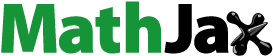
Abstract
High specific strength and good fatigue limit are the key properties to watch out during development of aerospace components. Aluminum composites are proven high specific strength materials especially graphene embedded composites worth a mention in this context. While, surface finish is an eminent parameter affecting fatigue strength of a component showcasing the importance of machining technique employed to transform a fabricated bulk into finished product. Current study, therefore emphasizes on electrical discharge machining (EDM) of aluminum composites embedded with graphene nanoplatelets (1.5 wt.%) fabricated through a hybrid approach of blending solid and liquid metallurgical routes. Further, mathematical and neurological forecast models are developed to individually predict the machining response variables namely surface roughness (SR), material removal rate (MRR) and tool wear rate (TWR). Among machining parameters current (I), pulse on-time (Ton), pulse off-time (Toff) and flushing pressure (P) considered; Ton is noted to greatly influence the surface quality of composite while TWR and MRR are affected by current during ANOVA analysis. On comparative understanding of forecast models, neurological models outperform quadratic non-linear mathematical models where accuracy of prediction achieved by developed artificial neural network (ANN) model is 96% for surface roughness. The error performance plots, error histograms and overall fit plots depict a marginal over-fit neurological model. However, significantly high coefficient of correlation (R) of 99% possessed by ANN model illustrates their potential in forecasting response parameters.
1. Introduction
The ever-increasing demand for new age engineering materials to sustain sophisticated technological advancements ushered us in the era of alternative state-of-the-art materials (composites, ceramics, polymers, and super alloys) for ease of design and manufacturing. Thus, current industry quests for peculiar set of properties, that can accelerate the processing of composite materials composed of two or more different phases (Chawla and Chawla, Citation2014). The matrix is one of the phases of a composite that is larger in proportion with respect to the other, that is, reinforcement. The primary property of the matrix is comprehensiveness, that is, between any two arbitrary points in the matrix, a path exists and surrounds the reinforcement. These multifunctional materials have exceptional properties compared with those of individual alloys.
Present pollution norms insist that automobile manufacturers use ultra-high specific strength materials, that is, materials ahead of conventional metal matrix composites (MMCs) with micro-sized reinforcements (Raju et al., Citation2016). On the arrival of nanomaterials, major innovations have been mainly oriented towards the development of metal matrix nanocomposites (MMNCs) to overcome the limitations of composites with micro-sized reinforcements in the materials world (Bhat et al., Citation2021). However, the strength of the final composite greatly depends on the choice of reinforcement and matrix material. Although, specific strength of magnesium is 40% greater than aluminum and 50% greater than steel, its usage is limited on the basis of its major disadvantages like limited high temperature strength, high degree of shrinkage during solidification, high chemical reactivity etc. Therefore, aluminum has evolved as the predominant matrix material for lightweight applications (Garg et al., Citation2019). Despite the large number of techniques available for the fabrication of aluminum metal matrix composites (AMMCs), stir casting is the most widely used technique owing to its ease of fabrication and cost-effectiveness (Kumar et al., Citation2019; Kotteda et al., Citation2022). However, it suffers from a lack of wettability between the nanoreinforcement and the matrix, which is yet to be explored.
Recently, Graphene Nanoplatelets (GNP) have gained significant interest as an excellent reinforcement material to revolutionize the industrial landscape owing to their phenomenal electrical, mechanical, and thermal properties and chemical inertness (Ghazanlou and Eghbali, Citation2021). Rahman et al. (Rahman et al., Citation2018) reported that carbon agglomeration is a major drawback ordinarily confronted in the development of GNP/Al composites, which has led to an impact on their mechanical behavior. To overcome this hindrance, researchers fabricated Al/GNP composites using combined stir casting and powder metallurgy and reported that there is a significant enhancement in compressive strength of 23.61% and hardness of 24.65% (Sharma et al., Citation2019). Zhang et al. (Zhang et al., Citation2022) employed a combination of deformation and pre-distribution to avoid carbon agglomeration in GNP reinforced AMMCs and achieved a 293.3% increase in the strength. However, this infusion of strength, exhibited a nuanced relationship with the weight % of GNP. However, the subsequent increase in reinforcement concentration led to a depletion in performance, which was attributed to the agglomeration of GNP (Bisht et al., Citation2017).
Because of the heavy tool wear caused by the presence of hard reinforcement, it is extremely difficult to process particle reinforced AMMCs using a traditional machining approach that worsens the surface of the composite sample (Ho and Newman, Citation2003). In addition, various engineering components of cast composites used in the automobile and aerospace sectors often possess near-net-structures; however, they require further machining to attain pertinent dimensional accuracy and surface quality, exclusively for achieving proximate tolerances in complex profiles if needed (Singh, Citation2012). In view of these circumstances, non-traditional machining methods have proven to be a promising solution for machining AMMCs. In which, electrical discharge machining (EDM) is highly suitable for machining cast aluminum composites (Kotteda et al., Citation2022). EDM process involves melting the material by the application of heat produced by a series of electric discharges generated between the workpiece material and tool electrode (Lin et al., Citation2001). The cost-effective nature of EDM along with its ability to achieve a high MRR (material removal rate), low SR (surface roughness), less TWR (tool wear rate), and close tolerances with geometric precision, have contributed to its widespread usage. Materials subjected to EDM undergo changes in the microstructure, thereby increasing the wear resistance and corrosion resistance owing to the deposition of carbide and oxide layers (Chauhan et al., Citation2022). An examination of the available literature revealed approximately 20 process parameters linked to die-sinking EDM. These parameters collectively govern the operational attributes of the machining procedure (Bédard et al., Citation2020).
