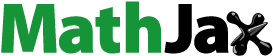
Abstract
Customer demand fulfilment is essential for production systems in the highly competitive market environment. Because of that fact, a reliable production line is necessary for timely demand fulfilment, and the system must maintain an appropriate spare parts inventory to run the production line smoothly. This research aims to study the impact of spare part inventory policy on production line availability and demand fulfilment. This paper considers a steel pipe manufacturing company that runs continuously. This company uses a continuous raw material flow and produces discrete finished items. The company’s production system is multi-state with unique characteristics in which the machines can be operated at partial capacity under certain failures or disturbances. Hence, three states of the production system, namely fail, working under failure, and success (denoted as 0, 0.5, and 1, respectively) are considered. In addition, the company follows s,S policy for its spare parts inventory system. To deal with this problem, a discrete-event simulation approach is developed as the problem environment is complex with interdependency and variability. A multi-level reliability block diagram is also used to build the production system model. The proposed simulation model is run for different scenarios with either cost or performance as the objective measure. Based on the analysis of results, the proposed approach can improve the current operation in terms of both production line availability and demand fulfilment.
REVIEWING EDITOR:
1. Introduction
In this paper, we study the problem of determining spare part inventory policy considering machine availability and demand fulfillment in one of Indonesia’s largest metal pipe manufacturing industries. This particular company we observe is unique due to its production system setting, which allows operating while experiencing failures.
When it comes to the performance of a production system, reliability and availability are related to each other. Reliability is defined as the probability of a system performing the expected function during a certain period that provides the overall performance of a system (Xu et al., Citation2017). In the other hand, availability describes an ability of system to be in a state to perform as and when needed under certain circumstances (Dhillon, Citation2006; Melani et al., Citation2022). Hence, availability can be calculated by comparing the uptime duration of a machine operation and the duration of operation of the system (Barringer, Citation1997) with the help of metrics called mean time between failure (MTBF) and mean time to failure (MTTF) (Ireson et al., Citation1996). Since a system may consist of more than one component, therefore we can utilize a reliability block diagram (RBD) to evaluate the overall system’s availability or reliability by considering the way how elements are arranged within the system (Elsayed, Citation2012).
In a production system setting running continuously, it is expected to have a high level of availability. By doing that, the production process can maximize its throughput so that most demands are satisfied. A spare parts inventory is necessary to ensure that the production system is at its high level of availability. Spare parts are stockpiled to accommodate the uncertainty of when the failure occurs. However, keeping too many inventories is not good for a company. Therefore, determining the appropriate spare part inventory policy and strategy which guarantees a high service level of spare parts with minimum inventory cost is a challenging problem in practice (Tersine, Citation1994). Additionally, the other strategy that could be implemented to ensure that spare parts will always be available just in time is by using a 3D printing technology (Lolli et al., Citation2022; Xu et al., Citation2021). In this case, it is possible to produce and procure spare parts on demand, hence the resilience of the production system could be improved as expected. However, under certain circumstances, even with the latest technology, not all components or spare parts can be produced in-house by 3D printing technology (Manda et al., Citation2018; Sathish et al., Citation2018). Hence, procurement of some spare parts has to rely on conventional provisioning by ordering from suppliers or vendors.
This paper uses availability and demand fulfillment rates as system metrics and objectives for the study. This paper is an extension of studies conducted by Salsabila et al. (Citation2019) and Alrabghi et al. (Citation2017) which aimed to maximize availability, reliability, and production throughput. Other similar studies related to availability and reliability have also been carried out for various objectives such as system reliability modeling (Jang & Park, Citation2017; Sharda & Bury, Citation2008), reliability model review (Rao & Naikan, Citation2016), system reliability improvement (Ram & Manglik, Citation2016), maintenance strategy determination that boost system reliability (Zhou et al., Citation2018) as well as minimizing maintenance cost (Alrabghi et al., Citation2017; Alrabghi & Tiwari, Citation2016; Golbasi & Turan, Citation2020). As an extension of Salsabila et al. (Citation2019) and Alrabghi et al. (Citation2017), this paper incorporates not only production throughput but also incoming demand. Since both demand and production throughput are stochastic and related to each other, it is wise to consider both aspects in this paper. If only production throughput is picked as metric without considering the incoming demand, it is difficult to guarantee that improved throughput means improved demand being fulfilled since there is unavailable number of demands to compare. Therefore, in this paper, by considering production throughput and demand, another metric is proposed namely demand fulfilment rate. Demand fulfilment rate describes the proportion of total demand in a particular period that can be satisfied from on-hand inventory (Snyder & Shen, Citation2011). In this case, we assume that the production throughput goes directly into inventory and is used to fulfil customer demand.
This paper also observed a company that runs in a continuous process flow, continuous raw material but producing a discrete product. This paper differs from other papers which follow only one specific characteristic either continuous production process and continuous product (Alrabghi et al., Citation2017; Golbasi & Turan, Citation2020; Kolawole et al., Citation2019; Olukanni et al., Citation2018; Salsabila & Siswanto, Citation2019; Salsabila et al., Citation2019; Sharda & Bury, Citation2008; Wakiru et al., Citation2019) or discrete production process and discrete product (Alrabghi et al., Citation2017; Chang et al., Citation2017, Citation2019; Chen et al., Citation2022; Jang & Park, Citation2017; Nnamdi, Citation2018; Zahedi-Hosseini et al., Citation2017; Zhu et al., Citation2020; Zhang et al., Citation2020).
We discovered that not all system availability and reliability studies considered and included spare part inventory systems. Nnamdi (Citation2018) used an analytical approach to spare parts inventory management which minimizes dead stock. On the other hand, Wakiru et al. (Citation2019) considered spare parts inventory to investigate the interaction effect existence between maintenance action and spare parts demand. Some studies also included the spare parts inventory model in the reliability system model as a subject of modification and experimentation by determining the optimal spare part inventory parameter level (Alrabghi & Tiwari, Citation2016; Salsabila & Siswanto, Citation2019; Zhu et al., Citation2020) and the best spare part inventory policy (Bülbül et al., Citation2019; Zahedi-Hosseini et al., Citation2017). In their study, Salsabila and Siswanto (Citation2019) changed only the minimum and maximum inventory level parameter value, sticking to the initial inventory policy, while Zahedi-Hosseini et al. (Citation2017), in their studies, tried various inventory policies to find the best one to implement. In this paper, the author tried to combine what Salsabila and Siswanto (Citation2019) and Zahedi-Hosseini et al. (Citation2017) had done, which is changing the inventory parameter value as well as experimenting with a different type of inventory policy.
Most papers discussing reliability and availability assume only two states (success and failure) possible to occur which can lead to inaccurate result (Xu et al., Citation2017). Hence, it is almost necessary to consider more than two states that occur in a system. This paper is an extension of studies conducted by Dendauw et al. (Citation2021), Chang et al. (Citation2017, Citation2019), Chen et al. (Citation2022), and Zhang et al. (Citation2020) that already took multi-state system into consideration. This paper attempts to replicate what Chang et al. (Citation2017) had carried out in their research by adapting the condition in which production capacity is heavily affected by multi-state system. However, those studies had not considered the utilization of multi-level RBD yet to model the production system. It is also interesting to report that Salsabila and Siswanto (Citation2019) have already modeled a production system using multi-level RBD. Multi-level RBD helps break down a system into smaller subsystems to inspect and determine the system’s reliability. Unfortunately, Salsabila and Siswanto (Citation2019) still considered a two-states system in their studies. Hence, to filling this gap, this paper tried to extend research of Chang et al. (Citation2017) and Salsabila and Siswanto (Citation2019) by incorporating multi-states (more than two states) system which affect the production capacity as well as benefiting multi-level RBD to model the production system.
