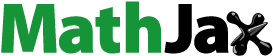
Abstract
This research develops an optimization model of supplier selection and order allocation with transportation mode alternatives under carbon cap and trade policy. The objective function of the model is to maximize the manufacturer’s total profit. Total revenue comes from the selling of both good items and imperfect items. Total cost in this research consists of purchase cost, transportation costs from supplier to manufacturer and from manufacturer to retailer, transaction cost, holding cost, inspection cost and carbon emission cost. Transaction cost is applied for each selected supplier in each period without considering the type and quantity of the item. This research considers a supply chain with multiproducts, multiperiods, multisuppliers and multiretailers. We assumed that the manufacturer inspects all the products come from the suppliers and some of the products are defective. Those defect items are sold in a lower price at a secondary market. This research has a contribution in the inclusions of carbon emission and defect product in the model. Hence, the model is in line with the sustainability issue and closer to the real condition in terms of imperfect manufacturing. A mixed integer linier programming (MILP) model is developed in this research, and the model was solved using Lingo 15.0 software with branch and bound method. The result of sensitivity analysis shows that the objective function is sensitive to the changes of demand, selling price of good item, transportation cost, purchasing cost and holding cost. While the decision variables of order allocation and the number of transportation mode are sensitive to the changes of demand, transportation cost and purchasing cost.
1. Introduction
Supply Chain Management (SCM) has been recognized as an effective tool and strategy to improve company’s business performance. According to a research by Parilla (Citation2020), from 258 companies under investigations, more than 70% of them got 26%–75% profit margin by adopting effective SCM. Another study by Lee (Citation2021) revealed that SCM strategies and organizational competencies has a significant effect on the operational and financial performance of the companies under research. With the pressures of globalization and intense competition, manufacturing companies have to make continuous improvements to increase their competitive advantage over their competitors. In addition to policies regarding global sourcing, supplier selection and order allocation (SSOA) are very important and complex decisions (Basa et al., Citation2018). Hence, it gets a great attention in various business and management literature (Rezaei & Davoodi, Citation2007). According to Ebrahim et al. (Citation2009), the purpose of supplier selection is to determine the best combination of suppliers that can provide the best goods or services for the company and be part of important decisions in the company’s supply chain.
An effective supplier selection strategy will directly impact supply chain performance in terms of productivity and profitability. A study by Ekiyor et al. (Citation2019) showed that supplier selection criteria had a positive effect on company profitability of about 49%. While in the study of Van der and Ntshingila (Citation2020), supplier selection and development had a positive impact on company business performance. There are two types of supplier selection problems, namely single sourcing and multisourcing. In single sourcing, the supplier can meet all the demands of the manufacturer, whereas in the case of multisourcing, no single supplier can fulfill the demand as required by the manufacturer. In this case, the manufacturer has to make decisions in supplier selection and the respective order allocation (Ghodsypour & O’Brien, Citation1998).
Many researches have been conducted in the field of SSOA. Commonly, due to the nature of the problems, supplier selection involved multicriteria decision making using various multicriteria decision techniques. While the order allocation decision is solved using both linear and nonlinear mathematical programming. Environmental impact and disruption risks have become the major concern in SSOA and become the important aspects in supply chain decision makings. In terms of environmental impact, one of the quantitative measures commonly used in supply chain is the carbon emissions. According to the data from International Energy Agency, transportation sector consumed of about 29% of energy and shared about 25% of the total carbon emissions and 20% of global gas house emission (Jiang et al., Citation2024; Wang et al., Citation2023). Hence, both transportation cost and its impact on environment should be considered in SSOA.
SSOA has been recognized as complex and challenging task. Despite of many models have been developed in the area of SSOA, few attempts have been made to provide a mathematical model to address the existence of alternative carriers in raw materials and finished goods delivery as well as product defects under carbon cap and trade policy. The rest of the article is structured as follows. Section 2 provides a review of the related literature. Section 3 provides the system description. Section 4 focuses on model development and detail explanation of the objective function and constraints of the model. Section 5 provides the numerical example to show the model application. Section 6 provides the results and discussion including the sensitivity analysis of the model. Section 7 concludes the research and gives some directions for further research.
