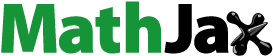
Abstract
Machining of Inconel 800 superalloy is associated with inherent issues like lower tool life and lower quality of machined surface owing to the work-hardening nature of the superalloy and increased mechanical and thermal stresses. The employment of cutting fluid in machining negatively affects the locale. Hence, dry machining is a feasible alternate solution. This work aims to optimize the cutting parameters (CP): cutting speed, feed rate and depth of cut in the high-speed dry turning of Inconel 800 employing an uncoated carbide insert to minimize the responses: tool wear (TW) and surface roughness (SR). The superalloy machining is conducted as per the experimental runs designed applying the Taguchi analysis. Then, the effects and contributions of the CP on the outputs were examined employing the signal-to-noise (S/N) ratio and the analysis of variance (ANOVA). Additionally, the multi-objective optimization (MOO) method grey relational analysis was employed to optimize CP. The results of the research work showed that cutting speed, feed rate, and depth of cut have noteworthy sway on TW and SR with a % contribution of 33.3, 13.8 and 23.7, respectively. Additionally, evaluation of SEM images of the cutting insert revealed that the abrasion, adhesion and diffusion are the primary wear mechanisms leading to abrasion groves, crater, chipping, built-up-edge and notch formation.
REVIEWING EDITOR:
1. Introduction
Certain mechanical components operating under the worst conditions are expected to retain their properties and are manufactured from superalloys. However, machining the superalloys with higher precision is a challenge. Inconel 800 is one of the nickel-chromium-iron superalloy from the Inconel family widely used in steam tubing, heat exchangers and cooling holes. Some specific application includes superheater tubes in the Kapar power station in Malaysia (Ahmad et al., Citation2010), tubing for Canada Deuterium Uranium (CANDU) reactors and steam generators for pressurized water reactors with German design (Griffiths, Citation2019). Inconel 800 is referred to as a ‘hard to cut material’ due to the work-hardening effect, extreme tool wear (TW) and poor surface quality. Thus, studying the machinability of Inconel 800 and modeling TW and surface roughness (SR) has attracted considerable interest. Sustainable and cleaner production relies on the concept of producing products that do not disturb the environment and conserve natural resources. The same idea can also be expressed by the 3 P approach, which stands for ‘People, Planet and Profit’ (Gimenez et al., Citation2012). In the current scenario, the manufacturing industries are looking for sustainable production techniques due to strict environmental norms (Sarma et al., Citation2020).
Dry or near-dry machining is considered one of the sustainable techniques in machining sciences (Roy et al., Citation2019). Dry machining does not utilize cutting fluids. Dry machining is rapidly being used by manufacturers, particularly those who make metal items and is becoming quite common in today’s modern manufacturing processes. Nevertheless, dry machining faces many challenges, such as surface flaws, lower tool life, high temperature, etc. Thus, it is essential to carry out the dry machining and optimize the responses to benefit the industrialist. Many researchers are currently working even on dry machining to help the manufacturing society. Wagri et al. (Citation2023) investigated the dry turning (DRT) of 4340 alloy steel and reported that the feed rate was the critical factor influencing the surface quality, followed by cutting speed on surface quality. Alemayoh et al. (Citation2023) concluded that cutting speed was the most prominent factor, contributing 56.85% to the responses during the DRT of 1030 carbon steel. The optimal CP from grey relational analysis (GRA) for cutting speed, depth of cut and feed rate reported as 90 m/min, 0.25 mm and 0.15 mm/rev, respectively. Grigoriev et al. (Citation2023) examined the effect of the coating during the DRT of Inconel 718. The findings revealed that the coefficient of friction increased with thermal load; however, after reaching 800 °C, friction values started to decrease. Vukelic et al. (Citation2020) investigated DRT on Inconel 60 l and indicated that lower feed rate and depth of cut and higher cutting speed and insert radii were favorable. The authors specified that adopting relatively high cutting speed was a better strategy from a sustainable point of view. Francis Xavier et al. (Citation2021) conducted the DRT of Nimonic C263 and observed that the feed rate was a prominent parameter influencing the responses viz., Flank wear (FW) and SR. The average prediction error between the experimental and response surface methodology (RSM) model for FW and SR was found to be 2.79% and 4.76%, respectively. Thakur et al. (Citation2016) observed lower SR during the DRT of Nimonic 6263 for the uncoated carbide inserts than the coated carbide insert. However, the multilayer coated insert performed better at higher cutting speed for given feed rate and depth of cut values. Jovicic et al. (Citation2023) worked on optimizing SR, TW and metal removal rate (MRR) during DRT of Inconel 601 alloy. The process parameter was modeled using ANN and optimized using genetic algorithm. The experimental findings suggest that augmenting the feed positively influences MRR but negatively impacts SR and TW. Similarly, escalating the cutting speed has a positive effect on MRR and SR but a negative impact on TW.