In the current scenario, the processing cost and performance are significant parameters that determine the application of machining. Thus, the use of EDM may be facilitated by reducing the machining budget. This extent of investigation is possible by implementing the Taguchi technique to feasibly design a set of experiments. The contribution of the process parameters to attaining the machining response was assessed by performing ANOVA analysis (Uğur et al., Citation2020). Technological advancements in the past decade have resulted in the use of mathematical modelling and artificial neural networks to predict the machining performance of EDM on various materials (Debnath et al., Citation2018). Recently, Shyn et al. (Shyn et al., Citation2023) developed an intelligent neural network model for EDM response parameters while machining Al composites reinforced with SiC and B4C, individually fabricated through a stir casting route.
Therefore, innovative high-specific-strength materials are needed to replace steels in aerospace applications, especially components such as stringers that are subjected to continuous tensile forces developed to sustain the explosive stress induced by pressure potential at higher altitudes. This necessity helps establish the novel research contributions as follows.
Fabrication of Al/GNP composites through a novel hybrid approach with a 5:1 launching vehicle to nanoreinforcement ratio.
The die-sinking EDM process was performed for the Al/GNP sample, achieving the best tensile strength to scrutinize the effect of machining parameters on the response variables individually based on the L25 orthogonal array.
Various linear and quadratic 3D mathematical models have been established to predict responses such as MRR, SR and TWR, through various machining parameters such as current, pulse on-time, pulse off-time, and flushing pressure.
Develop and explore the capabilities of neurological models in forecasting machining responses employing the Levenberg-Marquardt training algorithm.
A comparative analysis was performed between the mathematical and neurological models based on the prediction accuracy to identify the best model.
2. Materials, fabrication and evaluation
2.1. Materials involved
The present investigation involved aluminum–copper alloy graded AA2024 with a chemical composition shown in , which is considered as a matrix material, while Graphene Nanoplatelets with a surface area of 300 m2/g were used as the reinforcement material (). Further, 75 µm aluminum powder was employed as the launching vehicle to introduce nanographene platelets into the liquidus matrix.
Table 1. Chemical composition of Al-Cu alloy (AA2024).
2.2. Fabrication process
2.2.1. Predistribution
The Aluminum launching vehicle and Graphene Nanoplatelets were ball-milled in a planetary ball mill with 20 tungsten carbide balls at 300 rpm for 2 h. This powder metallurgy procedure was performed to pre-distribute the graphene reinforcement onto launching vehicles. The ratio of launching vehicles to nanoreinforcements considered for ball milling was 5:1 (Raju et al., Citation2015).
2.2.2. Stir casting
Argon gas-controlled environment stir casting equipment was used to fabricate the Aluminum/GNP composite sample, as depicted in step-1 of . Cylindrical ingots of matrix material were initially placed in an Inconel crucible, and the entire casting setup was then isolated from its surroundings using a vacuum pump, followed by the introduction of argon gas, as shown in . The flow of argon gas was verified by immersing the gas outlet in a vessel filled with water to observe the gas bubbles generated by the gas flow. The matrix material in the crucible was heated to 700 °C until the two-stage stirrer was rotated, indicating the transformation of the solid to the liquid phase (Sita Rama Raju et al., Citation2016). The matrix melt was then held at 700 °C for approximately 20 min, while the stirrer was rotated at 200 rpm to ensure uniformity in temperature throughout the crucible. The predistributed launching powder was heated to 200 °C before being progressively introduced into the matrix melt for approximately 15 min. During this procedure, the stirrer rotates at 200 rpm, leading to the formation of a vortex in the melt that assists the predistributed powder from entering the lower parts of the matrix melt. After completely adding the pre-distributed powder, the stirrer was stopped, and the pneumatic lever controlling the bottom-pouring mechanism was activated to release the molten composite into the die chamber.
2.2.3. Mechanical behaviour
A detailed mechanical study with vigorous metallurgical findings is presented in our earlier publication (Kotteda et al., Citation2023) involving X-ray maps and Energy Dispersive Spectroscopy (EDS) to understand the strengthening behavior of all cast composite samples, as listed in . All bulk samples were prepared according to the ASTM E8 standards before performing tensile tests. The tensile test results obtained for various samples are listed in . The sample achieving superior strength, that is, sample D from possessing 203 MPa is considered for later analysis in this investigation.
Table 2. Composition of different cast composite samples.
Table 3. Ultimate tensile strength and % elongation of various cast composite samples.
2.2.4. Metallurgical behaviour
The metallurgical findings of all the samples were analyzed in our earlier investigation (Kotteda et al., Citation2023), correlating the superiority of the sample with the Hall patch effect and the Orowan strengthening phenomenon. Strength degradation through Fe intermetallic compounds with aluminum alloy was not evident in any of the samples, although an iron-rich inconel alloy was involved as a crucible in the casting process. Field emission scanning electron microscopy (FESEM) images of the fracture and composite surfaces of the superior sample are shown in . In summary, a superior composite sample was considered for further analysis.
3. Parametric design and machining
3.1. Design of experiments
Design of experiments (DOE) is used as an analytical approach to establish the correlation among various factors influencing the process. The application of DOE necessitates perfect planning, advisable configuration of the experiment, and proficient assessment of experimental outcomes by simply reducing the set of experiments to attain feasible situations (Radhika et al., Citation2014). The design plan of the current study according to CREATOR CR-6C model EDM setup available, is to examine the four process parameters (current, pulse on-time, pulse off-time and flushing pressure) of the electrical discharge machining that significantly implies the quality and economic feasibility of the product, where each parameter is to be varied for 5 levels. However, for a 4 factor-5 level setup the total number of experiments planned to be performed was 625 (i.e., 54 = 625 combinations) as a full factorial design. It is difficult to practically run a full factorial design of experiments; thus, the Taguchi method has been noted in the literature to implement the same design with a minimum number of experimental runs (Laxman and Raj, Citation2015). Thus, MINITAB 14 software was used to generate the optimal design of experiments by forming an L25 orthogonal array. lists the selected EDM process parameters and the corresponding levels considered in this study.
Table 4. Levels of various process parameters considered.