2. Literature review
There is many research that have been conducted to solve SSOA problems. Basnet and Leung (Citation2005) developed a supplier selection model and determined the optimal lot size to meet the demand of raw materials using a basic model developed by Wagner and Whitin (Citation1958). Benson (Citation2005) proposed supplier capacity limit by considering multi-supplier supply chain to determine the optimal procurement strategy. Mohammed et al. (Citation2018) developed a model to solve SSOA problems in two separate stages. In the first stage, supplier selection was solved using Fuzzy Analytic Hierarchy Process (FAHP) and Fuzzy TOPSIS (FTOPSIS). In the second stage, order allocation problem was solved using multiobjective optimization involving several objective functions in terms of cost, carbon emission and total value purchasing. The final solution was obtained using TOPSIS based on the results of nondominated solution of the model. Gören (Citation2018) developed a sustainable SSOA model. He employed Fuzzy Decision-Making Trial and Evaluation Laboratory (FDEMATEL) was employed to determine the weight of the sustainable criteria. The weight was then used as the input for the Taguchi loss function to determine the rank of each supplier. Finally, the results became the input for bi-objective integer linear programming optimization model to determine the optimal order allocation. Mohammed et al. (Citation2019) also employed FAHP and FTOPSIS to determine the criteria weight of the suppliers and the order allocation problem was solved using constraints approach.
Environmental impact and disruption risks have become the major concerned and the important aspects in supply chain decision makings. Hence, some researchers developed models to determine the environmental impact of order allocation decisions and the impact of disruptive events on supply chain decisions. In terms of environmental impact, one of the quantitative measures commonly used in supply chain is the carbon emissions. Lamba and Singh (Citation2019) and Lamba et al. (Citation2019) developed models to study several policies regarding the implementation of carbon emission costs. Three policies were studied, namely cap and trade, strict cap and carbon tax policies. Feng and Gong (Citation2020) proposed linguistic entropy weight method to select several qualified green suppliers and employed multiobjective programming to determine the order allocation. The model considered multiproduct and multiperiod allocation problem to simultaneously minimize several objectives consisting costs, energy consumption and carbon emission. In terms of disruption, Hosseini et al. (Citation2019) developed an SSOA model considering the supplier resiliencies. The model simultaneously considered additional supplier capacity, supplier reliability, back-up suppliers and geographic segregation. Moreover, Kaur and Singh (Citation2021) proposed a model considering not only traditional disruption risks, but also disruptive technology.
According to Chopra and Meindl (Citation2007), transportation becomes one of the important aspects in supply chain. Hence, transportation cost and its impact on environment should be considered in the SSOA. In many studies, the transportation cost has been included in the total cost of supply chain (Mendoza & Ventura, Citation2009). The inclusion of transportation cost in the SSOA will further improve supply chain efficiency. Blumenfeld et al. (Citation1985) discussed the need to analyze the relationship between supply chain and transportation cost. The objective of the model is to minimize the total inventory and routing costs simultaneously in determining the optimal route and shipping size of the vehicles used as the carriers. The simultaneous determination of supplier selection, order allocation and carrier selection can help to avoid the suboptimal solutions that might result from completing three separate decisions. Dullaert et al. (Citation2005) considered a combinatorial optimization method to determine the optimal outcome of a mixed alternative modes of transportation to minimize the total logistics costs when goods are shipped from suppliers to customers. The total logistics costs included ordering costs, transportation costs and inventory costs. Pazhani et al. (Citation2015) developed a model for the selection of suppliers and supplies at multiple levels in the supply chain by considering purchasing costs, setup costs, storage costs and transportation costs. Chan et al. (Citation2015) developed a bi-objective model for three echelon supply chains by taking into consideration the decision of carrier selection. Further, Wicaksono et al. (Citation2019) considered dynamic supplier in their model to allow for the raw materials to be provided by different supplier at each period.