There are several approaches used in studying system availability and reliability. An approach such as exact optimization (Zhu et al., Citation2020), heuristic (Bülbül et al., Citation2019; Chang et al., Citation2017, Citation2019; Chen et al., Citation2022; Dendauw et al., Citation2021; Ram & Manglik, Citation2016; Zhou et al., Citation2018; Zhang et al., Citation2020), and metaheuristic (Olukanni et al., Citation2018) are utilized to achieve each of study objectives. However, most studies related to the availability and reliability of the system took advantage of simulation as an approach. Zahedi-Hosseini et al. (Citation2017), Jang and Park (Citation2017), Sharda and Bury (Citation2008), Salsabila et al. (Citation2019), Alrabghi et al. (Citation2017), Alrabghi and Tiwari (Citation2016), Salsabila and Siswanto (Citation2019), Wakiru et al. (Citation2019), and Golbasi and Turan (Citation2020) used a specific type of simulation called discrete-event simulation (DES) since DES is capable of modeling system with the high level of complexity caused by uncertainty and interdependency among system components. Simulation, especially DES, in this case, allows faster processes in modeling and result generation. Additionally, in simulation, it is possible to perform experimentation without interrupting or interfering with the real system. In some cases, it is possible to experiment directly on the real system if the size of the system, cost, and risk of experimentation are relatively small. However, when the system is large and complex, and the cost and risk of experiments are enormous, the model should be established, and simulation is recommended to be utilized (Harrell et al., Citation2004; Kelton et al., Citation2015). Since the system we will model contains random input, the simulation approach is more suitable to exploit (Law, Citation2013). In this paper, DES is used to model a multi-state production system constructed with basis of multi-level RBD, the incoming demand to be compared with throughput, and the spare part inventory system.
To sum up, this paper aims to describe a new system reliability and availability study to improve the current availability and demand fulfillment rate with the best spare parts inventory policy to implement with the possible lowest inventory cost. Compared to previous studies, this paper includes new conditions and complexity, such as (1) inclusion of incoming demand to calculate demand fulfillment rate, (2) continuous production flow with discrete items produced, (3) modification of spare parts inventory policy by altering the parameter value and the entire policy, (4) production system with three observable states: success, fail, working under failure (denoted as 1, 0, and 0.5 consecutively which later affecting production capacity), and (5) utilization of multi-level RBD to model the production system. To show the position of this paper compared to previous studies, is provided to present the summary.
Table 1. Comparison of current research with previous research.
The remaining part of this paper is organized as follows: Section 2 describes the state of the problem. Section 3 explains the model development, data collection and analysis, and verification and validation process. Section 4 analyzes the generated results, designs experimentation, and tests solution robustness through sensitivity analysis. Section 5 concludes this paper by offering a few suggestions for future research.
2. Problem description
This study considers a real-world problem from an Indonesia’s largest metal pipe manufacturers. The business operation is facilitated by seven operating plants spread across Indonesia. Most plants run production continuously, 24 hours a day, seven days a week. The production runs continuously unless there is planned downtime. One of the main plants, Plant 1, is situated in one of Indonesia’s major cities. This unit implements the high-frequency welding (HFW) technique and demonstrates a production capacity of 6.250 MT as of 2017. This unit exhibits five functioning production lines capable of producing pipe with various diameters ranging from ¼“ to 8”. Unit 1 incorporates service facilities such as galvanizing, slitting, shearing, and recutting. The production process is identical among lines. After being cut using a slitter, the steel coil is uncoiled and accumulated into smaller sizes or batches of steel stripe as work-in-process (WIP) material. The strip then enters the forming process, forming into a cylindrical shape. Next, the tube is welded along its length and cooled subsequently. Ensuring no hole on the weld, eddy current machine is utilized to perform such tasks. The following process is performed to size the pipe according to the designed diameter and cut the pipe based on the determined length. Post-production processes such as end-facing, hydro testing, or threading are performed when necessary.
Plant 1 incorporates five operating production lines; the most important and frequently used line is called R1 that consists of an uncoiling machine, accumulator, forming machine, welding machine, sizing machine, cutting machine, and conveyor. Despite being the most crucial line among the existing lines, R1 demonstrates a higher probability of failing. According to company data, three main failure contributors of this line arise from the cutting machine, accumulator, and welding machine, with the value of 41%, 24%, and 18%, respectively. These machines must be inspected regularly to ensure appropriate operation of the line.
Upon line failure, spare part replacement is a common practice for recovery. In this plant, the spare part is categorized into two types: the consumable spare parts provided by the Department of Spare Part Storage (PBS) and the repairable spare parts fabricated by the Department of Maintenance in their workshop by refurbishing defective spare parts. Mainly consumable spare parts are required during maintenance in this case. Currently, PBS implements s,S inventory policy, where s denotes the minimum stock calculated as the average spare part usage over time. In contrast, S means maximum stock, which is twice the minimum stock. However, this policy is not working well. There are many situations where the necessary parts are unavailable for replacement, resulting in higher downtime. In some other situations, some machines may run with some technical trouble at a less-than-ideal capacity. That means, the machine can be operated at reduced capacity until the spare part is available. Unfortunately, the company cannot to disclose their data on capacity reduction due to partial failure. Since the success condition is usually denoted as 1 (full production capacity) and 0 denotes failure condition (the machine does not work), we assume that the machine that runs under partial failure condition will have half its designated capacity to represent the average capacity reduction. Therefore, this is why we denote this type of situation as 0.5. Hence, we end up with production system modelling with three states, namely 0, 0.5, and 1. shows the problem description and element connections within the observed system.
With the situation described above, a study on the determination of an appropriate spare part inventory policy for a production system with three states (success, failure, working under failure) is an important research problem for the given industry. So, this study aims to maximize the availability of the production lines by ensuring the company’s high service level in fulfilling its customer demand.
For this research, we limit our scope by observing only the R1 production line since it frequently experiences failure, as described earlier. We also only consider spare parts provided by PBS. Hence, the ones fabricated by the Department of Maintenance are neglected. We also assume that the inventory system considered in this research is exclusively assigned to R1. This research also does not consider the penalty cost of lost sales resulting from machine breakdown. Besides the scope limitations, several assumptions are also assigned to this research. We assume that there is no operational rule change on both the production and reliability system, operators or manpower to perform activities on the production floor are always available, raw materials are always available to process, and the spare part unit cost is not affected by the time value of money.
3. Research methodology
shows the flow of how this research is performed. This research is started by studying the system being observed. This includes defining system elements, system variables, as well as key performance indicator (KPI). This is followed by data collection and processing. In this step, we are categorizing data into three types: structural, operational, and numerical data. Afterwards, we will develop the model both conceptual and simulation models. Verification and validation of the model must be carried out to ensure that we already build the right model and build the model correctly. Initial output analysis is performed to observe the output of the simulation model and later will be used as basis of designing scenario in experimentation. The result of experiment later will be compared with initial output and check if the result generated from the experiment is better than the existing condition. Lastly, a conclusion is constructed and recommendation for future research will be given.
3.1. Study of system
It is necessary to get a better understanding upon the system that we are going to observe. In this case, there are several points that we are going to study about the system. In this section, we will give detail information about the system elements, system variables, and defined KPI metrics.
3.1.1. System elements
From a simulation point of view, a system consists of some key elements namely entities, activities, resources, and control which define who, what, where, when, and how the entity is processed within the system (Harrell et al., Citation2004). Within the system under this study, the entity is raw material of steel pipe which is steel coil. Activities are defined as the processes of transforming steel coil into steel pipe. The processes start from uncoiling the steel coil to cutting the pipe and moving it to conveyor. Resources of the system are the machines arranged into production line. provides a clear illustration regarding the activities and resources used in this system. Lastly, control of the system is about how the machines are arranged in the production line and how the equipment is arranged within the system. In this case, all machines and equipment are arranged in series.
3.1.2. System variables
The system variables can be divided into three types: decision, response, and state variables (Harrell et al., Citation2004). Decision or independent variable is the variable that can be controlled in terms of its values or characteristics. In this simulation model, the decision variable is defined as the spare part inventory policy to be implemented. It covers the method of review, whether periodically or continuously as well as the implementation of the maximum level inventory (S) or EOQ. The value of s, S, and EOQ are also modified as a part of experimentation.
