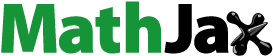
Abstract
Trichoderma Reesei is a mesophilic and filamentous fungus. It is an anamorph of the fungus Hypocrea jecorina, in addition, T. reesei can secrete large amounts of cellulolytic enzymes and form dextrose PDA (potato dextrose agar) and potato injection. After the preparation of fungi, it is added to the cracked samples. The experimental samples were 150 mm3 cubic compression and 70 mm x 30 mm x 15 mm cracks on the surface of each cube. Different fungi water extracts were used with 0, 50.5, 6.37, and 8.42 liters of water per ml. The results show that the addition of 8.42 (ml) of the mushroom extract with one liter of water has the maximum compressive strength with more than 18.99 MPa for 28 days, 16.7 for 14 days, and 14.5 for 7 days. In this study, linear regression, lasso regression, and rigid regression have been used to predict compressive strength, also the cooperation between mushroom juice per milliliter and compressive strength has been predicted. To find the accuracy, Correlation Coefficient (R2), Mean Absolute Errors (MAE), and Root Mean Square Error have been used. The results of machine learning show that the results of linear regression and rigid regression R2 were more than 0.98. In addition, the relationship compressive strength prediction results showed that R2 for fungi broth with one liter of water was 5.05 mL was more than 0.98. Finally, this study shows that the fungus Trichoderma reesei is an effective agent for curing concrete and improving the compressive strength of concrete.
1. Introduction
Self-healing concrete is generally divided into the following three categories: the use of auxiliary cementitious materials for repair by directly adding mineral additives, crystalline additives, and polymer materials to concrete. The use of carriers with healing agents for self-healing, where the healing agents are stored in the capsule wall of micro or macroscopic capsules. In case of changes in pH and other damaging factors, the capsule wall ruptures, and the internal healing agent is released to play a self-healing role. For example, by adding microorganisms and nutrients to concrete, bacteria can produce calcium carbonate to repair concrete cracks (Huang & Zhou, Citation2022).
The strategy is to create a concrete mix that encapsulates the bacteria inside microcapsules that multiply when water meets and collects in the cracks. Bacteria may grow and form calcium during this process, healing the crack before it is washed away by the water. Incorporating bacteria into concrete provides a higher level of protection against steel corrosion. These bacteria not only cooperate to propagate cracks but also use oxygen otherwise be seen in the corrosion process of steel bars. Not only, self-healing concrete can increase the range of concrete structures, but also cuts repair costs in half and helps reduce man-made carbon dioxide emissions.
Concrete, possibly cementitious composites, and superabsorbent polymers (SAP), which are almost always avoided by using poly (ethylene glycol) unless a waterproof coating (such as sand and epoxy resin) provided appear to be the main functions of the capsules. Product performance of healing and self-healing of damaged mortar, as well as capsule structure and hydrolyzed mechanisms, were all investigated. The results showed that the main components of the capsule may react with water and cause the SAP to swell in the gaps and the PEG to dissolve (Basu et al., Citation2015).
Another proposed method is formulated to use a type of selective microbial packaging operation. Researchers from around the world are also investigating self-healing cement systems based on calcium carbonate deposition associated with bacterial growth. This rather conceptual process enabled the development of bacterial concrete, a completely non-toxic concrete that inhibits bacteria to repair defects in mortar and concrete (Salem et al., Citation2022). The refinement of these four customization options was noticed through the utilization of a central composite design. With enhanced mortar endurance, the sporulation mechanism is proposed, but peripheral devices like Ca2+, Fe2+, and Mn2+, were uncovered to facilitate sporulation. A 0.3 mm mortar fracture is also mended in only seven days using a mixture of L. boronitolerans YS11 fungal powder, concrete, water, sand, yeast extract, calcium lactate, and paste. On the crack’s base, calcium carbonate condensation can also be seen (Bhosale & Vijayalakshmi, Citation2015).
Some studies used fungus as a self-healing material of concrete which can produce CaCO3. Zhao et al. (Citation2022) analyzed the effect of fungi in healing the micro and macro cracks of concrete. They concluded, however, that more research is needed on the addition of fungi to concrete to optimize the improvement of concrete, the use of fungi is effective. Khan et al. (Citation2023) studied the effect of fungi (Fusarium oxysporum and Trichoderma longibrachiatum) as potential bio-admixtures to self-healing materials of Recycled Aggregate Concrete (RAC). The composition of the crack filler was confirmed as bio-calcite through energy dispersive X-ray spectroscopy (EDX), also the compressive strength of concrete will increase by more than 11% and 30%. In the current studies, researchers understood that the effect of Trichoderma Reesei Fungus is more impressive than other types of fungus. Luo et al. (Citation2018) studied different types of fungus to find the effect and the best of these on the healing of concrete. Finally, they confirmed that the crystals deposited on the fungal hyphae of Trichoderma reesei are composed of calcite, which promotes the healing of concrete. However, a lot of research has been done on the fungal effect of Trichoderma reesei on self-healing concrete, and the effect of water content on these fungi has not been studied yet. In addition, there is no staining to find the compressive strength due to the addition of water to the mushrooms. On the other hand, there is no suitable study that combines experimental study and numerical study like machine learning.
Data mining, Artificial intelligence (AI), and Machine Learning (ML) have different applications in engineering, and technology (Zheng et al., Citation2022). Data mining is used in many types of production to solve complex problems. The most use of data mining is in the financial process for large companies. Not only financial companies use data mining, but also engineers and developers use data mining. For example, predicting the compressive strength of concrete is a good reason for data mining (Dębska, Citation2022).
Data mining, ML, and AI are used by industries, and other engineers to find new, and optimal ways to reduce production time, and materials (Agrawal, Citation2022; AlAlaween et al., Citation2022; Khorasani et al., Citation2022). Currently, many researchers tend to utilize data mining, ML, and AI to find the mechanical properties of concrete. Some research used some limited variable properties of concrete materials to find the compressive strength (Akande et al., Citation2014; Erdal, Citation2013; Farooq et al., Citation2021; Khan et al., Citation2021; Peng et al., Citation2022; Yang et al., Citation2022). For example, Shahmansouri et al. (Citation2022) presented a new method to forecast the compressive strength of natural zeolitic concrete. Their current study’s important variable was the curing period of concrete. More than 56 mixture designs were used in their study. The Root Mean Square Errors (MSE) were evaluated to find the accuracy of the prediction results. Their compressive strength prediction results show that the MSE was 1.6. The prediction results show high accuracy. While, they did not index the quality of concrete, and mixture designs through ML.