3.2. Performance measurements
In general, the performance of any machining process is characterized by the following set of output parameters: MRR and SR. Material removal rate is a significant machining parameter that considerably influences productivity and overall machining time. It can be defined as the amount of material in terms of the volume removed per unit time and is calculated as the ratio of the difference between the final and initial weights of the workpiece to the product of the machining time and workpiece density (from EquationEq. (1)(1)
(1) ). A larger is a better criterion usually considered for the output response MRR.
(1)
(1)
Here, and
refer to the weights of the workpiece before and after machining in grams, respectively; t is the machining time in minutes; and
represents the density of the workpiece material. Moreover, surface roughness is the second output response considered in the present study, which is evaluated by the deflections in the regulation of the normal vector of the actual surface with respect to its ideal one. If these deflections are large, the surface appears rough. If they are small, the surface is indexed as smooth. Here, the index of the surface was obtained by measuring the mean absolute deviation from the average surface level using computer-controlled surface roughness tester. A smaller is a better criterion usually considered for the output response SR. Furthermore, tool wear rate was measured as the third output response in the machining study. It measures the wear rate of the electrode on the workpiece sample while the machining is going on. During the electrical discharge machining process, the electrode progressively eroded owing to frequent electrical discharges (Chekuri et al., Citation2022). However, it can be expressed as the ratio of the difference between the final and initial weights of the electrode tool to the product of the machining time and the density of the electrode material (from EquationEq. (2)
(2)
(2) ). Generally, a smaller is the better criterion for the output response TWR. Here,
and
refer to the weights of the electrode tool before and after machining in grams, respectively; t is the machining time in minutes; and
represents the density of the electrode material.
(2)
(2)
3.3. Experimental findings
Metals tend to possess higher hardness owing to the addition of reinforcement, leading to inferior machining performance using traditional methods, as noted in the literature. Electrical discharge machining is an advanced and conversant approach engaged in making intricate forms (Paswan et al., Citation2020). The present study involved experiments to examine the impact of several input parameters on the machinability of the workpiece sample. Thus, the main aim of our study is to evaluate the influence of I, Ton, Toff and P on machining performance characteristics such as MRR, SR and TWR. From section-2, after identifying the best composite sample based on the strength perspective, that is, sample D (AA2024/1.5 wt. % GNP composite) of ultimate tensile strength 203.51 MPa, hardness 81.5 BHN and density 2.671 g/cm3, the experiments are conducted on die-sinking EDM setup of CREATOR CR-6C model (step-2 of ) where commercially accessible EDM oil is utilized as a dielectric medium. Initially, this dielectric fluid helps accelerate a reliable and controllable spark gap ionization environment by acting as a semiconductor between the anode (workpiece connected to positive polarity) and cathode (electrode connected to negative polarity). However, it acts as a flushing agent to wash and expel deteriorated debris from the spark-gap region (Chakraborty et al., Citation2015). Moreover, copper-tungsten electrode material (Cu50/W50) which is prepared in equilibrium mixture of both the metals with its diameter 10 mm, length 120 mm and density 11.85 g/cm3 used in the current study. For each experimental run, the sample workpiece and electrode tool were removed from the test setup fixtures for cleaning and polishing; thus, the system was free of foreign particles. In addition, for every trial, the weights of the electrode and specimen were measured before and after machining using a Scientech Se-391 electronic balance with an accuracy of 0.0001 gm, which helps to calculate the MRR and TWR as discussed in section 3.2. The surface roughness on the machined surface was measured using a Talysurf tester by taking the average of three values. lists the experimentally observed values of the output responses such as MRR, SR and TWR.
Table 5. Experimental observations of Al/GNP composite machined samples on EDM.
The surface roughness values obtained in current study ranges from 1.686 to 3.98 µm while Shyn et al. (Shyn et al., Citation2023) reported a minimum of 2.5 µm and a maximum of 5.8 µm while performing EDM process on Al6064/B4C & SiC composites. This distinction in surface roughness is related to the presence of ceramic reinforcements, that is, B4C and SiC, which lead to higher surface roughness. However, graphene nanoplatelets are non-ceramic in nature, and carbon materials provide good surface quality in comparison to ceramic reinforcements.
4. Results and discussion
This section describes the performance evaluation of the machining responses MRR, SR and TWR based on signal-to-noise ratio plots and ANOVA analysis. Furthermore, mathematical and neurological forecast models were developed to predict the machining behavior of the composite sample (AA2024/1.5 wt. % GNP).
4.1. Performance evaluation
4.1.1. Signal-to-noise ratio (S/N) analysis
Achieving exceptional quality of surface finish with minimal machining time is the major criterion adopted in every type of machining action, either traditional or non-traditional. Moreover, there may be a trade-off between the rate of material removal and surface roughness of the machining sample. In addition to the machining parameters, workpiece material, and type of dielectric fluid used, the precision of electric discharge machining can also be affected by the type of electrode used (Singh et al., Citation2023). The following discussion precisely exemplifies the effect of the process variables on each machining response during the processing of composite samples using EDM based on S/N plots.
In EDM operations, material erosion arises owing to local melting, which is further splashed with a dielectric medium. However, the medium cannot flush out all of the waste material, resulting in the formation of a re-cast layer that affects the material characteristics. depicts the plot of the main effects for signal to noise ratios of the machining response MRR. The major contribution to the process parameters of the performance outcome MRR is illustrated by the response divergence line with respect to the x axis. Among the various input parameters considered in the EDM process, the current is the most significant, followed by the pulse on-time, whereas the pulse off-time showed a marginal impact on MRR. The performance measures of the S/N ratios for MRR based on the experimental data and the order of priority among the input parameters are listed in .
Table 6. Response table for SN ratios of MRR on larger is better criterion.
