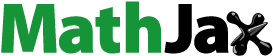
Abstract
The line transect survey method is commonly used to estimate the population size. However, recent developments in this field have tended to prefer practical mathematical models for this purpose. In this study, a new model called the weighted Weibull detection model was introduced to specifically address certain criteria related to line transect data. This study thoroughly explored the characteristics of this new model, including its shapes, moments, and probability density functions. The maximum likelihood estimation and Bayesian estimation methods were employed to ensure accurate parameter estimation. To gauge the performance of this model, population size estimates were generated and compared with various existing parametric estimation methods that are commonly used in the field. Through simulations, the resulting estimates were assessed and compared to widely adopted approaches for estimating population size. Additionally, this mathematical model was applied to real-world data involving perpendicular distances, allowing for a direct comparison of its performance against both traditional and contemporary methods using various measures of goodness of fit. Moreover, the study calculates statistical metrics, such as the variance–covariance matrix, parameter confidence intervals, and estimated population size, were obtained using the proposed detection model. These metrics provide valuable insights into the precision and uncertainty associated with estimated parameters and population size estimates. Confidence intervals offer a range of plausible parameter values, whereas the variance–covariance matrix quantifies the relationships and uncertainties between the estimated parameters.
Reviewing Editor:
1. Introduction
In place of plot or strip sample techniques, the line transect approach has been employed over the past 50 years to determine population density or abundance. It can take a lot of time to establish a plot and then count every object of interest in it. It can also be difficult to define plots in some habitats, such as those with fast-moving species or at sea. In ecology and wildlife biology, the line transect method is widely used for determining the density or abundance of a specific species in a given area. Using this method, a straight line or transect is drawn through the research area, and the number of individuals or signs of the target species that are seen along that line are counted and recorded in a methodical manner. For instance, see Burnham et al. (Citation1980). A retrospective study of this topic is proposed below, with the main references provided.
The critical component for estimating population abundance is the detection function, which can be evaluated using nonparametric, semiparametric, or parametric methods.
Gates et al. (Citation1968) introduced a function characterized by an exponential distribution and a single scaling parameter, while Hemingway (Citation1971) developed a function based on the half-normal distribution with a shoulder function as its foundation.
Parameter
representing the probability density function (PDF) at distance 0, it also plays a central role in these functions. Consequently, under the shoulder condition, the population abundance, often denoted as D in the literature, was calculated using the various approaches described in the following sections.
We will cover the parametric estimation methods which are proposed by Burnham and Anderson (Citation1976), Pollock (Citation1978), Ramsey (Citation1979), Karunamuni and Quinn (Citation1995), Buckland (Citation1985), Eberhardt (Citation1978), Eidous (Citation2004), Ababneh and Eidous (Citation2012), Quinn and Gallucci (Citation1980), Eidous and Al-Eibood (Citation2018), Ameeq et al. (Citation2023), Muneeb Hassan et al. (Citation2023), Naz et al. (Citation2023), and Hassan et al. (Citation2023). Al-Hussaini (Citation1991), Fewster et al. (Citation2008), Laake et al. (Citation2008), Porteus et al. (Citation2011), Rodriguez (Citation1977), Schmidt et al. (Citation2012), and Naz et al. (Citation2023).
Buckland and Turnock (Citation1992) employed primary and secondary observation platforms in a whale survey to challenge the assumption that every whale can be perfectly detected. Mark–recapture distance sampling (MRDS) was developed by Amstrup et al. (Citation2001) represent to estimate the population of polar bears (Ursus maritimus) in northern Alaska. Their methodology also considers population estimation variables such as group size.
Quang and Becker (Citation1997) introduced an MRDS model using a stratified Lincoln–Petersen estimate and data obtained from a line transect. Becker and Quang (Citation2009) opted for an iteratively reweighted least-squares approach instead of maximum likelihood when fitting the model, and they incorporated a logistic detection technique that considered contour transect factors. They also utilized the Horvitz–Thompson estimate (Horvitz & Thompson, Citation1952) to determine the size of the brown bear population, sometimes referred to as grizzly bears.
To estimate the population of harbor porpoises, Borchers et al. (Citation1998) developed an MRDS model with various variables and relied on the Horvitz–Thompson estimate. In their data collection efforts related to harbor porpoises, Amstrup et al. (Citation2001), Becker and Quang (Citation2009), and Borchers et al. (Citation1998) utilized survey designs that involved two observers on a single aerial survey platform.
