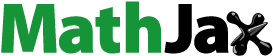
Abstract
In this paper, some exact solutions of the stochastic generalized nonlinear shallow water wave equation are investigated. This equation is important in fluid mechanics’ fields since it can model the propagation of disturbances in water and other incompressible fluids. Opposite to what is usually considered in the literature, the two dispersion coefficients of the nonlinear terms are considered dependent random quantities as a more realistic case. The modified extended-tanh function (METF) method is combined with the random variable transformation (RVT) technique to get full probabilistic solutions of the problem via computing the probability density functions (PDFs) of the solution processes. Based on the probability density function, any statistical moment of the solution can be evaluated. Through two different applications for the input random variables (dispersion coefficients), my findings are applied efficiently. Finally, numerical results are presented graphically along the spatial dimension at a certain wave speed and time. The obtained results ratify that the proposed technique is efficient and powerful for obtaining analytical probabilistic solutions for the problem.
1. Introduction
The subject of stochastic differential equations (SDEs) (Ghanem and Spanos, Citation2003; Øksendal, Citation2010) has a rapidly developing life of its own as a fascinating research field with many interesting open questions. This is because the SDEs have a wide range of applications outside mathematics and there are many fruitful connections to other mathematical disciplines. The origin of this area of research is the observed uncertainties that are involved in many physical properties. For examples: infection rates, such as epidemic prevalence and social addiction in epidemiology, the diffusion and advection coefficients of mass transport processes in physics, the coefficient of viscosity in fluid mechanics, etc., are variables subject to substantial uncertainty. Thus, their deterministic modelling is clearly unrealistic. This promotes the search for mathematical models that consider randomness in their formulation. The consideration of uncertain quantities in deterministic differential equations (DDE) has demonstrated to be useful in mathematical representations for modelling numerous real problems. Uncertainty can be directly introduced into differential equations by considering coefficients, source function term and/or initial/boundary conditions are random variables (RVs) and/or stochastic processes (SPs). This treatment leads to an area commonly referred to as SDEs. Its substantial goal is to extend classical deterministic results to random forms.
Seeking analytical as well as numerical solutions for SDEs has continued to attract attention through the last few decades. In this area, Intensive studies have been conducted. Currently, they have a considerable impact on the analysis and results of several models in engineering and sciences (He, Citation2009; Hussein et al., Citation2008; Hussein and Selim, Citation2013). Most of these studies are established on mean square calculus and its generalizations (Cortés et al., Citation2012; Golmankhaneh et al., Citation2013). The main results of these contributions are the solution SP and its first and second statistical moments. On the other hand, the more desirable and complicated goal is the computing of the probability density function (PDF) associated to the solution SP. The PDF represents the complete stochastic solution of the problem since from it, all higher order statistical moments of the solution SP can be conducted. In this context, several recent articles have dealt with the computation of PDF in (Bevia et al., Citation2023; Casabán et al., Citation2015; El-Tawil et al., Citation2007; Hussein and Selim, Citation2019; Hussein and Selim, Citation2015; Hussein and Selim, Citation2021; Hussein and Selim, Citation2012; Hussein and Selim, Citation2009). In (Bevia et al., Citation2023), the stochastic model of the generalized logistic differential equation was investigated through conducting the sample-path and mean-square solution and computing its PDF by using the random variable transformation (RVT) technique and Liouville’s equation. In (Hussein and Selim, Citation2019), the PDF of the solution SP for the stochastic Milne problem was obtained by combining the Karhunen–Love expansion with the RVT technique. In (Hussein and Selim, Citation2015), a deterministic analytical technique (modified extended tanh-function) and the RVT technique were consolidated to calculate the PDF of the exact wave solutions for the shallow water wave equation with a single random nonlinear coefficient. In (El-Tawil et al., Citation2007), the authors combined a deterministic numerical technique (finite element method) with the RVT technique to evaluate a closed form PDF of the solution SP for a randomly excited ordinary differential equation with a random operator. In addition, through (Casabán et al., Citation2015; Hussein and Selim, Citation2009; Hussein and Selim, Citation2012; Hussein and Selim, Citation2021) comprehensive probabilistic behaviors have been presented, via computing the solution PDF using the RVT technique, for various stochastic nonlinear models like dust plasma model in (Hussein and Selim, Citation2021), random SI-type epidemiological model in (Casabán et al., Citation2015), stochastic radiative transfer equation with Rayleigh scattering in (Hussein and Selim, Citation2012) and the stochastic transport equation of neutral particles with anisotropic scattering in (Hussein and Selim, Citation2009).
