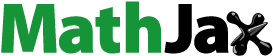
Abstract
The construction industry has a significant multiplier effect on the economy as a whole. It provides the basis upon which other sectors can grow by constructing the physical facilities required for the production and distribution of goods and services. Building construction projects is one of the major development constraints in developing countries since their development highly depends on the growth of their physical infrastructures. Developing countries allocate a considerable amount of their scarce financial resources towards the development of their infrastructure needs. However, most of these infrastructure projects in developing countries encounter considerable quality management controlling problems, especially in public building construction projects. These results in decreasing customer satisfaction, lower productivity, and decreased service delivery times. Thus, the study focuses on exploring fuzzy AHP approaches for quality management control practices in public building construction projects. The data analysis for this study was carried out by using fuzzy AHP methods. This method is used to determine the preference weights of the input variables and for ranking. To achieve the objective, the data were collected from primary and secondary sources. Microsoft Excel was used as an analysis tool. The study revealed that quality management plans and quality management control tools are identified as mostly practiced quality management control systems in public building construction projects. Finally, it can be recommended that the project participants implement and communicate the organization’s mission, vision, strategy, policies, and processes throughout the organization to enhance the productivity of the projects.
Reviewing editor:
1. Introduction
The construction industry has a significant contribution to the national economy of any country. The industry is a distinct sector of the economy, which makes its direct contributions to economic growth. It provides the basis upon which other sectors can grow by constructing the physical facilities required for the production and distribution of goods and services. The industry has a significant multiplier effect on the economy as a whole (Gastelum, Citation2017). Building construction projects is one of the major development constraints in developing countries since their development highly depends on the growth of their physical infrastructures.
Developing countries allocate a considerable amount of their scarce financial resources towards the development of their infrastructure needs. However, most of these infrastructure projects in developing countries encounter considerable quality management controlling problems in today’s construction projects. Quality management systems have many applications in the construction industry and are implemented either at the company level or at the project level. Quality in the construction industry is linked with client satisfaction and the implementation of a quality management system is a key tool in consistently and reliably managing the goal of client satisfaction (Othman et al., Citation2018).
Project quality is often taken for granted and inadequate attention has been given to this parameter. Subsequently, in the absence of effective quality management procedures, considerable time, and resources are wasted every year (Steyn, Citation2012). This is due to the high level of uncertainties surrounding the definition of quality and the subjectivity associated with the assessment of quality as well as the large number of variables involved in its assessment (Othman et al., Citation2018).
The underlying problems of the building construction sector where the building construction projects cannot be completed in conformance to user satisfaction and face a variety of errors in work that affect the end product of the building construction projects due to a total commitment and involvement from top management to lower level of management in all areas of the building construction. Public building construction projects are often taken for improper and inadequate attention has been given to quality management control. The absence of an effective quality management controlling system results in poor product quality decreased customer satisfaction, and lower productivity, and it affects the service delivery times of building construction projects (, & , 2015). Quality management controlling systems have not been fully explored in the previous studies and this provides an opportunity for a scientific study to be undertaken for investigation to achieve good, and effective quality management control systems.
Therefore, due to the stated problems study on quality management control systems was identified as the proposed problem to be studied in the building construction projects. Thus, the study was focused on quality management control practices in public building construction projects.
The significance of this study provides as a reference guide for the quality manager professionals and also for the public building construction projects in general. This study can provide an extended contribution to make practical implications on quality control policies and procedures for construction projects. The study further provides a significant source of secondary data for academicians and researchers to get knowledge, skill, and understanding of quality management control systems in building projects and make further exploration on the topic as required. The study is expected to propose an appropriate quality management mechanism to maximize its benefits delivering high-quality services to meet the expectations of the customers.
The remainder of this paper is organized as follows. Section 2 quality management control practice and Section 3 present how the fuzzy AHP approaches can be adopted. Section 4 shows numerical analysis and fuzzy AHP results along with some discussions related to quality management control practice. Section 5 presents discussions of the findings, managerial implications, and limitations. Finally, general conclusions and remarks are then presented in Section 6.
2. Literature review
The construction industry has a great impact on the economy of all countries. It is one of the sectors that provide crucial ingredients for the development of an economy. The construction industry is a vital element of the economy and has a significant effect on the efficiency and productivity of other industry sectors (Gad & Shane, Citation2014).
The role the construction industry plays in socio-economic development is significant. It provides the basis upon which other sectors can grow by constructing the physical facilities required for the production and distribution of goods and services. The construction industry has a significant multiplier effect on the economy as a whole (Priyadharsan & Naveen Raja, Citation2020).
The construction project is a mission, undertaken to create a unique facility, product, or service within the specified scope, and quality. In Ethiopia, the present state of the construction industry falls short of meeting domestic and international quality standards and the performance demand expected from the sector (Tesfaye et al., Citation2016).
2.1. Quality management
Quality is one of the critical factors in the success of construction projects. Quality of construction projects, as well as project success, can be regarded as the fulfillment of expectations or the satisfaction of the project participants (Sturtevant et al., Citation2021).
Quality begins with the design of a product following the customer’s specifications further it involves the established measurement standards, the use of proper material, the selection of a suitable manufacturing process, etc., quality is a relative term and it is generally used concerning the end use of the product (Oyebisi et al., Citation2019). defined as “Quality is conformance to requirement or specifications.” (Priyadharsan & Naveen Raja, Citation2020) defined as “Quality is fitness for use.” “The Quality of a product or service is the fitness of that product or service for a meeting or exceeding its intended use as required by the customer.”
According to (Mane & Patil, Citation2017) quality is defined by the customers’ requirements, a quality product could also mean meeting the minimum specifications set to get a job done which could occur in the construction sector. Quality management has grown from some simple control techniques into a system of improvement that is increasingly becoming a common practice in both the public and private service sectors (Solomon et al., Citation2019).
Quality management is a management philosophy embracing all activities through which the needs and expectations of the customer, community, and the objectives of an organization are satisfied most efficiently and cost-effectively by maximizing the potential of all employees in a continuous drive for improvement (Oyebisi et al., Citation2019).
Quality Management is a meeting of internal and external customer requirements, and the main difference between quality and Quality Management is that the quality term usually focuses on a temporary process. An example is in construction, to obtain a proper strength for concrete according to the specification, it should be cured for 7 days until the required quality is achieved, so this is a temporary process. Quality Management is a long-term process and adopts a strategic dimension, to guide each production, financial, marketing, and administrative plan in the direction that supports the strategic dimension (Mane & Patil, Citation2017).
Quality Management is an organization’s quality-focused management approach, based on the participation of all its members for long-term success through customer satisfaction and benefits to all members of the organization and the society as a whole (Ismail et al., Citation2019). TQM is a set of management practices throughout the organization geared to ensure the organization consistently meets or exceeds customer requirements (Mane & Patil, Citation2017).
2.2. Quality management control practice
Quality control (QC) is a component of process control and is an essential element of the quality management system. It monitors the processes related to the examination phase of the construction and allows for detecting errors in the construction system (Mane & Patil, Citation2017).
The process through which the standards are established and meet with standards is called quality management control. This process consists of observing our activity performance, comparing the performance with some standard, and then taking action if the observed performance is significantly too different from the standards (Harrison-Zotsi & Dansoh, Citation2021). The control process involves a universal sequence of steps for carrying out the control process was as follows: Choose the control object, choose a unit of measure, set the standard value, choose a sensing device that can measure, measure actual performance, interpret the difference between actual and standard, and take action (Javadikasgari et al., Citation2018). Quality control practice in the construction project requires, improved accuracy and reduced uncertainty need to be balanced against requirements for timeliness and cost-effectiveness. A good quality control practice seeks to achieve that balance and to enable continuous improvement of resource estimates utilized in the building construction project (Yaacob, Citation2012). Quality management control practices identified in the literature can be defined as follows.
2.2.1. Quality management team
A Quality management team is a group of high-level associates hired by the owner of the construction company to take on essential responsibilities within the construction projects (Othuman Mydin et al., Citation2014). The team demonstrates their commitment to quality at all levels of the company. Top management must show leadership and commitment to quality improvement. They must also ensure that quality objectives are consistent with the company’s strategic direction (Leader et al., Citation2020).
2.2.1.1. Effective communication with the project team
Good communication is an important component in project management, allowing projects to progress smoothly and on time. It ensures team members are aligned on project goals and understand exactly what’s expected of them. It also helps build trust so everyone works better together from project start to finish (Khan et al., Citation2019).