Response variable, also referred to as the output or dependent variable, is the measurement of system performance in terms of the decision variable. The production system in this case is measured in terms of the production throughput. It is defined as the amount of produced item that fulfills the demand, and it does not include the lost sales owing to late fulfillment or rejection. In addition, the availability of the production line is also measured since it enables understanding the impact of the spare part inventory policy on production line availability.
3.1.3. Key performance indicators
Key performance indicator (KPI) is the measurement of system success in achieving the goals. In this case, the KPI observed within this study is the response variable mentioned at previous part which are production line availability as well as production throughput measured in demand fulfilment rate.
EquationEquation (1)(1)
(1) below shows how to measure demand fulfilment rate which is by dividing the throughput by the demand of corresponding period. EquationEquation (2)
(2)
(2) shows how to obtain the availability of production system. Since the system is arranged by subsystems in series, therefore, the availability rate is obtained by multiplying availability of every subsystem observed.
(1)
(1)
(2)
(2)
Availability rates of uncoiler, accumulator, forming machine, welding machine, sizing machine, cutting machine, and conveyor are denoted as AU, AA, AF, AW, AS, ACu, and ACo, consecutively.
3.2. Data collection and processing
Data are collected through several methods. To obtain primary data, direct observation of real conditions and interviews of certain relevant stakeholders are performed. Observation is performed for enhanced understanding of the real system condition. The interview is conducted for confirmation purposes and acquiring expert judgment. Meanwhile, secondary data are acquired by aggregating company documentation and recording. The types of collected data include structural, operational, and numerical data.
3.2.1. Structural data
Structural data primarily describe the system configuration and identify the entity to be processed within the system and the resource that must process the entity; this includes machine type, production process, and product type. In this case, the entity to be processed is a demand of the pipe manufactured from steel coil. The resources used are machines within the production line. Each machine is divided into several smaller units called equipment. Later, the spare part related to the equipment will be determined as well. In this case, after performing Pareto analysis to determine the most critical spare parts, we arrive at 26 selected spare parts that are used in all equipment. shows the deployment of machines into equipment and the selected spare parts.
Table 2. Deployment of machine into equipment and the related spare parts.
3.2.2. Operational data
Operational data explain the working of the system. The operational data collected includes the process flow, reliability system for determination of the RBD arrangement, and spare part inventory policy implemented by PBS. illustrates the processing of raw material in the form of steel coil to produce end-product which is steel pipe. The process starts from uncoiling the steel coil and accumulating it as the buffer before entering the forming process. In forming process, the coil is shaped into a cylindrical shape, and subsequently, the welding process is entered. The sizing process is essentially the activity through which the pipe is sized according to the diameter. Subsequently, the pipe is cut according to the predetermined length and the conveyor is entered.
Operational data also cover the spare part inventory policy as mentioned before. Spare part inventory policy of this company follows s,S policy. The inventory is reviewed continuously at least once a day up to a maximum of three times a day. There are at least four inventory parameters applied in the policy: reordering point (s), maximum stock (S), and ordering quantity (Q).
The reordering point or s defines the inventory position at which the stock should be replenished, and the order must be placed. S is defined as the maximum inventory threshold, which illustrates the maximum storage capacity of corresponding spare parts. (Q) is determined by subtracting the current inventory level from the maximum quantity. In this research, both s and S values are directly obtained from company data. Meanwhile, Q could be determined by using EquationEquation (3)(3)
(3) as follow.
(3)
(3)
3.2.3. Numerical data
Numerical data provide quantitative data about the system. There are many numerical data for collection, such as production capacity, history, target, operations working shift, inventory parameter, part ordering lead time, and failure history, including the timestamp and type and quantity of the spare part required, failure duration, repair duration, and inventory cost. Most numerical data are obtained from secondary data. shows the inventory parameters of spare parts. The parameters include ROP denoted as s, maximum stock, and ordering lead time (LT).
Table 3. Spare part inventory parameters.
We have already mentioned that we utilize a simulation approach due to the random input of the system. Some numerical data from the system are stochastic, such as failure and repair durations. We identified 73 random inputs consisting of MTBF and MTTR of 23 different equipment, 26 spare part demands, and customers’ demand for finished products.
Once we identified the random input of the system, then fitting distribution analysis is performed to determine its statistical distribution representing the system behavior. According to Law (Citation2013), distribution fitting is required because when a probability distribution is replaced by its mean (because of no fitting approaches), the actual behaviour is not reflected in the simulation process and its generated results. A set of data is fit to the theoretical distribution. For example, the Weibull distribution is better for explaining the mean time between failures (Altiok & Melamed, Citation2007; Elsayed, Citation2012), while the Erlang distribution is more suitable for modelling the mean time to repair (Altiok & Melamed, Citation2007). The distribution fitting result of MTTF and MTTR is shown in . A production demand can also be approached using the normal distribution (Pujawan & Er, Citation2017; Tersine, Citation1994; Waters, Citation2003) expressed as NORMAL (1.13 × 105, 1.84 × 104) with the value generated being rounded to nearest integer.
Table 4. Distribution fitting result for MTTF and MTTR data.
The probability of the spare parts used, and their probable quantity are also determined. Lastly, the likelihood of 0.5 status (half-capacity) occurrence is assessed using the historical data of maintenance activity. and illustrate these relevant data.
Table 5. Probability of using spare part and quantity required.
Table 6. Probability of half capacity of machines.
3.3. Model development
A model is constructed to simulate the actual system as closely as possible. Constructing a model enables simulating several scenarios toward the ultimate aim. shows the conceptual model illustrating how decision variables interact and affect response variable. From , we can learn that the decision variables which is spare part inventory policy has a direct impact to spare part availability in inventory. The spare part availability will also determine whether a maintenance action due to failure could be carried out. It also dictates if the production line system could run and available to not. The production line system availability will be measured as well as its throughput to satisfy received demand.
Accordingly, a simulation model is developed comprising several sub-models to conduct experiments based on various predetermined scenarios to determine a suitable spare part inventory policy, such that the production line availability remains high and demand fulfillment improves. above shows the overview of the simulation model developed. There are five sub-models within the main model. Production is the primary key to simulation. The production process occurs across several machines, whose arrangement is modeled into RBD. This RBD model communicates the functioning of the series arrangement as well as the triggering and resolution of failure. To resolve the failure, it necessary to inspect if the maintenance action requires any spare part. Thus, the sub-model of failure is related to the sub-model of spare part inventory. In the following discussion, detailed information regarding the sub-models is provided. This section also discusses the definition of system variables; the acquisition, processing, and analysis of data; and the validation performance procedure.
3.3.1. Sub-model 1: line reliability block diagram
This sub-model shows the arrangement of components within the production line into an RBD, in a series, parallel, or mixed arrangement. The R1 arrangement is divided into several RBD levels, starting from level 0 to level 2. The R1 line is defined as level 0 RBD, whereas machines within R1 are categorized into level 1 RBD. The machine constitutes several pieces of equipment, later described as level 2 RBD.
Detailed information pertaining to dividing the RBD level is shown in . Level 1 RBD consists of seven observable machines: uncoiler, accumulator, forming, welding, sizing, cutting, and conveyor machine. These seven machines are arranged in series shown that those seven machines are arranged in a straight line. In this kind of arrangement, the entire system fails when at least one machine fails. Furthermore, when at least one machine experiences reduced capacity due to failure, the line output is also reduced. Level 2 RBD consists of equipment comprising the machine. The equipment of the seven machines is also arranged in series. Take uncoiler for an example. Uncoiler has two observable equipment which are brake system and hoist crane. These types of equipment are arranged in straight line implying that equipment inside uncoiler is arranged in series. For another example, let’s look at forming machine. This machine consists of at least four selected equipment namely stand roll, gearbox oil pump, oil pump, and forming gearbox. Similar to equipment inside uncoiler, that four equipment are also arranged in series.