In order to estimate the unidentified population abundance, represented by D, an observer walks a distance L that is divided into K randomly spaced transects that cover the target region of interest. During the survey, the observer records the number of objects encountered and measures the perpendicular distance x from the centerline to each object’s location. Let’s assume there are n identified objects, and their distances from each other are denoted as These distances are used to estimate the population density. The detection function
quantifies the conditional probability of observing an object at a specific perpendicular distance x from the transect line. It provides information about the likelihood of detecting an object at different distances. The detection function
represents the probability of detecting an object given its perpendicular distance x from the line transect. It can be mathematically defined as:
In order to assess the population abundance D of unidentified items in a research area, an observer walks a distance L divided into K randomly distributed transects using the line transect sampling method. The observer records the perpendicular distance x from the centerline to each object found, and the detection function represents the probability of detecting an object at distance x from the line transect. If an object is found at a perpendicular distance x, there exists a PDF
that has the same shape as
but scaled. For
this PDF can be written as
where
is the scaling constant. Since not all objects in a given region are detected using the line transect technique, detection probabilities can be estimated. The likelihood of identifying an object increases with proximity to the center of the transect line. Mathematically, if
and
are observed perpendicular distances such that
then
Considering that the probability of spotting an object at zero distance is one (
), the population density can be estimated using
where n is the number of identified objects and
is an approximate sample estimate of
The equation
can be used to determine the projected population size N in the target area of area A. Indicating that detection is still certain or virtually definite at a close proximity to the line transect’s center, the detection function
is anticipated to come from a shoulder (
).
Various references provide in-depth discussions on line transect sampling, including Marques et al. (Citation2001), Barabesi (Citation2000), Eidous (Citation2015), Eidous and Al-Salman (Citation2016), Jang and Loh (Citation2010), Seber (Citation1982), Pollock (Citation1978), Strindberg and Buckland (Citation2004), Eberhardt (Citation1978), Quang and Becker (Citation1997), Drummer and McDonald (Citation1987), Burnham and Anderson (Citation1984), Routledge and Fyfe (Citation1992), Southwell et al. (Citation2008), Brockelman and Srikosamatara (Citation1993), Chen (Citation1996), Melville and Welsh (Citation2001), Anderson et al. (Citation2001), and Naz, Al-Essa, et al. (Citation2023). Line transect sampling can underestimate the true PDF for known-sized populations, as observed in kangaroo populations by Southwell (Citation1994). This underestimation may occur when animals on the centerline become disturbed and move before being observed, violating a fundamental assumption. Mark–recapture experiments and line transect estimation can be combined to directly test the detectability.
The article is organized as follows. The proposed detection function is discussed in Section 2, which also shows that it complies with all requirements for a shoulder function. This section also includes plots of various detection functions for different parametric values. The characteristics of the density function related to the suggested detection function are presented in Section 3, and the rth moments, which can be used to determine the mean and variance, are specifically explored. In Section 4 of the referenced article, the population abundance and PDF at a distance of 0 are calculated using the maximum likelihood method. The simulation is used in Section 5 to evaluate the performance of the calculated parameters. The simulation was performed using Mathematica 10, and plots and graphs were used to provide visual insight. Section 6 describes the application of the proposed function to practical perpendicular distance datasets. The authors demonstrated how the model performs by computing the related measurements for these datasets. Finally, Section 7 concludes the article. This study combines mathematical analysis, simulation, and practical data application to present and evaluate a new detection function based on the Weibull distribution.
2. The suggested detection method
The one-parameter model exhibits a lack of flexibility, which may result in a rigid shape that does not adequately capture the detection curve. Consequently, the model estimator lacked robustness. However, we propose a weighted Weibull model (WWM) with two parameters and a user-supplied detection function to solve this problem. As a result, the adaptability of WWM was higher than that of the one-parameter model. Therefore, the robustness of the estimator and the ability of the model to conform to the shape of the detection curve can be improved by adding more parameters. Additionally, customization is made possible by the user-supplied detection function, which enables the model to consider particular aspects of the detection process. By combining the Weibull distribution and the detection function, WWM provides a more accurate representation of the detection curve. The Weibull distribution is a commonly used model for survival data, and we modified it by multiplying it by a detection function. This integration ensures that the detection curve aligns more closely with the observed data, resulting in a more reliable estimation.
By utilizing the line transect method, estimate the population density D. Any reliable detection function
should satisfy three main assumptions (Burnham et al. Citation1980). Those assumptions are justified for the proposed
as follows:
that is the objects with perpendicular distance 0 will never be missed.
where
is the first derivative of
at
i.e. the shoulder function of the detection function ought to be close to the origin (Burnham et al., Citation1980). Specifically, this means that within a relatively small distance of the line transect, the detection probability should continue to be nearly certain.
for all
this shows that while the detection function monotonically decreases in x, the likelihood of detecting an object decreases as its perpendicular distance x increases.
The likelihood of detecting an object given its perpendicular distance on the line transect is one, hence this detection function states that the probability of detection on the line transect center is assured. The detection function’s first derivative with respect to x is,
which gives results
for
and the
is uniformly monotonically declining
Various shapes confirm this shoulder condition once more (see ). The PDF related to
is possible by averaging the detecting function as
where
Then the PDF is
(2.1)
(2.1)
In , plots of the PDF are given for various parametric values.
The shoulder condition assumptions are satisfied since shows similar behavior to
but scaled to be a PDF, and it is monotonically decreasing in x. Also,
has the same shape as
with different scales.
