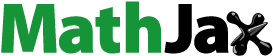
Abstract
The motive of this study is to evaluate the machinability properties of AZ31 Mg alloy in the face milling operations. Depth of cut, rate of feed and speed of cut are three input variables in the face milling process, while surface roughness (Ra) and tool nose radius deviation (dR) are performance characteristics. For three input variables each with three levels, to decrease the number of experiments, an L9 orthogonal array (OA) of Taguchi was employed which enables an efficient approach for conducting a full-factorial design of experiments. Selection of optimal parameters for the face milling process to achieve the required surface quality is accomplished through the utilization of a modified Taguchi approach and a novel multi objective optimization procedure. The work establishes empirical relationships that correlate the performance characteristics (i.e. Ra and dR) with three input variables. Corrections to the empirical relations were specified to obtain the expected range of Ra and dR. Ra and dR estimates were validated with existing test data.
1. Introduction
The combination of lightweight, high strength, good machinability, excellent damping and thermal conductivity properties make Mg alloys attractive for wide collection of applications in electric vehicle, biomedical, aerospace, electronic industries that prioritize weight reduction, performance optimization and energy efficiency (Muni Tanuja et al., Citation2022; Tan & Ramakrishna, Citation2021; Mordike & Ebert, Citation2001). While the hexagonally close packed (HCP) crystalline arrangement of Mg alloys limits their formability and also active slip systems, which limits their suitability for certain structural applications (Sivam et al., Citation2018; Brandão et al., Citation2016). Currently, the predominant research in Mg alloys is primarily focused to plastic processing technologies (such as forging, rolling, drawing, extrusion and pressing) and various heat treatment methods (including annealing, quenching, solid state dissolution and aging) (Jiang et al., Citation2015; Sivam et al., Citation2018; Wang et al., Citation2015; Jiang et al., Citation2016). In a study by Zakaria et al. (Citation2022) to compensate the lack of cooling in dry machining, a new submerged convective cooling (SCC) approach was used to perform the turning process of AZ31 Mg alloy, which increased the cutting performance.
An analysis is conducted to evaluate the effects of cryogenic and dry machining conditions on high speed milling of Mg alloy in the regime of high cutting speeds, since Mg alloy chips are highly flammable, it essential to find effective ways to reduce the temperature effect in the cutting areas. The effects of these cutting conditions on the milling process are being investigated to improve the safety and performance of Mg alloy machining (Jouini et al., Citation2023; Ghosh et al., Citation2007). In their study, Lu et al. (Citation2016) examined the effect of cutting variables (i.e. depth of cut, rate of feed) on microstructure, surface roughness and work hardening. As speed of cut increases, depth of stress layer, roughness and hardening decrease. On the contrary, it was noticed that with higher the depth of cut and rate of feed increase, these parameters show an upward trend. Speed of cut, axial and depth of cut in radial direction, and feed per tooth are important to achieve increased machining stability and excellent surface finish, and the recommended a tool with a 200helix angle for finish milling process (Zagórski et al., Citation2022). By refining the microstructures with unique elements, it may be possible to improve the mechanical properties, heat treating the material and thermo-mechanical treatment as well. By designing a die for severe plastic deformation (SPD), improved homogeneity of deformation can be observed in the pressed sample (Anantha et al., Citation2023a, Citation2023b; Moradpour et al., Citation2018). Anantha et al. (Citation2023a, Citation2023b) implemented a genetic algorithm (GA) multi-criterion optimization tool to find the optimal die geometry (Groove width = 3 mm, Groove angle = 50° and Coefficient of friction = 0.22).
The S/N ratio transformation introduced by Taguchi (Ross, Citation1989; Konduri et al., Citation2017) offers a way to account for variability in repeated tests by consolidating the output responses into a single value. Without implementing the S/N ratio transformation, several optimal solutions have been achieved in various applications. These include minimizing damage to composites caused by drilling (Singaravelu et al., Citation2012), ensuring collision-free satellite separation processes (Koneru et al., Citation2022), increasing the performance of heat pipes (Ramya et al., Citation2018; Koneru et al., Citation2023), optimizing the design of planetary gears (Miladinovic et al., Citation2021), improving manufacturing processes (Satyanarayana et al., Citation2018; Rajyalakshmi & Boggarapu, Citation2018; Rajyalakshmi & Rao, Citation2019; Satyanarayana et al., Citation2019; Dharmendra et al., Citation2019; Dharmendra et al., Citation2020; Satyanarayana et al., Citation2021; Buddi et al., Citation2018), optimizing painting processes using robot (Danthala et al., Citation2021), increasing the performance of a biodiesel engine using additives (Sanjeevannavar et al., Citation2022) and stir casting technique (Saritha et al., Citation2019).