3.3.2. Sub-model 2: failure
A failure sub-model is established to model the failure logic of the equipment and link it to the spare part inventory. This sub-model interacts with level 2 RBD or equipment level. The logic behind this sub-model is explained in . A failure event expressed by MTTF is defined as the input of this sub-model. A regular review is conducted to check if the equipment works suitably. If the equipment experiences total failure, then the status of 0 is assigned to the said item, implying zero production capacity or functional inability. Furthermore, if the equipment can still run under the failure condition, we assign a status of 0.5, implying the assumption of the production capacity to be set to half of the designed capacity.
The next step is to check if a particular spare part is required to perform reparation. Reparation can be conducted immediately if no spare part is required. However, spare part type and quantity identification are necessary if parts are required. After the quantity of parts is identified, the stock available in the PBS must be checked. If the existing amount is less than that required, a part is ordered, and the arrival of parts is anticipated according to the predetermined lead time. Otherwise, the stock is reduced to as many parts as required to perform reparation.
Reparation occurs over a duration defined as MTTR. Upon the completion of reparation, equipment status needs to be updated to 1, indicating that the equipment functions suitably at full capacity. As mentioned previously that this sub-model interacts with level 2 RBD, therefore this sub-model is developed for all 26 equipment.
3.3.3. Sub-model 3: series arrangement failure
Since all sub-systems are arranged in series, a sub-model defining the underlying logic of series arrangement becomes necessary. presents the logic of failure occurrence in a system arranged in series and restoration of its status to 1.
A review must be performed to check the status of the system. If no component within the system or sub-system fails, the status of the unit remains 1, implying effective operation. However, if at least one component fails, the entire system is considered to have failed. However, in this case, there is an additional condition that involves checking whether the component fails completely; if such failure is observed, then the status of the component is set to 0. However, if the component is still functional, the status is set to 0.5, defining that the component functions only at its half capacity. The failing component must be repaired to restore its status to 1.
Since all machines and equipment follow series arrangement, therefore this sub-model applies to all seven machines and 26 equipment when developing the simulation model.
3.3.4. Sub-model 4: spare part inventory
Inventory of spare parts becomes critical in this study. Without the parts being available in stock, reparation or maintenance action is difficult to perform, leading to disturbed production activity. Currently, the company applies s,S inventory policy, where s denotes their minimum inventory level or reorder point, and S represents the maximum inventory level. PBS places a part order whenever the inventory level falls on or below s. In this study, developing a sub-model explaining the operation of spare part inventory policy is essential. depicts the logic underlying this sub-model.
The inventory should be reviewed regularly to check the level. As mentioned, when the inventory level reaches on or below s, an order should be placed to replenish the stock. The order amount is also determined at this step. Implementing s,S policy, the company calculates the quantity ordered (Q). The order quantity is calculated using EquationEquation (3)(3)
(3) .
Once the order is placed, arrival of the parts within the agreed lead time with the supplier is anticipated. Inventory level update is performed when the stock needs to be replenished. As for this model, the logic will be applied for all 26 observed spare parts.
3.3.5. Sub-model 5: production
A production sub-model is developed to explain the delay due to reparation. The sub-model logic is shown in . The failure event represented by MTTF is used as the input of this sub-model. Before production activity, it is necessary to check if the system’s status is ON. After ensuring that the entire system functions effectively, the production process begins.
In the production process, failure cannot be completely avoided. If failure occurs and is resolved outside the production activity, such as scheduled maintenance or overhaul, the production activity continues without any issue. However, it is problematic if the failure occurs during the production process. Under such circumstances, the delayed time due to reparation must be calculated. The component of this time could be the reparation duration and the lead time owing to anticipation of the arrival of the spare part if there is insufficient or no available stock.
To determine the type of delay and calculate the delay time, the failure combination must be considered. This relates to when and where failure occurs during the production process. The study conducted by Salsabila et al. (Citation2019) is referred to model this combination. There are three failure combinations applicable to this case. illustrates the point at which failure occurs in terms of the production range time. The calculation of the delay time for every occurrence of failure is elaborated below.
Failure Combination 1
Failure occurs outside the production time. Therefore, the failure does not affect production, and there is no delay time added.
Failure Combination 2
Failure is initiated against ongoing production, and failure progresses until the production process is complete. Therefore, delay time is identified as D2 and calculated as follows:.
(4)
(4)
D2 defined as delay time added to production time, T2 is production finish time, and F1 is starting repair time.
Failure Combination 3
Failure starts and resolves during the production process. Delay time is identified as D1 and calculated as follows:.
(5)
(5)
D1 is defined as delay time added to production time, F1 is starting repair time, and F2 is finish repair time.
In a simulation, this model will be modelled for every machine. Since we have seven observable machines under the production line, thus this model is replicated seven times. Lastly, the production throughput is recorded, and demand fulfilment rate is calculated in percentage.
3.4. Verification, replication, and validation
Verification is one of the most important steps when developing a model, particularly a simulation model. Verification is a process of determining whether a model operates as expected or at least functions (Harrell et al., Citation2004). Verification is mainly concerned with developing the model correctly by comparing it to its conceptual model (Banks et al., Citation1995). There are two types of verification: syntax error verification and semantic error verification. Syntax error verification is conducted to ensure error-free coding. In contrast, verification of semantic error is conducted to check if the model is already in line with the conceptual model.
In this step, the first logic checked is the line status. Since the line machines are arranged in series, whenever at least one machine exhibits the status of 0, the entire line updates its status to 0. If one machine exhibits a status of 0.5, then the line updates its status to 0.5. The second logic checked is the inventory level. When an inventory level falls to or below ROP or there is insufficient stock to perform maintenance, an order must be placed at a certain quantity. The last logic verified is the production system. It is necessary to check if the production line is functional if the status is not zero (either 1 or 0.5 causes the line to be functional).
Since simulation exhibits random input random output (RIRO) behavior, performing simulation only once is not sufficient to represent the actual system. Replication is required, and simulation output cannot generate estimation intervals without it. A parameter of demand fulfillment rate (throughput versus demand) is used as the main output to determine the number of replications. shows the demand fulfillment percentage result from simulation conducted ten times compared to the value of actual system.
Table 7. Simulation result compared to actual system value.
On the first attempt, we simulate 10 replications. With 10 replications, the half-width (hw) for demand fulfilment rate is 3.19%. To determine if 10 replications are enough, we must calculate the expected half-width (hw’). In this case, we desired that the error not exceed 5% of the demand fulfilment rate from the actual system (which is 92.23%), which has a value of 4.61%. From this value, we can conclude that additional experiment replications are not required because the desired hw has been achieved.
Validation is a process of determining whether a model is a meaningful and accurate representation of an actual system (Hoover & Perry, Citation1990). Validation is performed by comparing the simulation output with the system output. Since the samples are independent, Student’s t hypothesis test is performed. Demand fulfillment rate is still used to perform validation. Using 5%, the hypothesis is formulated as follows:
Based on the calculation, the t-stat value is obtained as -1.538. Comparing this value with the t-crit value, it is concluded that the null hypothesis should not be rejected. Therefore, the simulation model is valid and can represent the actual system.
4. Results and discussion
This section analyzes the initial results, performs experiments by designing a scenario, and analyzes model sensitivity.
4.1. Existing condition
The simulation model is run based on 10 replications over a length of 10 months for each replication, in order to obtain an apple-to-apple comparison with the actual data obtained. Note that we set the warm-up period to 7 days while the actual simulation period is 304 days (equal to 10 months). Since simulation follows RIRO behavior, the result of one replication to each other should be different. Hence, the result of both availability and demand fulfilment rate should be expressed not only by average (mean) but also variance.
After simulating such a configuration, we obtain the result shown in , where the line availability is averaging 0.9268 with variance of 0.00028. This implies that the production line is available for 92.68% of the time, indicating that the remaining 7.32% is spent in downtime. Furthermore, on average, 90.06% demand is fulfilled with variance of 0.00198, implying that only 90.06% of demand is satisfied at the given production rate and availability. At this point, we must also assess 26 selected spare part availability to better understand this scenario.