This paper presents a study of an important application of SDEs in nonlinear dynamics which is the generalized shallow water wave (GSWW) equation with random coefficients. This equation is given by:
(1)
(1)
where
is the vertical displacement of the surface and
and
are the dual random dispersion coefficients of the nonlinear terms. This equation is a stochastic nonlinear partial differential equation that is important in the fluid mechanics’ field. The shallow water wave (SWW) equations model the propagation of disturbances in water and other incompressible fluids. The underlying assumption is that the depth of the fluid is much smaller compared to the wavelength of the disturbance. The SWW equations were applied to atmospheric flows, tidal waves, tsunami prediction and waves in bathtubs (Hussein and Selim, Citation2015). The study of these equations is crucial for gaining insights into the behavior of waves and disturbances in fluid systems, and it has practical applications in various fields such as oceanography, meteorology, and civil engineering. Therefore, the established solutions of EquationEq. (1)
(1)
(1) can be used to represent a wide variety of significant physical phenomena.
The deterministic versions of SWW equations have been discussed extensively by many authors (El-Wakil et al., Citation2005; Krishnan et al., Citation2011; Li and Liu, Citation2018; Liu and He, Citation2017). On the other hand, the work, in literature, for the stochastic versions is still inadequate. Recently, a stochastic modified shallow water wave equation in the sense of the M-truncated derivative (Hamza et al., Citation2023) and with beta-derivative (Mohammed et al., Citation2023) was investigated. The stochasticity was considered in the added force term. Opposite to what is usually assumed in the literature, the authors in (Hussein and Selim, Citation2015) have studied the stochastic version of the problem where the nonlinear coefficients were considered random quantities. But for simplicity, they have assumed that the dual nonlinear coefficients are equal. This simplifying assumption makes the problem to be with a single random variable (RV). As a continuation of that work, the contribution in this article is to solve the more realistic version of the problem with two non-equal dependent random coefficients. The methodology used to solve the problem is based on combining a deterministic technique, to find closed form analytical wave solutions for the considered nonlinear partial differential equation (NLPDE), with the RVT technique (Casabán et al., Citation2016; Casabán et al., Citation2014; Walpole et al., Citation2011) as a stochastic technique. In this context, diverse analytical deterministic approaches for solving NLPDEs are available in the literature. For instance, the METF method (El-Wakil et al., Citation2005; El-Wakil and Abdo, Citation2007; El-Wakil et al., Citation2002), Lie symmetry analysis and the extended Jacobian elliptic function expansion method (Kumar et al., 2022), generalized exponential rational function method (Kumar et al., Citation2023), Hirota’s bilinear method (Akinyemi, Citation2023; Kumar and Mohan, Citation2021), the generalized Rickety equation mapping method (Kumar and Mann, Citation2023), the extended sine-Gordon expansion approach (Fahim et al., Citation2022), the extended direct algebraic method (Tasnim et al., Citation2023), modified Khater’s (mK) method (Tripathy et al., Citation2023), the improved F-expansion approach (Mirzazadeh et al., Citation2022) and the sub-equation method (Akinyemi et al., Citation2021).
Specifically in the present work, the METF method and the bivariate version of the RVT technique are incorporated to get some general probabilistic solutions to the GSWW Equationequation (1)(1)
(1) .
This article is organized as follows: in section 2, the bivariate RVT theorem and a general proposition of it, that will be applied in the subsequent sections, are presented. In section 3, the exact probabilistic solution of the GSWW Equationequation (1)(1)
(1) is constructed. The solution PDF and its statistical moments are acquired in general form utilizing the METF method incorporated with the RVT technique. The obtained general probabilistic solutions are investigated through two different types of variabilities for the random dispersion coefficients in Subsections (3.1) and (3.2). In section 4, graphs of the PDF and mean of the obtained rational, solitary, and periodic wave solutions are plotted for the two applications at certain wave speed, time and specific distribution’s parameters. The conclusions of this study are introduced in section 5. Finally, Appendix A is added for presenting the deterministic solutions of the GSWW equation using the METF method in detail.