Santhakumar and Iqbal (Citation2022) conducted interesting studies on the effect of milling parameters (speed of cut, depth of cut and rate of feed) on surface quality and tool nose radius in Mg alloys (see ). They implemented the transformation of S/N ratio to the measured Ra and dR choosing the smaller-the-better as standard. They performed a grey relational Taguchi analysis to adjust the cutting parameters of dry face milling for achieving lower Ra and dR. In fact, it has been recommended to apply the transformation of S/N ratio to the repeated test response to take care for the experimental variance, while most researchers have applied this transformation to the mean value of the repeated test data.
Figure 1. Experimental setup for dry face milling of AZ31 Mg alloy (Santhakumar & Iqbal, Citation2022): (A) Coolant nozzle (OFF); (B) Collet; (C) Workpiece.

Encouraged by the research of the aforementioned researchers, the problem is re-examined by applying a modified Taguchi method and a reliable multi objective optimization procedure for selecting a group of input process parameters (viz. depth of cut, rate of feed and speed of cut) to achieve higher Ra and lower dR in face milling of AZ31 Mg alloy.
2. Analysis
Dry face milling is accomplished on AZ31 Mg alloy by means of a cutting tool of 12 mm TiCN-coated carbide grade inserts. The dimensions of the work-piece are
The experimentation setup involved utilizing a 3-axis VMC (Vertical Machining Center) equipped with a 7.5 kW motor and a spindle speed maintained below 8000 rpm. Speed of cut (A), rate of feed (B) and depth of cut (C) are milling parameters. The AZ31 Mg alloy’s chemical composition (Santhakumar & Iqbal, Citation2022) in confirms with others (Hsiang & Lin, Citation2019; Karthik et al., Citation2021; Goyal et al., Citation2022; Ömer, Citation2019).
Table 1. AZ31’s chemical composition.
To perform dry milling experiments, each input parameter in is assigned with three levels. Taguchi’ L9 orthogonal array (OA) is considered for conducting fewer experiments and set the input parameters for each test run. For levels to each input parameter, the number of input parameters (
) according to the Taguchi’s L9 OA can be found from (Ross, Citation1989)
(1)
(1)
Table 2. Different levels of dry milling processing variables and measured performance characteristics of AZ31 Mg alloy.
For 9 tests, 4 input parameters can be accommodated. In this case, only three input parameters (i.e. A, B and C) were set and hence, a dummy (fictitious) parameter is introduced i.e. D in to assess the error in estimates for output responses. This aspect was thoroughly discussed by Miladinovic et al. (Citation2021). Measured data of dry milling process for the nine sets of input parameters are tabulated in .
An analysis of variance (ANOVA) was carried using the measured data of performance characteristics presented in summarizes the results of the ANOVA. Results depicted in clears that, rate of feed (B) has the maximum contribution to ‘Ra’ and ‘dR’ of 85.1% and 85.6%, respectively. Speed of cut (A) and depth of cut (C) have contributions of 8% and 2.5% to Ra, while 4.2% and 5% contribution to dR. The sum of the contributions of all the process variables (A, B, C and D) to ‘Ra’ and ‘dR’ is 100%. The fictitious parameter (D) has contribution of 4.3% to Ra, while 5.2% to dR. Majority in manufacturing problems depend on experimentation to observe the phenomenon prior to mathematical modeling. Some unknown parameters may influence the process resulting scatter in the performance characteristics in repeated tests. Though the Taguchi’s L9 OA is applicable to four parameters with three levels, the present problem specifies only three parameters with three levels. Considering the unknown fourth parameter (fictitious) as D, ANOVA is performed and arrived the %Contribution. The parameter D can be treated as the unknown influencing parameter (or error in measurements if any) of the performance characteristics.
Table 3. ANOVA results indicating the significance of milling process parameters.