Table 8. Existing condition result.
We attempt to plot the inventory movement of all spare parts under observation. illustrates examples of the inventory movement plot, showing the ROP, maximum stock, inventory level, and average inventory level. To generate these graphs, we run the simulation only once, but with a time span period of 3 years (1100 days) to capture more phenomena. From this type of graph, we could gain more insight into the mechanism and time point of the inventory level change over time as well as the frequency with which the inventory level falls below ROP, causing inventory stockout. As for inventory movement for SP 2 shown by , it can be learned that for 3 years span, 12 orders take place and 9 of them are happened when stock is zero or below ROP. From , only 2 orders of SP 7 happen for 3 years but all of them happened when stock is below ROP. Next is inventory movement for SP 15 shown by . From the figure, we can learn that all the orders happen when inventory level falls below ROP and even 3 orders happen when stock reaches zero. Last is inventory movement for SP 19 shown by . We can also learn that all of SP 19 orders take place when inventory level reaches zero. We also use a similar plot to illustrate inventory movement for the remaining spare parts and we find the similar event.
Since we are running the simulation with 10 replications therefore, we get the different value spare part availability level for every replication. Hence, we present to show the average availability of those spare parts after running the simulation model 10 times. Most spare parts have high availability, given 15 spare parts (58%) are always available whenever downtime happens given that the availability value is 100%, and seven spare parts (27%) exhibit availability value below 100% but above 97%. Thus, four spare parts with the lowest availability remain: SP 2, SP 7, SP 15, and SP 19, with availability rates of 96.9%, 95.8%, 97.4%, and 92.2%, respectively. Therefore, we select those spare parts as critical spare parts. This phenomenon is caused by the inventory policy as well as the usage. We find that every single usage of corresponding spare part causes the stock to become zero as impact of the maximum inventory level being set. Even when it is replenished to its maximum stock, it becomes zero instantly whenever particular parts are required. Low S value will significantly impact such an event to occur. Therefore, the minimum and maximum stock numbers need correction.
Table 9. Spare parts availability.
4.2. Scenario development
A scenario is established as a part of the experiment to propose an improvement in the output parameter. In designing this scenario, we consider certain conditions to change in terms of the spare part inventory policy. We use the existing inventory parameter value as the basis of designing scenario especially for the spare parts with the lowest availability level. The following conditions must be ensured:
Increase s and S value of SP 2 (s = 3, S = 6),
Increase s and S value of SP 7 (s = 2, S = 4),
Increase s and S value of SP 15 (s = 8, S = 16),
Increase s and S value of SP 19 (s = 3, S = 6),
Increase s by 50% and 80%, and S value of all spare parts,
Implement s,Q policy.
We use a study conducted by Salsabila and Siswanto (Citation2019) as the reference to build our scenario. However, we add a modification in which s,Q inventory policy is added while at Salsabila and Siswanto (Citation2019) did not. To calculate EOQ (Q), EquationEquation (6)(6)
(6) is used (Wilson, Citation1934). C denotes ordering cost, R is the annual demand in units, P is the purchasing cost per unit, and F denotes an annual holding cost as a fraction of unit cost. The result of EOQ calculation is summarized in .
(6)
(6)
Table 10. EOQ (Q) calculation of spare parts.
Based on the combinations, we designed 19 scenarios for experimentation. The scenario result will later be compared to the existing condition output through t-test analysis. The output analysis is performed for all 19 scenarios to determine the scenario results that change significantly. shows the example of the output analysis performed, showing the scenarios that result in insignificant change, while shows those that result in significant change.
Table 11. Output analysis of Scenario 1 results: insignificant change.
Table 12. Output analysis of Scenario 17 results: significant change.
shows the result summary of all the scenarios run and the significance of the result compared to the existing result. From this, we can find out that only 3 out of 19 scenarios are proven significant result compared to existing condition. Those scenarios are called Scenario 17, Scenario 18, and Scenario 19.
Table 13. Scenario and change significance of the test output.
4.3. Result analysis and discussion
In this section, we are going to analyze as well as discuss the result of this research. This section itself will be divided into two parts. First, we will discuss the general and existing condition of the system. And second, the result of conducting experimentation will be elaborated further.
4.3.1. General and existing condition
This research intends to observe the effect of spare part inventory policy towards production line availability and demand fulfilment on a multi-state production system. In this case, we observe a system containing three states (success, fail, working under failure). State of success, working under failure, and complete fails are denoted as 1, 0.5, and 0 consecutively. In this case, we consider both 0 and 0.5 status as failing status, yet 0.5 status still indicates that system or subsystem is still running with less-than-ideal capacity. Hence, both 0.5 and 0 status do affect spare part inventory level at certain time. When a system or subsystem has status of 0.5 or 0, it means the reparation or maintenance action should be performed. Therefore, spare parts are most likely required and will be taken out of the inventory. Status of 0.5 also affects the availability of production line in a way. When the system or subsystem can somehow operate under that failure, we declare the availability rate as 0.5, not 0 or completely fails. Lastly, 0.5 status also allows the system or subsystem to produce goods but with limited capacity as assumed here is half capacity. Thus, even though the system or subsystem is ‘failing’, it still has ability to produce the item so that the system is trying to fulfil the demand given its condition.
A system entirely arranged in series is highly sensitive to failure. Even only one small failing unit causes the entire system to fail. In this case, the production line in this study is sensitive to any failure. Therefore, to ensure that the production line is highly available, we expect that spare parts are always available. Hence, maintenance action can be conducted on time. Based on the result obtained while plotting inventory level movement of all spare parts, we have found some interesting phenomenon. Certain spare parts are ordered when the inventory level falls below ROP or s, or even zero. This is explained by the fact that a single usage of spare parts causes a significant fall in the inventory level. The effect of the small value of S set by the company is revealed.
4.3.2. Experimentation analysis
By running the simulation completely, we found that there is at least four spare parts with the lowest availability which are SP 2, SP 7, SP 15, and SP 19. Those four spare parts are used as the basis of developing scenarios by increasing its s and S value. However, changing only one spare part’s inventory parameter does not result in any significant change. Therefore, we decided to change parameters of two, three, and four spare parts simultaneously. We do this similarly to what Salsabila and Siswanto (Citation2019) did on their research in which changing those critical spare parts’ inventory parameter simultaneously resulted in significant change. Unfavorably, in our case, modifying the parameter of those four critical spare parts, no significant result is reached. Therefore, we have attempted to change all spare part parameters simultaneously by increasing the s value. By increasing s by 50%, no significant result is still obtained. Eventually, significant change is realized upon increasing the s value by 80% reflected in Scenario 17.
We would like to observe the impact of implementation of Scenario 17 to the inventory level movement. We do the same method to plot a graph like . shows the inventory level movement for those four chosen spare parts. From , we can learn that the inventory level movement is getting better given that most of spare part orders are placed when the level touches ROP and no orders placed when inventory level is 0.
Scenario 17 increases the availability from 0.9268 to 0.9407 and improves demand fulfillment from 90.06% to 91.74%. With this result, we find that enlarging value of s and S does improve both availability and demand fulfilment. This result is validated by finding of research done by Salsabila and Siswanto (Citation2019) which also found that increasing both s and S value improve their output parameter which are availability and calendar days. In this case, this research and Salsabila and Siswanto (Citation2019) research agree that increasing s and S value will increase availability. In this case, Salsabila and Siswanto (Citation2019) got significant results when changing inventory parameters value of only critical spare parts while this research results significant change when modifying inventory parameters value of all observed spare parts.