Recently, researchers have paid attention to high-speed machining (HSM) of difficult-to-machine materials. In HSM, the cutting speed employed is relatively higher, which results in increased MRR and lower cutting force. Thus, HSM improves overall machining efficiency and productivity (Qazi et al., Citation2021; Sahoo et al., Citation2013; Tu et al., Citation2022). Patel et al. (Citation2022) conducted HSM studies on stainless steel under dry conditions and observed lesser wear and extended tool life under PVD inserts. Tu et al. (Citation2022) investigated the HSM of GH4169 superalloy under dry conditions and recommended the optimal cutting speed of 200–300 m/min. The authors observed the tool’s rapid wear and catastrophic failure at 100 m/min. Zhou et al. (Citation2019) compared the HSM of Inconel 718 and AD730 alloy and observed lower SR for AD730 with lesser feed marks. Tian et al. (Citation2021) performed the HSM of iron-based superalloy under dry conditions using a carbide insert. Increase in FW, SR showed a trend that decreased first and then rose. Also, the authors recommend avoiding the usage of coated tools at high cutting speed. Thus, many researchers have worked on the HSM and observed favorable results. The study also aims to probe the HSM of Inconel 800 under DRT conditions. Xue et al. (Citation2022) evaluated TW mechanisms during HSM of Inconel 718 using ceramic insert. In the early phase, the formation of notches was contingent on the ceramic tools’ thermal shock resistance and fracture toughness. As the notching progressed, the adhesion mechanism took precedence in influencing the evolution. The oxidation of the workpiece has the potential to diminish the tool’s affinity, consequently exerting a positive influence on reducing notch wear in ceramic tools.
It is a well-known fact that industries focus on enhancing overall production by reducing the tool costs. In that context, choosing optimal cutting parameters (CP) is a must to obtain optimal machining performance. Therefore, optimization techniques are very much needed for selecting a suitable CP that maximizes or minimizes the output performance. Bagaber and Yusoff (Citation2017) conducted MOO of CP using RSM in DRT of stainless steel 316. The optimization results showed that the minimum values of TW, SR and power consumption occurred at 0.184 mm, 0.98 µm and 24.94 Whr, respectively. Senthil Kumar et al. (Citation2021) utilized a regression model to predict the responses of DRT of Nimonic C 263 using CBN inserts. The error % between the predicted responses (temperature and cutting force) using the regression model and experimental results were found to be 1.63% and 2.34%, respectively. Frifita et al. (Citation2020) optimized the CP using RSM during the turning of Inconel 718 and observed that cutting speed and nose radius were the most prominent CP on SR. From RSM analysis, the optimal CP, i.e. cutting speed, feed rate and nose radius for best productivity were reported as 70 m/min, 0.09 mm/rev and 0.4 mm, respectively. Eskandari et al. (Citation2022) performed MOO for the turning operation of Inconel 718 using RSM and noted that cutting speed influenced cutting force in a greater proportion. The feed rate was found to affect FW. However, feed rate and depth of cut both influenced SR.
The literature survey underlines the keenness of research community on studying the effect of CP on the responses by developing models or applying optimization techniques. Further, Inconel 800 is an essential material for various applications at high-temperature working conditions; machining studies (particularly turning) on Inconel 800 are limited. Furthermore, no studies have been noted on the HSM of Inconel 800. Thus, this study investigates the DRT of Inconel 800 at relatively higher cutting speed and inspects the effect of CP (cutting speed, feed rate and depth of cut) on the responses (FW and SR). The major contribution of this article is (i) to investigate the DRT of Inconel 800 under higher cutting speed and (ii) to study the effect of CP on the responses using statistical and optimization techniques.
2. Methodology
The overall methodology implemented in this study is shown in . It starts with the selection of workpiece and corresponding cutting tool for machining. From the literature survey, this study has chosen Inconel 800 as work material and uncoated carbide insert as tool material. Initial studies have been conducted to select actual experimental CP. Based on specified levels of CP (speed, feed and depth of cut), DOE is applied using Taguchi’s method. Turning experiments have been conducted on Inconel 800 based on the DOE plan. After each experiment, SR and TW are measured and tabulated. Also, each stage TW is analyzed using a microscope, and SEM images of some of the TW patterns are captured. Further, some statistical analysis (S/N ratio and ANOVA) is conducted to analyze the effect of speed, feed and depth of cut on the SR and TW. Finally, the MOO technique (GRA) is employed to get the optimal CPs to minimize the SR and TW. The GRA optimization technique is employed on the experimental results provided in this study, to find the optimal combination of the CPs.
3. Experimental procedure
The Inconel 800 workpiece 200 mm in length and 50 mm in diameter is prepared for DRT on a computer numerical control (CNC) turning center (Jobber Jr., ACE Designers LTD. Make). shows the CNC turning center with the workpiece and tool fixed. furnishes details regarding the chemical composition of Inconel 800. The inclusion of Ni promotes ductility, Cr improves heat resistance and Fe strengthens the resistance to internal oxidations. Uncoated carbide inserts (WIDIA make) with the configuration CNMG 120408 are used for the turning operations. displays the specifications of the cutting insert. The PCLNL 2525 M12 tool holder (WIDIA make) is used for turning operations (Widia make).