2. The bivariate RVT technique
Theorem 1
(Hussein and Selim, Citation2019): Let be a two-dimensional random vector with joint probability density function
Let
be a two dimensional continuous mapping of
with inverse is
Assuming the Jacobian
and all derivatives are continuous. Then, the joint PDF of the random vector
is given by:
(2)
(2)
Remark 1:
To apply the RVT theorem, the number of input variables, must equal the number of output variables,
If they are not equal, we must introduce fictitious random outputs to apply the theorem (Hussein and Selim, Citation2019).
Remark 2:
According to the previous theorem, we can find the marginal density distribution of any transformed random variable, say through the following integral:
(3)
(3)
where
is the domain over which the RV,
is defined.
Proposition 1:
(RVT technique: general reciprocal for sum of two RVs)
Let be a two-dimensional random vector with joint probability density function
Then the PDF of the single output
is given by:
(4a)
(4a)
or
(4b)
(4b)
Proof:
Using Theorem 1 with introducing a fictitious random output (according to Remark 1), the random vector
will take the form:
(5)
(5)
with inverse transformation
takes the form:
(6)
(6)
The Jacobian of transformation, EquationEq. (6)(6)
(6) , is simplified to
(7)
(7)
Consequently, from EquationEq. (2)(2)
(2) , the joint PDF of the random vector
is given by:
(8)
(8)
In view of Remark 2 and EquationEq. (3)(3)
(3) , the PDF of the RV,
will take the form:
(9)
(9)
Or equivalently,
(10)
(10)
Note: if we introduce the fictitious variable as =
and follow the same procedures, we will get formula in EquationEq. (4b)
(4b)
(4b) .
3. The probabilistic solution of the GSWW equation
In this section, the aim is to find the complete probabilistic solutions of the stochastic GSWW equation described by EquationEq. (1)(1)
(1) where the two dispersion coefficients
are assumed continuous RVs. This can be achieved by computing the PDF of the dependent variable
As clarified in Appendix A, the modified extended tanh-function (METF) method is applied to solve EquationEq. (1)
(1)
(1) in deterministic scenario. All acquired travelling wave solutions are in the form of trigonometric (periodic), hyperbolic (solitary), and rational function solutions. The evaluated solutions will be considered, in the following analysis, as the random transformations for the RVT technique to get the PDFs of the corresponding random solutions.
Let’s consider the formulas of the solutions, i = 1,2,…,5, given in Appendix A by EquationEqs. (A-20) to Equation(A-24), as the input-output relations for the RVT technique, where the RVs,
and
are the inputs and the solution SP,
is the single system output. To compute the PDF of the solution
proposition 1 will be used with
and
Therefore, using Eq. (4), the PDF of the stochastic solution is:
(11)
(11)
Using the PDFs, any statistical moment of the solution SPs can be calculated via the expression
(12)
(12)
It is evident that the PDF of the solution SP is constructed whenever the solution SP, can be computed as a closed form in the input random variables. In addition, this closed form relation must be reversible such that every input random quantity can be computed explicitly as a function of the solution process,
and all-other random quantities. This is necessary to compute the Jacobian of the inverse transformations and apply the RVT theory. Hence, the application of this technique depends on the type of input random variables and its relationship with the solution process in the closed form solution.
3.1. Applications
The above mathematical treatment of the problem is valid regardless the probability distribution of the random coefficients. To illustrate our theoretical results, the variabilities of the random dispersive coefficients, and
are assumed to be general uniform or exponential variabilities. Considering the coefficients are dependent random variables, which is the more general situation, I will suggest that the joint PDF of the input RVs. α. and
is constructed using a copula transformation (Nelsen, Citation1999). In the following, these details will be declared.
Application 1: General exponential variabilities for 

Let us assume that and
are general dependent exponential RVs with parameters,
and
respectively. These RVs are transformed by the Farlie-Gordon-Morgenstern (FGM) copula (Nelsen, Citation1999) to define a two-dimensional random vector
with the following joint PDF:
(13)
(13)
Substituting from EquationEq. (13)(13)
(13) into EquationEq. (11)
(11)
(11) , a general closed form for the PDFs of all solution SPs is obtained in the following form:
(14)
(14)
where
(15)
(15)
and
are defined in Appendix A.