Some perdition method such as Artificial Neural Network (ANN) is established to find the compressive strength of concrete (Akande et al., Citation2014; Akter et al., Citation2018; Fl ¨ory & Pottmann, Citation2019). For example, Chopra et al. (Citation2016) predicted the compressive strength of concrete through ANNs. The ANN method was the curing period between 28 days and 91 days. The Leveberg-Marquardt (LM) training function is applied through ANN to find the compressive strength results. Their results indicated that forecasting compressive strength was accurate. The Coefficient of Determination (R2) result was more than 0.8. Using the curing periods as the main variable, is an advantage however, other parameters such as the qualities have been neglected in the ANN method. The programming is not able to understand the quality of a mixture of materials, and programmers have to add a new way to indicate the quality. Another neglect of the programming method was the lack of collecting data, the programming method is not able to collect all data about the concrete. Another example, Topcu and Saridemir (Topçu & Sarıdemir, Citation2007) used the ANN, and the Fuzzy Logic (FL) method to predict the compressive strength of concrete. In the current study, the predicted method was based on fly ash as a variable material. Their results show that R2 was more than 0.9. On the one hand, their results were accurate according to the R2 results, on the other hand, their results were influenced by the fly ash as the main variable. In another example, Kashyzadeh et al. (Citation2022) applied a data mining method to forecasting the compressive strength of concrete through the shape and size of the dry aggregate as the main variable material. They understood that the shape, and size of aggregates are affected by compressive strength. They found that the compressive strength of aggregates in the cold wind process increased by more than 8%. They used two important parameters, first the shape of aggregates which are related to the quality of materials, and then the environmental progress of the material. However, Kashyzadeh et al. (Citation2022) presented new types of data mining algorithms, their study neglected the effect of other parameters such as the curing period, the quality of cement, etc. In another example of environmental factors, Kashyzadeh et al. (Citation2022) studied more than 108 cubes of concrete with different types of aggregates, and curing temperatures to predict the compressive strength of concrete. They understood that 15 °C is the best temperature for water in the curing period. In another example, Nguyen et al. (Citation2020) collected more than 330 mixture designs to predict the compressive strength and the best method of compressive strength prediction. The variable materials were fly ash and geo-polymer. The lack of focus on the other types of material was a big gap in their prediction method.
Kumar et al. (Citation2022) applied ML techniques, Gaussian Progress Regression (GPR), Support Vector Machine Regression (SVMR), and Ensemble Learning (EL), to lightweight-concrete compressive strength forecasting. Their results show that R2 was more than 0.98 for the GPR prediction method. They used many types of programming methods to find accurate prediction compressive strength results, while, the results did not have enough variables. Sami Ullah et al. (Citation2022) established SVR and Random Forest (RF) methods to find the prediction results of lightweight foam concrete (LFC) compressive strength. Their results show that the RF method was able to predict with high accuracy. However, they did not indicate the quality of the LFC parameter to find reliable results. Hematibahar et al. (Citation2022) established a Logistic function to find the accurate compressive strength results. Their method was unrelated to the materials of concrete. However, they used a new way to predict concrete compressive strength, but their method was not able to find the optimal mixture and material of concrete mixture. In another example, Hasanzadeh et al. (Citation2022) used ML to find the mechanical properties of basalt fiber high-performance concrete. They used a new method to predict the mechanical properties through the relationship between mechanical properties, and different specimens of concrete. Their results show that the ML technique makes it possible to predict mechanical properties with high accuracy. According to their results, the compressive, tensile, and flexural strengths are predicted with R2 more than 0.9. The R2 results show that their results were reliable. The previous studies concentrated on using some variables to find the compressive strength prediction. While, the compressive strength of concrete is related to many variables such as materials, quality of materials, and environmental conditions. A complete study about the reliability of the prediction results of concrete through estimated the mixture design by data mining is neglected subject.
Some researchers have studied the prediction of self-healing concrete mechanical behavior. For example, Onyelowe et al. (Citation2022) studied the concrete with additional Carbone and bacteria to heal the cracks. They used R2 to find the accuracy the algorithm of. They found that the current algorithm has been predicting the crack healing of concrete with more than 0.93 (R2). In another example, Huang et al. (Citation2021) predicted the healing performance of concrete. They used an artificial Neural Network (ANN), k-nearest Neighbours (kNN), a Gradient Boosting Regression (GBR), Decision Tree Regression, (DTR), A Support Vector Regression (SVR), and a Random Forest (RF). Parameters of These Algorithms. Finally, they found that R2 0.93 for GBR was more accurate than other types. Zhuang and Zhou (Citation2019) predict Bacteria-Based Bactria-based self-healing concrete through Machine Learning. They used Support Vector Regression (SVR), Decision Tree Regression (DTR), Gradient Boosting Regression (GBR), Artificial Neural Network (ANN), Bayesian Ridge Regression (BRR) and Kernel Ridge Regression (KRR). According to their results, the GBR has the best R2 with more than 0.93.
The present study investigated the effect of Trichoderma Reesei Fungi as a concrete healing agent. The fungi used for Trichoderma Reesei (TR) were obtained from the Microbial Type Culture Collection and Gene Bank (MTCC). Trichoderma reesei is a mesophilic and filamentous fungus. It is an anamorph of the fungus Hypocrea jecorina, in addition, T. reesei can secrete large amounts of cellulolytic enzymes and form dextrose PDA (potato dextrose agar) and potato injection. In the current study, the effect of Trichoderma Reesei Fungus effect on the healing of the concrete has been investigated. This type of fungi assists in making more Ca2 on concrete and cementum matrix and heals small cracks in the depth and surface of the concrete. Moreover, the effect of Trichoderma Reesei Fungus in producing CacO3 in the concrete is obvious. To cover the gap in other studies, the attention of this research is to add different amounts of water to fungal broth with 0, 50.5, 6.37, and 8.42 liters of water per ml to find the effectiveness of cracking and compressive strength. Another novelty of this paper is the use of machine learning to find predictions. In this way, the machine learning method has been used to predict compressive strength through linear regression, lasso regression, and rigid regression. As another innovation of the article the relationship between fungal water in ml and compressive strength is found by the quadratic equation. Also, the compressive stress-strain curve is predicted by the logistic function with high accuracy. Finally, the results show that the Trichoderma Reesei fungus can assist in healing the cracks and increase the compressive strength of concrete.