2.2.1.2. Responsible quality management team
The quality team is responsible for promoting a quality culture throughout the unit. It plays a supporting role in the development and implementation of quality management systems. depending on the size of the unit, quality teams should consist of at least 5–6 members, with representation from different function areas and staff grades within each unit (Khan et al., Citation2019).
2.2.1.3. Coordination of the team with the project purchase department
Project coordination is focused on the daily tasks required to keep a project running smoothly, while project management involves more high-level planning such as determining project objectives and deliverables. Project managers are often responsible for both project management and project (Leader et al., Citation2020).
2.2.1.4. Commitment of quality management team
Commitment In general, a commitment of construction financing provided to the Contractor or Professionals by the Owner for a project that has been approved by the agent responsible for funding the project (Tan & Rahman, Citation2008).
2.2.1.5. Quality of workmanship in all construction activities
This means that the contractor must carry out the works with reasonable skill and care, to the reasonable satisfaction of the contract administrator. Disputes often arise as to whether or not workmanship is following the specifications (Othuman Mydin et al., Citation2014).
2.2.2.6. Quality leadership
Quality leadership in construction provides control of the overall processes of the construction, and evaluates the workmanship quality of a building construction work based on construction industry standards (Ziegel et al., Citation1999).
2.2.1.7. Attitude towards quality
The “attitude of quality” is about wanting to do the right things. Even if they are not prescribed that way. When no one is watching. When it takes a bit of extra effort. When you are not paid ‘extra’ to do it. In the current scenario (and the time to come), a professional’s ‘attitude of quality’ will be a key differentiator for his/her success (Ziegel et al., Citation1999).
2.2.1.8. Effective/efficient construction method
Select building materials based on affordability, structural capacities, and design aesthetics. Wood is cheaper than steel. Masonry and concrete usually fall somewhere in between. Understanding where certain materials fall on the cost spectrum will help make cost-effective decisions (Abdel-Hamid & Abdelhaleem, Citation2019).
2.2.2. Quality management plan
Quality management plans are a set of fundamental beliefs, norms, rules, and values that are accepted as true and can be used as a basis for quality management (Yaacob, Citation2012).
Quality management plans in construction can be described as a tool that guides construction professionals on the proper execution of construction projects in terms of quality. A finished and acceptable project should be able to meet the required quality standard, and provide customer satisfaction and value for money (Neyestani, Citation2017).
2.2.2.1. Quality plan, and schedule
According to (Othman et al., Citation2018) quality planning in construction projects is to guarantee efforts to accomplish the necessary level of quality for the outcome which are well planned and organized (Mane & Patil, Citation2015).
The purpose of a quality control plan is to provide structure and order to the processes through which a company ensures that its products and procedures meet the appropriate internal and external requirements, such as through customer expectations or federal laws (Harrison-Zotsi & Dansoh, Citation2021; Mane & Patil, Citation2015). Quality planning focuses on taking all of the information available to you at the beginning of the project and figuring out how you will measure quality and prevent defects (Othman et al., Citation2018). Quality planning is a set of activities whose purpose is to define quality system policies, objectives, and requirements, and to explain how to apply these policies, how to achieve these objectives, and how to meet these requirements (Mane & Patil, Citation2015).
2.2.2.2. Review meetings
A building contract review meeting is a crucial step in any construction project, and it is important to make the most of it. Project status review meetings are integral to meeting the client’s needs of finishing the project on time, on budget, and with good quality. They are an essential part of a team’s ability to quickly make adjustments to achieve project goals, accurately report progress, and control the timing of project phases (Marasini & Quinnell, Citation2010).
2.2.2.3. Maintain sequence of construction drawings
A drawing log is a series of spreadsheets used by construction managers to keep track of the numerous moving parts in a commercial build as well as monitor their progress. Drawing logs are comprised of a series of documents that are grouped categorically. At the start of each project, you generally have a bid set, which is used when a project owner wants to price out a project. The bid set may include an addendum that adds revised scope and revised specifications (Harrison-Zotsi & Dansoh, Citation2021).
2.2.2.4. Set procedures to control quality
Quality control procedures are a code of guidelines (usually written down and kept in quality documentation) that set and establish the quality standards and norms that ensure consistent quality across the company and projects (Solomon et al., Citation2019).
2.2.2.5. Quality policy and strategy
The quality policy is a document that is typically created by quality experts and fully supported by top management. The policy should state the quality objectives, the level of quality acceptable to the organization, and the responsibility of the organization’s members for executing the policy and ensuring quality (Mane & Patil, Citation2015).
2.2.3. Quality management control tool
In construction building projects to make rational decisions using data obtained on the product, process, or from the consumer, organizations use certain graphical tools (Yaacob, Citation2012). These methods help us learn about the characteristics of a process, its operating state of affairs, and the kind of output we may expect from it. Graphical methods are easy to understand and provide comprehensive information; they are a viable tool for the analysis of product and process data. These tools affect quality improvement. The quality control tools used for the improvement of quality were discussed as follows (Neyestani, Citation2017).
2.2.3.1. Pareto analysis
Pareto charts help prioritize by arranging them in decreasing order of importance. In an environment of limited resources, these diagrams help companies decide on the order in which they should address problems. Pareto chart aims to figure out the different kinds of “nonconformity” from data figures, maintenance data, repair data, parts scrap rates, or other sources. Also, the Pareto chart can generate a means for investigating quality improvement and improving efficiency, “material waste, energy conservation, safety issues, and cost reductions (Neyestani, Citation2017).
2.2.3.2. Check sheets
Check sheets facilitate systematic record keeping or data collection observations are recorded as they happen which reveals patterns or trends. Data collection through the use of a checklist is often the first step in the analysis of quality problems. A checklist is a form used to record the frequency of occurrence of certain product or service characteristics related to quality (Abdel-Hamid & Abdelhaleem, Citation2019).
2.2.3.3. Cause and effect diagram
It is sometimes called a Fishbone diagram. It was first developed by Kaorv Ishikawa in 1943 and is sometimes called as Ishikawa diagram. The diameter helps the management trace customer complaints directly to the operations involved. The main quality problem is referred to Fish-head; the major categories of potential cause structural bones and the likely specific causes to ribs. It explores possible causes of problems, with the intention being to discover the root causes. This diagram helps identify possible reasons for a process to go out of control as well as possible effects on the process (Marasini & Quinnell, Citation2010).
2.2.3.4. Control charts
It distinguish special causes of variations from common causes of variation. They are used to monitor and control processes on an ongoing basis. A typical control chart plots a selected quality characteristic found from a sub-group of observations as a function of sample number. Characteristics such as sample average, sample range, and sample proportion of non-conforming units are plotted (Marasini & Quinnell, Citation2010). Two limits known as the upper control limit (UCL) and lower control limit (LCL) are also shown on control charts. These limits are constructed so that if the process is operating under a stable system of chance causes, the problem of an observation falling outside these limits is quite small.
2.2.3.5. Scatter diagram (scatter plots)
It often indicates the relationship between two variables. They are often used as follow-ups to a cause-and-effect analysis to determine whether a stated cause truly does impact the quality characteristics.
2.2.3.6. Histogram (bar charts)
It displays the large amounts of data that are difficult to interpret in their raw form. A histogram summarizes data measured on a continuous scale showing the frequency distribution of some quality characteristics (in statistical terms the central tendency and the dispersion of the data). Often the mean of the data is indicated on the histogram. A bar chart is a series of bars representing the frequency of occurrence of data characteristics, the bar height indicates the number of times a particular quality characteristic was observed (Neyestani, Citation2017).
2.2.3.7. Flow charts (graphs)
It shows the sequence of events in a process. They are used for manufacturing and service operations. Flow charts are often used to diagram operational procedures to simplify the system. They can identify bottlenecks, redundant steps, and non-value-added activities. A realistic flow chart can be constructed by using the knowledge of the person who is directly involved in the particular process. The flow chart can identify where delays can occur (Ziegel et al., Citation1999).
2.2.4. Quality assessment
A quality assessment system in construction is a system or method to measure the technical and interpersonal aspects of workmanship in building construction projects (Mane & Patil, Citation2015). He also stated that Quality assessment is the process of measuring and evaluating the quality and outcomes of a product, service, or process in building construction projects.
2.2.4.1. Quality inspection
Inspection is an important tool to achieve quality concepts. It is necessary to ensure the confidence of the manufacturer and aim for satisfaction to the customer. Inspection is an indispensable tool of the modern manufacturing process. It helps to control quality, reduces manufacturing costs, and eliminates scrap losses and assignable causes of defective work (Othman et al., Citation2018). It also specified the types of inspection devices to use and the procedures to follow to measure the quality characteristics (Marasini & Quinnell, Citation2010).