Additionally, we have attempted to develop an additional scenario by applying s,Q policy to observe its effect on the system. Although the nature of spare parts demand, in general, is intermittent (Kannan et al., Citation2020; Pinçe et al., Citation2021); however, some researchers suggested that it is still possible to implement s,Q policy for spare part inventory (Barik et al., Citation2022; Istiningrum et al., Citation2021; Wongmongkolrit & Rassameethes, Citation2011). In the case of Wongmongkolrit and Rassameethes (Citation2011), intermittent demand is approached using the failure rate of the equipment. As for this research, we did an almost similar thing by using MTBF to determine when the demand of particular spare parts appears. For Scenario 18, in which we implement s,Q policy with the current s value, we obtained another significant result. Applying this policy improved availability to 0.9370 and demand fulfillment to 91.61%. Compared with Scenario 17, this improvement value is not as significant as that in Scenario 17. In order to obtain better results, we have developed Scenario 19. In this scenario, we implemented the s,Q policy with increased s by 50%. We are satisfied that Scenario 19 results in the best solution among all scenarios. Scenario 19 improves the availability to 0.9407, while demand fulfillment is enhanced to 92.10%.
and show the inventory level movement of four chosen spare parts for Scenario 18 and 19 respectively. Since s,Q policy does not apply S or maximum inventory level, hence it is allowed to keep spare parts with higher quantity than when implementing s,S. As for SP 2, s,Q policy allows us to store more than 6 units in inventory compared to Scenario 17. A similar thing happens to SP 7 and SP 19 as well where this policy also allows their inventory level to go up 3–4 times higher than S value at Scenario 17. Extremely, the s,Q policy causes the inventory level of SP 15 skyrockets higher than 100 units. This is aligned with the goal of implementing s,Q policy itself. Having EOQ or Q value, the policy allows us to have a fixed order quantity, which could lead us to less frequent order being placed, and thus minimum cost is expected (Tersine, Citation1994; Waters, Citation2003).
It is also found that completely altering the spare part inventory policy from s,S to s,Q improves both availability and demand fulfilment. This finding aligns with findings by Zahedi-Hosseini et al. (Citation2017) in their research. Zahedi-Hosseini et al. (Citation2017) found out that applying s,Q policy resulted in lower failure occurrence. Hence, this fact implies that implementing s,Q inventory policy results in higher availability rate which leads to higher demand fulfilment rate.
It seems like there is a strong correlation between spare part inventory policy, production line availability, and demand fulfilment rate. To test whether there is a correlation among those three, we try to visualize the number showing the relation of spare part inventory policy, production line availability, and demand fulfilment rate for 4 conditions: existing condition, Scenario 17, Scenario 18, Scenario 19. As for spare part inventory policy, its number will be represented by average availability of all spare parts under this observation. illustrates the summary of average availability of spare parts, production line availability, and demand fulfilment. From that figure, we can take a glance and make an early conclusion that there is indeed correlation between spare part inventory policy, production line availability, and demand fulfilment rate. shows the statistical computation regarding correlation value of those three components assuming significance level is 5%. From , we can conclude that there is a positive correlation between spare part inventory policy (represented by spare part availability) to both production line availability (Tersine, Citation1994; Zahedi-Hosseini et al., Citation2018) and demand fulfilment rate. Besides, it is also proven that the production line availability does communicate with demand fulfilment rate (Alrabghi et al., Citation2017; Alrabghi & Tiwari, Citation2016; Salsabila et al., Citation2019).
Table 14. Correlation value of spare part availability, line availability, and demand fulfilment rate.
4.3.3. Selecting scenarios
From previous discussion and elaboration, it is obtained that three scenarios which are Scenario 17 (s,S with s increased by 80%), Scenario 18 (s,Q), and Scenario 19 (s,Q with s increased by 50%). However, for implementation itself, the best scenario needs to be determined. Therefore, further analysis needs to be performed regarding the inventory cost incurred.
The inventory policy features its own advantages and disadvantages. Take s,Q policy as an example, it decreases the number of orders to be placed resulting in the reduction of the ordering cost (Tersine, Citation1994; Waters, Citation2003). However, since the amount of orders is always constant, it will have impact on the purchasing cost and holding cost because units in warehouse might be high. TIC is calculated annually. According to Wilson (Citation1934), the component building this cost consists of the ordering cost (OC), purchasing cost (PC), and holding cost (HC). Therefore, TIC is formulated as follows:
(7)
(7)
We compare the TIC of the existing condition to the significant scenarios. shows the recapitulation of TIC of existing condition and 3 significant scenarios. None of the scenario results in lower inventory costs compared to existing condition with Scenario 17 being the highest among of all. However, we could see that 3 scenarios have lower ordering cost with s,Q policy owns the lowest ordering cost. This proves that s,Q policy reduces ordering costs significantly. As for unit purchasing cost, since s,Q applies EOQ to all spare parts, the value seems to be similar thanks to the fixed order quantity being implemented. In contrast, Scenario 17 exhibits higher purchasing cost which is caused by the large S value. Holding cost varies for all conditions since it depends on the usage of the spare part when failure happens which is probabilistic in nature.
We cannot simply use these numbers to choose which scenario to implement. Hence to obtain better comparison, we compare the increased annual TIC to improve every 1% of both availability and demand fulfillment. The increased TIC is obtained by comparing the TIC of the existing condition to the scenarios. From , we can observe that Scenario 17 exhibits the highest increased annual inventory cost, only to improve every percent of KPI. Based on our analysis, TIC of Scenario 17 is exceedingly high owing to the holding cost and purchasing cost. Since we simultaneously increase s and S significantly, the inventory level is also increased. Hence, based on this, Scenario 17 is unfavorable.
Considering Scenario 18, Scenario 18 shows the lowest increment on the annual TIC followed by Scenario 19. By slightly adding the annual inventory cost from Scenario 18, applying Scenario 19 leads us to the best solution. Based on this finding, Scenario 18 is more suitable if the company is cost-oriented, and Scenario 19 is the best alternative if the company is performance-oriented. However, as it is shown by and that inventory level of SP 15 reaches more than 100 units being kept in stock. Therefore, stakeholders should ensure the space or storage availability to keep SP 15 or probably other spare parts in large numbers. If there is no available space or storage, additional investment or perhaps a storage rearrangement should be taken place to accommodate this.
4.4. Sensitivity analysis
Sensitivity analysis is performed to assess and predict the robustness of the model constructed toward the change in uncontrollable variables. In this case, we perform sensitivity analysis on the model developed to design Scenario 18 as the chosen alternative. The aspect to test in this analysis is delay lead time for procuring spare parts.
Through sensitivity analysis, the output changes are tested when the delay lead time for ordering spare parts is also altered. In this case, we attempt to change the order lead time of all observed spare parts by decreasing or increasing it. All observed spare parts require seven days to arrive, but only one spare part requires 14 days.
From and and , by cutting the lead time by 50%, we can improve line availability to 0.9477 and demand fulfillment to 92.53%. However, accelerating the lead time may result in sourcing another supplier which is more responsive. Naturally, a supplier with a high degree of responsiveness tends to have higher cost. This could be something to consider for stakeholders. Nevertheless, we can also observe that as the ordering lead time increases, the performance of availability and demand fulfillment decreases to the point where availability becomes only 0.8853, and 71.3% of demand is fulfilled when the ordering lead time is tripled. Based on this result, we can conclude that availability and demand fulfillment are sensitive to the order lead time. Therefore, maintaining shorter or prompt ordering lead time becomes essential.
Table 15. Sensitivity analysis on ordering lead time.
5. Conclusion
Demand fulfilment or throughput corresponds to the availability of a production line. High production line availability guarantees a high demand fulfilment rate. However, the line availability strongly depends on the spare part inventory. Maintenance action could be carried out immediately if spare parts are always or often available when the production line fails. Therefore, the production line will have a high availability rate. In this research, we consider a production line with three states denoted as 0, 0.5, and 1 and a spare part inventory following the s,S policy. Based on this research, testing a production line and spare part inventory with the aforementioned conditions, we observe that the existing availability rate of the production line is 0.9268, and 90.06% of demand is fulfilled.