Filcek and Józefczyk (Citation2012) considered a model consisting of suppliers, production and retailers to determine optimal raw material allocation and transportation. Basa et al. (Citation2018) developed a multisource problem model for one item involving lot sizing, order allocation and carrier selection decisions by considering quantity discounts. The model involved storage costs, ordering costs, transportation costs and goods prices with several constraints including waiting time, supplier capacity and truck capacity. Hu et al. (Citation2018) developed an integrated model of SSOA to determine optimal supplier’s configuration and its order allocation, as well as selecting the appropriate logistics service providers. Yaghin and Darvishi (Citation2020) developed an order allocation and transportation planning model under uncertainty in apparel industry environment. The model considered multisources, multiproducts and multicarriers with price discount to minimize total logistic cost, late delivery and value purchasing. Heinold and Meisel (Citation2020) developed an intermodal freight transportation planning model considering emission limits and allocation. Two transportation modes were considered, namely road and railway transportation services. A bi-objective optimization model was developed by Sobhanolahi et al. (Citation2020) to solve SSOA problem. The model involved single product sold with discounts considering transportation cost and solved using nondominated sorting genetic algorithm (NSGA II). Similar to Sobhanolahi et al. (Citation2020), the research of Abrishami et al. (Citation2020) developed a model to solve SSOA problem for multi raw materials sold with discount to minimize total cost including transportation cost. Using the same objective function to minimize total cost, the research of Ghorbani and Ramezanian (Citation2020) included carrier selection and transportation cost to solve SSOA in humanitarian logistics.
In a production environment, it is often found that not all products are of good quality. Some of them are defective products which cannot be sold directly to consumers. They have to be repaired or reworked according to the conditions of the products. A considerable cost should be expensed by the company to withdraw the products which has reached the consumer. Hence, it is more appropriate to consider quality-related costs in determining the optimal ordering policy. Rezaei and Davoodi (Citation2007) developed a supplier selection model taking into account the imperfect quality of goods and storage capacity. Salameh and Jaber (Citation2000) argued that products that are not perfect or below the specified quality standards are not always a defective product. However, it can be used in certain conditions with a lower level of quality standards. A total inspection can be carried out to determine the good quality items and the imperfect ones.
shows the summary of literature review and the relative position of this research. From the table, this article contributes to the inclusions of defect, transportation mode selection and its corresponding cost under carbon cap and trade policy. Hence, an SSOA model is developed for a three echelon supply chain environment consisting of suppliers, manufacturers and retailers. The model considers the existing of several choices of suppliers with one manufacturer to meet the demands of several retailers. The manufacturer will order a certain number of each type of raw materials from suppliers who produce the materials. The manufacturer then transforms the raw materials into final products to meet the retailers’ demand. The model involves transportation modes selection to deliver raw materials from suppliers to the manufacturer and from manufacturer to retailers under carbon cap and trade policy. A mixed integer linear programming (MILP) model is proposed in this research to solve the problem.
Table 1. Literature review.
3. System description
We consider a supply chain consisting of multisupplier, single manufacturer and multiretailer. The supply chain planning involves multiproducts, multiperiods and multitransportation modes. A certain number of suppliers is available to supply the required products. Each supplier may provide more than one product, and each product may be provided by more than one supplier. Hence, the manufacturer has to select a subset of suppliers from the set of available suppliers. After selecting the suppliers, the manufacturer has to determine the optimal order allocation of each product to the selected suppliers. Afterward, the manufacturer will make the inspections for the incoming products. The good products will be sold in normal price, while the defect products will be sold in a lower price at a secondary market. Finally, the final products are then used to fulfill the demand of retailers. Several carriers are available to deliver the products from the suppliers to manufacturer and from manufacturer to the retailers. depicts the description of the general system, while shows the more detail description of the system.
