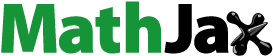
Abstract
The uncertainty of in situ radioactivity measurements can be influenced by the depth of sediment sampled by the spectrometer and the vertical distribution of the target radionuclide in the sediments. This study used Particle and Heavy Ion Transport Simulation code (PHITS) to evaluate the effective detection depth of a plastic scintillation fiber system (PSF) for monitoring radiocesium (RCs) in bottom sediments of agricultural ponds in Fukushima and thereafter, used 2015–2019 field measurement data from 47 ponds to evaluate the depth dependence of PSF calibration factors and its influence on measurement uncertainty. Though no significant differences were observed in PSF-derived RCs concentrations by calibration depth, PSF calibration factors and normalized mean square error (NMSE) suggest a potential dependence on the depth of sediment containing 90% of RCs inventory (L90) such that it may be the optimal choice for calibrating PSF. Accordingly, revision of PSF calibration depth from the currently adopted top 10 cm to the top 15–20 cm may be necessary for optimizing PSF measurement uncertainty not only because their calibration factors had the best coefficients of determination and yielded lowest NMSE, but also because they constituted the largest proportion of L90 among the 206 samples.
REVIEWING EDITOR:
Introduction
After the radioactive fallout from accident at Fukushima Daiichi Nuclear Power Station (FDNPS), Japan Atomic Energy Agency (JAEA) developed plastic scintillation fibers (PSFs) for monitoring radiocesium (RC; 134 + 137Cs, hereon RCs; T½: 2 y and 30 y, respectively) distribution over wide areas of bottom sediments in agricultural ponds in Fukushima, which has over 3500 reservoirs that can accumulate and spread RCs (Yoshimura et al., Citation2014; Yamada et al., Citation2015). RCs heterogeneities across any type of reservoir terrain can be rapidly identified by PSF because of its flexibility and excellent spatial resolution (50 cm) over its 20 m length (Sanada et al., Citation2014; Sanada et al., Citation2015).
Initially, PSF’s counting rate (CRpsf, s−1) was converted to RCs concentration (Bq kg−1) by regressing it on the RCs concentration measured by a 3′′ x 3′′ cerium-doped lanthanum bromide (LaBr3:Ce) in situ spectrometer (Sanada et al., Citation2014; Sanada et al., Citation2015), which was calibrated by Monte Carlo simulation (Sanada et al., Citation2014; Sanada et al., Citation2015). This approach was validated by good agreements in RCs concentration estimated by the PSF and the laboratory-measured mean concentration for the top 10 cm of sediments sampled from points coinciding with PSF measurement lines in 20 ponds. Later, PSF calibration factors, CFpsf {(Bq kg−1).(s−1)−1}, were evaluated by comparing CRpsf directly to the mean RCs concentration in the top 10 cm of sediment core samples (Katengeza et al., Citation2020).
Among the sources of uncertainty associated with in situ bottom sediment radioactivity measurements with gamma-ray spectrometers are the sediment thickness used for calibration being unrepresentative of that ‘sampled’ by the detector and the non-uniform vertical distribution of radioactivity (Jones et al., Citation1984; Jones et al., Citation1988; Ohnishi et al., Citation2016). Ohnishi et al. (Citation2016) further posited the dependence of CF on the depth of sediment, l, over which the activity concentration is averaged, but their study focused on the influence of detector burial considering only one mixing depth (l = 3 cm). For PSF, the calibration depth (lcal) is important because CFpsf are obtained by direct comparison with the average concentration within that depth of sediment. It is important to clarify the effect of calibration depth on PSF calibration, measurement uncertainty, and systematic biases. Accordingly, the aims of this study were to: (a) estimate the effective detection depth for PSF, (b) evaluate the depth dependence of CFpsf and their influence on PSF measurement uncertainty and systematic biases.