Generally, the surface of an electrically discharged machining sample entails cavities, micro voids, globules of debris, deposited layers, cracks, etc., leading to the generation of irregularities on the surface texture, which is referred to as surface roughness (Naik et al., Citation2022). shows that the pulse on-time is the most prominent factor influencing the surface texture of the machining sample, followed by the current and pulse off-time. However, the trend of the flushing pressure exhibited a negligible change in SR. shows the performance measures of the S/N ratios for SR. The order of priority among the input parameters was ranked. The plots of the main effects of the S/N ratios on TWR are shown in . Current and pulse on-time are noted to have an inverse relationship with TWR while pulse off-time and flushing pressure show a biased trend. The response table plotted on the smaller the better criterion () illustrated current as the most promising factor influencing TWR with rank 1 followed by Ton, Toff and P. However, the EDM process carried out on various composite samples showed identical trends of current influencing MRR (Ammisetty et al., Citation2021) and pulse on-time affecting SR (Radhika et al., Citation2014). The collective effect of current and pulse on-time impacts TWR reported in earlier studies (Palanisamy et al., Citation2020).
Table 7. Response table for SN ratios of SR on smaller is better criterion.
Table 8. Response table for SN ratios of TWR on smaller is better criterion.
4.1.2. ANOVA analysis
ANOVA analysis is a compilation of statistical models, and their corresponding evaluation procedures help determine the variation among the means. Indirectly, it helps to validate the influence of one or more process parameters on the output response of machining by comparing the means of different runs (Balaji et al., Citation2023). If a process parameter has attained a larger contribution, there is a greater probability of a greater effect on the performance of the machining process. The final outcomes obtained from the ANOVA analysis for the various responses are summarized as:
The factor that primarily impacts the MRR as per the statistics of ANOVA analysis (from ), is current that holds almost 73.02% of its total contribution, followed by the pulse on-time and pulse off-time contributing 12.80% and 8.84%, respectively. While, the flushing pressure implies a nominal effect of 1.53% only.
Similarly, for SR outcomes in ANOVA analysis (from ), the most significant factor is pulse on-time, which holds 76.12% preceded by current and pulse off-time of 10.88% and 4.46%, respectively, whereas flushing pressure shows marginal impact of 1.83%.
From the TWR results in ANOVA analysis (from ), current is the most influential factor that affects 51.57% of total contribution, followed by the pulse on-time and pulse off-time standing, with 23.97% and 14.81%, respectively. While, the flushing pressure has negligible impact of 0.67%, and the remaining is error in contribution.
Table 9. Analysis of Variance for MRR.
Table 10. Analysis of Variance for SR.
Table 11. Analysis of Variance for TWR.
4.2. Prediction analysis
The transformation of bulk samples into useful products necessitates the awareness of appropriate machining conditions to achieve the desired response characteristics. This is usually achieved by performing all possible machining combinations on the bulk sample and evaluating their responses. This traditional procedure increases the cost of the final product because of losses in the competitive pricing strategy. This is where prediction models are helpful in forecasting the response variables from a combination of machining parameters. Broadly, forecast models can be classified into linear and nonlinear models, where achieving a coefficient of determination (R2) of 1 is a criterion (Sahoo et al., Citation2021). In this section, linear and nonlinear models are developed to predict response variables, such as MRR, SR and TWR.
4.2.1. Mathematical modelling
A simple mathematical model to predict responses is developed through linear regression, which estimates the linear relationship between the machining parameters and response variables. The EquationEqs. (3–5) are the linear equations developed using Minitab software with an R2 values of 91.4%, 87.2%, and 88.7% for MRR, SR and TWR respectively. The R2 value of 1 or 100% depicts the best fit model to forecast the response variable i.e., the model is capable of predicting the responses with 100% accuracy. The developed linear regression models, however, show majority of values below 90% elucidating an improper fit model.
First order model
(3)
(3)
(4)
(4)
(5)
(5)
A sample graphical representation showing the effect of two machining parameters, that is, current and pulse on-time, on the MRR is shown in . The contour plot is a 2D representation of the 3D space, where the top-right corner depicts the highest MRR while the bottom left corner indicates the lowest MRR. The change in color of the contour indicates an increase in elevation or MRR value, which indicates that at a high current and high Ton, the MRR is also high. The 3D linear surface plot shows the presence of points either above or below the surface, indicating that the model is inefficient for estimating the behavior of the response variable with machining parameters. The residual plot also illustrates the same; a large residual error seems to be present as very few points reside over the surface, while most of them possess either positive or negative residues. In summary, real-time machining data involve unavoidable noise and outliers that are complex in nature, which are difficult for a linear model to estimate. The developed linear model cannot predict the response with good accuracy, and there seems to be a non-linear relationship between independent and response variables, as observed from the graphical representation. Therefore, it is vital to develop nonlinear models such as quadratic and polynomial regression models.
Figure 7. Contour (a), surface (b) and residual plots (c) between Current and Pulse-on-time with MRR.

A second-order nonlinear quadratic model was developed to predict the response variables MRR, SR and TWR as given in EquationEqs. (6–8), respectively. The R2 values are 99%. 93.8%, and 99.5% respectively for MRR, SR and TWR. These R2 values were above 93% for SR and above 99% for MRR and TWR, indicating that they are the best fit models to estimate the responses. The predicted responses employing the above quadratic equations are listed in . Finally, the quadratic model can predict MRR and TWR with great accuracy; however, the SR model is not as significant as the rest of the models. Surface roughness is a vital parameter for any finished product and a better model is imperative.
Table 12. Predicted responses from second order non-linear model (E means Experimental and R means Regression).
Second order model
(6)
(6)
(7)
(7)
(8)
(8)
4.2.2. Neurological model analysis
The artificial neural networks (ANN) that we employ is a theoretical clone of a biological neuron network in the brain, where every signal from a neuron has its hierarchy based on the importance of the signal (Okewu et al., Citation2021). This hierarchy in our ANN was matched with the weights and biases. There are three functions that build a simple neuron, which is a building block of ANN: weight, input, and transfer functions. These functions are responsible for all the changes in the neurons of ANN. In a simple form, the output is a transfer function of the input function, which is the sum of the weight function and the bias, as depicted in . ANN is extensively used nowadays in all fields to develop forecast models in various business and research activities, naming deep learning (Sarker, Citation2021). Hence, in the present investigation, this deep learning neural network was deployed to estimate outputs from the given input data.