2.2.4.2. Training and seminars on quality management
In general, this approach is the systematic approach to the processes of work that looks to remove waste, loss, rework, frustration, etc. to make the processes of work more effective, efficient, and appropriate (Mane & Patil, Citation2015).
2.2.4.3. Improvement of quality
Quality improvement refers to the application of methods and tools to close the gap between current and expected levels of quality by understanding and addressing system deficiencies and strengths to improve, or in some cases, re-design project processes. These approaches differ in terms of time, resources, and complexity, but share the four steps in quality improvement: identify, analyze, develop, and test (Oakland, Citation2012).
Continuous improvement is a key factor to reduce quality cost and a key factor of quality improvement is a corrective action (Marasini & Quinnell, Citation2010). According to (Othman et al., Citation2018), quality is ‘’conformance to requirements’’, and the requirements of a product need to be defined and specified clearly so that they are properly understood.
2.2.4.4. Quality supervision
Quality assurance establishes organizational procedures and standards for quality implementation. It ensures periodic review and evaluation of the overall project to provide confidence that the project will satisfy the relevant quality standard (Solomon et al., Citation2019).
2.2.5. Measurement, and improvement of quality control
The measurement of quality in the construction industry is very dicey and well is strange the construction business is different than the businesses based on mass production. Measurement of quality in the construction industry must control the quality parameters according to the period of construction activities which are, quality before construction, quality during construction, and quality after construction (Azhar et al., Citation2021).
2.2.5.1. Quality audits
A program of audits is established annually to verify that quality assurance activities comply with the arrangements set out in the QMS and supporting documentation. The Auditor is responsible for verifying the effectiveness of corrective actions. Progress with the implementation of corrective actions is monitored by the Auditor and reported regularly to the Directors and other relevant staff (Shah and Pitroda, Citation2018).
Quality Monitoring and Measurement: Monitoring and measurement of processes key measures have been established to monitor process performance.
2.2.5.2. Control of nonconformities
The organization shall ensure that a product that does not conform to requirements is identified and controlled to prevent its unintended use or delivery. The controls, related responsibilities, and authorities for dealing with nonconforming products shall be defined in a documented procedure (Esmaili-Dooki et al., Citation2017). Arrangements have been established for the reporting, review, resolution, and recording of non-conformities. These arrangements include, for non-conformances with significant quality implications, the identification of root causes and actions to prevent recurrence (Mane & Patil, Citation2015).
2.2.5.3. Quality review
The measures are reviewed and updated to reflect changing business conditions and priorities. The information is analyzed to identify risks, determine forward strategy and opportunities for improvement (Alotaibi, Citation2014).
2.3. Fuzzy AHP Approaches for quality management control practices
As a result of the literature review, it is seen that the research focuses on exploring the quality management control practices in public building construction projects. In this study, multiple decision-making criteria are used, and it has been determined that each building construction project has its quality-controlling practices. In this study, five main criteria were considered by making evaluations with the quality management staff. The determining criteria are presented under basic headings in the previous section.
In many fields, decision-makers (DMs) need to address a problem systematically, accurately, and reliably according to their preferences. Multi-Criteria Decision Making (MCDM) is particularly useful in this area, given that each problem requires a unique decision. MCDM deals with complex problems depending on the solution methods selected by DMs (Azhar et al., Citation2021). One of the first research studies on multi-criteria decision-making was developed by Benjamin Franklin when he published his research on the moral algebra concept (Azhar et al., Citation2021). One of the most interesting topics which is attracted researchers’ attention is associated with personnel selection as an MCDM problem (Mardani et al., Citation2015).
Various techniques are available to solve MCDM problems, such as AHP, TOPSIS, ELECTRE, rough sets theory, and Multi-objective programming (Esmaili-Dooki et al., Citation2017). The main differences between these methods are related to the complexity level of algorithms, the weighting methods for criteria, the way of representing preferences evaluation criteria, uncertain data possibility, and finally, data aggregation type (Taherdoost & Madanchian, Citation2023). In comparison with other MCDM methods, the AHP technique is widely used in MCDM and other problems successfully (Esmaili-Dooki et al., Citation2017). The AHP method has emerged as a useful decision-making technique that breaks down a problem into small pieces to help DMs view the issue at hand for solving and analyzing complex problems (Azhar et al., Citation2021).
The AHP method in a fuzzy environment seems the best way to deal with the uncertainty and ambiguity of evaluations (Mardani et al., Citation2015). Therefore, this paper applied the Fuzzy AHP approach to the quality management controlling practice of public building construction projects for the first time that has not been investigated so far due to the literature, and the considered criteria proposed in the present study are completely new.
In real life, the evaluation of the various subjective criteria and the weights of the criteria are usually expressed in linguistic terms. Also, to efficiently resolve the ambiguity frequently arising in available information and do more justice to the essential fuzziness in human judgment and preference, the fuzzy set theory has been used to establish ill-defined multiple-criteria decision-making problems (Alavi & Alinejad-Rokny, Citation2011).
2.3.1. Fuzzy AHP Approaches
The Analytic Hierarchy Process (AHP) proposed by Thomas L. Saaty is a Multi-Criteria Decision Making (MCDM) technique applied under uncertain conditions or to overcome needed problems. By disparting the decision problem into sub-sections that can each be interpreted separately and are more naturally understood, it reduces the risk of making wrong decisions and ensures the most appropriate decision (Mumcu & Gök, Citation2021).
The Analytic Hierarchy Process (AHP) is a method of “measurement through pairwise comparisons and relies on the judgments of experts to derive priority scales” (Ayalew et al., Citation2022). It has been one of the most widely used multiple-criteria decision-making tools. It is used by decision-makers and researchers because it is a powerful tool to solve complex decision problems. Any complex problem can be decomposed into several sub-problems using AHP in terms of hierarchical levels where each level represents a set of criteria or attributes relative to each sub-problem (Basahel & Taylan, Citation2016).
The AHP method is a multi-criteria method of analysis based on an additive weighting process, in which several relevant attributes are represented through their relative importance (Hummel et al., Citation2014). Through AHP, the importance of several attributes is obtained from a process of paired comparison, in which the relevance of the attributes or categories of drivers of intangible assets is matched two-on-two in a hierarchic structure.
The main advantage of AHP is that it is effective in handling multiple criteria to make decisions and it can cater to qualitative and quantitative data (Zolkepli & Aris, Citation2019). It accepts the pair-wise comparison of each alternative that belongs to each criterion and offers a decision support tool for multi-criteria decision-making problems. In the classic AHP method, the objective is situated on the first level, the criteria is situated on the second level, and the available alternatives are situated on the third level (Zolkepli & Aris, Citation2019). So, the application of Saaty’s AHP has some shortcomings as follows (Basahel & Taylan, Citation2016).
They pointed out that the AHP method is mainly used in nearly crisp-information decision applications; the AHP method creates and deals with a very unbalanced scale of judgment; the AHP method does not take into account the uncertainty associated with the mapping of human judgment to a number by natural language; the ranking of the AHP method is rather imprecise; and have great influence on the AHP results (Hummel et al., Citation2014).
Furthermore, it is also recognized that human assessment of qualitative attributes is always subjective and thus imprecise. Therefore, conventional AHP seems inadequate to capture the decision-maker’s requirements explicitly. To model this kind of uncertainty in human preference, fuzzy sets could be incorporated with the pairwise comparison as an extension of AHP. Therefore, fuzzy AHP was proposed to equip classic AHP with the ability to accept uncertainty and ambiguity in human thinking style (Russo & Camanho, Citation2015; Zolkepli & Aris, Citation2019).
Fuzzy AHP is a technique where pairwise comparisons of the criteria and the alternatives are achieved by using fuzzy triangular numbers to represent linguistic variables. One of the earliest applications of fuzzy AHP provides more realistic results than the original crisp method (Rahim et al., Citation2021) where the choice is between several alternatives under overlapping judgments. Fuzzy numbers were used to represent expert opinion in ranking alternatives across a set of criteria (Figueiredo et al., Citation2006). The fuzzy numbers were used to describe experts’ preferences and fuzzy arithmetic was used to compute fuzzy ranking.
2.3.2. Fuzzy sets and fuzzy numbers
Human logic can take advantage of the concepts and knowledge that do not have well-defined borders (Mehrjerdi, Citation2012). Fuzzy logic was first introduced by Zadeh to deal with problems in which a source of vagueness is involved and has been utilized for incorporating imprecise data into the decision framework (Fathi et al., Citation2011; Sun, Citation2010). Thus the vague data may be represented using fuzzy numbers, which can be further subjected to mathematical operation in the fuzzy domain.