Materials and methods
PSF effective detection depth
PSF’s effective detection depth for RCs (137Cs) in bottom sediment was evaluated by Monte Carlo simulation with Particle and Heavy Ion Transport Simulation code (PHITS) version 3.22 (Ibaraki, Japan) which is a revision of the earlier reported PHITS3.02 (Sato et al., Citation2018). The PSF system () comprises (Sanada et al., Citation2014; Sanada et al., Citation2015) a bundle of nineteen 20 m long 1 mm-diameter fiber sensors (SCSF-3HF, Kuraray Co. Ltd, Tokyo, Japan) optically coupled at both ends to photomultiplier tubes (PMT); constant-fraction discriminators to shape PMT’s electrical signal; a time-to-amplitude converter to measure the difference in time of flight (TOF) of directional scintillation light from their production point within the fibers to the PMT; a multichannel analyzer (MCA) to sort the TOF into specific channels corresponding to position; and a computer to display the positional spectrum.
Figure 1. (a) PSF system showing (a.i) field measurement arrangement, (a.ii–iii) bundled fibers coupled to PMT, (b) Geometry in PHITS showing (b.i) the PSF location on the water-sediment interface and (b.ii–iii) the constituents’ composition as depicted in PHITS.

In PHITS (), the 19-fiber PSF bundle was modeled as one 20 m long fiber of radius 1.4 cm covered by a 0.2 cm thick PVC layer. The PSF's core, and inner and outer claddings contributed 96%, 2% and 2%, respectively, to the simulated diameter according to manufacturer specifications for SCSF-3HF fibers (Kuraray Co. Ltd., Citation2017). summarizes the constituents’ composition. Sediment composition (53.3% H, 40.0% O and 6.7% Si) was adopted from the PSF’s initial calibration in 2013 (Sanada et al., Citation2014; Sanada et al., Citation2015), but the density (1.3 g cm−3) was taken from the average of the 2015–2019 samples studied herein. Focusing on the longer-lived RCs isotope, photons (107) from point and plane 8 kBq 137Cs radioisotope sources were tracked and their flux (cm−2 source−1) in PSF’s core region were plotted as a spectrum of range 0–662 keV using T-deposit tally. The point and plane sources were modeled as a dot and a 28 by 28 m square source, respectively, with the latter representing the area of a relatively small pond. The source position was varied in 5 cm increments from the sediment surface (z = 0) to a depth where the flux of 662 keV gamma photons (Фfull) in the PSF core region was so diminished that the peak was indistinct. The effective detection depth was below this depth.
Table 1. Composition of material constituents simulated in PHITS.
PSF depth-dependent calibration factors and measurement uncertainty
In 2015–2019, PSF-sediment monitoring campaigns were conducted in numerous agricultural ponds. CRpsf were acquired in three to six minutes with 5 m separation between lines and sediment core (length ≈ 30 cm) samples were collected with HR-type corer (Rigo Co., Tokyo, Japan) from points coinciding with the PSF measurement lines (). To evaluate the depth dependence of PSF calibration and its influence on PSF measurement uncertainty, PSF-sediment datasets (N = 206) from 47 ponds within 80 km of FDNPS were selected. With varying shapes, the ponds’ average depth and area were 1.92 m (0.3–4.95 m) and 18,088 m2 (231–92,090 m2), respectively and about 70% were located in forest catchments, 15% in paddy catchments and 15% in urban areas. Some of PSF-sediment data (N = 60) were used in a previous study (Katengeza et al., Citation2020) for calibrating PSF measurements in five of the 47 ponds using the top 10 cm of the sediments. RCs inventory (IRCs, Bq m−2) and concentration (ARCs on wet basis, Bq kg−1wet) in 5 cm sections of sediment core samples were determined by gamma-spectrometry with a p-type high purity germanium detector (Ortec Seiko EG & G, Woodmere, New York, USA) coupled to a multichannel analyzer (Ortec Seiko EG & G, Woodmere, New York, USA) and calibrated by standard gamma radiation sources (Radioisotope Association of Japan). Uniformity in ARCs with depth was evaluated by statistical tests (t-test) on successive 5 cm layers and the thickness of sediment containing 90% of the total core IRCs (L90) was estimated by the cumulative inventory (IRCs,n) in n successive 5 cm sediment layers where EquationEquation (1)(1)
(1) approximated 90%.