It is clear that the domain of is sensitive to the form of the deterministic functions,
and its related variables and parameters. Hence, the type of deterministic solution of the problem will affect this domain. Hereinafter, the PDF corresponding to every type of solution will be presented more specifically.
Case 1:
The PDF of the rational solution
The rational solution is given in EquationEq. (A-20) and
In this case, the PDF associated to
will take the following specific general form:
(16)
(16)
where
is defined according to EquationEq. (15)
(15)
(15) for i = 1 and
(17)
(17)
Case 2:
The PDF of the Solitary solutions
The PDF of the solitary stochastic solution, as given in EquationEq. (A-21), is given by:
(18)
(18)
where
is defined according to EquationEq. (15)
(15)
(15) , with
and i = 2.
Similarly, for the solution, as given in EquationEq. (A-22), the PDF is given by:
(19)
(19)
where
is defined according to EquationEq. (15)
(15)
(15) , with
and i = 3.
Case 3:
The PDF of the periodic solutions
The PDF of the periodic stochastic solution, as given in EquationEq. (A-23), is given by:
(20)
(20)
where
is defined according to EquationEq. (15)
(15)
(15) , with
and i = 4,
Similarly, for the last solution, as given in EquationEq. (A-24), one can get the following PDF:
(21)
(21)
where
is defined according to EquationEq. (15)
(15)
(15) , with
and i = 5.
Application 2: General positive Uniform variabilities for 

Consider and
are general dependent uniform RVs defined on the intervals (0, a) and (0, b) respectively and
As in application 1, a transformation using the Farlie-Gordon-Morgenstern copula (Nelsen, Citation1999) is applied to define a two-dimensional RV
with the following joint PDF:
(22)
(22)
Similarly, as in application 1, the general closed form for the PDFs of all solution SPs is obtained in the following form:
(23)
(23)
where
(26)
(26)
(27)
(27)
(28)
(28)
and
are defined in Appendix A.
4. Numerical results
In realizing the above work, let us assign numerical values for the distributions’ parameters used in the two previous applications. Specifically, and
in application 1 and
and
in application 2. In each application the arbitrary constant
Now, all solutions can be represented graphically along the spatial dimension,
at specific wave speed,
and time,
The numerical results are presented for both applications. Specifically, some results corresponding to exponentially distributed dispersion coefficients,
and
are illustrated through and for uniformly distributed ones, are illustrated through .
Figure 1. Variations of: (a) PDF of the rational solution along the spatial dimension,
at wave speed,
and
(b) PDF of the rational solution
with wave speed,
at
and
(c) Mean rational solution,
with wave speed,
at
and
(Exponential distributions).
![Figure 1. Variations of: (a) PDF of the rational solution ϕ1(x,t) along the spatial dimension, x, at wave speed, λ=1.4 and t=3.0; (b) PDF of the rational solution ϕ1(x,t) with wave speed, λ, at x=10 and t=3; (c) Mean rational solution, E[ϕ1], with wave speed, λ, at x=10 and t=3.0, (Exponential distributions).](/cms/asset/06f76e0a-a437-4e4e-a1e8-538449418ca4/oaen_a_2345516_f0001_c.jpg)
Figure 2. Variations of: (a) PDF of the solitary solution along the spatial dimension,
at wave speed,
and
(b) PDF of the solitary solution
with wave speed, λ, at
and
(c) Mean solitary solution,
with wave speed,
at
and
(Exponential distributions).
![Figure 2. Variations of: (a) PDF of the solitary solution ϕ3(x,t) along the spatial dimension, x, at wave speed, λ=1.5 and t=3.0; (b) PDF of the solitary solution ϕ3(x,t) with wave speed, λ, at x=10 and t=3.0; (c) Mean solitary solution, E[ϕ3], with wave speed, λ, at x=10 and t=3.0, (Exponential distributions).](/cms/asset/c5fba566-69a0-4b74-86d7-0002f0da3e64/oaen_a_2345516_f0002_c.jpg)
Figure 3. Variations of: (a) PDF of the periodic solution along the spatial dimension,
at wave speed,
and
taking
(b) PDF of the periodic solution,
with wave speed, λ, at
and
(c) Mean periodic solution,
with wave speed,
at
and
(Exponential distributions).