The utilization of the modified Taguchi method (Ramya et al., Citation2018; Koneru et al., Citation2023; Miladinovic et al., Citation2021; Satyanarayana et al., Citation2018) enables the determination of the range of estimates in mechanical properties (such as Surface roughness [Ra], Tool nose radius [dR]) within a given range. By considering the specified process parameters as input variables, namely Speed of cut (A), Rate of feed (B) and Depth of cut (C), this approach facilitates process design by providing insights into the expected scatter in repeated experiments. By considering as a measure of performance (Ra or dR) and employing the additive law (Ross, Citation1989), EquationEquation (2)
(2)
(2) allows for the prediction of
based on the levels of the input variables.
(2)
(2)
can be obtained from ANOVA . Subscript ‘
’ with
stands for the milling process parameters (A, B, C and D), and ‘
’ with
stands for the 1-mean, 2-mean, 3-mean of the performance indicator (Ra or dR) to the levels of (A, B, C and D).
is the grand mean of
for all 9 test runs. Considering Ra as the performance indicator,
= 1.4731
and
=1.4798 in ANOVA , which corresponds to the 2-mean of Ra for the level-2 of the milling process parameter, B. Here, the estimates of Ra and dR from are utilized for comparing with the experimental results obtained from the 9 test runs conducted using Taguchi’s L9 OA (orthogonal array).
= 3 and
= 4 in EquationEquation (2)
(2)
(2) provide the estimates of the performance characteristics by excluding and including the contribution of the parameter, D. It is clearly visible about the discrepancy in the estimates in for
=3, whereas in case of
=4, the estimates are identical to the test data. The corrections to the estimates for
=3 are determined by calculating the minimum and maximum deviation of mean values related to the dummy parameter (D) from the grand mean. The estimated range of the performance characteristics can be obtained after application of these corrections to the estimates. Applying corrections −0.1799 µm and −0.01667 mm to the estimates of Ra and dR, one can obtain lower bound values. The upper-bound values of Ra and dR are attained by employing corrections of 0.1222 µm and 0.0233 mm, respectively, to the corresponding estimates.
Table 4. Estimates of Ra and dR.
To illustrate the simplicity of the present analysis, the estimates of Ra in Test Run-1 of are presented below. The levels of (A, B, C and D) in Test Run-1 are (1, 1, 1, 1) and the mean values of Ra from ANOVA are: 1.5527
0.7657
1.3358
and
1.5953
The grand mean,
= 1.4731
Using EquationEquation (2)(2)
(2) , estimate of Ra with exclusion of D (
) in Test Run-1:
Using EquationEquation (2)(2)
(2) , estimate of Ra with inclusion of D (
=4) in Test Run-1:
The corrections to the estimates of Ra for =3 are the minimum and maximum deviation of mean values related to the dummy parameter (D) from the grand mean:
Applying these corrections to the estimate of Ra with exclusion of D, the minimum and maximum estimates of Ra obtained for Test Run-1 are: 0.5281 (=0.7080 − 0.1799) and 0.8302 (=0.7080 + 0.1222).
For the specified levels of input parameters (A, B and C) in Taguchi L9 OA, the performance characteristics (Ra and dR) are evaluated using additive law (2). Appropriate corrections are applied to obtain the lower and upper limits of Ra and dR. Test data (Santhakumar & Iqbal, Citation2022) in fall within the estimated range. It is possible to estimate the performance characteristics for all combinations of the three levels and three milling process parameters using the additive law (2).
For the combination of three levels of three input parameters, 27 (=33) sets were created. These are in which subscripts to parameters (A, B, C) are the levels. They represent the full factorial design of experiments and allotted the sequence numbers from 1 to 27. The set of input parameters to the sequence numbers 1, 5, 9, 11, 15, 16, 21, 22 and 26 represent the test Runs 1–9 in Taguchi’s L9 OA. Using EquationEquation (2)
(2)
(2) , Ra and dR values were calculated for the framed 27 sets of input parameters. The performance characteristics (Ra and dR) for the above 27 sets of parameters including the test data of Taguchi’s L9 OA in are shown in and . Test data fall between the lower and upper limits.
Figure 2. Comparison between test results (Santhakumar & Iqbal, Citation2022) and Ra estimates.

Figure 3. Comparison between test results (Santhakumar & Iqbal, Citation2022) and dR estimates.