(1)
(1)
To obtain depth-dependent CFpsf, 30 cm long sediment samples whose ARCs dropped off to <61 Bq kg−1, PSF’s detection limit (Sanada et al., Citation2015), were selected for each year () and the average ARCs over sediment length, lcal (calibration depth), from the core’s surface was calculated in 5 cm increments from lcal = 5 cm to lcal = 30 cm. Next, CRpsf was regressed on the average ARCs for each lcal and the slope of the regression line was adopted as the CFpsf for that specific calibration depth, i.e. CFl (). Depth-dependent PSF-derived RCs concentration (
), in Bq kg−1, were obtained by multiplying CRpsf with CFl. illustrates the process for lcal = 15 cm using an example RCs depth profile from a 2015 sediment core sample () and calibration data (). The statistical uncertainty of CFl was propagated from CRpsf and
The use of PSF as gamma ray sensors emphasizes the excellence in proportionality between CRpsf and ARCs of calibration sources (Park & Kim, Citation2004). Accordingly, the degree of association between CRpsf and
was used to indicate depth-dependence of PSF calibration under the position that differences in PSF calibration coefficients of determination (lR2) arose from RCs depth distribution and an optimal calibration depth (loptimal) would maximize lR2 and minimize PSF measurement uncertainty (EquationEquation (2)
(2)
(2) ).
(2)
(2)
where NMSE is the normalized mean square error which quantifies the difference in measurement results obtained by two methods. Analysis of variance on
was conducted to test for significance in the differences by calibration depth, while systematic biases of
were evaluated as the relative deviation using EquationEquation (3)
(3)
(3) :
(3)
(3)
Figure 2. An illustration of the calibration process indicating (a) an example RCs concentration depth profile extracted from 2015 samples with 15 cm calibration depth (lcal = 15 cm) highlighted, and (b) the regression of PSF counting rate (CRpsf) on the average RCs concentration in the top 15 cm of sediment core samples () to obtain CF15 for 2015. Also shown in (b) are the 95% prediction (pred) and confidence (conf) intervals.

Table 2. Summary of datasets for calibrating and validating PSF in 2015–2019.
All statistical analyses were conducted in Microsoft Excel (Microsoft Corporation, 2018).
Results and discussion
PSF effective detection depth
Reflected in is Compton Scattering as the main gamma-ray interaction with PSF (Oka et al., Citation1998). The higher photon flux for the point source () was because the same number of photons (107) was tracked for both source types, and the plane source was spread over a wider area. The detected flux’s statistical uncertainty was 3–28%. Full energy (662 keV) photon flux (Фfull) decreased with source depth in sediments. At 20 cm, the 662 keV peak was distinct but Фfull approximately equal to low energy (<200 keV) scattered photons (Фlow). Beyond 20 cm, Фlow exceeded Фfull whose peak remained distinctive until 35 cm. PSF effective detection depth was approximated as 30 cm where the 662 keV peak was clearly detectable, and the plane and point source Фfull converged. This result is consistent with the 30–50 cm effective detection depth of most underwater in situ gamma spectrometers for bottom sediments (Jones et al., Citation1984; Ohnishi et al., Citation2016; Lee et al., Citation2020). The approach adopted in our study is consistent with Lee et al. (Citation2020) who used the energy spectra obtained by experiments in a water tank to decide on their spectrometer’s 50 cm effective detection depth. They observed a trend like that simulated for the PSF with the peak indistinctive for a point source located beyond 50 cm. Their Monte Carlo simulated spectra were consistent with experiments when energy window was applied such that only photons originating within 50 cm were detected (Lee et al., Citation2020). Relying on the results of Monte Carlo simulation for PSF’s effective detection depth is consistent with other studies that relied on simulation (Androulakaki et al., Citation2015) or calculation (Jones et al., Citation1984).
Figure 3. (a.i–xi) Energy distribution of photon flux in PSF core for a point source located at varying depths (z = 0 to z = –40 cm) in sediments and (b) Changes in photon flux in PSF core with point and plane source position in bottom sediments. The point and plane source converged at the evaluated effective detection depth (30 cm).