The manufacturer needs to maximize the total profit which is obtained by subtracting the total revenues from the total cost. The total costs considered by the manufacturer consist of the cost of purchasing the product, the cost of transportation from supplier to manufacturer and from manufacturer to retailer, transaction costs, storage costs, costs of quality inspection and carbon emission costs. The carbon emission costs come from the activities of transportations, product orders and storage.
4. Model development
4.1. Assumptions
The following assumptions are used in the development of the model:
Transaction cost is independent of the types and quantities of the products.
Demands of product i in period t for all of retailer (DR) are known over a planning horizon.
Shortage and backorder are not allowed.
4.2. Notations of the model
4.2.1. Index
I: index for product (i = 1, 2, …, I)
j: index for supplier (j = 1, 2, …, J)
p: index for retailer (p = 1, 2, …, P)
r: index for carrier (r = 1, 2, …, R)
t: index for time period (t = 1, 2, …, T)
4.2.2. Decision variables
Xi,j,t,r: order quantity for product i from supplier j in period t with carrier r.
Invi,t: inventory of product i in period t.
Numj,t,r: the number of carrier r to deliver from supplier j in period t.
Numwrp,t: the number carrier to deliver to retailer p in period t.
4.2.3. Parameters
Sgi: unit selling price of good quality of product i
Sdi : unit selling price of defect product i
Hi: unit holding cost of product i
Vi: unit inspection cost of product i
Oj: unit transaction cost of supplier j
TCwrp: unit transportation cost from manufacture to retailer p
Eot: unit carbon emission of placing an order in period t
Eht: unit carbon emission of holding a unit product in inventory at period t
Evt: unit variable carbon emission in each order at period t
Ccr: unit capacity of carrier r
maxnumr: available units of carrier r
PRij: average percentage of defective product i from supplier j
UPij: unit price of product i from supplier j
SCij: capacity of product i at supplier j
Dit: demand accumulation of product i at period t for all retailer
TCjr: unit transportation cost of carrier r from supplier j
DRipt: demand for product i from retailer p at period t
W: manufacturer storage capacity
C: carbon cap during the planning horizon
4.3. Objective functions and constraints of the model
With the above parameters and decision variables, the MILP model is expressed as follows:
(1)
(1)
where
(1a)
(1a)
(1b)
(1b)
(1c)
(1c)
(1d)
(1d)
(1e)
(1e)
(1f)
(1f)
(1g)
(1g)
(1h)
(1h)
(1i)
(1i)
EquationEquation (1)(1)
(1) is the objective function of the MILP model with the aim of maximizing the total profit which consists of nine parts. EquationEquations (1a)
(1a)
(1a) and Equation(1b)
(1b)
(1b) express the revenue comes from selling good quality products and defect products, respectively. EquationEquation (1c)
(1c)
(1c) is the formulation of purchase cost, EquationEquations (1d)
(1d)
(1d) and Equation(1e)
(1e)
(1e) show, respectively, the cost of transportation from suppliers to the manufacturer and from the manufacturer to retailers. EquationEquations (1f)–(1i) express, respectively, the transaction cost, holding cost, inspection cost and carbon emissions cost.
The following constraints are imposed to the model:
Demand fulfillment: Because shortage is not allowed in this model, the number of orders for each type of product to suppliers for each period cannot be less than the number of each type of product ordered by the retailer for each period.
(2)
(2)
There is a transaction cost for each selected supplier. This transaction cost does not consider the number of products ordered or the type of product ordered at the supplier.
(3)
(3)
Storage capacity: Because every product of imperfect quality is assumed to always be bought from outside the system, the only products stored in the warehouse are of good quality.