Transforming the three levels of the process parameters to −1, 0 and 1, it is possible to represent mean values (see ANOVA ) of the performance indicator (Ra or dR) in a quadratic polynomial form and use them in the additive law (2) to obtain empirical relationship for Ra and dR in terms of input variables (A, B, C). The transformed process parameters are
and
Using the mean values of Ra and dR in ANOVA , the following empirical relationships are developed.
(3)
(3)
(4)
(4)
To illustrate the effectiveness of EquationEquations (3)(3)
(3) and Equation(4)
(4)
(4) , the three levels of Test Run-1 having a set of parameters (A = 40, B = 0.05, C = 0.3) are considered. The transformed parameters for this set of parameters are (
). Using them in EquationEquations (3)
(3)
(3) and Equation(4)
(4)
(4) result
Applying corrections −0.1799 and 0.1222 µm, lower and upper bound values of Ra obtained are 0.5281 µm and 0.8303 µm, which are in good agreement with those in .
Applying corrections −0.01667 and 0.0233 mm, lower and upper bound values of dR obtained are 0.88 and 0.92 mm, which are in good agreement with those in .
The above illustration confirms the validity of the developed empirical relationships (3) and (4). For Ra and dR. Applying corrections −0.1799 µm and −0.01667 mm to Ra and dR for EquationEquations (3)(3)
(3) and Equation(4)
(4)
(4) , lower bound values of Ra and dR can be obtained. Upper-bound values of Ra and dR are achieved by employing corrections 0.1222 µm and 0.0233 mm to Ra and dR for EquationEquations (3)
(3)
(3) and Equation(4)
(4)
(4) . Results from EquationEquations (3)
(3)
(3) and Equation(4)
(4)
(4) are closer to those from additive law (EquationEquation (2)
(2)
(2) ).
3. Results and discussion
By examining ANOVA , it is possible to determine a set of milling process parameters (with subscripts indicating the respective levels of parameters) that result in the minimum Ra value and for achieving the minimum dR, the parameters
are necessary.
The sets of parameters for achieving the minimum Ra and minimum dR values are distinct from each other. In order to obtain one common set of milling parameters to minimize both Ra and dR, the current multi objective functions (Ra and dR) are converted into one single objective function, as presented in previous works (Koneru et al., Citation2022; Ramya et al., Citation2018; Koneru et al., Citation2023). In ANOVA results (), the values of Ra and dR were normalized with the respective maximum values, = 2.5459 µm; and
= 1.1233 mm. Defining
and
By minimizing the functions
and
one can achieve the minimum values for Ra and dR. By specifying positive weighing factors as
and
(that satisfy:
) as explained in the work (Koneru et al., Citation2022; Ramya et al., Citation2018; Koneru et al., Citation2023), one single objective function is formulated to minimize both Ra and dR can be written as,
(5)
(5)
By minimizing the single objective function (), the optimal milling parameters can be determined, provides minimum Ra and dR values. The minimum
Ra and dR with
and
is produced by minimizing for
=1 (
=0). In ANOVA , these two cases are displayed with bolded numerals. To achieve common optimal process conditions, two weighing factors (
and
) are taken into account.
In ANOVA , the mean values of Ra and dR are normalized by = 2.5459 m and
= 1.1233 mm, respectively. The mean values of
and
are calculated. EquationEquation (5)
(5)
(5) results in a single objective function
when mean values of
and
are multiplied with equalized weighing factors (and
). A set of optimal parameters was determined as
from the minimum mean values of
to obtain the minimum Ra and dR (see ). lists milling parameters for particular conditions with estimates of Ra and dR.
Table 5. Mean values of the single objective function in Equation (6) with different sets of weighing factors (
).
Table 6. Milling parameters and the predictions of Ra and dR under particular conditions.
depicts the change in Ra and dR with the speed of cut (A) for the specified rate of feed, and depth of cut,
Surface roughness (Ra) shows increasing-decreasing trend, whereas the tool nose radius deviation (dR) shows increasing trend with the speed of cut (A). represents the variation of Ra and dR with rate of feed (B) for the specified speed of cut,
and depth of cut,
Ra and dR increase with the rate of feed (B). indicate the variations of Ra and dR with depth of cut (C) for the specified speed of cut,
and rate of feed,
R
a shows increasing trend, whereas dR shows increasing-decreasing trend with the depth of cut (C). The curves in are generated from the developed empirical relations (3) and (4) and applying the corrections for the lower and upper bounds.