2. Materials and methods
2.1. Materials
In this study, conventional concrete has been chosen to compare the fungi and find the effect of Trichoderma Reesei Fungus. In this way, the ordinary Portland cement OPC grade 53 (), fine aggregates 4.75 mm, coarse aggregates size between 4.75 mm to 10 mm and 20 mm and 40 mm, tap water, superplasticizer, and healing bacteria have been used as the main material of the concrete for doing compressive strength experimental tests.
Table 1. Properties of cement M500.
Ordinary Portland cement is graded based on its strengths. The grade number indicates the compressive strength (MPa) of concrete after 28 days of curing or setting. The grade used in this research is OPC 53 cement. The softener used for this study is Fosroc Auramix. The fungus used for the above study is Trichoderma Reesei. (T R) of MTCC Fine aggregate of size ratio less than 4.75 mm is obtained.
Zone II sand has been used as fine-grained aggregate for this study. Coarse aggregates form the part with a size larger than 4.75 mm and a maximum of 40 mm. It should be noted that the coarse aggregate used in the mixture is of two types:
10 mm coarse aggregate.
20 mm coarse aggregate.
2.2 Self–healing methods
Micro cracks in concrete are self-healed due to the recrystallization of calcite. This study clearly shows that with the right combination of chemical and/or biological agents as well as the degree of saturation, concrete can repair defects by itself. Under the appropriate circumstances, carbon dioxide (CO2) in the environment gets diluted with water, but this carbon dioxide transforms and produces calcium carbonate. When it comes into contact with CO2, the calcium carbonate crystallization of the concrete surface cracks, while the cracks heal spontaneously through the hydrolysis of unhydrated cement by the carbonation of dissolved calcium hydroxide.
In concrete technology, further hydrolysis of unhydrated cement, calcite precipitation, and cement paste swelling are both common concrete strengthening technologies that, after mixing, can act as a buffer against the intrusion of harmful substances or help maintain the properties of the concrete and help recover materials. In , it is shown on a positive medium.
Figure 1. Autogenously self-healing concrete specifications (Mahmoodi & Sadeghian, Citation2019).

Unfortunately, the previously mentioned parameters impose limitations on that process. According to estimates, about 80% of the concrete hydration process takes place in the first 28 days. Similarly, the creation of calcite, an important component of the self-healing of concrete, is highly dependent on the environment. There needs to be appropriate potassium carbonate or bicarbonates that activate with Ca2+ ions). The structure of its triggered crack seems to have a consequence too. Each of these uncontrollable important determinants might illustrate how researchers revealed a broad spectrum of reconditioned fracture width (5 m to 300 m) related to autogenously self-healing. As stated previously, crack morphology becomes fundamental whenever trying to determine autogenously self-healing effectiveness; consequently, tweaking concrete fibers to produce micro-cracking activity is essential.
Many microorganisms and non-urolytic substances can be used to close micro cracks. Under the right conditions, microorganisms produce calcium carbonate crystals. When ureolytic bacteria are eliminated, the urease enzyme is synthesized. Ammonia produced by bacteria provides the basis for the synthesis of hydrogen peroxide to carbon dioxide and ammonia. The pH rises as such a core component of ammonium so as a logical consequence of hydrolysis, as described in EquationEquation (1)(1)
(1) , permitting Calcium (Ca2+) and CO2 to also be synthesized. Calcium carbonate (CaCO3) was produced by combining conductive polymer and in the detection of bacterial organisms, particularly described in EquationEquation (2)
(2)
(2) . The main concern is only an example of a chemical reaction
(1)
(1)
(2)
(2)
Where
- is Carbonic acid
- is Hydron
- is Bicarbonate
- Calcium cation
- is Hydroxide
- is Calcium carbonate
- is Water
The proportion and frequency of biogenic CaCO3 synthesized, which is directly proportional to the amount and rate of urea hydrolyzed, are strongly attributable to the crack healing/sealing productivity, and methodology appears significantly quicker inside the presence of urease, as described previously. As a result, bacterial urease productivity and urea concentration are indeed the two most critical regulators of CaCO3 precipitation rate and amount.
The current article used the ‘Fungi (Trichoderma Reesei) in (Potato Dextrose Agar) PDA’ method. In this term, the Fungi is used for Trichoderma Reesei. (TR) has been obtained from the Microbial Type Culture Collection and Gene Bank (MTCC) (Mahmoodi & Sadeghian, Citation2019). Fungi TR is detailed in MTCC according to (Parashar, Citation2017).
Table 2. Fungi TR is detailed in MTCC.
Trichoderma reesei is a mesophilic and filamentous fungus. It is an anamorph of the fungus (asexual reproductive stage) Hypocrea jecorina. T. reesei can secrete large amounts of cellulolytic enzymes (Cellulolytic enzymes are produced as a part of natural energy transfer and carbon cycle, especially by wood-degrading fungi and bacteria.) and form (Potato Dextrose Agar) PDA dextrose and potato injection. Dextrose is used to stimulate growth, the potato infusion provides the basic nutrients for the luxuriant growth of the fungi, and the agar acts as a solidifying agent.
shows the progression of fungi cultivation. The fungi culture (i.e. Trichoderma reesei) which is thus obtained by the above process is cultured in PDA medium and kept in incubation at 380 degrees Celsius for about 2 days. After culture formation in the PDA medium, when kept at room temperature, it forms a layer on top of the PDA broth as shown in and .