Since for
the parameter
is
(2.2)
(2.2)
Then, from EquationEquation (2.2)(2.2)
(2.2) ,
replacing this value with
in EquationEquation (2.1)
(2.1)
(2.1) , Then, the PDF of the WWM can be stated as
such that
(2.3)
(2.3)
(2.4)
(2.4)
This is determined by the two parameters and
The estimation of the parameter’s maximum likelihood will be based on this formula.
With regard to x, the first derivative of the PDF (2.1) is
It is clear from statement that
is immediately connected to
As a result,
and
share some characteristics, including
and the monotone decreasing shape property. and exhibit these features. The proposed model would then provide a reliable estimate for
known as the Shape Criterion (see Burnham et al., Citation1980). The detection function,
which adjusts
and has parameters
for scale and
for shape, can have a wide range of shapes, as can be seen when carefully examining these pictures. A desirable property for the detection model under consideration is that all plots steadily fade to 0 as
An integrated estimator of
corresponds to
and
if they are the estimators of the parameters
and
respectively.
(2.5)
(2.5)
where the estimate for population density (abundance)
is
To calculate the values of the parameters and
we shall employ the maximum likelihood method. Thus, we calculate
and D represents the population abundance. Section 4 will go into further detail.
3. A few statistical characteristics
In this section, we seek immediate outcomes that will aid in the calculation of parameter and, ultimately, population abundance. These instant results offer insightful statistical data regarding the distribution of random variables. These moments can be used to estimate the population abundance parameter,
By calculating and analyzing various moments, such as the mean, variance, and higher-order moments, we gain insight into the central tendency, dispersion, and shape of the distribution. These moment results aid in understanding the underlying characteristics of the population and enable estimation and inference about the parameter of interest. The specific calculations of moments for the PDF given in EquationEquation (2.1)
(2.1)
(2.1) would require the functional form of
Using this information, the integrals can be solved to obtain the moment results for a given random variable.:
where E denotes the expectation operator. After some algebra, we have
(3.1)
(3.1)
By using and
to determine the mean and variance, it can be calculated that
(3.2)
(3.2)
(3.3)
(3.3)
and
Based on EquationEquation (3.1)(3.1)
(3.1) , the skewness and kurtosis of X are calculated using the following formulas.
Ghitany et al. (Citation2013) and Wackerly et al. (Citation2014) conducted studies investigating the relationship between the model parameters and
and their impact on the statistical properties of the random variable X. In , various parameter options are presented alongside the corresponding mean, variance, skewness, and kurtosis values for X. It is observed that as the parameter values increase, these statistical measures decrease. The skewness and kurtosis values, however, remain constant for fixed
values. The 3D plots shown in and illustrate the relationships between the mean, variance, skewness, kurtosis, and the values of
and
within the model. These plots visually depict how changes in
and
significantly affect the skewness and kurtosis characteristics of the parameter X. The results indicate that adjusting the values of
and
allows for control over and a better understanding of the skewness and kurtosis behaviors of the parameter X, in addition to its mean and variance.
Table 1. For some parameter values, mean, variance, skewness, and kurtosis are present.
4. Inference
In this section, we focus on making statistical inferences about the parameter when the values of
and
are unknown. To achieve this, we employed maximum likelihood and Bayesian estimation (BE) methods, which involve determining the values of
and
that maximize the likelihood function. Consider the PDF
connected to the suggested detection function. The observed values of a random sample are given by equation
4.1. Maximum likelihood estimator of 

To simulate an n-dimensional random sample of perpendicular distances, denoted as from a random variable
defined by EquationEquation (2.5)
(2.5)
(2.5) , we can generate random numbers using the specified PDF with parameter values
and
The maximum likelihood estimators (MLEs)
and
for the parameters
and
respectively, may be estimated once we have the observed sample. The approach outlined in Burnham et al. (Citation1980) can then be used to compute the MLE of
(4.1)
(4.1)
(4.2)
(4.2)
Differentiating with respect to and
respectively, we get
and
where
and
The MLEs and
of
and
respectively, are the nonlinear equation’s solutions
and
They can be calculated mathematically with the use of any statistical program, such as Mathematica, Python, R, etc. The MLE of
is then obtained by substituting the values of
and
in EquationEquation (2.5)
(2.5)
(2.5) , which is where we get the population abundance estimator D. We consider the observed Fisher information matrix for calculating confidence intervals for the model parameters
about
given by
where
and
So, V, which is the inverse of I, is the variance–covariance matrix connected to
On the other hand, it is simple to derive the estimate of
from EquationEquation (2.5)
(2.5)
(2.5) . Furthermore, based on
given by EquationEquation (2.1)
(2.1)
(2.1) , we may calculate the MLEs numerically and then the confidence intervals for
and
Based on the above discussion, It is simple to calculate the approximate large sample confidence interval for
and
as
where
and
are the MLEs of the variances of the estimators behind
and
respectively.