(4)
(4)
Capacity of transportation mode from supplier to manufacture. The number of all products ordered does not exceed the total capacity of each type of transportation multiplied by the number of units available.
(5)
(5)
Capacity of transportation mode from manufacturer to retailer. The number of demands received does not exceed the total transportation capacity multiplied by the number of units available.
(6)
(6)
Supplier capacity: The number of products ordered to suppliers for each type does not exceed the supplier’s capacity to produce that type of product.
(7)
(7)
Carbon emission constraint: The amount of carbon emitted plus the amount of carbon sold must equal the allowable carbon limit plus the amount of carbon purchased.
(8)
(8)
Demand accumulation for each product in t period for all retailer.
(9)
(9)
The number of transportation mode that used to deliver from supplier to manufacture.
(10)
(10)
The number of transportation mode that used to deliver from manufacture to retailer.
(11)
(11)
Non-negative and integer constraints
(12)
(12)
Binary variables constraint
(13)
(13)
5. Numerical example
In this section, we present a numerical example with the purpose to show how the proposed model works. We consider three products, four suppliers, four retailers and three carriers alternative over six periods of planning horizon. The input parameter was generated randomly and set in such a way to satisfy all the constraints of the model. shows the demand from the retailers in six consecutive periods. shows the parameters for each supplier in terms of unit price, transaction cost, capacity and defect percentage of each product at each supplier. shows the carrier parameters to deliver the products from supplier to the manufacturer and the maximum number and capacity of each carrier. The transportation cost from manufacturer to each retailer is assumed to be $4000, $4200, $4900 and $4550, respectively.
Table 2. Retailer demand.
Table 3. Parameters of the suppliers.
Table 4. Carrier parameters.
The selling price of each product both good quality and defect product, unit holding cost and unit inspection cost are shown in . shows the carbon emission parameters in each period. The carbon emissions come from placing an order, holding the inventory and transportation are assumed to be the same in each period with the values of 100, 3 and 0.1, respectively. The carbon emission cap is set at 35,850.
Table 5. Price and cost parameters for each final product.
Table 6. The results of optimization.
6. Results and discussion
LINGO 15.0 software with the embedded branch and bound algorithm was selected to solve the model. Under a standard computer specification with Intel Core i3 and 4 GB of RAM, the optimal results were found after 41,127 iterations in 18.68 s. shows the optimal results of the model. The table shows the selected supplier for each product, along with the order allocation and the selected carrier at each period. For example, in period 1, product 1 is supplied by supplier 1 and supplier 4 using carriers 1 and 2. For product 2, orders in period 1 are supplied by all suppliers with different transportation modes. With this optimal decision, the manufacturer get the total profit of $181,305. The entries in shows the inventory of good quality product over six planning periods. From the table, we conclude that there are no shortages for each product demand in each period and no inventory exists in the last period.
Table 7. Inventory of good quality product.
Sensitivity analysis is performed to determine how the model responds to the changes of the input parameters. The sensitivity analysis scenario conducted in this research involves of the changes in demand (Dit), the selling price of good and defect products (Sgi and Sdi), transportation costs (TCjr), product purchase prices (UPij), holding costs (Hi) and transaction costs (Oj). shows the results of sensitivity analysis. The table shows the changes in the objective function when the parameters change according to the developed scenarios. From the table, we can see that the most sensitive parameter is the price of good quality product, followed by unit purchasing price, demand, unit holding cost and unit transportation cost. While the selling price of defect product and transaction cost are not sensitive to the profit of the manufacturer.
Table 8. The results of sensitivity analysis.