Fuzzy logic is comprised of a wide spectrum of theories and techniques mainly constructed upon the concepts of fuzzy sets, linguistic variables, probability distribution (membership function), and fuzzy if-then rules (Mehrjerdi, Citation2012). Fuzzy sets and linguistic variables are widely used as two fundamental concepts in qualitative assessments. The main idea of fuzzy set theory is that an element has a degree of membership in a fuzzy set (Zolkepli & Aris, Citation2019). A fuzzy set can be defined mathematically by a membership function µ
which assigns each element x in the universe of discourse X a real number in the interval [0, 1].
If the membership degree is zero, the element does not belong to the set. If the membership degree is one, the element belongs completely to the set. Finally, if the value lies within the interval of one and zero, the element has a certain degree of membership, which means that it partially belongs to the set (Zolkepli & Aris, Citation2019).
A Triangular Fuzzy Number (TFN) can be defined by a triplet
as illustrated in . The parameters l, m, and u (l ≤ m ≤ u), denote the smallest possible value, the most promising value, and the largest possible value that describes a fuzzy event. Each TFN has linear representations on its left and right side, such that its membership function can be defined as (Zolkepli & Aris, Citation2019):
(1)
(1)
Figure 1. Triangular fuzzy number (Zolkepli & Aris, Citation2019).

A fuzzy number (FN) can always be given by its corresponding left and right representation of each degree of membership as follows:
(2)
(2)
where,
and
denote the left-side representation and the right-side representation of a fuzzy number (FN), respectively.
Basic arithmetic operations on triangular fuzzy numbers where,
and
where,
can be shown as follows (Elkatawneh, Citation2013):
Additions:
(3)
(3)
Subtraction:
(4)
(4)
Multiplication: if k is a scalar
(5)
(5)
(6)
(6)
Division:
(7)
(7)
Reciprocal of the fuzzy number
(8)
(8)
Although multiplication and division operations on triangular fuzzy numbers do not necessarily yield a triangular fuzzy number, triangular fuzzy number approximations can be used for many practical applications (Elkatawneh, Citation2013). Due to the vagueness and uncertainty of the decision data, crisp data are often short and inadequate for real-life situations. Since preferences and judgment are often unclear and cannot be expressed by exact numerical values, the application of fuzzy concepts in decision-making is deemed to be relevant.
Fuzzification converts crisp values into fuzzy numbers. This conversion is represented by the membership function. A membership function is a curve that defines how each point in the input space is mapped to a membership value (degree of membership) between 0 and 1. These membership functions, in turn, are built from several basic functions (Rahim et al., Citation2021). This work utilized a triangular membership function which is represented by a center-based triplet approach known as triangular fuzzy numbers (TFN). The primary reason for using triangular fuzzy numbers can be stated as their intuitive and computational-efficient representation (Fathi et al., Citation2011).
A linguistic variable is defined as a variable whose values are not numbers, but words or sentences in natural or artificial language. The concept of a linguistic variable appears as a useful means for providing approximate characterization of phenomena that are too complex or ill-defined to be described in conventional quantitative terms (Zolkepli & Aris, Citation2019).
3. Fuzzy AHP methodology
The overall framework and steps of the newly proposed approach for exploring quality management practice by using the fuzzy AHP approach are presented in .
3.1. Questionnaire design
This study employs a fuzzy AHP approach to explore the current practice of quality management control systems. In doing this study, the questionnaire was organized into two sections. The first section focused on the experts’ profiles, and the second questionnaire was developed as a pairwise comparison matrix analysis with fuzzy scales. The questionnaire survey was distributed to ten decision experts who were chosen as the target populations, who have had sufficient work experience in quality management control practice, via physical presence. The relative weights of each factor were developed using a nine-point scale comparison matrix as recommended by Saaty (Saghafian & Hejazi, Citation2005). The scale ranges from 1= equally important up to 9 = absolutely important as it was presented in .
Table 1. Membership function of linguistic scale (47).
3.2. Experts panel selection
One of the key features of the Fuzzy AHP technique is the selection process of expert panel members. For the current study, ten decision experts were selected, because the experts in multi-criteria decision-making problems may depend on the nature of the problem and the availability of experts (Fu et al., Citation2020). AHP has been applied in studies with small sample sizes to solicit and determine the hierarchical analysis, typically based on experts’ opinions (Kil et al., Citation2016). Several studies reported findings from AHP with small numbers of experts, this provides to eliminate respondents who did not fit for the requirements (Sodangi et al., Citation2014). In this respect, different selection mechanisms were adopted to carefully select panel experts. These mechanisms for the selection of the decision expert include based on their prior experience in building construction projects, educational background (Masters and PhD), and their prior knowledge and commitment to participate in the study. To ensure that the invited professionals are well-informed about the technique of Fuzzy AHP and quality management control practice in the building construction projects. Furthermore, in this study a series of face-to-face interviews were conducted for each representative decision expert panels in a group to rank the importance levels of the quality management practices.
3.3. Data collection and analysis methods
In this study first, the data were collected from primary sources through a questionnaire survey. In the next part, the method of data collection was extracted according to what was mentioned in the previous studies that are suitable for carrying out the current study and was defined in the literature to answer the research question (Taherdoost, Citation2020). The study was prepared, and evaluated in a 9-point Likert scale questionnaire set by ten decision experts, and Microsoft Excel and Super Decisions v3 software package was used as a statistical tool. In this study, FAHP was applied via the geometrical mean method of Buckley for exploring the quality management practice. The data obtained from ten decision experts were made fuzzy, using fuzzy number scales associated with linguistic variables. Then, applying the Fuzzy arithmetical operations, the geometrical mean of survey data on each variable was taken and the new Fuzzy values obtained constituted the pairwise comparison matrix. Each comparison matrix was assessed according to the geometrical mean method of Buckley and the Fuzzy weights obtained were made clear according to the center of gravity method. Finally, the clarified values were obtained which give the importance level in the context of the criteria of each quality management controlling practice. Then, we will briefly introduce how to carry out the process of decision support (data analysis) in the study can be summarized in the following steps (Kuzu, Citation2020).
3.3.1. Step 1: Analyzing decision problems
In this step, AHP is to decompose and structure a complex decision problem. The decision hierarchy has been constructed by breaking a decision problem into smaller sub-problems, and the problem becomes more manageable. In this decision structure, the goal of the decision is placed at the highest hierarchical level. The first intermediate level consists of the quantitative and/or qualitative criteria that are meaningful to the decision-makers in comparing the alternatives. If required, each of these criteria can be subdivided into a cluster of sub-criteria at the next intermediate level (Kabir & Hasin, Citation2011). The decision variables are a finite set of variables that the decision-makers aim to compare (Kumar et al., Citation2013). The variables based on the feedback from the questionnaire are shown in in the form of a hierarchical diagram. The structures of the analytic hierarchy process were established by identifying five variable groups, and twenty-eight input variables.
3.3.2. Step 2: Establishing the hierarchy
The hierarchical structure of the variables includes three levels. The top of the hierarchy is the overall objective or goal, the middle nodes are the relevant attributes (variable group) and the last level is the decision input variables of the decision problem. The methodology of the current study was carried out according to the research aim, and the relationships among main variables and input variables are determined and reflected in the hierarchical model as shown in Figure as follows.
3.3.3. Step 3: Questionnaire survey and construct the pairwise comparison matrices, 
for all criteria
Based on the established hierarchical structure, a questionnaire was designed to sort out the quality management control practice, and improving mechanisms of quality management control were identified based on their prior experience in the building construction sector (5 years or more), educational background, and their prior knowledge and commitment to participate in the study. After carrying out the questionnaire survey, the results of the input variables for each objective were converted into a triangular fuzzy scale to reflect the expert’s rating of importance.
The pairwise comparison matrix was an n x n real matrix, where n was the number of criteria. Each element of the matrix denotes the importance of the ith criterion over the jth criterion. The experts made pairwise comparisons of the importance or preference between each pair of criteria (Nurul et al., Citation2023).
The comparison of the criterion was measured according to the numerical scale 1-9 in the form of linguistic variables adapted from (Ramík, Citation2017) as shown in . This can be achieved through questionnaires. Suppose that a decision group consists of K experts. The geometrical mean was used to create an aggregated fuzzy pairwise comparison matrix as seen in EquationEq. (9)(9)
(9) .
The pair-wise comparison judgments are represented by fuzzy triangular numbers denoted by ãij = (lij,mij, uij) and n(n − 1)/2 judgments are required for each comparison group for a level to construct a positive fuzzy reciprocal comparison matrix Ă = {ãij} (Basahel & Taylan, Citation2016). The matrix is expressed as follows.