A few scenarios are developed to determine the best improvement scenario to boost demand fulfillment and enhance line availability. Before we design the scenarios for implementation, we aim to determine the critical spare parts indicated by low availability. There are four spare parts with the lowest availability; the corresponding s and S values are changed through increment for the generation of scenarios. Changing only one to four spare part parameters is not effective. We eventually obtained a significant result by increasing the s value of all spare parts by 80% (Scenario 17). Implementing s,Q (Scenario 18) and s,Q with 150% s (scenario 19) also generate significant results. In Scenario 17, the availability and demand fulfillment are improved to 0.9407 and 91.74%, respectively. Scenario 18 shows improvement in the availability and demand fulfillment to 0.9370 and 91.61%, respectively. Lastly, the results of Scenario 19 show improvement in the availability and demand fulfillment to 0.9407 and 92.10%, respectively.
Comparing the significant results based on increased annual inventory cost to improve 1% of both performance metrics, we observe that Scenario 18 exhibits the lowest increment cost, followed by Scenario 19 and 17, consecutively. We conclude that Scenario 18 is the best alternative for the cost-oriented company. Furthermore, Scenario 19 is evidently the best solution if the company is performance oriented. Stakeholders should review their warehouse capability to accommodate large number of spare parts to be stored as the consequence of applying s,Q policy of Scenario 18 or 19.
Sensitivity analysis is also performed to assess the robustness of the model. Based on our findings, it is concluded that both availability and demand fulfillment are linearly related to the delivery lead time of spare parts. In other words, availability and demand fulfillment are sensitive to the change in spare part delivery lead time.
This study is performed only for one production line only and assuming the spare part inventory stock is dedicated for that production line only. In addition, we also assume that all subsystems own the same number of states being defined in this study which are success, working under failure, and completely fails. Besides, we only consider several relevant costs in this study such as inventory cost which consists of ordering cost, unit purchasing cost, and holding cost. Not only that, but we also assume that the maintenance action performed is only corrective action where the maintenance action is performed when the failure occurs. Therefore, for future research, it is suggested to observe the entire production plant system instead of only one production line. It is also recommended to extend this research to a more complex system with subsystem owning different number of states. Let’s say one certain subsystem has 3 states and the other has 2 states. We also suggest including more financial parameters such as inventory stockout cost, maintenance cost, and penalty cost as consequences of lost sales. Combining this study with the determination of maintenance strategy to apply preventive and predictive maintenance to optimize the cost incurred may enrich this research in the future.
Disclosure statement
The authors declare that they have no known competing financial interests or personal relationships that could have appeared to influence the work reported in this paper.
Additional information
Funding
Notes on contributors

Nurhadi Siswanto
Nurhadi Siswanto is a faculty member and the Head of Department of Industrial and Systems Engineering at Institut Teknologi Sepuluh Nopember, Surabaya, Indonesia. He earned his PhD degree from University of New South Wales, Canberra, Australia. His research interests include operations research, large-scale optimization, simulation, vehicle routing, inventory routing and modeling of maritime transportation. He has taught courses in Operations Research, Decision Analysis, Discrete Event Simulation, Simulation Modeling, and Quantitative Modeling.

Ahmed Raecky Baihaqy
Ahmed Raecky Baihaqy earned his bachelor’s degree in industrial engineering from Institut Teknologi Sepuluh Nopember (ITS), Surabaya, Indonesia. He was active as a laboratory assistant at Quantitative Modelling and Industrial Policy Analysis (QMIPA) Laboratory of Industrial Engineering ITS also had been appointed as coordinator of laboratory assistant. Currently, he is pursuing a master’s degree in industrial engineering at ITS fully funded by Indonesia Endowment Fund. Ahmed has more than 3 years of experience in logistics and supply chain management at one of biggest 3PL companies. His research interests include discrete-event simulation, inventory modelling, reliability modelling, as well as logistics and supply chain.

Mohd Shukor Salleh
Mohd Shukor Salleh is an Associate Professor at the Faculty of Manufacturing Engineering, Universiti Teknikal Malaysia Melaka (UTeM). Mohd Shukor has taught various courses, including materials testing and fracture analysis, advanced manufacturing processes, heat treatment, semi-solid metal processing, and CNC machining. He also supervises both master’s and Ph.D. students in various subjects related to materials processing, CNC machining, and liquid processing. He was an editor of many published books, has published numerous articles, and is currently the technical editor for the Journal of Advanced Manufacturing Technology, an international journal published by the faculty. His research interests include semi-solid metal processing, casting, CNC machining, and manufacturing processes.

Ruhul Sarker
Ruhul A Sarker obtained his PhD from Dalhousie University (former TUNS), Canada. He is a Professor in the School of Engineering and IT (SEIT) at UNSW Canberra located at ADFA. He served as the Director of Faculty PG Research (June 2015 to May 2020) and as the Deputy Head of School (Research) of SEIT (2011–2014). Prof. Sarker’s broad research interests are decision analytics, CI / evolutionary computation, operations research, and applied optimization with an emphasis on Augmenting Human Intelligence (AHI). His name appeared on the recent lists of top 2% of world’s scientists-researchers prepared by (i) Stanford University and also (ii) Elsevier/Scopus/digitalcommonsdata (Research fields: Artificial Intelligence and Operations Research).
References
- Alrabghi, A., & Tiwari, A. (2016). A novel approach for modelling complex maintenance systems using discrete event simulation. Reliability Engineering & System Safety, 154, 160–170. https://doi.org/10.1016/j.ress.2016.06.003
- Alrabghi, A., Tiwari, A., & Savill, M. (2017). Simulation-based optimisation of maintenance systems: Industrial case studies. Journal of Manufacturing Systems, 44, 191–206. https://doi.org/10.1016/j.jmsy.2017.05.008
- Altiok, T., & Melamed, B. (2007). Simulation modeling and analysis with arena. Elsevier.
- Banks, J., Carson, J., Nelson, B., & Nicol, D. (1995). Discrete-event simulation (3rd ed.). Prentice-Hall.
- Barik, R., Mahatha, B. K., & Mishra, C. R. (2022). Spare parts inventory management by using EOQ model. Journal of Emerging Technologies and Innovative Research, 9, d249–d258.www.jetir.orgd249
- Barringer, H. P. (1997). Availability, reliability, maintainability, and capability. Triplex Chapter of Vibration Institute.
- Bülbül, P., Bayındır, Z. P., & Bakal, İ S. (2019). Exact and heuristic approaches for joint maintenance and spare parts planning. Computers & Industrial Engineering, 129, 239–250. https://doi.org/10.1016/j.cie.2019.01.032
- Chang, P. C., Lin, Y. K., & Chen, J. C. (2017). System reliability for a multi-state manufacturing network with joint buffer stations. Journal of Manufacturing Systems, 42, 170–178. https://doi.org/10.1016/j.jmsy.2016.12.009
- Chang, P. C., Lin, Y. K., & Chiang, Y. M. (2019). System reliability estimation and sensitivity analysis for multi-state manufacturing network with joint buffers––A simulation approach. Reliability Engineering & System Safety, 188, 103–109. https://doi.org/10.1016/j.ress.2019.03.024
- Chen, Z., Chen, Z., Zhou, D., Shao, C., & Pan, E. (2022). Optimal replacement policy for multi-state manufacturing system with economic and resource dependence under epistemic uncertainty. International Journal of Production Research, 61(20), 6772–6790. https://doi.org/10.1080/00207543.2022.2137595
- Dendauw, P., Goeman, T., Claeys, D., Turck, K. D., Fiems, D., & Bruneel, H. (2021). Condition-based critical level policy for spare parts inventory management. Computers & Industrial Engineering, 157, 107369. https://doi.org/10.1016/j.cie.2021.107369
- Dhillon, B. S. (2006). Maintainability, maintenance, and reliability for engineers. Taylor & Francis.
- Elsayed, E. A. (2012). Reliability engineering (2nd ed.). John Wiley.
- Golbasi, O., & Turan, M. O. (2020). A discrete-event simulation algorithm for the optimization of multi-scenario maintenance policies. Computers & Industrial Engineering, 145, 106514. https://doi.org/10.1016/j.cie.2020.106514
- Harrell, C., Gosh, B. K., & Bowden, R. O. (2004). Simulation using promodel (2nd ed.). Mc. Graw Hill.