Although the model was solved in short computational time, the solving duration will be much more longer when the problem becomes more complex and larger. The addition of products, periods, suppliers and carrier alternatives will significantly increase the computational time. Chen and Tan (Citation2023) divide the optimization problems into two categories, namely Type S and Type R. Type R problems were considered to be more complex as the decision variables have strong dependencies, while for Type S problem, the decision variables were fully independent. The implementation of metaheuristic algorithms will increase the computational efficiency. Many metaheuristic algorithms have been proposed in the literature to solve large and complex problems through single metaheuristic algorithm or hybridization. Several metaheuristic algorithms have been used to solve large and complex problems such as nondominated sorting genetic algorithm, simulated annealing, tabu search and variable neighborhood search. Several new metaheuristic algorithms have been proposed such as self-adaptive fast fireworks algorithm, adaptive polypoid memetic algorithm (Chen & Tan, Citation2023; Dulebenets, Citation2021, Citation2023). Based on the research of Pasha et al. (Citation2022), the hybrid version of those metaheuristic algorithms consistently outperformed their nonhybridized versions. Hence, for future research, it is interesting to determine the performance of the hybrid version of the above algorithms to solve the larger and more complex version of the developed model in this research.
7. Conclusions
In this research, we developed a MILP model for SSOA with carrier selection by considering defect product, carbon emissions and the capacity of each supplier to maximize total profits. The results of the sensitivity analysis show that the objective function is sensitive to the changes of demand parameters, good product selling prices, transportation costs, product purchase prices and storage costs. Meanwhile, the changes in the selling price of defect products and transaction costs have no significant effect on the profit. For further research, a more complex model can be developed by adding the considerations of common and noncommon parts, shortage, unreliable delivery performance or lead time delivery. Another further research can be directed to solve the more complex and larger model using some hybrid metaheuristic algorithms.
Disclosure statement
No potential conflict of interest was reported by the authors.
References
- Abrishami, S. J., Vahdani, H., & Rezaee, B. (2020). An integrated lot-sizing model with supplier and carrier selection and quantity discount considering multiple products. Scientia Iranica, 27(4), 2140–2156.
- Basa, G., Becker, T., & Kedir, A. (2018). Supplier Selection and Order Allocation with Intermodal Transportation Cost. In M. Freitag, H. Kotzab, & J. Pannek (Eds.), Dynamics in Logistics. LDIC 2018. Lecture Notes in Logistics. Springer, Cham. https://doi.org/10.1007/978-3-319-74225-0_15.
- Basnet, C., & Leung, J. (2005). Inventory lot-sizing with supplier selection. Computers & Operations Research, 32(1), 1–14. https://doi.org/10.1016/S0305-0548(03)00199-0
- Benson, H. (2005). Optimal pricing and procurement strategies in a supply chain with multiple capacitated supplier model. Computers and Operations Research, 32, 1–14.
- Blumenfeld, D. E., Burns, L. D., Diltz, J., & Daganzo, C. F. (1985). Analyzing trade-offs between transportation, inventory, and production costs on freight networks. Transportation Research Part B: Methodological, 19(5), 361–380. https://doi.org/10.1016/0191-2615(85)90051-7
- Chan, F., Jha, A., & Tiwari, M. (2015). Bi-objective optimization of three echelon supply chain involving truck selection and loading using NSGA-II with heuristic algorithm. Applied Soft Computing, 38, 978–987. https://doi.org/10.1016/j.asoc.2015.10.067
- Chen, M., & Tan, Y. (2023). A self-adaptive fast fireworks algorithm for effective large-scale optimization. Swarm and Evolutionary Computation, 80, 101314. https://doi.org/10.1016/j.swevo.2023.101314
- Chopra, S., & Meindl, P. (2007). Supply chain management: Strategy, planning, and operations. Prentice-Hall.