Figure 4. (a) Variation of Ra with the speed of cut (A) for a given rate of feed, and depth of cut,
(b) Variation of dR with the speed of cut (A) for a given rate of feed,
and depth of cut,

Figure 5. (a) Variation of Ra with the rate of feed (B) for the a given speed of cut, and depth of cut,
(b) Variation of dR with the rate of feed (B) for the specified speed of cut,
and depth of cut,

Figure 6. (a) Variation of Ra with the depth of cut (C) for the specified speed of cut, and rate of feed,
(b) Variation of dR with the depth of cut (C) for the specified speed of cut,
and rate of feed,

Industries expect simple, reliable and easy-to-implement procedures to solve optimization problems. Several researchers (Satyanarayana et al., Citation2019) are using many algorithms like grey relational analysis (GRA) without assessing the capabilities in existing theories. Application of the S/N ratio transformation (Kurra & Regalla, Citation2015; Wankhede et al., Citation2023) may not provide any improvement over the optimal solutions for the performance characteristics of a single test run. Here, a modified Taguchi method is followed and found the range of the performance characteristics to the specified set of process variables and a simple multi-objective optimization technique. Empirical relations for Ra and dR are developed and validated with test data. Statistical analysis in this article is performed on Excel sheets without using Minitab/Design Expert.
4. Conclusions
In this article, the machinability properties of the AZ31 Mg alloy were examined during the face milling process. Three levels were allocated to each of input parameters: depth of cut, rate of feed and speed of cut and the Taguchi’s L9 OA was considered. To achieve the desired minimal ‘Ra’ and ‘dR’ in the workpiece, the study used a reliable and efficient multi-objective optimization technique. This article concludes the following points:
ANOVA results confirm that rate of feed (B) has maximum influence on ‘Ra’ with a contribution of 85.1% and on tool nose radius deviation (dR) with a contribution of 85.6%.
The optimal milling process variables to achieve a minimum surface roughness (Ra) are a speed of cut of 80 mm/min, a rate of feed of 0.05 mm/tooth and a depth of cut of 0.3 mm.
A speed of cut of 40 mm/min, a rate of feed of 0.05 mm/tooth and a depth of cut of 0.7 mm are the optimal milling process variables to achieve the minimum ‘dR’.
While an optimal set of parameters to achieve a minimum ‘Ra’ and ‘dR’ is a speed of cut of 80 mm/min, a rate of feed of 0.05 mm/tooth and a depth of cut of 0.3 mm.
Author contributions
Conceptualization: M.T.A., N.R.B. and S.K.; Methodology: N.R.B. and M.T.A.; Data Collection: P.P.T.P. and S.K.; Analysis and Results interpretation: N.R.B. and M.T.A.; Validation of Results: T.B. and S.K.; Preparation of Draft Manuscript: M.T.A., N.R.B., P.P.T.P.; Manuscript Review and Editing: K.I.U. and R.D.; visualization: T.B., R.D., M.T.A. and N.R.B.; supervision: T.B. and K.I.U. The final version of the manuscript was reviewed and approved by all authors.
Disclosure statement
The authors disclosed no potential conflicts of interest.
Data availability statement
The authors confirm that the data supporting the findings of this study are available within the article.
Additional information
Funding
Notes on contributors
Muni Tanuja Anantha
Muni Tanuja Anantha M.Tech (Ph.D), Assistant Professor, Anurag University, Hyderabad, Sheet Metal Processing.
Sireesha Koneru
Sireesha Koneru M.Tech (Ph.D), Assistant Professor, KLEF deemed to be university, Guntur, Design.
Parameshwaran Pillai Thiruvambalam Pillai
Parameshwaran Pillai Thiruvambalam pillai PhD (Manufacturing Engineering), University College of Engineering, BIT Campus, Tiruchirappalli, Fracture Mechanics, Composite Materials.
Nageswara Rao Boggarapu
Nageswara Rao Boggarapu Ph.D, Professor, KLEF deemed to be university, Theoretical and Applied Mechanics, Non-Destructive Testing.
Tanya Buddi
Tanya Buddi Ph.D, Associate Professor, GRIET, Hyderabad, Wood based Bio-Composites, Nano Composites, Sheet Metal Forming.
Kseniia Iurevna Usanova
Kseniia Iurevna Usanova M.Tech, Peter the Great St.Petersburg Polytechnic University: Sankt-Peterburg, Renewable Energy Resources.