Table 1. Inconel 800 chemical composition (Gupta, Citation2018; Paul et al., Citation2019).
Taguchi’s L27 orthogonal array is employed to design the experimental runs for three levels of the three CP shown in . To maximize the number of experimental runs and to analyze the variation of responses with CP, Taguchi’s L27 orthogonal array is selected as the design of the experiments (DOE) plan. The CP in are selected based on the initial studies on Inconel 800 alloy. Before performing actual experiments, few trial run have been performed to know the upper- and lower-level values of the CP. Based on the observed wear rate and by considering the constraints of machine tool, the CP are chosen. The actual experiments are conducted as per the obtained experimental plan using DOE (). Each experimental run constituted 10 passes covering 50 mm (a total length of 500 mm for 10 passes). The SR and TW are monitored offline following each run. The curved surface of the workpiece is divided into three equal segments with a spread of 120°, and these segments are used to measure the SR values. Nine randomly chosen locations are used to measure Ra values, and the average value is considered. The Surtronic 3+ (Taylor Hobson make) instrument is used to measure the average value of SR (Ra). The maximal FW width is measured using an Olympus microscope. provides the details of SR and TW measuring instruments. provides the CP and responses of the L27 orthogonal experimental runs.
Table 2. CP considered in the study.
Table 3. Details of measuring instruments.
Table 4. CP and responses for the L27 experimental run.
4. TW evaluation
Superalloys exhibit remarkable mechanical strength retention even at elevated temperatures, surpassing 650 °C and beyond. However, their challenging machinability arises from several key factors:
Rapid work hardening during machining.
Pronounced abrasive wear on cutting insert due to the existence of hard abrasive carbides.
Chemical wear resulting from chemical reactions at high cutting temperatures.
Material diffusion between the workpiece and the tool at elevated temperatures.
Repeated adhesion and welding of workpiece to the cutting tool, causing notable notching and spalling on the tool rake.
Generation of tenacious and continuous chips that are challenging to control during machining, leading to cutting tool deterioration through seizure and cratering.
The limited thermal diffusivity of superalloys, which often results in elevated temperatures at the tool tip and substantial thermal gradients within the cutting tool, leading to prominent thermal stresses on both the cutting tool and workpiece surfaces (Akhtar et al., Citation2014).
The TW in this context can be attributed to several factors, including flank wear, chipping, notching, extensive damage, and, in some cases, catastrophic failure. The predominant wear mechanisms encountered during the machining of superalloys are often attributed to mechanical processes, specifically abrasion and adhesion (Tu et al., Citation2023). These two mechanisms are frequently cited as the primary causes of wear in such machining operations. The Built-Up Edge (BUE) originated as chips from the workpiece adhered to the cutting tool surface through adhesive wear mechanism. The workpiece attached to the tool surface was not firmly bonded and detached under the high-pressure conditions during cutting. As a result, the cutting tool’s lifespan was prominently shortened, with fragments of the tool also breaking off concurrently. Additionally, increase in temperature near the cutting zone leads to heat concentration on tool tip. Thus, the tip acts a hub for material accumulation and leads to BUE formation. The abrasion wear mainly increases the friction and between the tool and workpiece through hard abrasive particles and increase the thermal load in the cutting zone. These abrasion and adhesion wear mechanisms are observed across a range of cutting temperatures, encompassing both low and high extremes. Their occurrence is largely influenced by factors such as the magnitude of contact loads between the tool and work material, as well as the specific combination of tool and work material being used. As cutting speed and, consequently, cutting temperatures rise, thermally activated wear mechanisms become increasingly substantial. This elevated temperature environment also promotes diffusion and chemical interactions between the tool and workpiece, leading to the activation of diffusion and chemical wear mechanisms. As a result, the rate of TW experiences a notable increase under these conditions (Ulutan & Ozel, Citation2011; Yin et al., Citation2020).
shows the SEM images of TW for various combinations of cutting speed and feed rate at depth of cut = 0.6 mm. It is clearly visible that with an increase in the level of CP, TW also rises. In , the abrasion marks are clearly noticeable due to the continuous friction between the flank face and the undeformed workpiece. These abrasion mechanisms will generate additional foreign particles within the cutting zone, thereby intensifying the TW through abrasion (Grigoriev et al., Citation2023; Jovicic et al., Citation2023). In , the formation of the crater with BUE can be readily observable on the tool’s rake face. Crater formation predominantly occurs on the rake face of the tool, attributed to the flow of hard chips with sharp edges possessing high energy, leading to the removal of material from the tool. The BUE occurrence is observed in almost all cases during machining. The increase in crater land weakens the cutting edge and undergoes the fracture. The BUE is always temporary in nature and undergoes a brittle fracture and removes the extra material from the tool surface.
Figure 4. SEM images of TW at various cutting speed and feed rate at depth of cut = 0.6 mm. (a) 175/0.15/0.6 (flank face). (b) 175/0.2/0.6 (flank face). (c) 200/0.2/0.6 (rake face). (d) 225/0.25/0.6 (rake face).