(9)
(9)
where,
3.3.4. Step 4: Calculating consistency ratio for criteria pairwise comparison matrix
As proposed by Saaty (Fu et al., Citation2020), the AHP verifies a consistency ratio (CR) to measure the consistency of experts’ judgments arranged in pairwise comparisons from the results of the survey. The value CR < 0.1 confirms the comparison matrix consistency, while otherwise the reason for inconsistency should be found and calculations repeated (Simjanovic et al., Citation2023).
The consistency ratio in AHP analysis can be calculated by using the Eigenvalue method, and Super Decisions v3 software package. In the Eigenvalue method, AHP calculates a consistency ratio (CR) comparing the consistency index (CI) of the matrix in question (the one with our judgments) versus the consistency index of a random-like matrix (RI) (Vahidnia et al., Citation2008).
The Eigenvalue method was suggested to perform the consistency check. The consistency ratio (CR) was defined as a ratio between the consistency of a given evaluation matrix and the consistency of a random matrix where RI is a random index that depends on N, as shown in .
Table 2. Random consistency index, RI (58).
Let denotes pairwise comparison matrix and priority weight matrix.
was computed by taking average of eigenvalues. N is denoted by several criteria. Finally, the consistency index (CI) and consistency ratio (CR) were computed according to EquationEqs. (10)
(10)
(10) and Equation(11)
(11)
(11) .
The value CR < 0.1 confirms the comparison matrix consistency and other steps can be performed while otherwise the reason for inconsistency should be found and calculations repeated.
(10)
(10)
(11)
(11)
Second, the consistency ratio can be performed by the Super Decisions v3 software package. According to (Berdie & Osaci, Citation2016), the consistency ratio of experts’ judgments is arranged in pairwise comparison measurements put in the Super Decisions v3 software package for checking inconsistency indexes. Super Decisions v3 is the latest version of the software package developed for the analysis, synthesis, and justification of complex decisions based on the analytic hierarchy process (AHP) methodology (Vahidnia et al., Citation2008).
In this study to measure the consistency of experts’ judgments Super Decisions v3 software package was preferred over than Eigenvalue method; this is due to the Super Decisions v3 software package used to avoid human discrepancies and inconsistency measurement as compared to the Eigenvalue method.
3.3.5. Step 5: Transforming fuzzy scales into triangular fuzzy numbers
As shown in , this study used a fuzzy nine-scale linguistic scaling to weigh the importance of each variable.
Figure 4. The membership values of linguistic variables for two variable comparisons (Fu et al., Citation2020).

The fuzzification can be done by using the triangular fuzzy numbers from the comparison matrix applying (Simjanovic et al., Citation2023).
(12)
(12)
(13)
(13)
This work transformed all fuzzy scales into triangular fuzzy numbers as shown in to weigh the significance of each variable in a fuzzy nine-scale linguistic scaling.
Table 3. The triangular fuzzy number of positive/negative linguistic scale (60).
3.3.6. Step 6: Aggregating the experts’ decision
To aggregate the experts’ decisions, this study adopted the geometric mean method, the most common operation used in fuzzy multiple-criteria decision-making. EquationEq. (14)(14)
(14) can be used to transfer expert opinions into TFN (Fu et al., Citation2020).
(14)
(14)
where,
is the integrated triangle fuzzy number by N experts.
is the i-th to the j-th variable pair comparison by expert k. ⊗ is the symbol of matrix multiplication.
3.3.7. Step 7: Calculate the geometric mean of fuzzy comparison values
Used the fuzzy geometrical mean technique to define the fuzzy geometrical mean of each criterion, which was calculated by EquationEq. (15)(15)
(15) .
(15)
(15)
3.3.8. Step 8: Calculating the fuzzy preference weights (Nurul et al., Citation2023)
By including the subs steps which is finding the vector summation of each as shown in EquationEq. (16)
(16)
(16) and the inverse of the summation vector. The fuzzy triangular was then replaced to make it in the form of increasing order. Defined the fuzzy preference weights of each criterion, which was calculated by using EquationEq. (17)
(17)
(17) .
(16)
(16)
(17)
(17)
where
is the fuzzy comparison value of variable i to variable n.
is the geometric mean of the fuzzy comparison value of variable i to each variable,
is the fuzzy weight of the ith variables, which can be indicated by a triangular fuzzy number. ⊕ is the symbol of matrix plus
Hence wi = (Lwi, Mwi, Uwi). Lwi, Mwi, and Uwi stand for the lower, middle, and upper values of the fuzzy weight of the i-th variables respectively.
3.3.9. Step 9: Defuzzifying the fuzzy preference weights of variables
The fuzzy weights need to be defuzzified because are still fuzzy triangular numbers. Defuzzification is used to renew the fuzzy number into a single crisp value (Fu et al., Citation2020). The Center of the area converts a fuzzy weight into a non-fuzzy value, and the calculation of the defuzzification can be done by using EquationEq. (18)
(18)
(18) below (Wang et al., Citation2019).
(18)
(18)
3.3.10. Step 10: Normalize the defuzzified weight of the criterion
According to (Kil et al., Citation2016) the normalization of the defuzzified weight of the criterion is required as is a non-fuzzy number. EquationEq. (19)
(19)
(19) below shows how to calculate the normalization of each criteria.
(19)
(19)
Step 10 will result in the normalized weights for the criterion, The results were then ordered according to the normalized weights for each of the criteria.
4. Numerical data analysis
4.1. Experts panel selection
In performing the FAHP methodologies first an expert panel was assembled to evaluate the list of criteria, provide feedback to complete the hierarchical structure of criteria, and conduct pairwise comparisons. The panel of experts was selected with care based on their credentials and expertise in relevant domains. The information provided by the expert panel is presented in , and their interpretations of their profile are presented in percentages as follows.
Table 4. The expert panel’s demographics.
The questionnaires were distributed to owners, consultants, and contractors of public building construction projects. The percentage value of the surveyed stakeholders for this study was 30.00% are clients, 40.00% are contractors, and 30.00% are consultants. Regarding the job position of this study, 20.00% were Construction Engineer, 30.00% were Civil Engineer, 30.00% were Project Manager, and 20.00% were Senior Engineer. From the educational level of the respondents, 100.00% were MSc. and above. Among the participants 80.00% of the respondents were male, and 20.00% of the respondents were female. Regarding their field of specialization was identified as 20.00% were CoTM, 30.00% were Construction Engineering, 20.00% were Structural Engineering, and 30.00% were Civil Engineering. As to the working experience of the respondents, 30.00% were between 6-10 years, 70.00% of the respondents were more than 15 years.
4.2. Results of fuzzy analytic hierarchy process methodology
4.2.1. Step 1: Analyzing decision problems, and establishing the hierarchy
The results of fuzzy AHP methodology in this study are described as follows, and this finding can be justified as it is in line with the findings of previous studies (Ayalew et al., Citation2022).
In the first step of this study, the author decomposes and structures a complex decision problem. The decision hierarchy has been constructed by breaking a decision problem into smaller sub-problems, and the problem becomes more manageable.
The hierarchical decision-making problem by considering the variable groups and input variables identified from the literature as the schematic structure depicted (see ). Its ultimate goal is to identify the most practiced quality management control practice in public building construction projects based on the relative importance weight of the input variables, which is placed in the first level in the hierarchical structure based on the pairwise comparison. The five variable groups and twenty-eight input variables are located in the second and third levels, respectively.
4.2.2. Step 2: Construct the pairwise comparison matrices, 
for all criteria
The data obtained from questionnaires were transformed into linguistic terms fuzzy numbers using . The importance comparison for each factor was performed via a questionnaire. The pairwise comparison matrix of the group and input variables evaluated by the decision experts was presented in .
Figure 5. Inconsistency report of group variables under the goal created in Super Decision software. Source: own work, 2023.

Figure 6. Inconsistency report of input variables under quality management team created in Super Decision software. Source: own work, 2023.

Figure 7. Inconsistency report of input variables under quality management plan created in Super Decision software. Source: own work, 2023.

Figure 8. Inconsistency report of input variables under quality management control tool created in Super Decision software. Source: own work, 2023.

4.2.3. Step 3: Calculating consistency ratio for the variables pairwise comparison matrix
In the Eigenvalue method, the expert judgments have been entered randomly and therefore it is expected to be highly inconsistent. Therefore, in this study, the Super Decisions v3 software package is employed to check the consistency of the expert preferences. In this study to measure the consistency of experts’ judgments arranged in pairwise comparisons measurements put in the Super Decisions v3 software package are done automatically to check inconsistency indexes.