PDA (potato dextrose agar) consists of dextrose and potato infusion. Dextrose is used for growth stimulation, potato infusion provides the nutrient base for the luxuriant growth of fungi and agar serves as a solidifying agent. In this term, 300 gr Diced potatoes, 20 gr, Glucose 15 gr, Agar, and 1000 ml Water have been composed.
2.3. Methodology
This study used fungus for self-healing concrete discussed the results of the present research with other studies and finally achieved the prediction results of self-healing concrete with the machine learning method. The method of preparation of Trichoderma reesei Fungi is important to understand the components of the performance and its components.
It should be noted that Trichoderma reesei is a mesophilic filamentous fungus. The fungus Hypocreajecorina has an anamorph. T. reesei appears to have an enzyme capable of converting a variety of cellulolytic enzymes. Microbial cellulosic are used in production to convert cellulose, which is a major component of plant biomass. The primary goal of creating a hypercellulolytic strain is to create a carbon catabolite-deficient strain. The type T. reesei may be able to form cellulose under any growth conditions, in the presence of glucose, as a result of this depression.
To prepare the fungus, eight samples were placed in Petri dishes with malt agar to investigate biofilm formation. Trichoderma reesei fungus is transplanted into five samples. Three samples could not be contaminated, from a Petri dish generation inoculated with Trichoderma reesei using only malt agar. Some fungal specimens may survive in the harsh conditions of concrete, including lack of moisture, excessive alkalinity, nutrient deficiency, and severe oxygen deficiency. Despite the hostile environment, some fungal samples can stimulate calcium mineralization in concrete. Fungi are more successful than fungi in crack repair because they may indirectly and directly cause calcium mineralization in such a hostile environment.
Next, the fungi materials to the concrete. According to the test method, fungal self-healing concrete is determined based on its effectiveness in filling cracks in concrete. The concrete sample blocks shall be cast using the same mix design used for the compression experimental test. The gap must then be created manually on the top surface of the block by inserting a thin sheet-like structure into the concrete just after the concrete mixture has been placed in the mound. The main precaution should be taken that the material selected for the preparation of the gap should be easily removable and oil before it is inserted. The solid plate must be removed before the final setting of the concrete. Then after 24 hours of casting, the blocks are molded and placed in the curing tank for 7 days. The block removed from the curing tank should be injected with fungal extract daily until it expels the calcium carbonate and fills the gap. The material thus filled by fungi should be tested for the presence of calcium carbonate. The crack shown in was 70 mm*30 mm*15 mm on the surface of the cubes. In addition, the mixture design was according to .
Table 3. Mixture design of the current study.
Trichoderma Reesei Fungi with different fungal broth/liter of added water. Normal concrete with a crack of 70 mm*30mm*15mm and three other types of mixed designs with 5.05, 6.73, and 8.42 were added to the concrete. The concrete samples were 150 mm*150mm*150mm for the compression experimental test (BS EN-1991-1-1 Eurocode 2, Citation2004; BS EN 206, Citation2014).
In this research, an experimental study was first created, and then the results of the machine layering method were used to find the prediction results.
Machine learning provides systems with the ability to automatically learn and improve processes from experience without explicit programming. Given the nature of the input that is provided to a machine-learning algorithm (De Marchi & Mitchell, Citation2019). Recently, ‘Python’ has become the most popular programming language for data science and ML. Therefore, in this research, three machine learning models including ‘lasso regression’, ‘ridge regression’ and ‘linear regression’ were implemented with ‘Python’ code using ‘Anaconda’ software. Spyder of the navigation ‘Anaconda’ was chosen to run the model. ‘Sklearn’, an open-source machine learning library for ‘Python’, was also used to analyze the predictive data.
Hematibahar et al. (Citation2022) studied the prediction of the compressive stress-strain curves of concrete. Finding the stress-strain curve of the concrete, the current study used the Logistic Algorithm to predict the compressive stress-strain curve of compressive strain. The current algorithm can find the compressive stress-strain curve of the concrete according to EquationEquation (3)(3)
(3) :
(3)
(3)
where
is the Logistic Function,
is the strain of the concrete on the compressive strength, the
maximum strain of the concrete.
is defined as the Real Parameter of the Logistic Function:
(4)
(4)
where f’c is compressive strength and u is the Real Parameter of the Logistic Function.
Two machine learning methods have been developed to predict compressive strength. Linear regression (LR) and ridge regression (RR) are applied to find the predicted compressive strength. Python software was used to prepare the program because of machine learning coding in this research.
Linear Regression (LR) equations are defined (Hasanzadeh et al., Citation2022):
(5)
(5)
where x and y are variables, and a, and b are the slope and intercept coefficient, respectively.
Lasso Regression is known as (Arvas et al., Citation2006):
(6)
(6)
where there is a good approximation of y and the parameters to minimize the residual sum of squares (RSS). xi and xij are known as corresponding samples. Lasso regression can minimize RSS.
Ridge Regression (RR) Ridge regression, also known as Tikhonov regularization, was used as the main statistical method for calibration. This regression technique is used to reduce the variability of estimates by intentionally introducing bias in coefficient estimation with a penalized estimator (Spencer et al., Citation2018) (EquationEquation (7)(7)
(7) ):
(7)
(7)
where Xi is the vector of independent variables in the observation I, n is the number of observations; p is the number of independent variables; j denotes the sequence of the number of parameters; and Kj denotes the ‘jth ‘regression coefficient within the total number of p; XiT denotes transposed Xi; yi is the dependent variable value in the observation I; K is the vector of regression coefficients; K0 is the intercept coefficient.
To find the accuracy of regression methods, correlation coefficient (R2), mean absolute errors (MAE), and root mean square error (RMSE) have been used. EquationEquation (8)(8)
(8) shows the formula of R2:
(8)
(8)
where y, ŷ, and
are the actual, predicted, and mean of the actual value, respectively.