4.2. Bayesian estimator of 

This section deals with the BE of the unknown parameters and
respectively. For the Bayesian parameter estimation, loss functions, including squared error loss (SEL) functions, can be taken into consideration by Tolba et al. (Citation2023), Alsadat et al. (Citation2023), Bhat et al. (Citation2023), and Chinedu et al. (Citation2023). We can consider applying independent gamma priors for the variables
and
with PDF
in the parameter prior detection function.
(4.3)
(4.3)
where the hyper-parameters
are selected to reflect the prior knowledge about the unknown parameters. The joint prior for
is given by
(4.4)
(4.4)
The corresponding posterior density, given the observed data is given by
(4.5)
(4.5)
Consequently, the posterior density function is denoted by
(4.6)
(4.6)
Given any function, such as under the SEL function, the Bayes estimator is given by
(4.7)
(4.7)
They can be calculated mathematically with the use of any statistical program, such as Mathematica, Python, R, etc. The Bayesian estimator of is then obtained by substituting the values of
and
in EquationEquation (2.5)
(2.5)
(2.5) , which is where we get the population abundance estimator D.
5. Simulation research and findings
To assess how well the WWM estimate, performs in comparison with other available estimates for the function
we perform a simulation exercise. We consider the estimates from the negative exponential model (NEM), half-normal model (HNM), and weighted exponential model (WEM) (see Saeed, Citation2013). We evaluated two different target detection functions, coupled with observations of
with the sample sizes of
and
to duplicate the perpendicular distances. These identification strategies were selected to highlight the range of shapes that might be present in a specific field (see Eidous, Citation2015). The preferred models for replicating the perpendicular distances are as follows:
Detection of exponential power (EP) (see Pollock, Citation1978):
(5.1)
(5.1)
where
is the standard gamma function defined as
The beta exponential (BE) detection function is described by Eberhardt (Citation1978):
(5.2)
(5.2)
Each model is shortened at specific distances w in order to simulate the data. In contrast to the BE detection function, which is truncated at and
the EP detection function is truncated at
and 2. A number of arbitrary
values are selected for both models. We consider sample sizes of
and generate 1500 samples of perpendicular distances for each model randomly. and present the relative bias (RB) and the relative mean square error (RME) for the different estimators. Based on these results, we draw the following conclusions:
Table 2. When data from the EP model are simulated, RB and RME are used for the various estimations.
Table 3. When data are simulating from the BE model, RB and RME for the various estimations.
Table 4. Information regarding wooden stakes parallel distances in meters.
As the sample size n increases, the RB and RME of all estimators decrease. This suggests that the estimators are reliable as the sample size grows.
The suggested estimate
exhibits lesser RB and RME compared to the NEM, HNM, and WEM estimators for all studied target detection functions, which shows that the proposed estimator outperforms the competing models, as shown in and .
depict the RME for
and
respectively.
6. Model compatibility and real-world data application
The primary subjects of discussion in this section are model selection and validation. However, choosing these models is the most difficult step in creating a good model. It was chosen after carefully weighing all the available information. The chosen model must be flexible enough to consider the trade-off between model complexity and ease of assessment while accurately reproducing the provided data. Additionally, it takes a lot of effort to simulate behaviors for extreme values of the relevant variable. In this situation, a variety of visualizations and goodness-of-fit checks are required as part of the statistical process step for validating the model.
6.1. Alternative models
Using some goodness-of-fit analyses, the performance of the proposed detection model is compared to a variety of other current detection models. For comparison, the following detection models are utilized:
‘New two-parameter detection model (NDM)’ (Bakouch et al., Citation2022):
‘Model (2013)’ (Saeed, Citation2013):
‘Generalized exponential model (GEM)’ (Quinn & Gallucci, Citation1980):
‘Exponential power series model (EPSM)’ (Pollock, Citation1978):
‘Reverse logistic model (RLM)’ (Eberhardt, Citation1978):
‘Exponential quadratic model (EQM)’ (Burnham et al., Citation1980):
‘Weighted half-normal model (WHNM)’ (Eidous & Al-Salman, Citation2016):
‘Model (2015)’ (Eidous, Citation2015):
‘Weighted exponential model (WEM)’ (Ababneh & Eidous, Citation2012):
‘Negative exponential model (NEM)’ (Gates et al., Citation1968):
To compare the MLEs with the data being discussed, we consider the pertinent PDF of each detection model. The effectiveness of the detection model is strongly correlated with its PDF, as shown previously.