The consistency ratio value for all the pairwise comparison matrices generated by Super Decisions v3 software shows comparisons (Node comparisons concerning goal”) and local priorities of the input variables concerning each one of the variables in the model under is less than 0.10; therefore, we can conclude that our judgments matrix of the pairwise comparisons has become reasonably consistent for AHP analysis.
4.2.4. Step 4: Fuzzification, and aggregating the experts’ decision
After checking the validation of the expert’s preference we can apply the fuzzy numbers defined in , all the fuzzy scales can be transferred into the corresponding triangular fuzzy numbers to weigh the significance of each variable in a fuzzy nine-scale linguistic scaling, and for carrying out further analysis. The computation of the TFN of the variables was calculated by using EquationEq. (14)(14)
(14) . displays the aggregated fuzzy pairwise comparison matrix Ă for the variable group about the objective with a TFN as a sample calculation, and the same computational procedures were performed for all variables.
Table 5. Aggregated fuzzy number for the variable group with respect to the goal.
4.2.5. Step 5: Calculate the geometric mean of fuzzy comparison values
The geometric mean of the fuzzy comparison values was found using the Fuzzy Analytic Hierarchy Process (FAHP) and Microsoft Excel for each variable. Using EquationEq. (15)(15)
(15) , the geometric mean for the group variable was calculated as follows:
With similar steps, other calculation of the geometric means of fuzzy comparison values for each variable is calculated. It also includes the total values, the inverse values, and the values in increasing order.
4.2.6. Step 6: Calculate the fuzzy preference weights
The results of fuzzy preference weights are calculated after fuzzy weights for each group variable by using EquationEq. (17)(17)
(17) as follows:
where,
was multiplied by the inverse of the summation vector in the form of increasing order.
Similarly, the remaining fuzzy weights wi values are:
4.2.7. Step 6: Defuzzifying the fuzzy preference weights of variables
According to (Wang et al., Citation2019) the Center of the area converts a fuzzy weight into a non-fuzzy weight or defuzzified weight of each variable, and the calculation of the defuzzification can be done by using EquationEq. (18)
(18)
(18) as follows.
4.2.8. Step 7: Normalizing the defuzzified weight of the criterion
According to (Kil et al., Citation2016) the normalization of the defuzzified weight of the criterion is required as is a non-fuzzy number. Then, the defuzzified weights must be normalized using EquationEq. (19)
(19)
(19) . Therefore, the normalization weight of V1 can be calculated as follows:
Similarly, the value of the remaining group variables and input variables can be obtained in a similar computational procedure and the results are presented in . The normalized weights of the Input variable placed at the third level in the hierarchy structure can be presented in .
Table 6. The fuzzy weights of the variable groups with respect to the goal.
Table 7. The fuzzy weights of the input variables with respect to the quality management team.
Table 8. The fuzzy weights of the input variables with respect to the quality management plan.
Table 9. The fuzzy weights of the input variables with respect to the quality management control tool.
Table 10. The fuzzy weights of the input variables with respect to quality assessment.
Table 11. The fuzzy weights of the input variables with respect to measurement, and improvement of quality control.
Table 12. Weighted values and rankings considered by decision experts.
shows the normalized local weights and global weight of the Input variables placed at the third level in the hierarchy structure and their ranking of all variables considered by the decision experts. The results were then ordered according to the normalized weights for each of the criteria.
5. Discussions, managerial implications, and limitations
5.1. Discussion of results and findings of the applied methodologies
The research results shown in , that the normalized local weights and global weights of the group variables and input variables with their ranking considered by the decision experts were discussed as follows. As it was observed in , the decision experts compared local weights in each group variable and ranked quality management plan (0.243), and quality management control tool (0.233) as the first and second most quality management control practices in public building construction projects. Thus, the decision experts believe that the quality management plan and quality management control tool have been exercised by the quality management staff in the building construction projects.
Quality Assessment (0.200) is the next quality management control practice in building construction projects identified by the decision experts followed by the quality management team (0.176). On the contrary, Measurement and improvement of quality control (0.149) is poorly practiced quality management control practice.
The results indicate that the decision experts are convinced that quality management control practices like quality management plans, and quality management control tools overshadow the practice in building construction projects. Hence, the quality management staff in building construction projects should be taken into consideration and put in more effort and attention to improve those quality management control practices.
Individually, further examining each input variable under their group variable, the greatest weighted value under the quality management team category were responsible quality management team (0.271), quality leadership (0.144), commitment of quality management team (0.125), effective communication with the project team (0.119), and effective/efficient construction method (0.109). On the contrary attitude towards quality (0.102), coordination of the team with the project purchase department (0.065), and quality of workmanship in all construction activities (0.065) are the lower weighted value under the quality management team category.
Next, the quality plans, and schedule (0.277), maintenance sequence of construction drawings (0.237), and review meetings (0.213) were identified as the most practiced in the building construction projects under the quality management plan. Contrariwise, quality policy, and strategy (0.146), and set procedures to control quality (0.125) were poorly practiced.
Furthermore, the highest mostly practiced input variables under the quality management control tool category were check sheets (0.301), Pareto analysis (0.152), flow charts (graphs) (0.149), and scatter diagrams (scatter plots) (0.141). Contrariwise, control charts (0.115), cause and effect diagrams (0.072), and histograms (bar charts) (0.071) are poorly practiced under quality management control tools.
Additionally, the greatest weighted values under the quality assessment category were training and seminars on quality management (0.386), and quality inspection (0.370). On the contrary, quality supervision (0.137), and improvement of quality (0.107) were identified as the poor practice under the quality assessment category in the building construction projects.
Finally, the results revealed that quality monitoring and measurement (0.404), and quality audits (0.373) were identified as the best quality management control practice under measurement and improvement of quality control category. Contrariwise, quality review (0.129), and control of nonconformities (0.094) were identified as poorly practiced quality management control practices.
Overall, the input variable with the highest-ranked final weights among global weights was training and seminar on quality management (0.077), quality inspection (0.074), check sheets (0.070), quality plan, and schedule (0.067), and quality monitoring and measurement (0.060).
The implication is that the level of awareness of the highest-ranked quality management control practice is good, thus, there is a need to upkeep those quality practices. So, it needs attention to advance those quality management control practices in building construction projects to achieve the goal of the projects.
On the contrary, quality of workmanship in all construction activities (0.011), coordination of the team with the project purchase department (0.011), and control of nonconformities (0.014) were identified as poorly practiced quality management control practices. Hence, the quality management staff in the building construction project ought to take into consideration and put in more effort and attention to improve those quality management control systems while handling managing quality.
The implication is that the level of awareness of the above-identified quality management control system is poor, thus, there is a need to intensify that quality management control system in building construction projects. Thus, the project staff should consider those and put in more effort and attention to advance quality management control practices.
5.2. Managerial implications
This study provides us with knowledge on the level of quality management control practice in building construction projects by integrating factor analysis and fuzzy AHP methods. It can also help us to comprehend how academicians carry out a study by using a comprehensive model. This study helps us with important implications for the practitioners, and project managers to have a clear understanding of the quality management control practice that is improperly conducted in their company. The findings of this study provide academia and practitioners with insightful information to enhance the current quality management control practice and help us to take proactive measures and get the optimum result for the poorly practiced quality management control system in public building construction projects.
Moreover, the implications of this study are not limited to researchers and construction industry practitioners alone. The Ethiopian Government could adopt the results of this study to make a remedial solution for the poorly practiced quality management control system. Furthermore, the study may also help the company to enhance efficiency and effectiveness in the implementation of quality management control systems in public building construction projects.
5.3. Limitations
As with any other opinion-based study, the present study also has some limitations. The main limitation of this study is related to the variable identification process, in most cases, a large number of input variables were considered for prioritizing the variables, which requires high computational requirements. This makes the fuzzy AHP model more complex and time-consuming as far as the formulation of the historical database is concerned. Furthermore, the study has been carried out in the Ethiopian context only so; the generalizability of this study might be limited. Hence the study has a limitation in this regard. This study looks forward to exploring the quality management control practice in building construction projects.
6. Conclusions and remarks
The aim of this study was to explore the current practice of quality management control practices in public building construction projects. To achieve the study, the hierarchical model was developed based on the variables identified from an extensive literature review conducted in the previous study. The hierarchical structural model is then used to draft a structural questionnaire to collect realistic data from a panel of experts based on a Fuzzy AHP technique. In this study, fuzzy AHP analysis was employed to analyze the results by using Microsoft Excel respectively. The analysis technique provides to identification of the most important quality management control practices.