presents the SEM images of TW for several mix of cutting speed and feed rate at depth of cut = 0.8 mm. Because of the increase in levels of CP, severe wear patterns can be noted on the rake of the tool due to extreme rubbing and chipping . Edge chipping typically occurs when the bonding strength between BUE and the cutting tool surpasses the cohesive strength of the tool material. When the stress applied during the cutting process overcomes the comparatively weaker cohesive strength of the tool material, it results in the removal of both the BUE and the tool material, ultimately leading to edge chipping. Chipping is identified as a primary mechanism in TW, often leading to tool failure. Other researchers have also made a similar observation during the machining of superalloys (Podder & Paul, Citation2012; Tan et al., Citation2018).
Figure 5. SEM images of TW at various cutting speed and feed rate at depth of cut = 0.8 mm. (a) 200/0.15/0.8 (rake face). (b) 225/0.25/0.8 (rake face).

shows the SEM images of TW for various combinations of cutting speed and feed rate at depth of cut = 1 mm. Excess BUE formation can be notable in at extreme cutting conditions due to increased cutting temperature and severe plastic deformation. The formation of excess BUE hinders the TW growth. With an increase in levels of CP, cutting temperature increases at the tooltip, and this tip zone acts as a region for material accumulation and results in excess BUE. This BUE formation may serve as beneficial for a certain number of passes. However, this brittle bond breaks and BUE dislodges from the tool surface, resulting in severe TW. An increment in the depth of cut resulted in severe flank wear and crater formation in , apiece. The sharp-edged deformed layers of material are found to rub the tool’s rake face while moving and thus result in severe crater formation.
5. Results and discussion
5.1. Signal-to-noise ratio (S/N ratio)
S/N ratio is employed to select the optimum CP and is described as the ratio of mean to standard deviation. Based on the optimum responses, Taguchi divided the S/N ratio into three groups viz., ‘lower the better, medium the better, and higher the better’. In this study, the responses are SR and FW, which requires the ‘lower the better’ criteria to augment productivity (EquationEq. (1)(1)
(1) ) (Abas et al., Citation2022; Eklarkar & Nandedkar, Citation2021). All Taguchi-based analyses are done using Minitab software.
(1)
(1)
where n = number of observations and R = response data.
5.1.1. Effect of CP on SR
The effect of CP on the SR is shown in . It was noted that as CP increased, the SR also demonstrated an increase due to elevated temperatures at high cutting speed. An increment in cutting speed increases the heat in the cutting zone. Thus, the work material becomes softer, loses chip debris, sticks onto the work material and brings down the surface quality. Additionally, severe vibration at high cutting speed will increase the SR. It is clear that the SR has greatly risen with a rise in feed rate (particularly at high feed rate) because the SR and the feed rate are proportionate to each other. This is evident from the equation
where Ra = SR, f = feed rate, r = nose radius (Abas et al., Citation2020; Sarıkaya & Güllü, Citation2014).
Additionally, the greater propensity to induce vibration and feed marks at higher feed rate might have served as a catalyst for the SR to grow. The rise in the SR trend with a rise in the depth of cut was noted owing to an increment in the temperature and MRR. Similar results have been observed in the previous literature (Gupta et al., Citation2021). The S/N ratio analysis (), provided the optimal CP for SR and are noted, as cutting speed, depth of cut and feed rate at 175 m/min, 0.6 mm and 0.15 mm/rev, respectively.
5.1.2. Effect of CP on FW
indicates that the FW rises with an increment in the cutting speed and the depth of cut. As the cutting speed rises, the cutting temperature in the cutting zone also rises, causing the tool material to become softer. The tool loses its strength gradually and the material will be removed from the tool surface, resulting in FW. This is in agreement with the results in Mou and Zhu (Citation2020). Likewise, an increment in the depth of cut rises the tool and workpiece contact; thus, more friction increases the wear rate. However, the feed rate has a neutral effect on the FW, as observed in .
5.2. Analysis of variance (ANOVA) for SR and FW
The significance of different CP on the DRT of Inconel 800 is analyzed using the ANOVA technique at a 95% confidence level. The results of ANOVA for SR and FW are displayed in and , respectively. Only the feed rate (f) was found to prominently affect the SR as the P-value (probability of significance) is less than 0.05 (5%), with a contribution of 33.9%. indicates that the P-values are less than 0.05 for vc, d and vc × d. Thus, vc, d and vc × d are found to prominently affect the FW with a % contribution of 54.8, 27.8 and 8.99, respectively.
Table 5. ANOVA table for SR.
Table 6. ANOVA table for FW.
5.3. Grey relational analysis
GRA was mainly developed based on the theory of grey systems in 1982 (Ju-Long, Citation1982), and it addresses the various deficiencies of regression analysis. GRA is an easy-to-understand method for computations and optimization. It is a technique for assessing many variables all at once. Many studies have applied the GRA technique to study the CP (Ramakrishna & Srinivas, Citation2021; Sivaiah & Chakradhar, Citation2018; Yelamasetti et al., Citation2021).