- Dulebenets, M. A. (2021). An adaptive polypoid memetic algorithm for scheduling trucks at a cross-docking terminal. Information Sciences, 565, 390–421. https://doi.org/10.1016/j.ins.2021.02.039
- Dulebenets, M. A. (2023). A diffused memetic optimizaer for reactive berth allocation and scheduling at marine container terminals in response to disruptions. Swarm and Evolutionary Computation, 80, 101334. https://doi.org/10.1016/j.swevo.2023.101334
- Dullaert, W., Maes, B., Vernimmen, B., & Witlox, F. (2005). An evolutionary algorithm for order splitting with multiple transport alternatives. Experts Systems with Applications, 28(2), 201–208. https://doi.org/10.1016/j.eswa.2004.10.002
- Ebrahim, R., Razmi, J., & Haleh, H. (2009). Scatter search algorithm for supplier selection and order lot sizing under multiple price discount environment. Advances in Engineering Software, 40(9), 766–776. https://doi.org/10.1016/j.advengsoft.2009.02.003
- Ekiyor, A., Amino, M. A., & Altan, F. (2019). The effect of supplier selection on operational performance in pharmacies. International Journal of Economics and Management Studies, 6(12), 190–196. https://doi.org/10.14445/23939125/IJEMS-V6I12P121
- Feng, J., & Gong, Z. (2020). Integrated linguistic entropy weight method and multi-objective programming model for supplier selection and order allocation in a circular economy: A case study. Journal of Cleaner Production, 277, 122597. https://doi.org/10.1016/j.jclepro.2020.122597
- Filcek, G., & Józefczyk, J. (2012). Heuristic algorithm for integrated allocation and transportation in three-stage supply network. Procedia - Social and Behavioral Sciences, 54, 1298–1307. https://doi.org/10.1016/j.sbspro.2012.09.844
- Ghodsypour, S., & O’Brien, C. (1998). A decision support system for supplier selection using an integrated analytic hierarchy process and linear programming. International Journal of Production Economics, 56–57, 199–212. https://doi.org/10.1016/S0925-5273(97)00009-1
- Ghorbani, M., & Ramezanian, R. (2020). Integration of carrier selection and supplier selection problem in humanitarian logistics. Computers & Industrial Engineering, 144, 106473. https://doi.org/10.1016/j.cie.2020.106473
- Gören, H. G. (2018). A decision framework for sustainable supplier selection and order allocation with lost sales. Journal of Cleaner Production, 183, 1156–1169. https://doi.org/10.1016/j.jclepro.2018.02.211
- Heinold, A., & Meisel, F. (2020). Emission limits and emission allocation schemes in intermodal freight transport. Transportation Research Part E, 141, 1–26. https://doi.org/10.1016/j.tre.2020.101963
- Hosseini, S., Morshedlou, N., Ivanov, D., Sarder, M. D., Barker, K., & Al Khaled, A. (2019). Resilient supplier selection and optimal order allocation under disruption risks. International Journal of Production Economics, 213, 124–137. https://doi.org/10.1016/j.ijpe.2019.03.018
- Hu, X., Wang, G., Li, X., Zhang, Y., Feng, S., & Yang, A. (2018). Joint decision model of supplier selection and order allocation for the mass customization of logistics services. Transportation Research Part E: Logistics and Transportation Review, 120, 76–95. https://doi.org/10.1016/j.tre.2018.10.011
- Jiang, M., Wang, B., Hao, Y., Chen, S., Wen, Y., & Yang, Z. (2024). Quantification of CO2 emissions in transportation: An empirical analysis by modal shift from road to waterway transport in Zhejiang, China. Transport Policy, 145, 177–186. https://doi.org/10.1016/j.tranpol.2023.10.026
- Kaur, H., & Singh, S. P. (2021). Multi stage hybrid model for supplier selection and order allocation considering disruption risks and disruptive technology. International Journal of Production Economics, 231, 107830. https://doi.org/10.1016/j.ijpe.2020.107830