The MAE method equation is equal to the sum of the numerical differences of the values of the community set divided by whole numbers (EquationEquation (9)(9)
(9) ). The MAE equation is defined as (EquationEquation (9)
(9)
(9) ):
(9)
(9)
RMSE calculates the average deviation of each actual data point and the predicted results. (EquationEquation (10)(10)
(10) ):
(10)
(10)
In general, the purpose of this article is to find the effect of Trichoderma Reesei Fungi on the healing of concrete. In addition, the effect of the amount of water added to the fungi Petri dishes and the concrete. In addition, find out the relationship of concrete compressive strength with water content and compressive strength prediction through machine learning.
2.3.1. Preparation of mortar specimen for checking the survival of T Reesei Fungi
A step-by-step procedure should be followed to prepare the air-entrained concrete mix. First, in proportion to the mixture, the ingredients must be weighed using the batching process. After separating each ingredient according to their quantity, dry mixing should be done. Mixing coarse aggregate, fine aggregate, and cement before adding water to it. After a few minutes of dry mixing, water should enter the phases along with the superplasticizer. Then aluminum is added in the required amount at the end to obtain porous concrete. According to the need, fungi along with PDA medium should be added to the concrete mixture and stirred manually, that is, manual mixing should be done. The obtained concrete should be placed in the required form and compacted in parallel. Then, after the final setting time of concrete, molding should be done. After molding the concrete samples, they should be kept for the necessary period for processing and tests should be performed accordingly.
Trichoderma reesei fungus. The mortar sample was prepared using normal Portland cement grade 53 and zone II sand and added to the mixture to make porous aluminum mortar. The ratio of the materials used to prepare the mortar sample is 0.5 water to cement weight ratio, 3 sand to cement weight ratio.
After taking the ingredients, mixing was done by manual mixing. Then, the dough was poured into Petri dishes measuring 15 x 100 mm, and after 10 hours (that is, the final setting of the concrete), the petri dish was filled with water, then the mushrooms were poured on the prepared mortar samples after processing for 7 days. With nutrients, it was then kept under observation for 10 days to provide daily nutrients to the fungi.
After 10 days of inspection, the water was poured into the Petri dish and then drained into the flask. Then the nutrients are poured into the flask and kept in an incubator at 38 degrees Celsius for 2 days. After 2 days of incubation, it was observed that the colonies had multiplied, thus indicating the survival of Trichoderma Reesei.
2.3.2. Survival test for T ressei in plasticizer and aluminum powder
Before introducing T reesei fungus into the concrete mixture, it is essential to check its survival in the first place. Therefore, checking its survival in the chemical compounds used in the concrete mixture is done. The softener, Uramix, is taken in a small amount and diluted with water in one glass, and a small amount of aluminum powder is taken in the other glass. Then, T reesei along with its nutrients were introduced into both jars and placed at room temperature for 7 days. Then, after 7 days of observation, the solution inside the glass was poured into two different flasks. Then these flasks were added with nutrients (i.e. PDA medium) and kept in an incubator for 3 days. After their removal from the incubators, T reesei colonies were observed to multiply on average, indicating that T Reese survived in both environments.
2.3.3. Compressive strength
The compressive strength experimental test is used to determine the hardness of cubic and cylindrical concrete samples. The strength of concrete samples depends on cement, aggregate, bond, water-cement ratio, thermal repair, and the age and size of the sample. Mix design is the main factor in setting concrete strength.
The sample size used for casting concrete cubes is 150x150x150 mm. The concrete mix is prepared based on the calculated mix plan. After placing the concrete in the sample, it should remain for 24 hours for an ideal setting. After 24 hours of concreting, the blocks can be molded and placed in the curing tank. Processing should be done for 7 days, 14 days, and 28 days. After the curing period, the cubes should be removed from the water tanks and dried before performing the surface compression test. After the surface of the block is dry, you should place it under the pressure testing machine and note the maximum load. Using the following formula and the test load, the compressive strength should be evaluated.
2.3.4. Crack filling ability of fungi in concrete
The ability of fungi to heal concrete is determined by their efficiency in filling cracks in concrete. The concrete sample blocks shall be cast using the same mix design used for the compression experimental test. The crack should then be created manually on the upper surface of the block by inserting a thin sheet-like structure into the concrete just after placing the concrete mixture in the sample. The main precaution should be taken that the material selected for the preparation of the gap should be easily removable and lubricated before it is inserted. The solid plate must be removed before the final setting of the concrete. Then after 24 hours of casting, the blocks are molded and placed in the curing tank for 7 days. The block removed from the curing tank should be injected with fungus extract daily until it expels the calcium carbonate and fills the gap. The material thus filled by fungi should be tested for the presence of calcium carbonate.
3. Results and discussion
3.1. Mechanical properties, and failure mechanism
In this section, the mechanical properties and failure mechanism of the self-healing concrete have been investigated. The compressive strength of the current study has been illustrated in . The results show that the material was able to fill the crack width and heal the concrete. Moreover, improves the compressive strength of concrete.
According to the results, the compressive strength of concrete will increase with adding with increasing the amount of healing materials. Trichoderma Reesei Fungi on a microscopic scale is similar to the small fiber that prevents the failure of the specimen in a compression test.
Other research also shows the same results, for example, Su et al. (Citation2021) Bacillus megatrium microbial spores, nutrients, and low-alkalinity carriers generally collected by extrusion are used to heal micro- and macro-cracks in concrete. According to their results, compared to glucose, calcium lactate when used as a precursor has a better therapeutic effect and the average healing depth reaches 4000 µm. Their results show that the reference concrete and the concrete with additional microbes have the same compressive strength at 7-day curing periods after cracking. In another example, Wang et al. (Citation2021) used CaCO3 to heal the concrete micro and macro cracks in the three different samples. The results show that the compressive strengths of each of the three samples have the same results and adding the CaCO3 was successful. For example, conventional concrete compressive strength was 41 MPa, when 1.5 gr CaCO3 was added to the concrete, the compressive strength grew to 41.2 MPa and in another specimen compressive strength was 43.9 MPa. On the other hand, Zhang et al. (Citation2021) have investigated the same topic as the current study, fungi. They studied the potential of filamentous fungi, Fusarium oxysporum to heal the concrete micro and macro cracks. The results show that mycelium hydrophobicity reduces water penetration to 17.22% of the normal surface of concrete, which is another advantage over Microbial Induced Carbon Precipitation (MICP) in improving the durability of concrete. In the current study, compressive strength of concrete has been increased step by step, the compressive strengths are 17.8 MPa, 18.3 MPa, 18.5 MPa, and 18.8 MPa for 0 ml, 5.05 ml, 6.73 ml, and 8.42 ml fungal broth with liter of water respectively.