A neural network framework from MATLAB was employed to develop forecast models for each response variable. The raw data used for the development of ANN model consisted of 25 data sets having four independent variables and three responses as shown in . shows the dataset allocation to various events of building and evaluating models, such as training, testing, and validation. For training, a Levenberg-Marquardt algorithm was employed with one hidden layer, and the performance measure was calculated through the mean squared error for all ANN models. The number of neurons in the hidden layer were varied from 3 to 10, and training was performed repetitively until an appropriate fit (coefficient of correlation (R) value equal to 1) was achieved. The initial weights and bias considered during training are taken as zeros unlike default random assignment done by the MATLAB, while a tangential sigmoidal activation function is employed. illustrates the neural network architecture that achieves the best-fit model. In the case of MRR, shows the use of a single hidden layer with five neurons. SR forecast model achieved the best fit, with a single hidden layer containing 10 neurons. The TWR neural network architecture had five neurons in a single hidden layer, achieving the best fit, as shown in .
Figure 9. ANN structure and training performance information of machining responses: MRR (M1 and M2), SR (S1 and S2), and TWR (T1 and T2).

Table 13. Data set allocation during model development.
Neural network training was terminated when any of the five criteria were met: i) minimum gradient magnitude, ii) maximum number of validations (in our case, it was set at 6), iii) maximum training time, iv) goal of minimum performance value (zero in our case), and v) maximum number of training epochs (1000 epochs in our case). The progress of ANN training is depicted in (M2, S2, and T2) for the MRR, SR and TWR samples, respectively. From all the images available, it is evident that among the five criteria, all samples are trained until the goal of zero error is met, as is evident from the fully shaded rectangular block adjacent to the performance metric. The training, testing, and validation processes are demonstrated by means of fit lines drawn between the targets and predicted outputs by the ANN model, as shown in .
Figure 10. Training, validation, testing, and overall fit plots of ANN for machining responses: MRR (M), SR (S), and TWR (T).

It is exclusively evident that all the three responses i.e., MRR, SR and TWR possessed best fit of R = 1 during training process, illustrating that the ANN model is capable of predicting the target with 100% accuracy. However, upon comparison of validation fit plot and testing fit plots, none of the ANN models were able to predict the targets of new data with 100% accuracy. Bharti et al. (Bharti, Citation2020), study using radial basis function neural network (RBFNN) achieved high accuracy of 96% during training the model with 32 data sets on EDM parameters. From , the minimum accuracy achieved for MRR was 98% during validation, for SR it is 92% on testing data and, 94% on testing data for TWR. This phenomenon demonstrated by ANN models possessing good accuracies for training data with poor performance on testing and validation data can be termed overfitting models. However, this overfitting is marginal and negligible, as the overall R value is sufficiently high. Identical phenomenon of marginal distinction in training (99%) and testing (91%) accuracies was also observed by Ramazan (Çakiroğlu, Citation2022) while investigating EDM parameters on titanium–aluminum alloy, where 18 data sets were used for training and four datasets for testing. However, identifying outliers and noise in the datasets is not illustrated by any means, and the validation of the developed model has not been reported.
The distribution of errors during neural network testing is shown through error histograms in . Each block-shaped entity in the diagram is referred to as a bin. In our case, 25 test samples were chosen to be illustrated into 20 bins on the x-axis (if placed one beside the other) to represent the error histogram. The x-axis of the histogram represents the error achieved by calculating the difference between the experimental outputs, called targets, and outputs obtained from the model. The y-axis shows the samples present in each bin, which are called instances. According to the distribution of raw data considered in the neural network analysis, as shown in , 17 random samples for training and 8 samples were equally allotted for validation and testing phases.
shows the error histogram of MRR, confirming an overfitting model. As discussed earlier, overfitting is identified when a model fits well for the training data and is inaccurate for validation and test data. This histogram depicts a bar representing the training data present in close proximity to the zero-error line, while the height of the bar is 17, which is the same as the samples considered for training. Hence, all the training data are well fit for the model; however, among the eight-remaining data, only two from validation (green color bins) and one from testing (red color bins) possess an error close to the zero line, that is, bins in red and blue colors present above the training data bar at the zero-error line, while one test bin is present close to the zero-error line. Moreover, three bins on the left and two bins on the right side seem to be highly unpredictable, indicating that the model is unable to accurately forecast the remaining six data samples. A similar overfitting phenomenon is observed for SR and TWR samples, as the training data bar heights of 17 and 15 were very close to the zero-error line, respectively. Further, the validation and testing data in SR and TWR failed to achieve errors at par with the training data, as shown in .
This overfitting phenomenon also resembles in the performance plots of all three response variables. exhibits a common behavior of a decrease in the error of the training data with an increase in epochs. However, the testing and validation lines failed to follow the training error curve, that is, no significant drop in error value was observed beyond six epochs in MRR (), beyond four epochs in SR (), and five epochs in TWR (). The best validation performance, that is, the least possible error, was achieved for the validation data at 16, 7, and 6 epochs for MRR, SR and TWR respectively. In summary, error histograms, error performance plots, and best-fit curves reveal a marginal overfitting ANN model.
Figure 12. Comparison of testing and validation error performance with training error for MRR (M), SR (S) and TWR (T).