The first step in GRA is to normalize the responses based on the ‘lower the better’ or ‘higher the better’ criteria. Since the working range of data greatly varies in magnitude and units, normalization of data helps in maintaining data uniformity. EquationEquation (2)(2)
(2) is used to normalize the responses based on ‘lower the better’ criteria.
(2)
(2)
where i = 1, …, P and k = 1, …, q. Here, p = the number of experiments, and q = the number of parameters.
represents the original sequence and
gives sequence after normalization.
denote the maximum and minimum values of
After normalization, the GRA-coefficient (GRC) ( for the kth quality characteristics in the ith experiment can be articulated as
(3)
(3)
where
= comparability sequence and
= reference sequence. In this study,
is taken as 0.5 as equal importance given to both the responses of machining. Following the computation of GRC, the GRA-based grades (GRG) of every experiment are established by taking into account the average of GRC for each inspection, as given in EquationEq. (4)
(4)
(4) .
(4)
(4)
In this study, the responses viz., SR and FW cannot be compared directly as they are of different magnitudes. Thus, the responses are normalized according to EquationEq. (2)(2)
(2) based on the ‘lower the better criteria’ defined by Taguchi. Further, the deviation sequence is calculated. Then, EquationEq. (3)
(3)
(3) is used to calculate the GRC. Using GRC, the GRG values are calculated using EquationEq. (4)
(4)
(4) . The maximum value of GRG indicates favorable performance. The calculation of GRG addresses the conversion of MOO into a single-objective optimization problem. Thus, single-objective optimization problem can be effectively solved using the Taguchi method. provides the values of GRA.
Table 7. GRA table.
A maximum GRG of 86.7% is obtained for experimental run 1, and it corresponds to a combination of CP (cutting speed, feed rate and depth of cut) of 175 m/min, 0.15 mm/rev and 0.6 mm, respectively. As Inconel 800 is difficult to cut material, at optimal condition, it gives better results in terms of lower SR and TW. The increase in the levels of CP will enhance the thermal load on the cutting environment and results the plastic deformation of tool material. Thus, tool will undergo wear at faster rate. As the thermal load rises, the work material tends to soften, allowing loosely flowing particles to adhere to the machined surface and potentially degrade its quality. Based on the GRG value, a rank is given to each experiment. The means of GRG for different experimental trials have been calculated using Minitab and are presented in . presents the mean effect plot and S/N ratio for GRG. and indicate the optimal parameters cutting speed, feed rate and depth of cut all at the first levels.
Table 8. Response table for GRG.
Further, ANONA is performed on GRG in Minitab, and results are displayed in . From ANOVA, cutting speed, feed rate and depth of cut are found to be prominent on the DRT of Inconel 800 with a % contribution of 33.3, 13.8 and 23.7, respectively.
Table 9. ANOVA table for GRG.
6. Conclusion
HSM of Inconel 800 superalloy from the Inconel family was carried out under DRT conditions. Using Taguchi’s design, experiments were conducted employing an uncoated carbide insert. The SEM images of cutting inserts were analyzed to evaluate the TW. The effect of CP (cutting speed, feed rate and depth of cut) on the outputs SR and FW were studied using S/N ratio, ANOVA and MOO technique, i.e. GRA. The findings are summarized as follows:
The adhesion, abrasion and diffusion were observed as primary TW mechanisms.
The chipping, abrasion marks, BUE formation, notch formation and crater formation were observed as some of the common TW patterns.
An increase in CP, increased the SR. However, the feed rate was noted as a prominent factor with a contribution of 33.9%.
From ANOVA, cutting speed, depth of cut and cutting speed × depth of cut were observed as prominent factors on FW with percentage contribution of 54.8, 27.8 and 8.99, respectively. The feed rate showed a neutral effect on FW.
From GRA, all three CP, i.e. cutting speed, feed rate and depth of cut, were noted as prominent factors with a percentage contribution of 33.3, 13.8 and 23.7 on the overall performance of DRT on Inconel 800. The optimal CP (cutting speed, depth of cut and feed rate) was noted as 175 m/min, 0.6 mm and 0.15 mm/rev, respectively.
Thus, optimal parameters could be effectively utilized by industries in HSM of Inconel 800, which may reduce the cost and time considerably.
This study focuses only on DRT on Inconel 800. However, a study on the use of different cooling techniques during the machining of the Inconel 800 is also essential. This may be considered as a scope for future work.
The study on modeling and other optimization techniques in HSM of Inconel 800 can be considered as a scope for future work. Also, the measurement of cutting force, temperature and residual stresses during the machining process need to be given due importance, which may be considered in further studies.
Abbreviations | ||
ANOVA | = | Analysis of variance |
BUE | = | Built-up-edge |
CANDU | = | Canada Deuterium Uranium |
CNC | = | Computer numerical control |
CP | = | Cutting parameters |
DRT | = | Dry turning |
FW | = | Flank wear |
GRA | = | Grey relational analysis |
GRC | = | GRA-coefficient |
GRG | = | GRA-based grades |
HSM | = | High-speed machining |
MOO | = | Multi-objective optimization |
MRR | = | Metal removal rate |
RSM | = | Response surface methodology |
S/N ratio | = | Signal-to-noise ratio |
SR | = | Surface roughness |
TW | = | Tool wear |
Disclosure statement
No potential conflict of interest was reported by the authors.