PSF depth-dependent calibration factors and measurement uncertainty
summarizes the distribution of L90, estimated by EquationEquation (1)(1)
(1) . Overall, L90 approximated 5 cm, 10 cm, 15 cm, 20 cm, 25 cm and 30 cm for 3%, 26%, 33%, 26%, 13% and 7% of the samples collected in 2015–2019 confirming the downward migration of RCs such that only 29% of the samples had L90 in the top 10 cm of sediments currently used for calibrating PSF. This deep distribution may be from sedimentation, vertical and lateral mixing, diffusion and other factors (Broberg, Citation1994; Otosaka, Citation2017; Tsuji et al., Citation2019). This study focuses on the potential influence of RCs depth distribution on PSF calibration and measurement uncertainty. Accordingly, we emphasize L90, while detailed analyses of depth profiles and their causes will be reported elsewhere. The isotopic signature of FDNPS-derived RCs was a ratio of one for 134Cs to 137Cs on the reference RCs deposition date, 15 March 2011 (Chino et al., Citation2016), and all the samples were within 3- 4% of the expected ratio with correction for radioactive decay confirming FDNPS origin.
Table 3. Distribution (%) of L90 amongst the sediment samples.
The distribution of CFl (propagated standard uncertainty: 1–7%) and corresponding iR2 (lcal) are shown in . Generally, the magnitude of CFl had an increasing trend with time in years (R2 = 0.59) pointing to the need to use the most recent field measurement data for calibrating PSF. This increasing CFl over time can be explained by the deeper penetration of RCs into sediment such that the ARCs required to register a unit count within the PSF increases with the radionuclide’s penetration. This is consistent with the earlier observed simulation result () where fewer counts were registered as the source distributed deeper into sediment and the distribution of L90 over the study period.
Figure 4. Distribution of (a) PSF depth-dependent calibration factors (CFl), (b) their coefficients of determination (lR2) for each year and (c) the entire 2015–2019 period.

The lR2 tended to rise, peak, and drop or plateau with increasing lcal for all years except 2016 where the plateau extended over lcal = 5–15 cm before falling off. The peak or plateau lR2 occurred at 15 cm in 2015–2018, and at 20 cm in 2019 () which also comprised the largest proportion of L90 (). This indicates lcal = 15 cm and l = 20 cm as loptimal with a potential dependence on L90. Considering the entire calibration data for the 2015–2019 period together (), the same trend was observed with lcal = 15 cm as loptimal. Because PSFl () was not normally distributed (Shapiro–Wilk test, p < 0.05), its depth dependence was tested by Kruskal–Wallis H test on the PSFl groups (PSF5–PSF30). There were no statistically significant differences in median PSFl (p > 0.05) suggesting that calibration depth may not be an important factor. To clarify this finding, the measurement uncertainty, and systematic biases of PSFl were evaluated.
Figure 5. Distribution of RCs concentration (a) derived by PSF for varying calibration depths (PSFl), (b) in successive 5 cm layers of bottom sediments sampled from 47 ponds in 2015–2019. Median is shown by horizontal lines in the boxes which cover the first to third quartiles with whiskers extending from and to the minima and maxima, respectively.

Consistent with the hypothesized trend for loptimal, NMSE () minima occurred at iR2 peak or plateau inception for all but 2018. For 2015, 2017 and 2019, the NMSE decreased with increasing lcal to a minimum at loptimal after which it increased. These years’ NMSE minima also coincided the largest proportion L90 (). Like NMSE, 2015’s RD was least biased at lcal = 15 cm (loptimal). RD’s for 2017 and 2019 became more positively biased and less negatively biased with increasing lcal, respectively (). The NMSE over 2016’s iR2 plateau (≈loptimal = 5–15 cm) also increased with lcal. However, it dropped from 17% at lcal = 15 cm to 9% at lcal = 20 cm before continuing to increase with lcal. The largest proportion of L90 for 2016 was also 20 cm further supporting the potential influence of L90 on PSF measurement uncertainty such that loptimal ≈ L90. Additionally, the 2016 RD for lcal = 5–20 cm were comparable (1–2%) indicating similar biases across this lcal range. The 2018 NMSE also increased with lcal before and after lcal = 10 cm while the RD at lcal = 5 cm was comparable to RD at lcal = 10 cm and became more negatively biased with lcal. Occurrence of 2018’s NMSE minimum (14%) at lcal = 10 cm instead of loptimal ≈L90 = 15 cm could be related to the distribution of ARCs in sediment layers.
Table 4. Variation of NMSE (%) and mean RD (%) for PSF-derived RCs concentration (PSFl) at each calibration depth.