For example, shows the Trichoderma Reesei Fungi microscopic scale that is similar to fibers. The concrete samples are changed to red color after using this type of material as the healing material.
also shows that as the scour width increases, the growth of water scratch is significantly higher than the water scratch levels. This hypothesis is because cracks develop in the mortar and the coating, which leads to more damage than scratching. As a result, the probability of water entering through cracks in the mortar is higher. Obviously, with the increase of water from 100 to 259 ml, the concrete crack increased from 2 mm to 30 mm.
3.2. Relationship between fungal and compressive strength
In this part, the article presented a relationship equation according to the mathematical evidence. The relationship between fungal water broth and compressive strength has been established according to the quadratic equation. The quadratic equation was chosen due to similarities between 28 days of concrete compressive strength ().
EquationEquation (11)(11)
(11) was obtained from fungal broth and a liter of water (mm) with concrete compressive strength and mathematical evidence, thus, the quadratic equation helped to find EquationEquation (11)
(11)
(11) due to its similarity to 28-day concrete compressive strength in
(11)
(11)
According to EquationEquation (11)(11)
(11) , where
is the compressive strength,
is the compressive strength of the ordinary concrete, and
is Fungal Broth with Liter of Water (mm). shows the predicted results according to EquationEquation 8
(8)
(8) and experimental results.
Table 4. Ordinary concrete prediction according to Equation (8).
Table 5. Prediction of compressive strength of concrete with 5.05 Fungal Broth with Liter of Water (ml) prediction according to Equation (8).
Table 6. Prediction of compressive strength of concrete with 6.73 Fungal Broth with Liter of Water (ml) according to Equation (8).
Table 7. Prediction of compressive strength of concrete with 8.42 Fungal Broth with Liter of Water (ml) according to Equation (8).
The results of show that ordinary concrete has the best prediction, also even also the reduction of Fungal Broth with a Liter of Water also decreased the accuracy of the concrete prediction. For example, the R2 was more than 0.98 for 5.05 Fungal Broth with a Liter of Water (mm) while R2 was more than 0.79 for 8.42 Fungal Broth with a Liter of Water (mm).
3.3. Machine learning prediction and data mining
The data science results show that the fungi have improved the crack compressive strength by more than 0.85%. The effect of the fungal improved gradually. In addition, the curing age is positively related to the compressive strength of concrete. It should be noted that fungal concentration has a positive relationship with compressive strength, while it has a negative relationship with cooking periods ().
For the prediction of the compressive stress-strain curves, the current articles used the Logistic Function Method (Hematibahar et al., Citation2022) (). shows the prediction stress-strain curve of each sample illustrating the the correlation between self-healing concrete parameters: conventional concrete at 7,14,28 days (), 5.05 lit/water at 7,14,28 days (), 6.73 lit/water at 7,14,28 days (), 8.42 lit/water at 7,14,28 days (). The Logistic Function is used in applied mathematics to find and predict the compressive stress-strain curve of concrete. The stress-strain curves of concrete samples have been predicted with high accuracy.
Figure 10. The correlation between self-healing concrete parameters; (a), conventional concrete, 7,14,28 days; (b), 5.05 lit/water 7,14,28 days; (c) 6.73 lit/water 7,14,28 days; (d), 8.42 lit/water, 7,14,28 days.

The prediction of the compressive strength is shown in . The Linear Regression (LR) results are shown in . According to the Linear Regression prediction results, the prediction was close to the experimental results. The R2 results were 0.98, RMSE 0.24, and MAE 0.19 ().
Lasso Regression (LAR) was another type of regression in this study, the results of R2 were more than 0.93, RMSE was 0.94 and MAE was 0.78. the accuracy of the current results was less than LR ().
This study has analyzed the Rigid Regression (RR) the results of the current regression was shown that the R2 was more than 0.98, RMSE 0.23, and MAE 0.21. In this term, two regressions of the LR and RR were suitable to predict the compressive strength in this study ().
4. Conclusion
In this study, the effect of Fungus Trichoderma Reesei on the self-healing of concrete has been investigated. The Fungus Trichoderma Reesei has to be grown under the special condition Trichoderma Reesei is a mesophilic filamentous fungus. The fungus Hypocreajecorina has an anamorph. T. reesei appears to have an enzyme capable of converting a variety of cellulolytic enzymes. Microbial cellulosic are used in production to convert cellulose, which is a major component of plant biomass. The primary goal of creating a hypercellulolytic strain is to create a carbon catabolite-deficient strain. This type of fungus helps to make Ca on the concrete and heal the micro-cracks on the deep surface of the concrete. The cubic sample was 150 mm3 for the compressive strength experimental test. The crack was 70 mm*30 mm*15 mm on the surface of the cubes. According to the method, fungal materials are added to the concrete cracks to have a healing effect. In this study, different numbers of fungal broth with liters of water in mile liter 0, 50.5, 6.37, and 8.42 have been added to the cracked concrete. In addition, the machine learning method has been applied to predict the compressive strength through Linear regression, Lasso regression, and Rigid regression. The relationship between Fungus water to ml and compressive strength has been found by the quadratic equation. Also, the compressive stress-strain curve has been forecasted by Logistic Function with high accuracy.
The results show that the effect of Trichoderma Reesei Fungus on compressive strength and healing cracks on concrete was positive. The improvement of cracks is related to the amount of additional water in the fungus broth, if there was more water, the crack would have grown. Finally, this research has been flowing the results:
The influence of water in the preparation process of Trichoderma reisi Fungus is one of the important issues. So the lower the amount of water, the better it is to heal the concrete crack, while the compressive strength increased with the addition of more water.
Trichoderma reesei is a mesophilic filamentous fungus. Microbial cellulose is used in production to convert cellulose, which is the main component of plant biomass. The results show that this type of fungus is effective for curing concrete and increasing compressive strength.