Additional information
Notes on contributors
Ganesha Prasad
Ganesha Prasad serves as a Research Scholar in the Department of Mechanical and Industrial Engineering at MIT Manipal, Karnataka. He holds a B.E. in Mechanical Engineering and an M. Tech. in Machine Design, both from NMAM Institute of Technology Nitte, Karnataka. With three and a half years of teaching experience, he has contributed significantly to the academic field. Ganesha Prasad has an impressive record of publications in reputable journals and holds a patent. His research interests span machining science, dynamics of machine structures, machine learning, image processing, and deep learning.
G. S. Vijay
Dr. VIJAY G. S. is a Professor with the Department of Mechanical and Industrial Engineering, Manipal Institute of Technology, Manipal Academy of Higher Education, Manipal, India. His research interests include bearing diagnostics, application of soft computing techniques to engineering and non-engineering domains, machine learning, applied numerical methods, meta-heuristic based multi-objective optimization, mechanical vibrations, finite element analysis, material science and metallurgy.
Raghavendra C. Kamath
Dr. Raghavendra Kamath C. is a professor in the department of Mechanical and Industrial Engineering, MIT Manipal, India. He has more than 20 years of teaching and research experience. He has published a patent on machine vision. He also co-authored a book titled “Data Analysis Theoretical concepts for non-IT engineers”. He also has received a grant for research proposal titled “Cryogenic machining of elastomers” in 2019. He has presented and published more than 50 papers in various national and international journals and conferences. His research areas include machine/deep learning, image processing, non-conventional machining, cryogenic machining, difficult to machine materials, composite materials, optimization techniques, Operations research, Simulation modeling and analysis, Modeling of machining processes.
Harshit Jairaj Hemmady
Harshit Jairaj Hemmady is an undergraduate student in thedepartment of Mechanical and Industrial Engineering, MIT Manipal, India. He was part of the present study.
References
- Abas, M., Alkahtani, M., Khalid, Q. S., Hussain, G., Abidi, M. H., & Buhl, J. (2022). Parametric study and optimization of end-milling operation of AISI 1522H steel using definitive screening design and multi-criteria decision-making approach. MDPI, 15(2022), 1–23.
- Abas, M., Sayd, L., Akhtar, R., Khalid, Q. S., Khan, A. M., & Pruncu, C. I. (2020). Optimization of machining parameters of aluminum alloy 6026-T9 under MQL-assisted turning process. Journal of Materials Research and Technology. 9(5), 10916–10940. https://doi.org/10.1016/j.jmrt.2020.07.071
- Ahmad, J., Purbolaksono, J., & Beng, L. C. (2010). Failure analysis on high temperature superheater Inconel® 800 tube. Engineering Failure Analysis, 17(1), 328–333. https://doi.org/10.1016/j.engfailanal.2009.06.013
- Akhtar, W., Sun, J., Sun, P., Chen, W., & Saleem, Z. (2014). Tool wear mechanisms in the machining of Nickel based super-alloys: A review. Frontiers of Mechanical Engineering, 9(2), 106–119. https://doi.org/10.1007/s11465-014-0301-2
- Alemayoh, G. H., Singh, B., & Tesfamariam, B. B. (2023). Experimental and numerical investigation of dry turning AISI 1030 carbon steel using CNC lathe machining. Engineering Research Express, 5(1), 015007. https://doi.org/10.1088/2631-8695/acb11e
- Bagaber, S. A., & Yusoff, A. R. (2017). Multi-objective optimization of cutting parameters to minimize power consumption in dry turning of stainless steel 316. Journal of Cleaner Production, 157, 30–46. https://doi.org/10.1016/j.jclepro.2017.03.231
- Eklarkar, S. V., & Nandedkar, V. M. (2021). FE simulation and optimization of drawbead parameters using Taguchi method for hemispherical cup, in. Materials Today: Proceedings, 44, 4709–4716. https://doi.org/10.1016/j.matpr.2020.10.941
- Eskandari, B., Bhowmick, S., & Alpas, A. T. (2022). Turning of Inconel 718 using liquid nitrogen : Multi‑objective optimization of cutting parameters using RSM. The International Journal of Advanced Manufacturing Technology, 120(5–6), 3077–3101. https://doi.org/10.1007/s00170-022-08906-w
- Francis Xavier, J., Ravi, B., Jayabalakrishnan, D., Ezilarasan, C., Jayaseelan, V., & Elias, G. (2021). Experimental study on surface roughness and flank wear in turning of Nimonic C263 under dry cutting conditions. Journal of Nanomaterials. 2021, 1–11. https://doi.org/10.1155/2021/2054399
- Frifita, W., Ben Salem, S., Haddad, A., & Yallese, M. A. (2020). Optimization of machining parameters in turning of Inconel 718 nickel-base super alloy. Mechanics & Industry, 21(2), 203. https://doi.org/10.1051/meca/2020001
- Gimenez, C., Sierra, V., & Rodon, J. (2012). Sustainable operations: Their impact on the triple bottom line. International Journal of Production Economics, 140(1), 149–159. https://doi.org/10.1016/j.ijpe.2012.01.035
- Griffiths, M. (2019). Ni-based alloys for reactor internals and steam generator applications. Elsevier Inc.