The box and whisker plot in shows the distribution of ARCs in successive 5 cm layers of all sediment core samples from the 47 ponds in the 2015–2019 period. Because the data was not normally distributed (Shapiro–Wilk test, p < 0.05) and Kruskal–Wallis H-test demonstrated that there was a difference (p < 0.05) in ARCs in at least one of the sediment layers, uniformity of ARCs by depth was tested by Mann–Whitney U-tests on ARCs in successive 5 cm layers the whole 2015–2019 period. Significant ARCs differences (p < 0.05) were observed between 10–15 cm and 15–20 cm layers, and 15–20 cm and 20–25 cm layers. From a statistical perspective, 0–5 cm and 5–10 cm layers were uniformly distributed because no significant differences were observed therein (Rostron et al., Citation2015; Rostron et al., Citation2014). This possibly explains the relatively symmetrical distribution of RD about the mean for lcal = 10 cm compared to other lcal. The inclusion of the other layers for calibrating PSF15–PSF30 likely skewed the results and influenced the observed RD and NMSE, particularly for 2018. Generally, PSF overestimated the RCs concentration 2015–2017 and underestimated RCs concentration in 2018 and 2019 (RD in ).
The current practice of using lcal = 10 cm may be justified by the observed uniformity in ARCs for the top 10 cm, relative symmetry of RD about the mean for lcal = 10 cm, and NMSE ≤ 15% for the whole 2015–2019 period for lcal = 10 cm. Also, all iR2 values were >0.8 indicating good linearity between CRpsf and sediment RCs concentration irrespective of calibration depth. However, the current findings suggest a potential dependence of PSF calibration factors, measurement uncertainty, and systematic biases on the depth of sediment containing 90% of RCs inventory. Accordingly, revision of PSF calibration depth may be necessary for optimizing measurement uncertainty. As RCs distributes more deeply into sediment, the proportion of photons originating from deep within sediments would increase and become more important contributors to CRpsf.
The NMSE and RD observed herein are comparable to those reported in other studies, such as Ochi et al. (Citation2018) who reported a 15% mean RD for 137Cs bottom sediment measurements in 64 ponds with A-subD, an underwater thallium-doped sodium iodide (NaI:Tl) in situ gamma-ray spectrometer. Ohnishi et al. (Citation2016) reported root mean square errors of 17% (RD: 8–58%) and 4.8% (RD: −14–6.3%) for 137Cs measurements at the mouth of Abukuma river and Sendai Bay, respectively, with another underwater in situ NaI:Tl spectrometer called RESQ hose.
Conclusion
This study demonstrated by Monte Carlo simulation an effective detection depth of approximately 30 cm for the JAEA-developed PSF system. The depth dependence of PSF calibration factors, PSF-derived RCs concentrations, and their corresponding measurement uncertainty were also evaluated. Though no significant differences were observed in PSF-derived RCs concentrations by calibration depth, PSF calibration coefficients and NMSE suggest a potential dependence on the depth containing 90% of RCs inventory such that it may be the optimal choice for calibrating PSF. Accordingly, revision of PSF calibration depth from the currently adopted top 10 cm sediment RCs concentration to that in the top 15–20 cm may be necessary for optimizing PSF measurement uncertainty not only because their calibration factors had the best coefficients of determination and yielded lowest NMSE, but also because they constituted the largest proportion containing 90% of the RCs inventory in sediment samples from 47 ponds collected in 2015–2019. This revision should be guided by the rate of RCs migration and the distribution of its inventory in sediments based on monitoring data. Based on the ponds studied herein, the top 10 cm can be utilized for PSF calibration in the first five years followed by the top 15 − 20 cm for the subsequent 5 years.
Acknowledgments
Japan Atomic Energy Agency and Midori Net Fukushima are gratefully acknowledged for assistance with field measurements and laboratory analyses.
Disclosure statement
Authors report that there are no competing interests to declare.
Additional information
Funding
Notes on contributors
Estiner W. Katengeza
Estiner W. Katengeza holds a PhD in Environmental Studies obtained from The University of Tokyo in 2022. She is a Lecturer at the Malawi University of Business and Applied Sciences’ Department of Physics and Biochemical Sciences with specializations in Physics (Applied Nuclear Physics, Energy, Environmental Sciences). Her research interests include environmental radioactivity, radiation measurement and protection, and energy. Her current research work includes natural background radiation and radon concentration measurements.