Lee and Lai study (Lee and Lai, Citation2021) on developing intelligent system to improve EDM efficiency using ANN showed an accuracy of 96.93% when 750 testing datasets. Therefore, it is quite common to see over-fitted models as several unavoidable factors, such as the presence of outliers, insufficient data, and the presence of noise, are typical in real data, irrespective of the size of the datasets considered for validation and testing. In our case, the 25 datasets obtained from design of experiments L25 model seem to be insufficient for ANN model to grasp all the behavior features, and every error histogram depicts bins at extreme farther ends from the zero-error line, indicating the presence of outliers in the data. The raw data obtained from EDM are provided to ANN without eliminating outliers, which further reduces the availability of datasets, making ANN model to extract the behavior of independent variables on responses. This may have caused overfitting of ANN model. Although overfitting was observed, the overall R values from were sufficiently high to deploy the ANN models to forecast MRR, SR and TWR. lists the predicted responses obtained from ANN model.
Table 14. Predicted responses from ANN models (E means Experimental and A means ANN).
4.2.3. Cross validation
Cross-validation of accuracy achieved among mathematical and neurological models is represented in as obtained by calculating the average error of 25 datasets obtained by either of the models. MRR had an accuracy of 86.4% with mathematical model and 93.72% with ANN model. For SR, the accuracy values were 94.84% and 96.85% with Mathematical and ANN models, respectively. Cross-validation of mathematical and artificial neural network models thus illustrates that ANN models are superior in forecasting responses when there exists a nonlinear relation between independent variables and response variables. Although the cross-validation of TWR shows marginal superiority of the mathematical model over ANN, the ANN models outperform the mathematical model in terms of overall performance.
5. Conclusion
Surface quality significantly affects the fatigue strength of aerospace components. Al/GNP high-specific-strength composites fabricated via the hybrid approach were machined by EDM to study the surface quality along with MRR and TWR response variables. In a short note
Aluminum composites embedded with 1.5 wt. % GNPs that are noted to achieve superior strength are selected for the EDM process with varying machining parameters of current, pulse on-time, pulse off-time and flushing pressure.
ANOVA analysis performed on the L25 orthogonal array illustrated that Ton greatly impacted the surface finish, while current significantly influenced TWR and MRR.
Mathematical nonlinear quadratic models have been observed to achieve superior accuracy over linear models. R2 values of 93.8% obtained from nonlinear SR model demand better forecast model.
The ANN model was developed individually for SR, MRR and TWR using the MATLAB software. Among the various neuron probabilities studied, five neurons in a single layer achieved prominent R values for TWR and MRR. However, 10 neurons in the hidden layer possessed the best R value in the case of SR.
Error histograms, error performance curves, and best fit plots conclusively illustrated the development of a best fit model for all the responses, achieving an accuracy of 94% and above.
Although a marginal overfit phenomenon is observed in neurological models, high prediction accuracies of 94% and above, along with overall R values of 96% and above, demonstrate the efficacy of the neurological models in forecasting response variables.
Author contributions
Tarun Kumar Kotteda performed the experiments, collected the data, and wrote the original draft; Manoj Kumar defined the methodology and worked on the software; Pramod Kumar supervised the work; Ajay Gupta reviewed and edited the manuscript; all authors read and approved the final manuscript.
Disclosure statement
No potential conflict of interest was reported by the author(s).
Data availability statement
Data sharing is not applicable to this article, as no datasets were generated or analyzed during the current study.
Additional information
Funding
Notes on contributors
Tarun Kumar Kotteda
Kotteda Tarun Kumar is a Research Scholar in the Department of Mechanical engineering at Dr B. R. Ambedkar National Institute of Technology, Jalandhar, India. He received his M.Tech. degree in Thermal Engineering from Jawaharlal Nehru Technological University, Kakinada, Andhra Pradesh, India. His areas of interest are Characterization of materials, Composites, Unconventional machining, Optimization and Alternative fuels. He had published 15 research papers in various International Journals and conferences to his credit. He had received gold medal from National Design Research Forum, Bangalore in the year 2018 for best project design in Mechanical Engineering Stream.
Manoj Kumar
Dr. Manoj Kumar is an Assistant Professor in the Department of Mechanical Engineering at Dr B. R. Ambedkar National Institute of Technology, Jalandhar, India. He received his M.Tech. degree in Design Engineering from Motilal Nehru National Institute of Technology, Allahabad. He obtained his Ph.D. degree from I.I.T Kanpur. His areas of interest are Solid Mechanics, Ductile Fracture: Continuum Damage Mechanics Model, Dynamic Fracture Mechanics, High Strain Rate Behavior, Large Deformation Elasto-Plastic Impact/Contact Problems, Finite Element Method and Computer-Aided Design. He had published more than 30 research papers in various International/National Journals and conferences.
Pramod Kumar
Dr. Pramod Kumar received his M.Tech. degree in Computer Integrated Design and Manufacturing from National Institute of Technology, Jamshedpur. He obtained his Ph.D. degree in Mechanical Engineering from Dr B. R. Ambedkar National Institute of Technology, Jalandhar, India. His areas of interest are Renewable Energy and Composite Materials. Currently he is serving as Professor in the Department of Mechanical Engineering at Dr B. R. Ambedkar National Institute of Technology, Jalandhar, India. He had published more than 40 research papers in various International/National Journals and conferences.
Ajay Gupta
Dr. Ajay Gupta received his M.Tech. and Ph.D. degree in Industrial Engineering from Dr B. R. Ambedkar National Institute of Technology, Jalandhar, India. His areas of interest are Operations Management, Materials Management and Lean Manufacturing. Currently he is serving as Associate Professor in the Department of Industrial and Production Engineering at Dr B. R. Ambedkar National Institute of Technology, Jalandhar, India. He had published more than 25 research papers in various International/National Journals and conferences.