6.2. Perpendicular distance datasets
Dataset 1: Information about wooden stakes parallel distances in meters
The dataset comprises perpendicular distances between a sagebrush meadow and wooden stakes. These distances were obtained by walking a 1000 m path and selecting a sample of 67 stakes from a total population of 150 stakes. The true density of the stakes is defined as stakes/meter. The recorded distances, denoted as
represent the perpendicular distances associated with the selected stakes along the single 1000 m path. The estimated value of
given the sample size n and stake density D, is determined as 0.11029 (refer to Karunamuni & Quinn, Citation1995; Zhang, Citation2001 for more details). In addition, the zone of interest’s dimensions, notably the sagebrush meadow east, are calculated as
using the equation
where
(see Karunamuni & Quinn, Citation1995; Zhang, Citation2001). Burnham et al. (Citation1980) and Barabesi (Citation2000) have both reviewed and reported on the data in great detail. The perpendicular distance value of 31.31 in the accompanying table significantly deviates from the transect path in contrast to other values, as is evident when looking at the wooden stake dataset. The accuracy of the estimation will therefore be increased by removing this outlier (as suggested by Zhang, Citation2001). As a result, the truncation point is removed.
presents the important statistics of the wooden stakes dataset, including the relevant theoretical metrics of the WWM (Wooden Stakes Analysis). Additionally, the accompanying box plot, shown in , provides further visual assistance. It is worth noting that the box plots display the minimum score of a dataset, first quartile (lower quartile), median, third quartile (upper quartile), and maximum score as part of the five-number summary.
Table 5. The wooden stakes dataset’s descriptive statistics and related theoretical WWM metrics.
Table 6. The Hemmingway’s data perpendicular distance data in meters.
Dataset 2: The Hemingway’s perpendicular distance data
The second dataset in comes from Hemmingway’s research on several ungulates in Africa (see Burnham et al., Citation1980). Seventy-three animals were found along a single-line transect that traveled for 60,000 m. Using the sighting method, the perpendicular distances are calculated. Sighted angles and distances. Hemmingway’s dataset does not include the true density D or the area A of the discovered items (not recorded during the survey). However, the TRANSECT software, which adapts the estimate of and D, which are, respectively, 0.0065 and 0.0396 animal per hectare (or 3.096106 animal per meter), creates the estimation technique. presents the important statistics of Hemmingway’s dataset, including the relevant theoretical metrics of the WWM. Additionally, the accompanying box plot is shown in .
Table 7. Hemmingway’s dataset descriptive statistics and related theoretical WWM metrics.
The following common criteria are applied in order to compare the fit performance of the models that were taken into consideration for the study of Datasets 1 and 2: the Kolmogorov–Smirnov test p value, the Bayesian information criterion (BIC), the Hannan–Quinn information criterion (HQIC), the Akaike information criterion (AIC), and the corrected AIC (CAIC). Their values are shown in . It is evident that our model has the lowest information criterion values and the greatest p value for the Kolmogorov–Smirnov test. We can therefore conclude that the suggested model works well in this situation.
Table 8. The MLEs, W*, A*, statistics K–S and p value for wooden stakes dataset.
Table 9. Information criteria and log-likelihood for wooden stakes dataset.
Table 10. The MLEs, W*, A*, statistics K–S and p value for Hemmingway’s dataset.
Table 11. Information criteria and log-likelihood for Hemmingway’s dataset.
The CIs of the PDF at 0 based on the MLEs are obtained in and (with appropriate truncation for the bottom bound of the intervals) based on Datasets 1 and 2 and the proposed model.
Table 12. For a wooden stake, the confidence intervals.
Table 13. For Hemmingway’s dataset, the confidence intervals.
The MLEs of all the models were used to produce the estimated population abundance, denoted as and the maximum likelihood estimate of the PDF at 0,
for the analyzed data. The theoretical standard deviation of the model is represented by SD, but the standard deviation of the sample is represented by
and display the findings for
and
According to the tables, the WWM exhibits the lowest absolute difference,
indicating a closer match between the estimated and theoretical standard deviations. Furthermore, among the examined detection models, the WWM yields
and
values that closely resemble the genuine values of
and D for the considered data.
Table 14. Estimated population abundance D and for the wooden stakes dataset using the
Table 15. Estimated population abundance D and for the Hemmingway’s dataset using the
7. Concluding remarks
This study introduced and examined a novel two-parameter detection model. The proposed model is beneficial for line transect data. This model ensures that it meets the shoulder requirement and that its perpendicular distance monotonically decreases. The proposed WWM is adaptable to suit line transect data because it depends only on two parameters. However, statistical techniques to estimate these parameters, such as MLEs, do not exist in closed form. Consequently, in this context, a numerical method, such as the Newton–Raphson method, is required. According to the simulation results from this study, the estimators obtained under the suggested model are promising for estimating population abundance using line transect approaches. Favorable properties of the proposed estimator, which were obtained using the suggested model, are shown in the simulation results. The performance of our model in comparison with other models shows how the fit can be improved using a valuable collection, such as a table of perpendicular distances and some information on goodness of fit. In addition, investigating the inclusion of a scale parameter in the detection function is a potential extension; however, more work needs to be done on the definition of the normalization constant and the suggested approach needs to be modified.
