). Modelling the spread of COVID-19 with new fractal-fractional operators: can the lockdown save mankind before vaccination? Chaos, Solitons, and Fractals, 136, 109860. https://doi.org/10.1016/j.chaos.2020.109860
- Atangana, A., & Akgül, A. (2020). Can transfer function and Bode diagram be obtained from Sumudu transform. Alexandria Engineering Journal, 59(4), 1971–1984. https://doi.org/10.1016/j.aej.2019.12.028
- Baleanu, D., Tenreiro Machado, J., & Luo, A. (2012). Fractional dynamics and control. New York, NY: Springer.
- Coutin, L., Guglielm, J., & Marie, N. (2018). On a fractional stochastic hodgkin-huxley model. Int. J. Biomath, 11, 1850061. https://doi.org/10.1142/S1793524518500614
- González-Ramírez, L. R. (2022). Fractional-order traveling wave approximations for a fractional-order neural field model. Frontiers in Computational Neuroscience, 16, 788924. https://doi.org/10.3389/fncom.2022.788924
- Herculano-Houzel, S. (2009). The human brain in numbers: A linearly scaled-up primate brain. Frontiers in Human Neuroscience, 3, 31. https://doi.org/10.3389/neuro.09.031.2009
- Huitt, W. (2000). The information processing approach. Educational Psychology Interactive (pp. 4–12). Valdosta, GA: Valdosta State University. http://chiron.valdosta.edu/whuitt/col/cogsys/infoproc.html.
- Jeffrey, D. S., Martin, P., & Geoffrey, F. W. (2007). Commented on top-down verses bottom up control of attention in the prefrontal and posterior parietal cortices. PubMed 44.
- Justin, M., Boudoue Hubert, M., Betchewe, G., Yamigno Doka, S., & Timoleon Crepin, K. (2020). Chaos in human brain phase transition. In Research advances in chaos theory. IntechOpen.
- Kargarnovin, S., Hernandez, C., Farahani, F. V., & Karwowski, W. (2023). Evidence of chaos in electroencephalogram signatures of human performance: A systematic review. Brain Sciences, 13(5), 813. https://doi.org/10.3390/brainsci13050813
- Khan, N., Ali, A., Ullah, A., & Khan, Z. A. (2023). Mathematical analysis of neurological disorder under fractional order derivative. AIMS Mathematics, 8(8), 18846–18865. https://doi.org/10.3934/math.2023959
- McKenna, T. M., McMullen, T. A., & Shlesinger, M. F. (1994). The brain as a dynamic physical system. Neuroscience, 60(3), 587–605. https://doi.org/10.1016/0306-4522(94)90489-8
- Namazi, H., & Kulish, V. V. (2015). Fractional diffusion based modelling and prediction of human brain response to external stimuli. Computational and Mathematical Methods in Medicine, 2015, 148534. https://doi.org/10.1155/2015/148534
- Natarajan, K., Acharya, U. R., Alias, F., Tiboleng, T., & Puthusserypady, S. K. (2004). Nonlinear analysis of EEG signals at different mental states. Biomedical Engineering Online, 3(1), 7. https://doi.org/10.1186/1475-925X-3-7
- Oestreicher, C. (2007). A history of chaos theory. Dialogues in Clinical Neuroscience, 9(3), 279–289. https://doi.org/10.31887/DCNS.2007.9.3/coestreicher
- Pritchard, W. S., & Duke, D. W. (1995). Measuring chaos in the BrainA tutorial review of EEG dimension estimation. Brain and Cognition, 27(3), 353–397. https://doi.org/10.1006/brcg.1995.1027
- Rahman, M., Althobaiti, A., Riaz, M. B., & Al-Duais, F. S. (2022). A theoretical and numerical study on fractional order biological models with caputo fabrizio derivative. Fractal and Fractional, 6(8), 446. https://doi.org/10.3390/fractalfract6080446
- Samuel, S., Richard, T., Tyavbee, A. J., & Terhemen, A. (2015). A mathematical model to study the human brain information processing dynamics. American Journal of Applied Mathematics, 3(5), 233–242. https://doi.org/10.11648/j.ajam.20150305.15
- Stam, C. J. (2005). Nonlinear dynamical analysis of EEG and MEG: Review of an emerging field. Clinical Neurophysiology: official Journal of the International Federation of Clinical Neurophysiology, 116(10), 2266–2301. https://doi.org/10.1016/j.clinph.2005.06.011
- Toufik, M., & Atangana, A. (2017). New numerical approximation of fractional derivative with non-local and non-singular kernel: application to chaotic models. The European Physical Journal plus, 132, 1–16.
- Wei, Z., Wang, X. J., & Wang, H. D. (2012). From distributed resources to limited slots in multiple-item working memory: A spiking network model with normalization. The Journal of Neuroscience: The Official Journal of the Society for Neuroscience, 32(33), 11228–11240. https://doi.org/10.1523/JNEUROSCI.0735-12.2012
- Weinberg, S. (2015). Membrane capacitive memory alters spiking in neurons described by the fractional-order hodgkin-huxley model. PloS One, 10(5), e0126629. https://doi.org/10.1371/journal.pone.0126629
- Xu, C. J., Cui, X. H., Li, P. L., Yan, J. L., & Yao, L. Y. (2023a). Exploration on dynamics in a discrete predator-prey competitive model involving time delays and feedback controls. Journal of Biological Dynamics, 17(1), 2220349. https://doi.org/10.1080/17513758.2023.2220349
- Xu, C., Mu, D., Liu, Z., Pang, Y., Liao, M., & Li, P. (2023b). Bifurcation dynamics and control mechanism of a fractional-order delayed Brusselator chemical reaction model. MATCH Communications in Mathematical and in Computer Chemistry, 89(1), 73–106. https://doi.org/10.46793/match.89-1.073X
- Xu, C., Mu, D., Pan, Y., Aouiti, C., & Yao, L. (2023c). Exploring bifurcation in a fractional-order predator-prey system with mixed delays. Journal of Applied Analysis and Computation, 13(3), 1119–1136. https://doi.org/10.11948/20210313
- Zekveld, A. A., Heslenfeld, D. J., Festen, J. M., & Schoonhoven, R. (2006). The top-down and bottom-up processes in speech comprehension. NeuroImage, 32(4), 1826–1836. https://doi.org/10.1016/j.neuroimage.2006.04.199
- Zhao, X., & Robinson, P. (2015). Generalized seizures in a neural field model with bursting dynamics. Journal of Computational Neuroscience, 39(2), 197–216. https://doi.org/10.1007/s10827-015-0571-7