Yukihisa Sanada
Yukihisa Sanada is a Senior Principal Engineer at the Japan Atomic Energy Agency’s Sector of Fukushima Research and Development in Fukushima, Japan. He obtained a PhD in Science from Niigata University in 2006. His research interests include radiation measurement and environmental radioactivity. His work after the Fukushima Daiichi NPP accident has focused on remote radiation surveys including airborne radiation monitoring, autonomous unmanned helicopters, and developing a new type of detector for visualizing radiation distribution in forests.
Kotaro Ochi
Kotaro Ochi is a Research Engineer at the Japan Atomic Energy Agency’s Sector of Fukushima Research and Development in Fukushima, Japan. He obtained a PhD in Environmental Studies from The University of Tokyo in 2023. His research interests also include radiation measurement and environmental radioactivity. His research since 2016 has been on radiocesium inventory assessments in terrestrial soils and water bodies. Currently, he is working on research and development of remote measurement techniques using drones equipped with radiation detectors.
Takeshi Iimoto
Takeshi Iimoto is a Professor in the Division of Environmental Studies at The University of Tokyo’s Graduate School of Frontier Sciences. He graduated with a PhD from Waseda University in 1996. His laboratory’s research field is broadly classified as Environmental Safety Management and covers all studies on safety and risk management, particularly in radiation environments.
References
- Androulakaki, E. G., Tsabaris, C., Eleftheriou, G., Kokkoris, M., Patiris, D. L., & Vlastou, R. (2015). Seabed radioactivity based on in situ measurements and Monte Carlo simulations. Applied Radiation and Isotopes: Including Data, Instrumentation and Methods for Use in Agriculture, Industry and Medicine, 101, 83–92. https://doi.org/10.1016/j.apradiso.2015.03.013
- Broberg, A. (1994). The distribution and characterization of 137Cs in lake sediments. Studies in Environmental Science, 62, 45–62. https://doi.org/10.1016/S0166-1116(08)71699-6
- Chino, M., Terada, H., Nagai, H., Katata, G., Mikami, S., Torii, T., Saito, K., & Nishizawa, Y. (2016). Utilization of 134Cs/137Cs in the environment to identify the reactor units that caused atmospheric releases during the Fukushima Daiichi accident. Scientific Reports, 6(1), 31376. https://doi.org/10.1038/srep31376
- Jones, D. G., Miller, J. M., & Roberts, P. D. (1984). The distribution of 137Cs in surface intertidal sediments from the Solway Firth. Marine Pollution Bulletin, 15(5), 187–194. https://doi.org/10.1016/0025-326X(84)90318-7
- Jones, D. G., Roberts, P. D., & Miller, J. M. (1988). The distribution of gamma-emitting radionuclides in surface subtidal sediments near the Sellafield plant. Estuarine, Coastal and Shelf Science, 27(2), 143–161. https://doi.org/10.1016/0272-7714(88)90087-X
- Katengeza, E. W., Sanada, Y., Yoshimura, K., Ochi, K., & Iimoto, T. (2020). The ecological half-life of radiocesium in surficial bottom sediments of five ponds in Fukushima based on: In situ measurements with plastic scintillation fibers. Environmental Science. Processes & Impacts, 22(7), 1566–1576. https://doi.org/10.1039/d0em00160k
- Kuraray Co. Ltd. (2017). Plastic scintillating fibers: Scintillating fibers, wavelength shifting fibers, clear fibers. Data Sheet. http://kuraraypsf.jp/pdf/all.pdf.