Rajesh Deorari
Rajesh Deorari Ph.D, Uttaranchal University, Dehradun, Face milling, Powder Metallurgy.
References
- Anantha, M. T., Buddi, T., & Boggarapu, N. R. (2023a). Multi-objective optimization basing modified Taguchi method to arrive the optimal die design for CGP of AZ31 magnesium alloy. International Journal on Interactive Design and Manufacturing (IJIDeM), 7, 1–10. https://doi.org/10.1007/s12008-022-01176-6
- Anantha, M. T., Buddi, T., & Boggarapu, N. R. (2023b). Utilisation of fuzzy logic and genetic algorithm to seek optimal corrugated die design for CGP of AZ31 magnesium alloy. Advances in Materials and Processing Technologies, 22, 1–15. https://doi.org/10.1080/2374068X.2023.2192135
- Brandão, P., Infante, V., & Deus, A. M. (2016). Thermo-mechanical modeling of a high pressure turbine blade of an airplane gas turbine engine. Procedia Structural Integrity, 1, 189–196. https://doi.org/10.1016/j.prostr.2016.02.026
- Buddi, T., Singh, S. K., & Rao, B. N. (2018). Optimum process parameters for plywood manufacturing using soya meal adhesive. Materials Today: Proceedings, 5(9), 18739–18744. https://doi.org/10.1016/j.matpr.2018.06.220
- Danthala, S., Rao, S. S., Rao, B. N., & Mannepalli, K. (2021). Multi-objective optimization with modified Taguchi approach to specify optimal robot spray painting process parameters. International Journal of Nonlinear Analysis and Applications, 12(2), 1163–1174. https://doi.org/10.22075/ijnaa.2021.5193
- Dharmendra, B. V., Kodali, S. P., & Rao, B. N. (2019). A simple and reliable Taguchi approach for multi-objective optimization to identify optimal process parameters in nano-powder-mixed electrical discharge machining of INCONEL800 with copper electrode. Heliyon, 5(8), e02326. https://doi.org/10.1016/j.heliyon.2019.e02326
- Dharmendra, B. V., Kodali, S. P., & Boggarapu, N. R. (2020). Multi-objective optimization for optimum abrasive water jet machining process parameters of Inconel718 adopting the Taguchi approach. Multidiscipline Modeling in Materials and Structures, 16(2), 306–321. https://doi.org/10.1108/MMMS-10-2018-0175
- Ghosh, N., Ravi, Y. B., Patra, A., Mukhopadhyay, S., Paul, S., Mohanty, A. R., & Chattopadhyay, A. B. (2007). Estimation of tool wear during CNC milling using neural network-based sensor fusion. Mechanical Systems and Signal Processing, 21(1), 466–479. https://doi.org/10.1016/j.ymssp.2005.10.010
- Goyal, K. K., Sharma, N., Dev Gupta, R., Singh, G., Rani, D., Banga, H. K., Kumar, R., Pimenov, D. Y., & Giasin, K. (2022). A soft computing-based analysis of cutting rate and recast layer thickness for AZ31 alloy on WEDM using RSM-MOPSO. Materials, 15(2), 635. https://doi.org/10.3390/ma15020635
- Hsiang, S. H., & Lin, Y. W. (2019). Optimization of the extrusion process for magnesium alloy sheets using the fuzzy-based Taguchi method. The Arabian Journal for Science and Engineering, 34(1C), 175–185. https://doi.org/10.1007/s12289-011-1041-z
- Jiang, M. G., Yan, H., & Chen, R. S. (2015). Twinning, recrystallization and texture development during multi-directional impact forging in an AZ61 Mg alloy. Journal of Alloys and Compounds, 650, 399–409. https://doi.org/10.1016/j.jallcom.2015.07.281
- Jiang, Y., Guan, L., & Tang, G. (2016). Recrystallization and texture evolution of cold-rolled AZ31 Mg alloy treated by rapid thermal annealing. Journal of Alloys and Compounds, 656, 272–277. https://doi.org/10.1016/j.jallcom.2015.09.155
- Jouini, N., Ruslan, M. S., Ghani, J. A., & Che Haron, C. H. (2023). Sustainable high-speed milling of magnesium alloy AZ91D in dry and cryogenic conditions. Sustainability, 15(4), 3760. https://doi.org/10.3390/su15043760