![Figure 3. Variations of: (a) PDF of the periodic solution ϕ4(x,t) along the spatial dimension, x, at wave speed, λ=0.4 and t=3.0, taking n=0,1,2; (b) PDF of the periodic solution, ϕ4(x,t) with wave speed, λ, at x=0.8 and t=3.0; (c) Mean periodic solution, E[ϕ4], with wave speed, λ, at x=0.8, t=3.0 and n=0, (Exponential distributions).](/cms/asset/dea17be3-260b-4851-b446-bd77943ce9e8/oaen_a_2345516_f0003_c.jpg)
Figure 4. Variations of: (a) PDF of the rational solution, along the spatial dimension,
at wave speed,
and
(b) PDF of the rational solution
with wave speed,
at
and
(c) Mean rational solution,
with wave speed,
at
and
(Uniform distributions).
![Figure 4. Variations of: (a) PDF of the rational solution, ϕ1(x,t) along the spatial dimension, x, at wave speed, λ=1.4 and t=3.0; (b) PDF of the rational solution ϕ1(x,t) with wave speed, λ, at x=10 and t=3; (c) Mean rational solution, E[ϕ1], with wave speed, λ, at x=10 and t=3.0, (Uniform distributions).](/cms/asset/492e608a-0d70-417b-ad26-4425818f17c3/oaen_a_2345516_f0004_c.jpg)
Figure 5. Variations of: (a) PDF of the solitary solution, along the spatial dimension,
at wave speed,
and
(b) PDF of the solitary solution
with wave speed, λ, at
and
(c) Mean solitary solution,
with wave speed,
at
and
(Uniform distributions).
![Figure 5. Variations of: (a) PDF of the solitary solution, ϕ3(x,t) along the spatial dimension, x, at wave speed, λ=1.5 and t=3.0; (b) PDF of the solitary solution ϕ3(x,t) with wave speed, λ, at x=10 and t=3.0; (c) Mean solitary solution, E[ϕ3], with wave speed, λ, at x=10 and t=3.0, (Uniform distributions).](/cms/asset/2794b2f2-2eb3-4b23-a750-8d36c536e53e/oaen_a_2345516_f0005_c.jpg)
Figure 6. Variations of: (a) PDF of the periodic solution, along the spatial dimension,
at wave speed,
and
taking
(b) PDF of the periodic solution
with wave speed, λ, at
and
(c) Mean periodic solution,
with wave speed,
at
and
(Uniform distributions).
![Figure 6. Variations of: (a) PDF of the periodic solution, ϕ4(x,t) along the spatial dimension, x, at wave speed, λ=0.4 and t=3.0, taking n=0,1,2; (b) PDF of the periodic solution ϕ4(x,t) with wave speed, λ, at x=0.8 and t=3.0; (c) Mean periodic solution, E[ϕ4], with wave speed, λ, at x=0.8, t=3.0 and n=0, (Uniform distributions).](/cms/asset/55259d5a-e8ed-41da-9ca2-063b439d89a5/oaen_a_2345516_f0006_c.jpg)
In application 1, , , and show the 3D plot of the PDF corresponding to the rational stochastic solution the solitary stochastic solution,
and the periodic stochastic solution,
They show the behavior of the PDFs with the spatial dimension, x, at a given wave speed,
and time, t. The effect of wave speed on the PDFs behavior is clarified through , and which are plotted for different
at fixed spatial dimension, x and time, t. It is noticeable that the mean values of the selected solutions’ SPs are increasing as wave speed,
increases. These behaviors can be noted more clearly through , and in which the behavior of the means of the solutions’ SPs,
and
that are calculated using EquationEq. (12)
(12)
(12) with
are elucidated. From these figures, one can note the considerable increase of the mean vertical displacement of the surface due to the increase of the wave speed which is physically acceptable.
For the sack of comparison, the corresponding solutions for application 2 are presented through . It is evident that the behaviors of the solutions in application 2 are the same as corresponding ones in applications 1. This assures the reliability of the obtained mathematical findings.
5. Conclusions
In this paper, the stochastic shallow water wave equation with dual random dispersion coefficients has been investigated via implementing a sound stochastic technique, named RVT, combined with the modified extended tanh-function (METF) Method. Firstly, this nonlinear partial differential equation has been solved deterministically using the METF method. Then, the RVT technique has been constructively applied to get the full probabilistic exact solution by deducing the PDF of the solution SP in a general closed form.