Based on the findings and discussion of the study the following conclusions are suggested:
This study examines the current practice of quality management control practices in public building construction projects by using the fuzzy AHP model to prioritize the newly developed questionnaires for the variables by decision experts in terms of their relative impotence, subjectivity, and uncertainty of human assessment are taken into account fuzzy set theory in a fuzzy environment.
From the proposed method, fuzzy AHP helps to find out that the quality management plan and quality management control tool are identified as the first and second-ranked quality management control practices followed by quality assessment by the decision experts in the public building construction projects. Thus, the decision experts believe that the quality management plan and quality management control tool have been exercised by the quality management staff in the building construction projects.
On the contrary, measurement and improvement of quality control are identified as rarely practiced quality management control practices.
The study also concluded that the top five quality management practices mostly practiced in building construction projects were training and seminars on quality management (0.077), quality inspection (0.074), check sheets (0.070), quality plan, and schedule (0.067), and quality monitoring and measurement (0.060).
The finding of this study motivates the authors to formulate recommendations to advance the practice of managing quality management control practices.
The authors recommended that the findings of the current study confirm that the fuzzy AHP technique is a powerful tool for evaluating MCDM regarding quality management practice.
Furthermore, this study recommends that the project participants and stakeholders must develop and implement quality control activities and communicate the organization’s mission, vision, strategy, policies, and processes throughout the organization to enhance the productivity of the projects.
The staff members of the building construction projects should be taken into consideration and put more effort and attention into the quality of workmanship in all construction activities (0.011), coordination of the team with the project purchase department (0.011), and control of nonconformities (0.014).
The paper provides a supportive practical solution for decision-makers in the quality management practice to enhance and improve their quality control practices in managing the quality of building construction projects.
Data availability
The data used to support the findings of this study are available from the corresponding author upon request.
Disclosure statement
No potential conflict of interest was reported by the authors.
Additional information
Funding
Notes on contributors

Girmay Getawa Ayalew
Girmay Getawa Ayalew is currently working as a lecturer at the Woldia Institute of Technology, Woldia University, P.O. Box 400, Woldia, Ethiopia. He is former a lecturer at the Gondar Institute of Technology, University of Gondar, P.O. Box 196, Gondar, Ethiopia. He received his MSc degree in Construction Engineering and Management from the University of Gondar, Ethiopia. His research interest includes BIM, Fuzzy AHP, Artificial intelligence, Quality Management, and Machine learning algorithm. Email: [email protected] Institutional Email: [email protected]
Lidiya Admasu Alemneh
Lidiya Admasu Alemneh is working as a lecturer at the Gondar Institute of Technology, University of Gondar, P.O. Box 196, Gondar, Ethiopia. She received her MSc degree in Construction Engineering and Management from the University of Gondar, Ethiopia. Her research interest includes Quality Management, Fuzzy AHP, and Project Management. Email: [email protected] Institutional Email: [email protected]
Genet Melkamu Ayalew
Genet Melkamu Ayalew is currently working as a lecturer at the Woldia Institute of Technology, Woldia University, P.O. Box 400, Woldia, Ethiopia. She is former a lecturer at the Gondar Institute of Technology, University of Gondar, P.O. Box 196, Gondar, Ethiopia. She received her MSc degree in Construction Engineering and Management from the University of Gondar, Ethiopia. Her research interest includes Quality Management, Fuzzy AHP, and Regression Modeling. Email: [email protected] Institutional Email: [email protected]
References
- Abdel-Hamid, M., & Abdelhaleem, H. M. (2019). Improving the construction industry quality using the seven basic quality control tools. Journal of Minerals and Materials Characterization and Engineering, 07(06), 412–420. https://doi.org/10.4236/jmmce.2019.76028
- Alavi, I., & Alinejad-Rokny, H. (2011). Comparison of fuzzy AHP and fuzzy TOPSIS methods for Plant species selection (case study: Reclamation plan of Sungun Copper Mine; Iran). Australian Journal of Basic and Applied Sciences, 5(12), 1104–1113.
- Alotaibi, F. M. S. (2014). Impact on quality culture of total quality management practices factors. International Journal of Business & Economic Development, 2(3), 35–48. [http://www.ijbed.org/
- Ayalew, G. G., Meharie, M. G., & Worku, B. (2022). A road maintenance management strategy evaluation and selection model by integrating Fuzzy AHP and Fuzzy TOPSIS methods: The case of Ethiopian Roads Authority. Cogent Engineering, 9(1), 1–35. https://doi.org/10.1080/23311916.2022.2146628
- Azhar, N. A., Radzi, N. A. M., & Wan Ahmad, W. S. H. M. (2021). Multi-criteria decision making: A systematic review. (Recent Advances in Electrical & Electronic Engineering (Formerly Recent Patents on Electrical & Electronic Engineering), 14(8), 779–801. (https://doi.org/10.2174/2352096514666211029112443
- Basahel, A., & Taylan, O. (2016). Using fuzzy AHP and Fuzzy TOPSIS approaches for assessing safety conditions. International Journal of Safety and Security Engineering, 6(4), 728–745. https://doi.org/10.2495/SAFE-V6-N4-728-745
- Berdie, A. D., & Osaci, M. (2016). A multicriteria approach in the selection of a SAP UI technology, 7, 1–8. https://doi.org/10.1007/978-3-642-33941-7-62
- Elkatawneh, H. H. (2013). Collaboration, cooperation, and coordination hassan elkatawneh strategic planning: Collaboration, cooperation and coordination date. SSRN Electronic Journal, (December), 1–4. https://doi.org/10.2139/ssrn.2366643
- Esmaili-Dooki, A., Bolhasani, P., & Fallah, M. (2017). An integrated fuzzy AHP and fuzzy TOPSIS approach for ranking and selecting the chief inspectors of bank: A case study. Journal of Applied Research on Industrial Engineering, 4(1), 8–23. https://doi.org/10.22105/jarie.2017.48258
- Fathi, M. R., Zarei Matin, H., Zarchi, M. K., & Azizollahi, S. (2011). The application of fuzzy TOPSIS approach to personnel selection for Padir Company, Iran. Journal of Management Research, 3(2), 1–14. https://doi.org/10.5296/jmr.v3i2.663
- Figueiredo, E. G., Clival, U. P., Rtery, B. A. A., Crawford, N. R., D, P. D., & Al, E. T. (2006). Comparative analysis of anterior petrosectomy. Neurosurgery, 58(February), 13–21.
- Fu, H., Chen, Y., & Wang, G. (2020). Using a fuzzy analytic hierarchy process to formulate an effectual tea assessment system. Sustainability, 12, 1–15.
- Gad, G. M., & Shane, J. S. (2014). Trust in the construction industry: A literature review. (May), 2136–2145. https://doi.org/10.1061/9780784413517.217
- Gastelum, D. (2017). A new approach to impacting the construction industry. Journal for the Advancement of Performance Information and Value, 9(1), 31–40. https://doi.org/10.37265/japiv.v9i1.33
- Harrison-Zotsi, H. A., & Dansoh, A. (2021). Quality control practices in building construction project in Ghana.
- Hummel, J. M., Bridges, J. F. P., & IJzerman, M. J. (2014). Group decision making with the analytic hierarchy process in benefit-risk assessment: A tutorial. The Patient, 7(2), 129–140. https://doi.org/10.1007/s40271-014-0050-7
- Ismail, A., Khan, A., & Hasnain, M. (2019). Effect of management commitment on total quality management in construction. Global Scientific Journal, 7(7), 943–951.
- Javadikasgari, H., Soltesz, E. G., & Gillinov, A. M. (2018). Surgery for atrial fibrillation. In Atlas of cardiac surgical techniques (pp. 479–488). Kolkata, West Bengal: Elsevier Health Sciences. https://doi.org/10.1016/B978-0-323-46294-5.00028-5
- Kabir, G., & Hasin, M. A. A. (2011). Evaluation of customer oriented success factors in mobile commerce using fuzzy AHP. Journal of Industrial Engineering and Management, 4(2), 361–386. https://doi.org/10.3926/jiem.2011.v4n2.p361-386
- Khan, A. H., Imran, A., & Hussain, M. (2019). Evaluation of quality during construction projects: A case study of Pakistan. Mehran University Research Journal of Engineering and Technology, 38(1), 69–82. https://doi.org/10.22581/muet1982.1901.06
- Kil, S. H., Lee, D. K., Kim, J. H., Li, M. H., & Newman, G. (2016). Utilizing the analytic hierarchy process to establish weighted values for evaluating the stability of slope revegetation based on hydroseeding applications in South Korea. Sustainability, 8(1), 58. https://doi.org/10.3390/su8010058
- Kumar, M., Jayaswal, P., & Kushwah, K. (2013). Exploring fuzzy SAW method for maintenance strategy selection problem of material handling equipment. International Journal of Current Engineering and Technology, 12(2008), 600–605.