References
- Ammisetty, S., Ammisetti, D., Satyanarayana, K., Chitturi, S., & Naik, N. S. (2021). Optimization of EDM process parameters on machining characteristics of sic and graphene reinforced Al 6061-T6nano-Composites. IOP Conference Series: Materials Science and Engineering, 1112(1), 012017. https://doi.org/10.1088/1757-899X/1112/1/012017
- Balaji, S., Sivakandhan, C., Maniarasan, P., Deepak, D., Senthamarai, K., & Alagarsamy, S. V. (2023). Effect of process parameters on machining behaviour using S/N ratio and ANOVA analysis. Materials Today: Proceedings, 74, 97–104. https://doi.org/10.1016/j.matpr.2022.12.126
- Bédard, F., Jahazi, M., & Songmene, V. (2020). Die-sinking EDM of Al6061-T6: interactions between process parameters, process performance, and surface characteristics. The International Journal of Advanced Manufacturing Technology, 107(1-2), 333–342. https://doi.org/10.1007/s00170-020-05109-z
- Bharti, P. S. (2020). Two-step optimization of electric discharge machining using neural network based approach and TOPSIS. Journal of Interdisciplinary Mathematics, 23(1), 81–96. https://doi.org/10.1080/09720502.2020.1741222
- Bhat, A., Budholiya, S., Aravind Raj, S., Sultan, M. T. H., Hui, D., Md Shah, A. U., & Safri, S. N. A. (2021). Review on nanocomposites based on aerospace applications. Nanotechnology Reviews, 10(1), 237–253. https://doi.org/10.1515/ntrev-2021-0018
- Bisht, A., Srivastava, M., Kumar, R. M., Lahiri, I., & Lahiri, D. (2017). Strengthening mechanism in graphene nanoplatelets reinforced aluminum composite fabricated through spark plasma sintering. Materials Science and Engineering: A, 695, 20–28. https://doi.org/10.1016/j.msea.2017.04.009
- Çakiroğlu, R. (2022). Analysis of EDM machining parameters for keyway on Ti-6Al-4V alloy and modelling by artificial neural network and regression analysis methods. Sādhanā, 47(3), 150. https://doi.org/10.1007/s12046-022-01926-y
- Chakraborty, S., Dey, V., & Ghosh, S. K. (2015). A review on the use of dielectric fluids and their effects in electrical discharge machining characteristics. Precision Engineering, 40, 1–6. https://doi.org/10.1016/j.precisioneng.2014.11.003
- Chauhan, P., Saloda, M. A., Nandwana, B. P., & Jindal, S. (2022). Parametric study on stainless steel 316L by die sinking EDM for biomedical application. In Proceedings of the International Conference on Industrial and Manufacturing Systems (CIMS-2020) Optimization in Industrial and Manufacturing Systems and Applications (pp. 215–230). Springer International Publishing.
- Chawla, K. K., & Chawla, N. (2014). Metal matrix composites: automotive applications. Encyclopedia of Automotive Engineering, https://doi.org/10.1002/9781118354179.auto279
- Chekuri, R. B. R., Eshwar, D., Kotteda, T. K., & Varma, R. S. (2022). Experimental and thermal investigation on die-sinking EDM using FEM and multi-objective optimization using WOA-CS. Sustainable Energy Technologies and Assessments, 50, 101860. https://doi.org/10.1016/j.seta.2021.101860
- Debnath, S., Rai, R. N., & Sastry, G. R. K. (2018). A study of multiple regression analysis on die sinking edm machining of ex-situ developed Al-4.5 cu-SiC composite. Materials Today: Proceedings, 5(2), 5195–5201. https://doi.org/10.1016/j.matpr.2017.12.101
- Garg, P., Jamwal, A., Kumar, D., Sadasivuni, K. K., Hussain, C. M., & Gupta, P. (2019). Advance research progresses in aluminium matrix composites: manufacturing & applications. Journal of Materials Research and Technology, 8(5), 4924–4939. https://doi.org/10.1016/j.jmrt.2019.06.028
- Ghazanlou, S. I., & Eghbali, B. (2021). Fabrication and characterization of GNPs and CNTs reinforced Al7075 matrix composites through the stir casting process. International Journal of Minerals, Metallurgy and Materials, 28(7), 1204–1214. https://doi.org/10.1007/s12613-020-2101-5
- Ho, K. H., & Newman, S. T. (2003). State of the art electrical discharge machining (EDM). International Journal of Machine Tools and Manufacture, 43(13), 1287–1300. https://doi.org/10.1016/S0890-6955(03)00162-7
- Kotteda, T. K., Eshwar, D., Balakrishna, G., Kuchampudi, S. V., Prasad, B. D., & Sadasivam, S. (2022). Experimental Investigation on Metal Matrix Nanocomposite: Aluminium Alloy 6061 and 7075 with SiC and Fly Ash. Journal of Nanomaterials, 2022, 1–14. https://doi.org/10.1155/2022/8368934
- Kotteda, T. K., Kumar, M., & Kumar, P. (2023). Experimental insights and micrographical investigation on graphene nanoplatelet–reinforced aluminum cast composites. The International Journal of Advanced Manufacturing Technology, (in press). https://doi.org/10.1007/s00170-023-12270-8
- Kotteda, T. K., Kumar, M., Kumar, P., & Chekuri, R. B. R. (2022). Metal matrix nanocomposites: future scope in the fabrication and machining techniques. The International Journal of Advanced Manufacturing Technology, (in press). https://doi.org/10.1007/s00170-022-09847-0
- Kumar, M. S., Begum, S. R., & Vasumathi, M. (2019). Influence of stir casting parameters on particle distribution in metal matrix composites using stir casting process. Materials Research Express, 6(10), 1065d4. https://doi.org/10.1088/2053-1591/ab4045
- Laxman, J., & Raj, K. G. (2015). Mathematical modeling and analysis of EDM process parameters based on Taguchi design of experiments. In Journal of Physics: Conference Series, 662(1), 012025. November)(IOP Publishing. https://doi.org/10.1088/1742-6596/662/1/012025
- Lee, C. H., & Lai, T. S. (2021). An intelligent system for improving electric discharge machining efficiency using artificial neural network and adaptive control of debris removal operations. IEEE Access. 9, 75302–75312. https://doi.org/10.1109/ACCESS.2021.3080297