The source of generality is that the dispersion coefficients were assumed to be non-equal RVs. Additionally, the proposed mathematical treatment of the problem permitted these random coefficients to be dependent RVs with arbitrary probability distributions. Further, using the obtained general PDFs, any statistical moments of the solution SPs could be derived through EquationEq. (12)(12)
(12) .
To verify the proposed technique of solution, two applications were presented using different variabilities for the dispersion coefficients. The graphical results for the two applications are physically acceptable and are of the same behavior for corresponding solutions that makes the mathematical findings dependable.
The contribution of this work is that the proposed randomized model is more general and realistic than the previously introduced in (Hussein and Selim, Citation2015). Moreover, the implemented technique gives new full probabilistic solutions, represented by the solutions’ PDFs, of the considered problem in closed forms. In addition, the symbolic analysis and established analytical solutions affirm that the current technique is effective, robust, reliable and straightforward.
To my knowledge, this contribution was not presented previously for this problem. Therefore, this is a stride for future solving of other nonlinear differential equations with random coefficients and/or with random force using the proposed methodology. Alternatively, solving the same model using RVT technique combined with other deterministic techniques, like Jacobi elliptic function and generalized exponential rational function method, to derive other new stochastic wave solutions.
Data availability statement
Data sharing is not applicable to this article since no data sets were taken from outside sources.
Disclosure statement
The author declares that he has no known competing financial interests or personal relationships that could have appeared to influence the work reported in this paper.
Additional information
Notes on contributors
A. Hussein
A. Hussein has been awarded the Ph.D. degree in Engineering Mathematics, Faculty of Engineering, Cairo Univ. Egypt, 2005. His research interests are in the areas: Methods for solving stochastic Differential Equations (Spectral and Transformation approaches), Analytical methods for solving Nonlinear evolution equations, Radiative transfer, and transport phenomena in stochastic media. His current Job: Associate Prof. of Engineering Mathematics, Applied College, Umm Al-Qura University, Saudi Arabia. He has published research articles in reputable international journals of mathematical and engineering sciences.
References
- Akinyemi, L. (2023). Shallow Ocean soliton and localized waves in extended (2 + 1)-dimensional nonlinear evolution equations. Physics Letters A, 463(5), 128668. https://doi.org/10.1016/j.physleta.2023.128668
- Akinyemi, L., Şenol, M., & Iyiola, O. S. (2021). Exact solutions of the generalized multidimensional mathematical physics models via sub-equation method. Mathematics and Computers in Simulation, 182, 211–233. https://doi.org/10.1016/j.matcom.2020.10.017
- Bevia, V., Calatayud, J., Cortés, J. C., & Jornet, M. (2023). On the generalized logistic random differential equation: Theoretical analysis and numerical simulations with real-world data. Communications in Nonlinear Science and Numerical Simulation, 116, 106832. https://doi.org/10.1016/j.cnsns.2022.106832
- Casabán, M. C., Cortés, J. C., Navarro-Quiles, A., Romero, J. V., Roselló, M. D., & Villanueva, R. J. (2016). A comprehensive probabilistic solution of random SIS-type epidemiological models using the random variable transformation technique. Communications in Nonlinear Science and Numerical Simulation, 32, 199–210. https://doi.org/10.1016/j.cnsns.2015.08.009
- Casabán, M. C., Cortés, J. C., Romero, J. V., & Roselló, M. D. (2015). Probabilistic solution of random SI-type epidemiological models using the Random Variable Transformation technique. Communications in Nonlinear Science and Numerical Simulation, 24(1-3), 86–97. https://doi.org/10.1016/j.cnsns.2014.12.016