The maximum compressive strength for adding 8.42 ml of mushroom broth with one liter of water was more than 18.99 MPa. Also, the prediction results show that linear regression R2 is more than 0.98, and R2 for rigid regression is also more than 0.98. Furthermore, the relationship prediction results show that the prediction of compressive strength decreases as the mushroom broth is reduced by one liter of water (ml).
Data availability statement
All data are included in the body of the article.
Disclosure statement
No potential conflict of interest was reported by the author(s).
References
- Agrawal, R. (2022). Sustainable design guidelines for additive manufacturing applications. Rapid Prototyping Journal, 28(7), 1221–1240. https://doi.org/10.1108/RPJ-09-2021-0251
- Akande, K. O., Owolabi, T. O., Twaha, S., & Olatunji, S. O. (2014). Performance comparison of SVM and ANN in predicting compressive strength of concrete. IOSR Journal of Computer Engineering, 16(5), 88–94. https://doi.org/10.9790/0661-16518894
- Akter, S., Ali, R. M. E., Karim, S., Khatun, S., & Alam, M. F. (2018). Geomorphological, geological and engineering geological aspects for sustainable urban planning of Mymensingh City, Bangladesh. Open Journal of Geology, 08(07), 737–752. https://doi.org/10.4236/ojg.2018.87043
- AlAlaween, W., Abueed, O., Gharaibeh, B., Alalawin, A., Mahfouf, M., Alsoussi, A., & Albashabsheh, N. (2022). The development of a radial based integrated network for the modelling of 3D fused deposition. Rapid Prototyping Journal, 29(2), 408–421. https://doi.org/10.1108/RPJ-04-2022-0121
- Arvas, M., Pakula, T., Lanthaler, K., Saloheimo, M., Valkonen, M., Suortti, T., Robson, G., & Penttilä, M. (2006). Common features and interesting differences in transcriptional responses to secretion stress in the fungi trichoderma reesei and Saccharomyces cerevisiae. BMC Genomics, 7(1), 32. doi:https://doi.org/10.1186/1471-2164-7-32.
- Basu, S., Bose, C., Ojha, N., Das, N., Das, J., Pal, M., & Khurana, S. (2015). Evolution of bacterial and fungal growth media. Bioinformation, 11(4), 182–184. https://doi.org/10.6026/97320630011182
- Bhosale, S., & Vijayalakshmi, D. (2015). Processing and nutritional composition of rice bran. Current Research in Nutrition and Food Science Journal, 3(1), 74–80. https://doi.org/10.12944/CRNFSJ.3.1.08
- BS EN 206. (2014). Concrete—specification, performance, production and conformity. British Standard Institutions.
- BS EN-1991-1-1 Eurocode 2. (2004). Actions on structures—Part 1-1: General rules and rules for buildings. British Standards Institution.
- C., Onyelowe, K., M., Ebid, A., Riofrio, A., Baykara, H., Soleymani, A., A., Mahd, H., Jahangir, H., & Ibe, K. (2022). Multi-objective prediction of the mechanical properties and environmental impact appraisals of self-healing concrete for sustainable structures. Sustainability, 14(15), 9573. https://doi.org/10.3390/su14159573
- Chopra, P., Sharma, R. K., & Kumar, M. (2016). Prediction of compressive strength of concrete using artificial neural network and genetic programming. Hindawi Publishing Corporation Advances in Materials Science and Engineering, 2016, 1–10. 10 https://doi.org/10.1155/2016/7648467
- De Marchi, L., & Mitchell, L. (2019). Hands-on neural networks: Learn how to build and train your first neural network model using python. Packt Publishing.
- Dębska, B. (2022). Assessment of the applicability of selected data mining techniques for the classification of mortars containing recycled aggregate. Materials (Basel, Switzerland), 15(22), 8111. https://doi.org/10.3390/ma15228111
- Erdal, H. I. (2013). Two-level and hybrid ensembles of decision trees for high performance concrete compressive strength prediction. Applied Artificial Intelligence, 26(7), 1689–1697. https://doi.org/10.1016/j.engappai.2013.03.014
- Farooq, F., Czarnecki, S., Niewiadomski, P., Aslam, F., Alabduljabbar, H., Ostrowski, K. A., Śliwa-Wieczorek, K., Nowobilski, T., & Malazdrewicz, S. (2021). A comparative study for the prediction of the compressive strength of self-compacting concrete modified with fly ash. Materials, 14(17), 4934. https://doi.org/10.3390/ma14174934
- Fl ¨ory, S., & Pottmann, H. (2019). Ruled surfaces for rationalization and design in architecture. In LIFE in: Formation. On Responsive Information and Variations in Architecture, pp. 103–109.