- Grigoriev, S., Vereschaka, A., Uglov, V., Milovich, F., Cherenda, N., Andreev, N., Migranov, M., & Seleznev, A. (2023). Influence of tribological properties of Zr-ZrN-(Zr,Cr,Al)N and Zr-ZrN-(Zr,Mo,Al)N multilayer nanostructured coatings on the cutting properties of coated tools during dry turning of Inconel 718 alloy. Wear, 512–513, 204521. https://doi.org/10.1016/j.wear.2022.204521
- Gupta, M. K. (2018). Machinability investigations of Inconel-800 super alloy under sustainable cooling conditions. MDPI 11(11), 1–13. Article ID: 2088.
- Gupta, M. K., Song, Q., Liu, Z., Sarikaya, M., Jamil, M., Mia, M., Singla, A. K., Khan, A. M., Khanna, N., & Pimenov, D. Y. (2021). Environment and economic burden of sustainable cooling/lubrication methods in machining of Inconel-800. Journal of Cleaner Production, 287, 125074. https://doi.org/10.1016/j.jclepro.2020.125074
- Jovicic, G., Milosevic, A., Kanovic, Z., Sokac, M., Simunovic, G., Savkovic, B., & Vukelic, D. (2023). Optimization of dry turning of Inconel 601 alloy based on surface roughness, tool wear, and material removal rate. Metals (Basel), 13(6), 1068. https://doi.org/10.3390/met13061068
- Ju-Long, D. (1982). Control problems of grey systems. System & Control Letters. 1(5), 288–294. https://doi.org/10.1016/S0167-6911(82)80025-X
- Mou, W., & Zhu, S. (2020). Vibration, tool wear and surface roughness characteristics in turning of Inconel 718 alloy with ceramic insert under LN2 machining. Journal of the Brazilian Society of Mechanical Sciences and Engineering, 42(7), 1–12. https://doi.org/10.1007/s40430-020-02438-8
- Patel, U., Rawal, S., Bose, B., Arif, A. F. M., & Veldhuis, S. (2022). Performance evaluations of Ti-based PVD coatings deposited on cermet tools for high-speed dry finish turning of AISI 304 stainless steel. Wear, 492–493, 204214. https://doi.org/10.1016/j.wear.2021.204214
- Paul, T. R., Saha, A., Majumder, H., Dey, V., & Dutta, P. (2019). Multi-objective optimization of some correlated process parameters in EDM of Inconel 800 using a hybrid approach. Journal of the Brazilian Society of Mechanical Sciences and Engineering, 41(7), 1–11. https://doi.org/10.1007/s40430-019-1805-9
- Podder, B., & Paul, S. (2012). Improvement of machinability in end milling of Nimonic C-263 by application of high pressure coolant. International Journal of Machining and Machinability of Materials, 11(4), 418–433. https://doi.org/10.1504/IJMMM.2012.047837
- Qazi, M. I., Abas, M., Khan, R., Saleem, W., Pruncu, C. I., & Omair, M. (2021). Experimental investigation and multi-response optimization of machinability of AA5005H34 using composite desirability coupled with PCA. Metals, 11(2), 235. https://doi.org/10.3390/met11020235
- Ramakrishna, M. V. A., & Srinivas, K. (2021). Grey relational analysis of friction stir welding parameters for the development of dissimilar joints between AA6082 and AA7075. Advances in Materials and Processing Technologies, 8(sup2), 954–965. https://doi.org/10.1080/2374068X.2021.1959112
- Roy, S., Kumar, R., Sahoo, A. K., & Das, R. K. (2019). A brief review on effects of conventional and nano particle based machining fluid on machining performance of minimum quantity lubrication machining. Materials Today: Proceedings, 18, 5421–5431. https://doi.org/10.1016/j.matpr.2019.07.571
- Sahoo, A. K., Orra, K., & Routra, B. C. (2013). Application of response surface methodology on investigating flank wear in machining hardened steel using PVD TiN coated mixed ceramic insert. International Journal of Industrial Engineering Computations, 4(4), 469–478. https://doi.org/10.5267/j.ijiec.2013.07.001
- Sarıkaya, M., & Güllü, A. (2014). Taguchi design and response surface methodology based analysis of machining parameters in CNC turning under MQL. Journal of Cleaner Production, 65, 604–616. https://doi.org/10.1016/j.jclepro.2013.08.040
- Sarma, J., Kumar, R., Sahoo, A. K., & Panda, A. (2020). Enhancement of material properties of titanium alloys through heat treatment process: A brief review. Materials Today: Proceedings, 23(Part 3), 561–564. https://doi.org/10.1016/j.matpr.2019.05.409