- Kuzu, Ö. H. (2020). Strategy selection in the universities via fuzzy AHP method: A case study. International Journal of Higher Education, 9(2), 107–117. https://doi.org/10.5430/ijhe.v9n2p107
- Leader, Q. T., Policy, Q., Manual, Q., Members, Q. T., & Manual, Q. (2020). Quality teams – roles and responsibilities. (May)
- Mane, P. P., & Patil, J. R. (2017). Quality management system at construction projects. 5(April 2015), 126–130.
- Mane, P. P., & Patil, J. R. (2015 Quality management system at construction projects [Paper presentation]. Proc. Civ. Eng. PG Conf. 2015, No. October, pp. 323–327,
- Marasini, R., & Quinnell, P. (2010 Investigation of quality management practices in building construction sites in the UK [Paper presentation]. Assoc. Res. Constr. Manag. ARCOM 2010 - Proc. 26th Annu. Conf., No. September, pp. 1307–1316,
- Mardani, A., Jusoh, A., Nor, K. M. D., Khalifah, Z., Zakwan, N., & Valipour, A. (2015). Multiple criteria decision-making techniques and their applications – A review of the literature from 2000 to 2014. Economic Research-Ekonomska Istraživanja, 28(1), 516–571. https://doi.org/10.1080/1331677X.2015.1075139
- Mehrjerdi, Y. Z. (2012). Developing fuzzy TOPSIS method based on interval valued fuzzy sets. International Journal of Computer Applications, 42(14), 7–18.
- Mumcu, A., & Gök, M. (2021). Application of fuzzy Ahp and topsis methods for manager selection. Sosyal Bilimler Araştırmaları Dergisi, 16(2), 270–280. https://doi.org/10.48145/gopsbad.906183
- Neyestani, B. (2017). Seven basic tools of quality control: the appropriate techniques for solving quality problems in the organizations. SSRN Electronic Journal, (March), 1–10. https://doi.org/10.2139/ssrn.2955721
- Nurul, W., Wan, H., Othman, S. A., Ramli, M., Aqilah, S., & Rashid, A. (2023). Utilizing the fuzzy analytical hierarchy process (FAHP) approach to identify the best online food delivery services. Journal of Mathematics and Computing Science, 9(1), 1–12.
- Oakland, J. (2012). Oakland on quality management. 3rd ed, 2022. British: Routledge. https://doi.org/10.4324/9780080479781
- Othman, I., Shafiq, N., & Nuruddin, M. F. (2018). Quality planning in construction project. IOP Conference Series: Materials Science and Engineering, 291(1), 012017. https://doi.org/10.1088/1757-899X/291/1/012017
- Othuman Mydin, M. A., Othman, N. A., & Sani, N. M. (2014). A prospective study on building quality: Relationship between workmanship quality and common building defects of low-cost construction projects. MATEC Web of Conferences, 17, 1001. https://doi.org/10.1051/matecconf/20141701001
- Oyebisi, S., Ede, A., Olutoge, F., Ngene, B., Ofuyatan, O., & Oluwafemi, J. (2019 Quality management in construction project: Empirical study of covenant university sports complex [Paper presentation]. ISEC 2019 - 10th Int. Struct. Eng. Constr. Conf., No. June, https://doi.org/10.14455/isec.res.2019.130
- Priyadharsan, M. A. K. S., & Naveen Raja, M. (2020). Impact of quality control and management in constructions. International Research Journal of Engineering and Technology, 7(May), 3968–3974.
- Rahim, A. A. A., Musa, S. N., Ramesh, S., & Lim, M. K. (2021). Development of a fuzzy-TOPSIS multi-criteria decision-making model for material selection with the integration of safety, health and environment risk assessment. Proceedings of the Institution of Mechanical Engineers, Part L: Journal of Materials: Design and Applications, 235(7), 1532–1550. https://doi.org/10.1177/1464420721994269
- Ramík, J. (2017). Ranking alternatives by pairwise comparisons matrix and priority vector. Scientific Annals of Economics and Business, 64(s1), 85–95. https://doi.org/10.1515/saeb-2017-0040
- Russo, R. D. F. S. M., & Camanho, R. (2015). Criteria in AHP: A systematic review of literature. Procedia Computer Science. 55(Itqm), 1123–1132. https://doi.org/10.1016/j.procs.2015.07.081
- Saghafian, S., & Hejazi, S. R. (2005 Multi-criteria group decision making using a modified fuzzy TOPSIS procedure [Paper presentation]. Proc. – Int. Conf. Comput. Intell. Model. Control Autom. CIMCA 2005 Int. Conf. Intell. Agents, Web Technol. Internet, Vol. 2, pp. 215–220, https://doi.org/10.1109/cimca.2005.1631471
- Shah, H., & Pitroda, J. R. (2018). A critical literature review on quality management for infrastructure projects. International Journal of Engineering Development, 14(3), 18–21.
- Simjanovic, D. J., Vesic, N. O., Randjelovic, B. M., & Vujadinovic, D. (2023). Cyber Security criteria: Fuzzy AHP approach. CEUR Workshop Proceedings, 3529(1), 50–55.
- Sodangi, M., Faris, M., Idrus, A., Hammad, D. B., & Ahmedumar, A. (2014). Best practice criteria for sustainable maintenance management of heritage buildings in Malaysia. Procedia Engineering. 77, 11–19. https://doi.org/10.1016/j.proeng.2014.07.017
- Solomon, O., Obodoh, D., & Felix, O. (2019). Quality management practices in construction; a key to successful building project delivery. Imperial Journal of Interdisciplinary Research, 2(August), 531–538.
- Steyn, H. (2012). A framework for managing quality on system development projects. https://doi.org/10.1016/B978-0-08-096704-2.50020-X
- Sturtevant, C., Metzger, S., Nehr, S., & Foken, T. (2021). Quality Assurance and Control. Springer Handbooks (pp. 47–90). Heidelberg, Baden-Wurttemberg, Germany: Springer Cham. https://doi.org/10.1007/978-3-030-52171-4_3
- Sun, C. C. (2010). A performance evaluation model by integrating fuzzy AHP and fuzzy TOPSIS methods. Expert Systems with Applications, 37(12), 7745–7754. https://doi.org/10.1016/j.eswa.2010.04.066
- Taherdoost, H. (2020). Validity and reliability of the research instrument; how to test the validation of a questionnaire/survey in a research. International Journal of Academic Research in Management, 5, 28–36.
- Taherdoost, H., & Madanchian, M. (2023). Multi-criteria decision making (MCDM) methods and concepts. Encyclopedia, 3(1), 77–87. https://doi.org/10.3390/encyclopedia3010006
- Tan, H. D., & Rahman, C. K. (2008 Top management commitment towards quality management in the context of Malaysian construction organisations [Paper presentation]. Int. Conf. Multi Natl. Constr. Proj., pp. 1–13,
- Tesfaye, E., Berhan, E., & Kitaw, D. (2016). A comprehensive literature review on construction project risk analysis. International Journal of Risk and Contingency Management, 5(4), 1–15. https://doi.org/10.4018/IJRCM.2016100101
- Tmeemy, W., & Hassen, S. M.. (2015). The consequences of poor quality on project management success of building projects. Diyala Journal of Engineering Sciences, 8, 172–182.
- Vahidnia, M. H., Alesheikh, a., Alimohammadi, a., & Bassiri, a (2008). Fuzzy analytical hierarchy process in GIS application. International Archives of the Photogrammetry, Remote Sensing and Spatial Information Sciences, 37(B2), 593–596.
- Wang, C. N., Yang, C. Y., & Cheng, H. C. (2019). A fuzzy multicriteria decision-making (MCDM) model for sustainable supplier evaluation and selection based on triple bottom line approaches in the garment industry. Processes, 7(7), 400. https://doi.org/10.3390/pr7070400
- Yaacob, Z. (2012). Control systems for quality management. Quality Management Practices, 10(May), 160–178. https://doi.org/10.5772/36753
- Ziegel, E. R., Swift, J. A., Ross, J. E., & Omachonu, V. K. (1999). Principles of total quality. Technometrics, 41(4), 381. https://doi.org/10.2307/1271376
- Zolkepli, M., & Aris, T. N. M. (2019). Fuzzy AHP and TOPSIS in cross domain collaboration recommendation with fuzzy visualization representation. International Journal of Machine Learning and Computing, 9(6), 849–854. https://doi.org/10.18178/ijmlc.2019.9.6.882