- Hasanzadeh, A., Vatin, N. I., Hematibahar, M., Kharun, M., & Shooshpasha, I. (2022). Prediction of the mechanical properties of basalt fiber reinforced high-performance concrete using machine learning techniques. Materials (Basel, Switzerland), 15(20), 7165. https://doi.org/10.3390/ma15207165
- Hematibahar, M., Vatin, N. I., Alaraza, H. A. A., Khalilavi, A., & Kharun, M. (2022). The prediction of compressive strength and compressive stress–strain of basalt fiber reinforced high-performance concrete using classical programming and logistic map algorithm. Materials, 19, 6975. https://doi.org/10.3390/ma15196975
- Huang, F., & Zhou, S. (2022). Review of lightweight self-healing concrete. Materials, 15(21), 7572. https://doi.org/10.3390/ma15217572
- Huang, X., Wasouf, M., Sresakoolchai, J., & Kaewunruen, S. (2021). Prediction of healing performance of autogenous healing concrete using machine learning. Materials (Basel, Switzerland), 14(15), 4068. https://doi.org/10.3390/ma14154068
- Kashyzadeh, K. R., Amiri, N., Ghorban, S., & Souri, K. (2022). Prediction of concrete compressive strength using a back-propagation neural network optimized by a genetic algorithm and response surface analysis considering the appearance of aggregates and curing conditions. Buildings, 12(4), 438. https://doi.org/10.3390/buildings12040438
- Khan, M. A., Memon, S. A., Farooq, F., Javed, M. F., Aslam, F., & Alyousef, R. (2021). Compressive strength of fly-ash-based geopolymer concrete by gene expression programming and random forest. Advances in Civil Engineering, 2021, 1–17. https://doi.org/10.1155/2021/6618407
- Khan, N., Anis Khan, H., Khushnood, R. A., Faraz Bhatti, M., & Ilyas Baig, D. (2023). Self-healing of recycled aggregate fungi concrete using fusarium oxysporum and trichoderma longibrachiatum. Construction and Building Materials, 392, 131910. https://doi.org/10.1016/j.conbuildmat.2023.131910
- Khorasani, M., Loy, J., Ghasemi, A., Sharabian, E., Leary, M., Mirafzal, H., Cochrane, P., Rolfe, B., & Gibson, L. (2022). Review of Industry 4.0 and additive manufacturing synergy. Rapid Prototyping Journal, 28(8), 1462–1475. https://doi.org/10.1108/RPJ-08-2021-0194
- Kumar, A., Harish, C. A., Raj Kapoor, N., Mazin, A. M., Kumar, K., Majumdar, A., & Thinnukool, O. (2022). Compressive strength prediction of lightweight concrete: Machine learning models. Sustainability, 14(4), 2404. https://doi.org/10.3390/su14042404
- Luo, J., Chen, X., Crump, J., Zhou, H. G., Davies, D., Zhou, G., Zhang, N., & Jin, C. (2018). Interactions of fungi with concrete: significant importance for bio-based self-healing concrete. Construction and Building Materials, 164, 275–285. https://doi.org/10.1016/j.conbuildmat.2017.12.233
- Mahmoodi, S., & Sadeghian, P. (2019 Self-healing concrete: A review of recent research developments and existing research gaps [Paper presentation]. 7th International Conference on Engineering Mechanics and Materials, CSCE Annual Conference.
- Nguyen, K. T., Nguyen, Q. D., Le, T. A., Shin, J., & Lee, K. (2020). Analyzing the compressive strength of green fly ash based geopolymer concrete using experiment and machine learning approaches. Construction and Building Materials, 247, 118581. https://doi.org/10.1016/j.conbuildmat.2020.118581
- Parashar, A. (2017). International depository authority and its role in microorganism’s deposition. Journal of Clinical and Diagnostic Research: JCDR, 11(8), DE01–DE06. https://doi.org/10.7860/JCDR/2017/29077.10408
- Peng, X., Zhuang, Z., & Yang, Q. (2022). Predictive modeling of compressive strength for concrete at super early age. Materials (Basel, Switzerland), 15(14), 4914. https://doi.org/10.3390/ma15144914
- Salem, G. G., Galishnikova, V. V., Elroba, S. M., Vatin, N. I., & Kharun, M. (2022). Finite element analysis of self-healing concrete beams using bacteria. Materials, 15(21), 7506. https://doi.org/10.3390/ma15217506
- Sami Ullah, H., Khushnood, R. A., Farooq, F., Ahmad, J., Vatin, N. I., & Zakaria Ewais, D. Y. (2022). Prediction of compressive strength of sustainable foam concrete using individual and ensemble machine learning approaches. Materials (Basel, Switzerland), 15(9), 3166. https://doi.org/10.3390/ma15093166
- Shahmansouri, A. A., Yazdani, M., Hosseini, M., Akbarzadeh Bengar, H., & Farrokh Ghatte, H. (2022). The prediction analysis of compressive strength and electrical resistivity of environmentally friendly concrete incorporating natural zeolite using artificial neural network. Construction and Building Materials, 317, 125876. https://doi.org/10.1016/j.conbuildmat.2021.125876
- Spencer, B., Alfandi, O., & Al-Obeidat, F. (2018). A refinement of lasso regression applied to temperature forecasting. Procedia Computer Science, 130, 728–735. https://doi.org/10.1016/j.procs.2018.04.127
- Su, Y., Zheng, T., & Qian, C. (2021). Application potential of bacillus megaterium encapsulated by low alkaline sulphoaluminate cement in self-healing concrete. Construction and Building Materials, 273, 121740. https://doi.org/10.1016/j.conbuildmat.2020.121740
- Topçu, I. B., & Sarıdemir, M. (2007). Prediction of properties of waste AAC aggregate concrete using artificial neural network. Computational Materials Science, 41(1), 117–125. https://doi.org/10.1016/j.commatsci.2007.03.010
- Wang, W., Chen, S., Yang, Z., Ren, J., Zhang, X., & Xing, F. (2021). Self-healing concrete incorporating mineral additives and encapsulated lightweight aggregates: Preparation and application. Construction and Building Materials, 301, 124119. https://doi.org/10.1016/j.conbuildmat.2021.124119
- Yang, D., Yan, C., Liu, S., Jia, Z., & Wang, C. (2022). Prediction of concrete compressive strength in saline soil environments. Materials (Basel, Switzerland), 15(13), 4663, https://doi.org/10.3390/ma15134663
- Zhang, X., Fan, X., Li, M., Samia, A., & (Bill) Yu, X. (2021). Study on the behaviors of fungi-concrete surface interactions and theoretical assessment of its potentials for durable concrete with fungal-mediated self-healing. Journal of Cleaner Production, 292, 125870. https://doi.org/10.1016/j.jclepro.2021.125870
- Zhao, J., Csetenyi, L., & Gadd, G. M. (2022). Fungal-induced CaCO3 and SrCO3 precipitation: A potential strategy for bioprotection of concrete. The Science of the Total Environment, 816, 151501. https://doi.org/10.1016/j.scitotenv.2021.151501
- Zheng, X., Peng, X., Zhao, J., & Wang, X. (2022). Trajectory prediction of marine moving target using deep neural networks with trajectory data. Applied Sciences, 12(23), 11905. https://doi.org/10.3390/app122311905
- Zhuang, X., & Zhou, S. (2019). The prediction of self-healing capacity of bacteria-based concrete using machine learning approaches. Computers, Materials & Continua, 59(1), 57–77. https://doi.org/10.32604/cmc.2019.04589