- Hoover, S., & Perry, R. (1990). Simulation: A problem solving approach. Addison-Wesley.
- Ireson, W. G., Coombs, C. F., & Moss, R. Y. (1996). Handbook of reliability engineering and management (2nd ed.). McGraw-Hill.
- Istiningrum, A. A., Munandar, L. M., & Sono. (2021). Reducing spare part inventory cost with shortage elimination through probabilistic economic order quantity. KINERJA, 25(2), 179–191. https://doi.org/10.24002/kinerja.v25i2.4371
- Jang, J. S., & Park, S. C. (2017). A reliability model for a wafer FAB. Cogent Engineering, 4(1), 1340817. https://doi.org/10.1080/23311916.2017.1340817
- Kannan, B. A., Kodi, G., Padilla, O., Gray, D., & Smith, B. C. (2020). Forecasting spare parts sporadic demand using traditional methods and machine learning – A comparative study. SMU Data Science Review, 3(2), 1–22. https://scholar.smu.edu/datasciencereview/vol3/iss2/9
- Kelton, W. D., Sadowski, R. P., & Zupick, N. B. (2015). Simulation with arena (6th ed.). McGraw Hill.
- Kolawole, A., Agboola, O. O., Ikubanni, P. P., Raji, O. G., & Okechukwu Osueke, C. (2019). Reliability and power loss analysis: A case study of a power plant in Nigeria. Cogent Engineering, 6(1), 1–13. https://doi.org/10.1080/23311916.2019.1579426
- Law, A. M. (2013). Simulation modeling and analysis (5th ed.). McGraw Hill.
- Lolli, F., Coruzzolo, A. M., Peron, M., & Sgarbossa, F. (2022). Age-based preventive maintenance with multiple printing options. International Journal of Production Economics, 243, 108339. https://doi.org/10.1016/j.ijpe.2021.108339
- Manda, V. R., Kampurath, V., & Msrk, C. (2018). 3D printing and its effect on outsourcing: A study of the Indian aircraft industry. Journal of Aerospace Technology and Management, 10, 1–22. https://doi.org/10.5028/jatm.v10.862
- Melani, A. H. D. A., Michalski, M. A. D. C., da Silva, R. F., Netto, A. C., & de Souza, G. F. M. (2022). Petri net-based reliability and availability analysis to support asset management: A CODOG propulsion system case study. IFAC-PapersOnLine, 55(19), 19–24. https://doi.org/10.1016/j.ifacol.2022.09.178
- Nnamdi, O. (2018). Strategies for managing excess and dead inventories: A case study of spare parts inventories in the elevator equipment industry. Operations and Supply Chain Management: An International Journal, 11(3), 128–138. https://doi.org/10.31387/oscm0320209
- Olukanni, D. O., Adejumo, T. A., Salami, A. W., & Adedeji, A. A. (2018). Optimization-based reliability of a multipurpose reservoir by genetic algorithms: Jebba Hydropower Dam, Nigeria. Cogent Engineering, 5(1), 1438740. https://doi.org/10.1080/23311916.2018.1438740
- Pinçe, Ç., Turrini, L., & Meissner, J. (2021). Intermittent demand forecasting for spare parts: A critical review. Omega (United Kingdom), 105, 102513. https://doi.org/10.1016/j.omega.2021.102513
- Pujawan, I. N., & Er, M. (2017). Supply chain management (3rd ed.). Andi.
- Ram, M., & Manglik, M. (2016). An analysis to multi-state manufacturing system with common cause failure and waiting repair strategy. Cogent Engineering, 3(1), 1266185. https://doi.org/10.1080/23311916.2016.1266185
- Rao, M. S., & Naikan, V. N. A. (2016). Review of simulation approaches in reliability and availability modeling. International Journal of Performability Engineering, 12(4), 369–388. https://doi.org/10.23940/ijpe.16.4.p369.mag
- Salsabila, N. Y., & Siswanto, N. (2019). A simulation study of availability analysis on a chemical process industry considering spare part inventory. IOP Conference Series: Materials Science and Engineering, 673(1), 012079. https://doi.org/10.1088/1757-899X/673/1/012079
- Salsabila, N. Y., Siswanto, N., Widodo, E., & Rochmadhan, O. A. (2019). Throughput analysis on a multi-state manufacturing system by considering availability. Jurnal Teknik Industri, 21(2), 69–78. https://doi.org/10.9744/jti.21.2.69-78
- Sathish, T., Vijayakumar, M. D., & Ayyangar, A. K. (2018). Design and fabrication of industrial components using 3D printing. Materials Today: Proceedings, 5(6), 14489–14498. www.sciencedirect.comwww.materialstoday.com/proceedings https://doi.org/10.1016/j.matpr.2018.03.036
- Sharda, B., & Bury, S. J. (2008 A discrete event simulation model for reliability modelling of a chemical plant [Paper presentation]. Proceeding of the 2008 Winter Simulation Conference. https://doi.org/10.1109/WSC.2008.4736260
- Snyder, L. V., & Shen, Z.-J M. (2011). Fundamentals of supply chain theory (1st ed.). John Wiley & Sons.
- Tersine, R. J. (1994). Principles of inventory and materials management (4th ed.). Prentice Hall.
- Wakiru, J. M., Pintelon, L., Muchiri, P. N., & Chemweno, P. K. (2019). A simulation-based optimization approach evaluating maintenance and spare parts demand interaction effects. International Journal of Production Economics, 208, 329–342. https://doi.org/10.1016/j.ijpe.2018.12.014
- Waters, D. (2003). Inventory control and management (2nd ed.). John Wiley & Sons.
- Wilson, R. H. (1934). A scientific routine for stock control. Harvard Business Review, 13(1), 116–129.
- Wongmongkolrit, S., & Rassameethes, B. (2011). The modification of EOQ model under the spare parts discrete demand: A case study of slow moving items. Proceedings of the World Congress on Engineering and Computer Science (WCECS), San Fransisco, USA, 19–21 October 2011.
- Xu, L., Chen, Y., Briand, F., Zhou, F., & Givanni, M. (2017). Reliability measurement for multistate manufacturing systems with failure interaction. Procedia CIRP, 63, 242–247. https://doi.org/10.1016/j.procir.2017.03.124
- Xu, X., Rodgers, M. D., & Guo, W. (. (2021). Hybrid simulation models for spare parts supply chain considering 3D printing capabilities. Journal of Manufacturing Systems, 59, 272–282. https://doi.org/10.1016/j.jmsy.2021.02.018
- Zahedi-Hosseini, F., Scarf, P., & Syntetos, A. (2017). Joint optimisation of inspection maintenance and spare parts provisioning: A comparative study of inventory policies using simulation and survey data. Reliability Engineering & System Safety, 168, 306–316. https://doi.org/10.1016/j.ress.2017.03.007
- Zahedi-Hosseini, F., Scarf, P., & Syntetos, A. (2018). Joint maintenance-inventory optimisation of parallel production systems. Journal of Manufacturing Systems, 48, 73–86. https://doi.org/10.1016/j.jmsy.2018.06.002
- Zhang, Y., Zhao, M., Zhang, Y., Pan, R., & Cai, J. (2020). Dynamic and steady-state performance analysis for multi-state repairable reconfigurable manufacturing systems with buffers. European Journal of Operational Research, 283(2), 491–510. https://doi.org/10.1016/j.ejor.2019.11.013
- Zhou, Y., Guo, Y., Lin, T. R., & Ma, L. (2018). Maintenance optimisation of a series production system with intermediate buffers using a multi-agent FMDP. Reliability Engineering & System Safety, 180, 39–48. https://doi.org/10.1016/j.ress.2018.07.008
- Zhu, S., Jaarsveld, W. V., & Dekker, R. (2020). Spare parts inventory control based on maintenance planning. Reliability Engineering & System Safety, 193, 106600. https://doi.org/10.1016/j.ress.2019.106600