- Lee, S., Lee, J. S., Kim, H. S., Park, J., Baek, S., Song, Y., Seo, J.-M., & Kim, S. M. (2020). In-situ remotely controllable ocean radiation monitoring system. Journal of Instrumentation, 15(06), P06027–P06027. https://doi.org/10.1088/1748-0221/15/06/P06027
- Ochi, K., Urabe, Y., Yamada, T., & Sanada, Y. (2018). Development of an analytical method for estimating three-dimensional distribution of sediment-associated radiocesium at a reservoir bottom. Analytical Chemistry, 90(18), 10795–10802. https://doi.org/10.1021/acs.analchem.8b01746
- Ohnishi, S., Thornton, B., Kamada, S., Hirao, Y., Ura, T., & Odano, N. (2016). Conversion factor and uncertainty estimation for quantification of towed gamma-ray detector measurements in Tohoku coastal waters. Nuclear Instruments and Methods in Physics Research Section A, 819, 111–121. https://doi.org/10.1016/j.nima.2016.02.065
- Oka, T., Fujiwara, H., Takashima, K., Usami, T., & Tsutaka, Y. (1998). Development of fiber optic radiation monitor using plastic scintillation fibers. Journal of Nuclear Science and Technology, 35(12), 857–864. https://doi.org/10.3327/jnst.35.857
- Otosaka, S. (2017). Processes affecting long-term changes in 137Cs concentration in surface sediments off Fukushima. Journal of Oceanography, 73(5), 559–570. https://doi.org/10.1007/s10872-017-0421-5
- Park, J. W., & Kim, G. H. (2004). Detection of gamma rays using plastic scintillating fibers. Journal of Nuclear Science and Technology, 41(4), 373–376. https://www.tandfonline.com/doi/abs/10.1080/00223131.2004.10875724.
- Rostron, P. D., Heathcote, J. A., & Ramsey, M. H. (2014). Comparison between in situ and ex situ gamma measurements on land areas within a decommissioning nuclear site: A case study at Dounreay. Journal of Radiological Protection, 34(3), 495–508. https://doi.org/10.1088/0952-4746/34/3/495
- Rostron, P. D., Heathcote, J. A., & Ramsey, M. H. (2015). Evaluation of uncertainties in in situ and ex situ gamma measurements on land areas with low contamination levels. Journal of Radiological Protection, 35(2), 391–399. https://doi.org/10.1088/0952-4746/35/2/391
- Sanada, Y., Takamura, Y., Urabe, Y., Tuchida, K., Nishizawa, Y., Yamada, T., Sato, Y., Hirayama, H., Nishihara, K., Imura M., Ishida, M., Ishibashi, S., Sase, T., Suzuki, M., Mori, H., Yonezawa, S., Torii, T. (2014). Research and development of in-situ measurement for radiation distribution of waterbed [Internet]. JAEA-Research 2014-005. Ibaraki. https://jopss.jaea.go.jp/pdfdata/JAEA-Research-2014-005.pdf.
- Sanada, Y., Urabe, Y., Orita, T., Takamura, Y., & Torii, T. (2015). ICONE23-2120 In-situ measurement of radiation distribution in bottom sediments of irrigation ponds using plastic scintillation fiber. The Proceedings of the International Conference on Nuclear Engineering (ICONE) (Vol. 2015–23). The Japan Society of Mechanical Engineers. https://doi.org/10.1299/jsmeicone.2015.23._ICONE23-2_46
- Sato, T., Iwamoto, Y., Hashimoto, S., Ogawa, T., Furuta, T., Abe, S. I., Kai, T., Tsai, P. E., Matsuda, N., Iwase, H., Shigyo, N., Sihver, L., & Niita, K. (2018). Features of particle and heavy ion transport code system (PHITS) version 3.02. Journal of Nuclear Science and Technology, 55(6), 684–690. https://doi.org/10.1080/00223131.2017.1419890.
- Tsuji, H., Tanaka, A., Komatsu, K., Kohzu, A., Matsuzaki, S. I S., & Hayashi, S. (2019). Vertical/spatial movement and accumulation of 137Cs in a shallow lake in the initial phase after the Fukushima Daiichi nuclear power plant accident. Applied Radiation and Isotopes: Including Data, Instrumentation and Methods for Use in Agriculture, Industry and Medicine, 147, 59–69. https://doi.org/10.1016/j.apradiso.2019.02.009
- Yamada, S., Kitamura, A., Kurikami, H., Yamaguchi, M., Malins, A., & Machida, M. (2015). Sediment and 137Cs transport and accumulation in the Ogaki Dam of eastern Fukushima. Environmental Research Letters, 10(1), 014013. https://doi.org/10.1088/1748-9326/10/1/014013
- Yoshimura, K., Onda, Y., & Fukushima, T. (2014). Sediment particle size and initial radiocesium accumulation in ponds following the Fukushima DNPP accident. Scientific Reports, 4(1), 4514. https://doi.org/10.1038/srep04514