- Casabán, M. C., Cortés, J. C., Romero, J. V., & Roselló, M. D. (2014). Determining the first probability density function of linear random initial value problems by the random variable transformation (RVT) technique: A comprehensive study. Abstract and Applied Analysis, 2014, 1–25. https://doi.org/10.1155/2014/248512
- Cortés, J. C., Jódar, L., Roselló, M. D., & Villafuerte, L. (2012). Solving initial and two-boundary value linear random differential equations: a mean square approach. Applied Mathematics and Computation, 219(4), 2204–2211. https://doi.org/10.1016/j.amc.2012.08.066
- El-Tawil, M. A., El-Tahan, W., & Hussein, A. (2007). Using FEM-RVT technique for solving a randomly excited ordinary differential equation with a random operator. Applied Mathematics and Computation, 187(2), 856–867. https://doi.org/10.1016/j.amc.2006.08.164
- El-Wakil, S. A., & Abdo, M. A. (2007). Modified extended tanh-function method for solving nonlinear partial differential equations. Chaos Solitons & Fractals, 31(5), 1256–1264. https://doi.org/10.1016/j.chaos.2005.10.072
- El-Wakil, S. A., El-Labany, S. K., Zahran, M. A., & Sabry, R. (2005). Modified extended tanh-function method and its applications to nonlinear equations. Journal of Applied Mathematics and Computing. 161(2), 403–412. https://doi.org/10.1016/j.amc.2003.12.035
- El-Wakil, S. A., El-Labany, S. K., Zahran, M. A., & Sabry, R. (2002). Modified extended tanh-function method for solving nonlinear partial differential equations. Physics Letters A, 299(2-3), 179–188. https://doi.org/10.1016/S0375-9601(02)00669-2
- Fahim, M. R. A., Kundu, P. R., Islam, M. E., Akbar, M. A., & Osman, M. S. (2022). Wave profile analysis of a couple of (3 + 1)-dimensional nonlinear evolution equations by sine-Gordon expansion approach. Journal of Ocean Engineering and Science, 7(3), 272–279. https://doi.org/10.1016/j.joes.2021.08.009
- Ghanem, R. G., & Spanos, P. D. (2003). Stochastic finite elements: A spectral approach (Revised ed.). Courier Dover Publ.
- Golmankhaneh, A. K., Porghoveh, N. A., & Baleanu, D. (2013). Mean square solutions of second-order random differential equations by using homotopy analysis method. Romanian Reports in Physics, 65(2), 350–361.
- Hamza, A. E., Alshammari, M., Atta, D., & Mohammed, W. W. (2023). Fractional-stochastic shallow water equations and its analytical solutions. Results in Physics, 53, 106953. https://doi.org/10.1016/j.rinp.2023.106953
- He, B. (2009). Building structures vibration differential equations under random excitation. In Yuan, Y., Cui, J., Mang, H.A. (Eds.), Computational structural engineering (pp. 787–793). Springer. https://doi.org/10.1007/978-90-481-2822-8_86
- Hussein, A., & Selim, M. M. (2009). Solution of the stochastic transport equation of neutral particles with anisotropic scattering using RVT technique. Applied Mathematics and Computation, 213(1), 250–261. https://doi.org/10.1016/j.amc.2009.03.016
- Hussein, A., & Selim, M. M. (2012). Solution of the stochastic radiative transfer equation with Rayleigh scattering using RVT technique. Applied Mathematics and Computation, 218(13), 7193–7203. https://doi.org/10.1016/j.amc.2011.12.088
- Hussein, A., & Selim, M. M. (2013). A general analytical solution for the stochastic Milne problem using Karhunen–Loeve (K–L) expansion. Journal of Quantitative Spectroscopy and Radiative Transfer, 125, 84–92. https://doi.org/10.1016/j.jqsrt.2013.03.018
- Hussein, A., & Selim, M. M. (2015). Solution of stochastic generalized shallow water wave equation using RVT technique. The European Physical Journal Plus, 130(12), 249. https://doi.org/10.1140/epjp/i2015-15249-3
- Hussein, A., & Selim, M. M. (2019). A complete probabilistic solution for a stochastic Milne problem of radiative transfer using KLE-RVT technique. Journal of Quantitative Spectroscopy and Radiative Transfer, 232, 54–65. https://doi.org/10.1016/j.jqsrt.2019.04.034