- Lin, Y. C., Yan, B. H., & Huang, F. Y. (2001). Surface improvement using a combination of electrical discharge machining with ball burnish machining based on the Taguchi method. The International Journal of Advanced Manufacturing Technology, 18(9), 673–682. https://doi.org/10.1007/s001700170028
- Naik, S., Das, S. R., Dhupal, D., & Khatua, A. K. (2022). Electrical discharge machining of engineered Al-22% SiC metal matrix composite: surface roughness analysis, optimization, economic analysis, and sustainability assessment. Process Integration and Optimization for Sustainability, 6(2), 223–251. https://doi.org/10.1007/s41660-021-00207-1
- Okewu, E., Adewole, P., Misra, S., Maskeliunas, R., & Damasevicius, R. (2021). Artificial neural networks for educational data mining in higher education: A systematic literature review. Applied Artificial Intelligence, 35(13), 983–1021. https://doi.org/10.1080/08839514.2021.1922847
- Palanisamy, D., Devaraju, A., Manikandan, N., Balasubramanian, K., & Arulkirubakaran, D. (2020). Experimental investigation and optimization of process parameters in EDM of aluminium metal matrix composites. Materials Today: Proceedings, 22, 525–530. https://doi.org/10.1016/j.matpr.2019.08.145
- Paswan, K., Pramanik, A., Chattopadhyaya, S., & Basak, A. K. (2020). A novel approach towards sustainable electrical discharge machining of metal matrix composites (MMCs). The International Journal of Advanced Manufacturing Technology, 106(3-4), 1477–1486. https://doi.org/10.1007/s00170-019-04816-6
- Radhika, N., Sudhamshu, A. R., & Chandran, G. K. (2014). Optimization of electrical discharge machining parameters of aluminium hybrid composites using Taguchi method. Journal of Engineering Science and Technology, 9(4), 502–512.
- Rahman, O. A., Sribalaji, M., Mukherjee, B., Laha, T., & Keshri, A. K. (2018). Synergistic effect of hybrid carbon nanotube and graphene nanoplatelets reinforcement on processing, microstructure, interfacial stress and mechanical properties of Al2O3 nanocomposites. Ceramics International, 44(2), 2109–2122. https://doi.org/10.1016/j.ceramint.2017.10.160
- Raju, K. S. R., Raju, V. R., Raju, P. R. M., & Ghosal, P. (2015). Launching particle to constant reinforcement ratio as a parameter for improving the nanoreinforcement distribution and tensile strength of aluminum nanometal matrix composites: Paper presented at “International Conference on Advances in Design & Manufacturing” (ICAD&M14), 5–7 December 2014, National Institute of Technology, Tiruchirappalli, Tamil Nadu, India. International Journal of Materials Research, 106(8), 909–914. https://doi.org/10.3139/146.111246
- Raju, K. S. R., Raju, V. R., Raju, P. R. M., Rajesh, S., & Partha, G. (2016). Enhancement of the mechanical properties of an aluminum metal matrix nanocomposite by the hybridization technique. Journal of Materials Research and Technology, 5(3), 241–249. https://doi.org/10.1016/j.jmrt.2015.11.005
- Sahoo, A. K., Panda, A., Nayak, B. B., Kumar, R., Das, R. K., & Nayak, R. K. (2021). Machinability model and multi-response optimisation of process parameters through regression and utility concept. International Journal of Process Management and Benchmarking, 11(3), 390–414. https://doi.org/10.1504/IJPMB.2021.115009
- Sarker, I. H. (2021). Deep learning: a comprehensive overview on techniques, taxonomy, applications and research directions. SN Computer Science, 2(6), 420. https://doi.org/10.1007/s42979-021-00815-1
- Sharma, A., Vasudevan, B., Sujith, R., Kotkunde, N., Suresh, K., & Gupta, A. K. (2019). Effect of graphene nanoplatelets on the mechanical properties of aluminium metal matrix composite. Materials Today: Proceedings, 18, 2461–2467. https://doi.org/10.1016/j.matpr.2019.07.095
- Shyn, C. S., Rajesh, R., & Dev Anand, M. (2023). Optimization-based hybrid intelligent model for decision making on Electrical Discharge Machining (EDM) process of A6061/6%B4C and A6061/9%SiC composite materials. Cybernetics and Systems, 54(6), 836–858. https://doi.org/10.1080/01969722.2022.2110685
- Singh, D. P., Mishra, S., Yadav, S. K. S., Porwal, R. K., & Singh, V. (2023). Comparative analysis and optimization of thermoelectric machining of alumina and silicon carbide-reinforced aluminum metal matrix composites using different electrodes. Journal of Advanced Manufacturing Systems, 22(02), 373–401. https://doi.org/10.1142/S0219686723500191
- Singh, S. (2012). Optimization of machining characteristics in electric discharge machining of 6061Al/Al 2 O 3 p/20P composites by grey relational analysis. The International Journal of Advanced Manufacturing Technology, 63(9-12), 1191–1202. https://doi.org/10.1007/s00170-012-3984-8
- Sita Rama Raju, K., Rama Murthy Raju, P., Rajesh, S., Raju, V. R., & Ghosal, P. (2016). An experimental and micrographical investigation on aluminum nano metal matrix composites. Journal of Composite Materials, 50(26), 3627–3641. https://doi.org/10.1177/0021998315623624
- Uğur, A., Nas, E., & Gökkaya, H. (2020). Investigation of the machinability of SiC reinforced MMC materials produced by molten metal stirring and conventional casting technique in die-sinking electrical discharge machine. International Journal of Mechanical Sciences, 186, 105875. https://doi.org/10.1016/j.ijmecsci.2020.105875
- Zhang, D. D., He, X. Y., Liu, Y., Li Gao, Y., & Geng, R. (2022). Nanoparticles reinforced Al-matrix composites fabricated by combination of pre-distribution and deformation: a review. Materials Science and Technology, 38(13), 883–901. https://doi.org/10.1080/02670836.2022.2068272