- Lamba, K., & Singh, S. (2019). Dynamic supplier selection and lot sizing problem considering carbon emissions in a big data environment. Technological Forecasting and Social Change, 144, 573–584. https://doi.org/10.1016/j.techfore.2018.03.020
- Lamba, K., Singh, S., & Mishra, N. (2019). Integrated decisions for supplier selection and lot sizing considering different carbon emission regulations in big data environment. Computers & Industrial Engineering, 128, 1052–1062. https://doi.org/10.1016/j.cie.2018.04.028
- Lee, R. (2021). The effect of supply chain management strategy on operational and financial performance. Sustainability, 13(9), 5138. https://doi.org/10.3390/su13095138
- Mendoza, A., & Ventura, J. (2009). Estimating freight rates in inventory replenishment and supplier selection decisions. Logistics Research, 1(3–4), 185–196. https://doi.org/10.1007/s12159-009-0018-5
- Mohammed, A., Harris, I., & Govindan, K. (2019). A hybrid MCDM-FMOO approach for sustainable supplier selection and order allocation. International Journal of Production Economics, 217, 171–184. https://doi.org/10.1016/j.ijpe.2019.02.003
- Mohammed, A., Setchi, R., Filip, M., Harris, I., & Li, X. (2018). An integrated methodology for a sustainable two-stage supplier selection and order allocation problem. Journal of Cleaner Production, 192(10), 99–114. https://doi.org/10.1016/j.jclepro.2018.04.131
- Parilla, E. S. (2020). Supply chain management adoption and its effect on profitability of Philippine MSMEs. Review of Integrative Business & Economics Research, 10(1), 167–188.
- Pasha, J., Nwodu, A. L., Fathollahi-Fard, A. M., Tian, G., Li, Z., Wang, H., & Dulebenets, M. A. (2022). Exact and metaheuristics algorithms for the vehicle routing problem with a factory-in-a-box in multi-objective settings. Advanced Engineering Informatics, 52, 101623. https://doi.org/10.1016/j.aei.2022.101623
- Pazhani, S., Ventura, J., & Mendoza, A. (2015). A serial inventory system with supplier selection and order quantity allocation considering transportation costs. Applied Mathematical Modelling, 40(1), 612–634. https://doi.org/10.1016/j.apm.2015.06.008
- Rezaei, J., & Davoodi, M. (2007). A deterministic, multi-item inventory model with supplier selection and imperfect quality. Applied Mathematical Modelling, 32(10), 2106–2116. https://doi.org/10.1016/j.apm.2007.07.009
- Salameh, M., & Jaber, M. (2000). Economic production quantity model for items with imperfect quality. International Journal of Production Economics, 64(1–3), 59–64. https://doi.org/10.1016/S0925-5273(99)00044-4
- Sobhanolahi, M. A., Mahmoodzadeh, A., & Naderi, B. (2020). A novel fuzzy multi-objective method for supplier selection and order allocation problem using NSGA II. Scientia Iranica, 27(1), 481–493.
- Van der, W., & Ntshingila, L. (2020). The effect of supplier selection, supplier development and information sharing on SME’s business performance in Sedibeng. International Journal of Economics and Finance Studies, 12(2), 290–304.
- Wagner, H., & Whitin, T. (1958). Dynamic version of the economic lot size model. Management Science, 5(1), 89–96. https://doi.org/10.1287/mnsc.5.1.89
- Wang, L., Wu, Z., & Wang, X. (2023). Multimodal transportation and city carbon emissions over space and time: Evidence from Guangdong-Hong Kong-Macao Greater Bay Area, China. Journal of Cleaner Production, 425, 138987. https://doi.org/10.1016/j.jclepro.2023.138987
- Wicaksono, P., Pujawan, I., & Widodo, E. (2019). A mixed integer linier programming model for dynamic supplier and carrier selection problems. International Journal of Procurement Management, 12(3), 276–297. https://doi.org/10.1504/IJPM.2019.099550
- Yaghin, R. G., & Darvishi, F. (2020). Order allocation and procurement transport planning in apparel supply chain: A utility based possibility-flexible programming approach. Fuzzy Sets and Systems, 398(1), 1–33. https://doi.org/10.1016/j.fss.2019.09.016