su2.eps
Download EPS Image (5.7 KB)picttt.eps
Download EPS Image (17.7 KB)pdf1.eps
Download EPS Image (156.2 KB)ttt1.eps
Download EPS Image (5.2 KB)ep1.eps
Download EPS Image (5.4 KB)model1.eps
Download EPS Image (165.7 KB)pdf2.eps
Download EPS Image (205.3 KB)pichisto2d.eps
Download EPS Image (230.3 KB)pichisto1.eps
Download EPS Image (252.9 KB)box2.eps
Download EPS Image (5.3 KB)pichisto1a.eps
Download EPS Image (242.2 KB)BurrDnew.dvi
Download (44.9 KB)ep4.eps
Download EPS Image (5.4 KB)sum1.eps
Download EPS Image (5.7 KB)sum2.eps
Download EPS Image (5.7 KB)su1.eps
Download EPS Image (5.7 KB)histogram 1.eps
Download EPS Image (240.6 KB)model2.eps
Download EPS Image (274.5 KB)be4.eps
Download EPS Image (5.4 KB)boxplot1.eps
Download EPS Image (3.6 KB)be1.eps
Download EPS Image (5.5 KB)pdf_lomax.eps
Download EPS Image (13.2 KB)pichisto2.eps
Download EPS Image (230.3 KB)picbox1.eps
Download EPS Image (3.6 KB)sum3.eps
Download EPS Image (5.8 KB)BurrDnew updated.tex
Download Latex File (76 KB)pictt.eps
Download EPS Image (5 KB)be3.eps
Download EPS Image (5.5 KB)su3.eps
Download EPS Image (5.7 KB)box1.eps
Download EPS Image (3.6 KB)ttt2.eps
Download EPS Image (3.6 KB)ep2.eps
Download EPS Image (5.4 KB)picttt2.eps
Download EPS Image (22 KB)be2.eps
Download EPS Image (5.5 KB)su4.eps
Download EPS Image (5.1 KB)fhisto.eps
Download EPS Image (190.2 KB)ep3.eps
Download EPS Image (5.4 KB)picttt2a.eps
Download EPS Image (22 KB)picbox2.eps
Download EPS Image (3.5 KB)sum4.eps
Download EPS Image (5.1 KB)Disclosure statement
No potential conflict of interest was reported by the authors.
References
- Ababneh, F., & Eidous, O. M. (2012). A weighted exponential detection function model for line transect data. Journal of Modern Applied Statistical Methods, 11(1), 144–151. https://doi.org/10.22237/jmasm/1335845400
- Al-Hussaini, E. K. (1991). A characterization of the Burr type XII distribution. Applied Mathematics Letters, 4(1), 59–61. https://doi.org/10.1016/0893-9659(91)90123-D
- Alsadat, N., Elgarhy, M., Tolba, A. H., Elwehidy, A. S., Ahmad, H., & Almetwally, E. M. (2023). Classical and Bayesian estimation for the extended odd Weibull power Lomax model with applications. AIP Advances, 13(9), 095316-1–095316-20. https://doi.org/10.1063/5.0170848
- Ameeq, M., Tahir, M. H., Hassan, M. M., Jamal, F., Shafiq, S., & Mendy, J. T. (2023). A group acceptance sampling plan truncated life test for alpha power transformation inverted perks distribution based on quality control reliability. Cogent Engineering, 10(1), 2224137. https://doi.org/10.1080/23311916.2023.2224137
- Amstrup, S. C., Durner, G. M., McDonald, T. L., Mulcahy, D. M., & Garner, G. W. (2001). Comparing movement patterns of satellite-tagged male and female polar bears. Canadian Journal of Zoology, 79(12), 2147–2158. https://doi.org/10.1139/z01-174
- Anderson, D. R., Burnham, K. P., Lubow, B. C., Thomas, L. E. N., Corn, P. S., Medica, P. A., & Marlow, R. W. (2001). Field trials of line transect methods applied to estimation of desert tortoise abundance. The Journal of Wildlife Management, 65(3), 583–597. https://doi.org/10.2307/3803111
- Bakouch, H. S., Chesneau, C., & Abdullah, R. I. (2022). A pliant parametric detection model for line transect data sampling. Communications in Statistics – Theory and Methods, 51(21), 7340–7353. https://doi.org/10.1080/03610926.2021.1872640
- Barabesi, L. (2000). Local likelihood density estimation in line transect sampling. Environmetrics, 11(4), 413–422. https://doi.org/10.1002/1099-095X(200007/08)11:4<413::AID-ENV422>3.0.CO;2-P
- Becker, E. F., & Quang, P. X. (2009). A gamma-shaped detection function for line-transect surveys with mark-recapture and covariate data. Journal of Agricultural, Biological, and Environmental Statistics, 14(2), 207–223. https://doi.org/10.1198/jabes.2009.0013
- Bhat, A. A., Ahmad, S. P., Almetwally, E. M., Yehia, N., Alsadat, N., & Tolba, A. H. (2023). The odd Lindley power Rayleigh distribution: Properties, classical and Bayesian estimation with applications. Scientific African, 20, e01736. https://doi.org/10.1016/j.sciaf.2023.e01736
- Borchers, D. L., Buckland, S. T., Goedhart, P. W., Clarke, E. D., & Hedley, S. L. (1998). Horvitz-Thompson estimators for double-platform line transect surveys. Biometrics, 54(4), 1221–1237. https://doi.org/10.2307/2533652
- Brockelman, W. Y., & Srikosamatara, S. (1993). Estimation of density of gibbon groups by use of loud songs. American Journal of Primatology, 29(2), 93–108. https://doi.org/10.1002/ajp.1350290203
- Buckland, S. T. (1985). Perpendicular distance models for line transect sampling. Biometrics, 41(1), 177–195.