- Karthik, R., Viswanathan, R., Balaji, J., Sivashankar, N., & Arivazhagan, R. (2021). Optimization of WEDM parameters for machining of AZ31B Mg alloy using Taguchi method. IOP Conference Series: Materials Science and Engineering, 1013(1), 012005. https://doi.org/10.1088/1757-899X/1013/1/012005
- Konduri, S. S., Kalavala, V. M., Mandala, P., Manapragada, R. R., & Boggarapu, N. R. (2017). Application of Taguchi approach to seek optimum drilling parameters for woven fabric carbon fibre/epoxy laminates. MAYFEB Journal of Mechanical Engineering, 1. https://doi.org/10.11648/J.AJNAME.20160101.11
- Koneru, S., Srinath, A., & Nageswara Rao, B. (2022). Multi objective optimization for the optimal heat pipe working parameters based on Taguchi’s design of experiments. Heat Transfer, 51(3), 2510–2523. https://doi.org/10.1002/htj.22410
- Koneru, S., Srinath, A., Rao, B. N., & Buddi, T. (2023). Simplified optimal design of NU202 cylindrical roller bearing and validation through GA. International Journal on Interactive Design and Manufacturing (IJIDeM), 22, 1–2. https://doi.org/10.1007/s12008-023-01402-9
- Kurra, S., & Regalla, S. P. (2015). Multi-objective optimisation of single point incremental sheet forming using Taguchi-based grey relational analysis. International Journal of Materials Engineering Innovation, 6(1), 74–90. https://doi.org/10.1504/IJMATEI.2015.069802
- Lu, L., Hu, S., Liu, L., & Yin, Z. (2016). High speed cutting of AZ31 magnesium alloy. Journal of Magnesium and Alloys, 4(2), 128–134. https://doi.org/10.1016/j.jma.2016.04.004
- Miladinovic, S., Gajević, S., Devada, L., & Boggarapu, N. R. (2021). Parameters identification and minimization of safety coefficient for surface durability of internal planetary gear using the modified Taguchi approach. Test Engineering and Management. https://scidar.kg.ac.rs/handle/123456789/16600
- Moradpour, M., Khodabakhshi, F., & Eskandari, H. (2018). Microstructure–mechanical property relationship in an Al–Mg alloy processed by constrained groove pressing-cross route. Materials Science and Technology, 34(8), 1003–1017. https://doi.org/10.1080/02670836.2017.1416906
- Mordike, B. L., & Ebert, T. (2001). Magnesium: properties—applications—potential. Materials Science and Engineering: A, 302(1), 37–45.(00)01351-4 https://doi.org/10.1016/S0921-5093
- Muni Tanuja, A., Kumar, A., & Nageswara Rao, B. (2022). Review on the application of CGP to improve AZ31 Mg alloy properties. Applications of computational methods in manufacturing and product design: Select proceedings of IPDIMS 2020 (pp. 237–246). Springer Nature Singapore. https://doi.org/10.1007/978-981-19-0296-3_21
- Ömer, A. S. (2019). Optimization of surface roughness in turning of AZ31 magnesium alloys with Taguchi method. Gazi University Journal of Science Part A: Engineering and Innovation, 6(1), 25–32. http://dergipark.gov.tr/gujsa
- Rajyalakshmi, K., & Boggarapu, N. R. (2018). Expected range of the output response for the optimum input parameters utilizing the modified Taguchi approach. Multidiscipline Modeling in Materials and Structures, 15(2), 508–522. https://doi.org/10.1108/MMMS-05-2018-0088
- Rajyalakshmi, K., & Rao, B. N. (2019). Modified Taguchi approach to trace the optimum GMAW process parameters on weld dilution for ST-37 steel plates. Journal of Testing and Evaluation, 47(4), 3209–3223. https://doi.org/10.1520/JTE20180617
- Ramya, K. U., Koneru, S. I., & Santhosh Kumar, R. (2018). Multivariable optimization of surface grinding process using genetic algorithm. International Journal of Mechanical and Production Engineering Research and Development, 8(2), 77–486. https://doi.org/10.1007/s001700170167
- Ross P. J. (1989). Taguchi techniques for quality engineering. McGraw-Hill.