- Senthil Kumar, S., Sudeshkumar, M. P., Ezilarasan, C., Palani, S., & Veerasundaram, J. (2021). Modelling and simulation of machining attributes in dry turning of aircraft materials Nimonic C263 using CBN. Manufacturing Review, 8, 30. https://doi.org/10.1051/mfreview/2021030
- Sivaiah, P., & Chakradhar, D. (2018). Multi performance characteristics optimization in cryogenic turning of 17-4 PH stainless steel using Taguchi coupled grey relational analysis. Advances in Materials and Processing Technologies, 4(3), 431–447. https://doi.org/10.1080/2374068X.2018.1452132
- Tan, D. W., Guo, W. M., Wang, H. J., Lin, H. T., & Wang, C. Y. (2018). Cutting performance and wear mechanism of TiB2-B4C ceramic cutting tools in high speed turning of Ti6Al4V alloy. Ceramics International, 44(13), 15495–15502. https://doi.org/10.1016/j.ceramint.2018.05.209
- Thakur, A., Gangopadhyay, S., Mohanty, A. M., & Maity, K. (2016). Experimental assessment on performance of TiN/TiCN/Al2 O3/ZrCN coated tool during dry machining of Nimonic C-263. International Journal of Machining and Machinability of Materials, 18(5/6), 452–465. https://doi.org/10.1504/IJMMM.2016.078985
- Tian, X., Yan, K., Wang, Z., Xie, F., Liu, Y., Wang, L., Chen, X., Yuan, J., & Liu, H. (2021). Performance of carbide tools in high-speed dry turning iron-based superalloys. Proceedings of the Institution of Mechanical Engineers Part B: Journal of Engineering Manufacture, 236(4), 427–439. https://doi.org/10.1177/09544054211028515
- Tu, L., Deng, Y., Zheng, T., Han, L., An, Q., Ming, W., & Chen, M. (2022). Wear and friction analysis of cubic boron nitride tools with different binders in high-speed turning of nickel-based superalloys. Tribology International. 173, 107659. https://doi.org/10.1016/j.triboint.2022.107659
- Tu, L., Lin, L., Liu, C., Zheng, T., Deng, Y., Han, L., An, Q., Ming, W., & Chen, M. (2023). Tool wear characteristics analysis of cBN cutting tools in high-speed turning of Inconel 718. Ceramics International. 49(1), 635–658. https://doi.org/10.1016/j.ceramint.2022.09.034
- Tu, L., Ming, W., Xu, X., Cai, C., Chen, J., An, Q., Xu, J., & Chen, M. (2022). Wear and failure mechanisms of SiAlON ceramic tools during high-speed turning of nickel-based superalloys. Wear, 488–489, 204171. https://doi.org/10.1016/j.wear.2021.204171
- Ulutan, D., & Ozel, T. (2011). Machining induced surface integrity in titanium and nickel alloys: A review. International Journal of Machine Tools and Manufacture, 51(3), 250–280. https://doi.org/10.1016/j.ijmachtools.2010.11.003
- Vukelic, D., Simunovic, K., Simunovic, G., Saric, T., Kanovic, Z., Budak, I., & Agarski, B. (2020). Evaluation of an environment-friendly turning process of Inconel 601 in dry conditions. Journal of Cleaner Production, 266, 121919. https://doi.org/10.1016/j.jclepro.2020.121919
- Wagri, N. K., Jain, N. K., Petare, A., Das, S. R., Tharwan, M. Y., Alansari, A., Alqahtani, B., Fattouh, M., & Elsheikh, A. (2023). Investigation on the performance of coated carbide tool during dry turning of AISI 4340 alloy steel. Materials (Basel, Switzerland), 16(2), 668. https://doi.org/10.3390/ma16020668
- Xue, C., Wang, D., & Zhang, J. (2022). Wear mechanisms and notch formation of whisker-reinforced alumina and sialon ceramic tools during high-speed turning of Inconel 718. Materials (Basel, Switzerland), 15(11), 3860. https://doi.org/10.3390/ma15113860
- Yelamasetti, B., Venkat Ramana, G., Manikyam, S., & Saxena, K. K. (2021). Multi-response Taguchi grey relational analysis of mechanical properties and weld bead dimensions of dissimilar joint of AA6082 and AA7075. Advances in Materials and Processing Technologies, 8(sup3), 1474–1484. https://doi.org/10.1080/2374068X.2021.1946340
- Yin, Q., Liu, Z., Wang, B., Song, Q., & Cai, Y. (2020) Recent progress of machinability and surface integrity for mechanical machining Inconel 718: A review. The International Journal of Advanced Manufacturing Technology, 109(1–2), 215–245. https://doi.org/10.1007/s00170-020-05665-4
- Zhou, J., Chen, Z., Persson, H., Peng, R. L., Saoubi, R. M., & Gustasson, D. (2019). Comparative assessment of the surface integrity of AD730 ® and IN718 superalloys in high-speed turning with a CBN tool. Journal of Manufacturing and Materials Processing, 3(3), 73. https://doi.org/10.3390/jmmp3030073