- Buckland, S. T., & Turnock, B. J. (1992). A robust line transect method. Biometrics, 48(3), 901–909. https://doi.org/10.2307/2532356
- Burnham, K. P., & Anderson, D. R. (1976). Mathematical models for nonparametric inferences from line transect data. Biometrics, 32(2), 325–336.
- Burnham, K. P., & Anderson, D. R. (1984). The need for distance data in transect counts. The Journal of Wildlife Management, 48(4), 1248–1254. https://doi.org/10.2307/3801785
- Burnham, K. P., Anderson, D. R., & Laake, J. L. (1980). Estimation of density from line transect sampling of biological populations (Wildlife Monograph No. 72). The Wildlife Society (pp. 3–202).
- Chen, S. X. (1996). A kernel estimate for the density of a biological population by using line transect sampling. Journal of the Royal Statistical Society: Series C (Applied Statistics), 45(2), 135–150. https://doi.org/10.2307/2986150
- Chinedu, E. Q., Chukwudum, Q. C., Alsadat, N., Obulezi, O. J., Almetwally, E. M., & Tolba, A. H. (2023). New lifetime distribution with applications to single acceptance sampling plan and scenarios of increasing hazard rates. Symmetry, 15(10), 1881. https://doi.org/10.3390/sym15101881
- Drummer, T. D., & McDonald, L. L. (1987). Size bias in line transect sampling. Biometrics, 43(1), 13–21. https://doi.org/10.2307/2531944
- Eberhardt, L. L. (1978). Transect methods for population studies. The Journal of Wildlife Management, 42(1), 1–31. https://doi.org/10.2307/3800685
- Eidous, O. M. (2015). Nonparametric estimation of f(0) applying line transect data with and without the shoulder condition. Journal of Information and Optimization Sciences, 36(4), 301–315. https://doi.org/10.1080/02522667.2013.867726
- Eidous, O., & Al-Eibood, F. (2018). A bias-corrected histogram estimator for line transect sampling. Communications in Statistics – Theory and Methods, 47(15), 3675–3686. https://doi.org/10.1080/03610926.2017.1361987
- Eidous, O., & Al-Salman, S. (2016). One-term approximation for normal distribution function. Mathematics and Statistics, 4(1), 15–18. https://doi.org/10.13189/ms.2016.040102
- Eidous, U. (2004). A parametric family for density estimation in line transect sampling. Jordanian Scientific Journals, 13(2), 315–326.
- Fewster, R. M., Southwell, C., Borchers, D. L., Buckland, S. T., & Pople, A. R. (2008). The influence of animal mobility on the assumption of uniform distances in aerial line-transect surveys. Wildlife Research, 35(4), 275–288. https://doi.org/10.1071/WR07077
- Gates, C. E., Marshall, W. H., & Olson, D. P. (1968). Line transect method of estimating grouse population densities. Biometrics, 24(1), 135–145.
- Ghitany, M. E., Al-Mutairi, D. K., Balakrishnan, N., & Al-Enezi, L. J. (2013). Power Lindley distribution and associated inference. Computational Statistics & Data Analysis, 64, 20–33. https://doi.org/10.1016/j.csda.2013.02.026
- Hassan, M. M., Tahir, M. H., Ameeq, M., Jamal, F., Mendy, J. T., & Chesneau, C. (2023). Risk factors identification of COVID-19 patients with chronic obstructive pulmonary disease: A retrospective study in Punjab-Pakistan. Immunity, Inflammation and Disease, 11(8), e981. https://doi.org/10.1002/iid3.981
- Hemingway, P. (1971). Field trials of the line transect method of sampling large populations of herbivores. In E. Duffey & A. S. Watts (Eds.), The scientific management of animal and plant communities for conservation (pp. 405–411). Blackwell.