- Sanjeevannavar, M. B., Banapurmath, N. R., Soudagar, M. E., Atgur, V., Hossain, N., Mujtaba, M. A., Khan, T. Y., Rao, B. N., Ismail, K. A., & Elfasakhany, A. (2022). Performance indicators for the optimal BTE of biodiesels with additives through engine testing by the Taguchi approach. Chemosphere, 288(Pt 2), 132450. https://doi.org/10.1016/j.chemosphere.2021.132450
- Santhakumar, J., & Iqbal, U. M. (2022 Optimization of process variables for surface roughness and tool wear deviation of AZ31 alloy during face milling [Paper presentation]. AIP Conference Proceedings (Vol. 2460). https://doi.org/10.1063/5.0095647
- Saritha, P., Satyadevi, A., & Raju, P. R. (2019). Investigation of surface roughness on hybrid composites using stir casting technique. I-Manager’s Journal on Mechanical Engineering, 9(3), 26. https://doi.org/10.26634/jme.9.3.15891
- Satyanarayana, G., Narayana, K. L., & Nageswara Rao, B. (2018). Identification of optimum laser beam welding process parameters for E110 zirconium alloy butt joint based on Taguchi-CFD simulations. Lasers in Manufacturing and Materials Processing, 5(2), 182–199. https://doi.org/10.1007/s40516-018-0061-7
- Satyanarayana, G., Narayana, K. L., & Nageswara Rao, B. (2019). Optimal laser welding process parameters and expected weld bead profile for P92 steel. SN Applied Sciences, 1(10), 1. https://doi.org/10.1007/s42452-019-1333-3
- Satyanarayana, G., Narayana, K. L., & Rao, B. N. (2021). Incorporation of Taguchi approach with CFD simulations on laser welding of spacer grid fuel rod assembly. Materials Science and Engineering: B, 269, 115182. https://doi.org/10.1016/j.mseb.2021.115182
- Singaravelu, J., Jeyakumar, D., & Nageswara Rao, B. (2012). Reliability and safety assessments of the satellite separation process of a typical launch vehicle. The Journal of Defense Modeling and Simulation: Applications, Methodology, Technology, 9(4), 369–382. https://doi.org/10.1177/1548512911401939
- Sivam, S. S. S., Karuppaiah, S. M., Yedida, B. K., Atluri, J. R., & Mathur, S. (2018). Multi response optimization of setting input variables for getting better product quality in machining of magnesium AM60 by grey relation analysis and ANOVA. Periodica Polytechnica Mechanical Engineering, 62(2), 118–125. https://doi.org/10.3311/PPme.11034
- Sivam, S. S., Saravanan, K., Pradeep, N., Moorthy, K. S., & Rajendrakumar, S. (2018). Grey relational analysis and anova to determine the optimum process parameters for friction stir welding of Ti and Mg alloys. Periodica Polytechnica Mechanical Engineering, 62(4), 277–283. https://doi.org/10.3311/PPme.12117
- Tan, J., & Ramakrishna, S. (2021). Applications of magnesium and its alloys: A review. Applied Sciences, 11(15), 6861. https://doi.org/10.3390/app11156861
- Wang, T., Zhu, T., Sun, J., Wu, R., & Zhang, M. (2015). Influence of rolling directions on microstructure, mechanical properties and anisotropy of Mg-5Li-1Al-0.5 Y alloy. Journal of Magnesium and Alloys, 3(4), 345–351. https://doi.org/10.1016/j.jma.2015.11.001
- Wankhede, P., Ram, M. V. K., Suresh, K., & Priyadarshini, A. (2023). Integrated fuzzy AHP and fuzzy TOPSIS for multi response optimization in incremental forming process. International Journal on Interactive Design and Manufacturing (IJIDeM), 17(2), 83–95. https://doi.org/10.1007/s12008-023-01246-3
- Zagórski, I., Szczepaniak, A., Kulisz, M., & Korpysa, J. (2022). Influence of the tool cutting edge helix angle on the surface roughness after finish milling of magnesium alloys. Materials, 15(9), 3184. https://doi.org/10.3390/ma15093184
- Zakaria, M. S., Mustapha, M., Azmi, A. I., Ahmad, A., Danish, M., & Rubaiee, S. (2022). Machinability investigations of AZ31 magnesium alloy via submerged convective cooling in turning process. Journal of Materials Research and Technology, 19, 3685–3698. https://doi.org/10.1016/j.jmrt.2022.06.127