- Horvitz, D. G., & Thompson, D. J. (1952). A generalization of sampling without replacement from a finite universe. Journal of the American Statistical Association, 47(260), 663–685. https://doi.org/10.1080/01621459.1952.10483446
- Jang, W., & Loh, J. M. (2010). Density estimation for grouped data with application to line transect sampling. The Annals of Applied Statistics, 4(2), 893–915. https://doi.org/10.1214/09-AOAS307
- Karunamuni, R. J., & Quinn, T. J. (1995). Bayesian estimation of animal abundance for line transect sampling. Biometrics, 51(4), 1325–1337. https://doi.org/10.2307/2533263
- Laake, J., Dawson, M. J., & Hone, J. (2008). Visibility bias in aerial survey: Mark–recapture, line-transect or both? Wildlife Research, 35(4), 299–309. https://doi.org/10.1071/WR07034
- Marques, F. F., Buckland, S. T., Goffin, D., Dixon, C. E., Borchers, D. L., Mayle, B. A., & Peace, A. J. (2001). Estimating deer abundance from line transect surveys of dung: Sika deer in southern Scotland. Journal of Applied Ecology, 38(2), 349–363. https://doi.org/10.1046/j.1365-2664.2001.00584.x
- Melville, G. J., & Welsh, A. H. (2001). Line transect sampling in small regions. Biometrics, 57(4), 1130–1137. https://doi.org/10.1111/j.0006-341x.2001.01130.x
- Muneeb Hassan, M., Ameeq, M., Jamal, F., Tahir, M. H., & Mendy, J. T. (2023). Prevalence of COVID-19 among patients with chronic obstructive pulmonary disease and tuberculosis. Annals of Medicine, 55(1), 285–291. https://doi.org/10.1080/07853890.2022.2160491
- Naz, S., Al-Essa, L. A., Bakouch, H. S., & Chesneau, C. (2023). A transmuted modified power-generated family of distributions with practice on submodels in insurance and reliability. Symmetry, 15(7), 1458. https://doi.org/10.3390/sym15071458
- Naz, S., Tahir, M. H., Jamal, F., Ameeq, M., Shafiq, S., & Mendy, J. T. (2023). A group acceptance sampling plan based on flexible new Kumaraswamy exponential distribution: An application to quality control reliability. Cogent Engineering, 10(2), 2257945. https://doi.org/10.1080/23311916.2023.2257945
- Pollock, K. H. (1978). A family of density estimators for line-transect sampling. Biometrics, 34(3), 475–478. https://doi.org/10.2307/2530611
- Porteus, T. A., Richardson, S. M., & Reynolds, J. C. (2011). The importance of survey design in distance sampling: Field evaluation using domestic sheep. Wildlife Research, 38(3), 221–234. https://doi.org/10.1071/WR10234
- Quang, P. X., & Becker, E. F. (1997). Combining line transect and double count sampling techniques for aerial surveys. Journal of Agricultural, Biological, and Environmental Statistics, 2(2), 230–242. https://doi.org/10.2307/1400405
- Quinn, T. J., & Gallucci, V. F. (1980). Parametric models for line-transect estimators of abundance. Ecology, 61(2), 293–302. https://doi.org/10.2307/1935188
- Ramsey, F. L. (1979). Parametric models for line transect surveys. Biometrika, 66(3), 505–512. https://doi.org/10.1093/biomet/66.3.505
- Rodriguez, R. N. (1977). A guide to the Burr type XII distributions. Biometrika, 64(1), 129–134. https://doi.org/10.1093/biomet/64.1.129
- Routledge, R. D., & Fyfe, D. A. (1992). Confidence limits for line transect estimates based on shape restrictions. The Journal of Wildlife Management, 56(2), 402–407. https://doi.org/10.2307/3808843
- Saeed, G. A. A. (2013). New parametric model for grouped and ungrouped line transect data [Doctoral dissertation]. Yarmouk University.
- Schmidt, J. H., Rattenbury, K. L., Lawler, J. P., & Maccluskie, M. C. (2012). Using distance sampling and hierarchical models to improve estimates of Dall’s sheep abundance. The Journal of Wildlife Management, 76(2), 317–327. https://doi.org/10.1002/jwmg.216
- Seber, G. A. F. (1982). The estimation of animal abundance and related parameters. Acta Theriologica, 27, 376. London, Charles Griffin & Co. Ltd. 654 pp. https://doi.org/10.4098/at.arch.82-33
- Southwell, C. (1994). Evaluation of walked line transect counts for estimating macropod density. The Journal of Wildlife Management, 58(2), 348–356. https://doi.org/10.2307/3809401
- Southwell, C., Paxton, C. G., Borchers, D., Boveng, P., Rogers, T., & William, K. (2008). Uncommon or cryptic? Challenges in estimating leopard seal abundance by conventional but state-of-the-art methods. Deep Sea Research Part I: Oceanographic Research Papers, 55(4), 519–531. https://doi.org/10.1016/j.dsr.2008.01.005
- Strindberg, S., & Buckland, S. T. (2004). Zigzag survey designs in line transect sampling. Journal of Agricultural, Biological, and Environmental Statistics, 9(4), 443–461. https://doi.org/10.1198/108571104X15601
- Tolba, A. H., Muse, A. H., Fayomi, A., Baaqeel, H. M., & Almetwally, E. M. (2023). The Gull Alpha Power Lomax distributions: Properties, simulation, and applications to modeling COVID-19 mortality rates. PLoS One, 18(9), e0283308. https://doi.org/10.1371/journal.pone.0283308
- Wackerly, D., Mendenhall, W., & Scheaffer, R. L. (2014). Mathematical statistics with applications. Cengage Learning.
- Zhang, S. (2001). Generalized likelihood ratio test for the shoulder condition in line transect sampling. Communications in Statistics – Theory and Methods, 30(11), 2343–2354. https://doi.org/10.1081/STA-100107690