- Hussein, A., & Selim, M. M. (2021). Propagation of dust acoustic waves in multi-components plasma with random parameters. The European Physical Journal Plus, 136(8), 797. https://doi.org/10.1140/epjp/s13360-021-01746-9
- Hussein, A., El-Tawil, M., El-Tahan, W., & Mahmoud, A. A. (2008). Solution of randomly excited stochastic differential equations with stochastic operator using spectral stochastic finite element method (SSFEM). Structural Engineering and Mechanics, 28(2), 129–152. https://doi.org/10.12989/sem.2008.28.2.129
- Krishnan, E. V., Triki, H., Labidi, M., & Biswas, A. (2011). A study of shallow water waves with Gardner’s equation. Nonlinear Dynamics, 66(4), 497–507. https://doi.org/10.1007/s11071-010-9928-7
- Kumar, S., & Mann, N. (2023). A variety of newly formed soliton solutions and patterns of dynamic waveforms for the generalized complex coupled Schrödinger–Boussinesq equations. Optical and Quantum Electronics, 55(8), 723. https://doi.org/10.1007/s11082-023-04869-8
- Kumar, S., & Mohan, B. (2021). A study of multi-soliton solutions, breather, lumps, and their interactions for kadomtsev-petviashvili equation with variable time coefficient using Hirota method. Physica Scripta, 96(12), 125255. https://doi.org/10.1088/1402-4896/ac3879
- Kumar, S., Mann, N., Kharbanda, H., & Inc, M. (2023). Dynamical behavior of analytical soliton solutions, bifurcation analysis, and quasi-periodic solution to the (2 + 1)-dimensional Konopelchenko–Dubrovsky (KD) system. Analysis and Mathematical Physics, 13(3), 40. https://doi.org/10.1007/s13324-023-00802-0
- Kumar, S., Rani, S., & Mann, N. (2022). Diverse analytical wave solutions and dynamical behaviors of the new (2 + 1)-dimensional Sakovich equation emerging in fluid dynamics. The European Physical Journal Plus, 137(11), 1226. https://doi.org/10.1140/epjp/s13360-022-03397-w
- Li, Y. Z., & Liu, J. G. (2018). Multiple periodic-soliton solutions of the (3 + 1)-dimensional generalised shallow water equation. Pramana, 90(6), 71. https://doi.org/10.1007/s12043-018-1568-3
- Liu, J. G., & He, Y. (2017). New periodic solitary wave solutions for the (3 + 1)-dimensional generalized shallow water equation. Nonlinear Dynamics, 90(1), 363–369. https://doi.org/10.1007/s11071-017-3667-y
- Mirzazadeh, M., Akbulut, A., Taşcan, F., & Akinyemi, L. (2022). A novel integration approach to study the perturbed Biswas-Milovic equation with Kudryashov’s law of refractive index. Optik, 252, 168529. https://doi.org/10.1016/j.ijleo.2021.168529
- Mohammed, W. W., Al-Askar, F. M., Cesarano, C., & Aly, E. S. (2023). The soliton solutions of the stochastic shallow water wave equations in the sense of beta-derivative. Mathematics, 11(6), 1338. https://doi.org/10.3390/math11061338
- Nelsen, R. B. (1999). An introduction to copulas. Springer.
- Øksendal, B. (2010). Stochastic differential equations: An introduction with applications (6th ed.). Springer.
- Tasnim, F., Akbar, M. A., & Osman, M. S. (2023). The extended direct algebraic method for extracting analytical solitons solutions to the cubic nonlinear Schrödinger equation involving beta derivatives in space and time. Fractal and Fractional, 7(6), 426. https://doi.org/10.3390/fractalfract7060426
- Tripathy, A., Sahoo, S., Rezazadeh, H., Izgi, Z. P., & Osman, M. S. (2023). Dynamics of damped and undamped wave natures in ferromagnetic materials. Optik, 281, 170817. https://doi.org/10.1016/j.ijleo.2023.170817
- Walpole, R. E., Myers, R. H., Myers, S. L., & Ye, K. (2011). Probability and statistics for engineers and scientists (8th ed., pp. 212–217). Printice Hall.
Appendix A
A.1 The modified extended-tanh function (METF) method
The METF method, as introduced by El-Wakil et al. (Citation2005) and El-Wakil and Abdo (Citation2007), can be summarized in the following:
Consider the following (1 + 1) PDE:
(A-1)
(A-1)
Assuming a traveling wave solution where
EquationEq. (A-1)
(1)
(1) is converted into the following ordinary differential equation (ODE):
(A-2)
(A-2)
with
Integrating of EquationEq. (A-2) will reduce its order, considering the integration constant to be zero. After that, the solution may be represented by the formula:
(A-3)
(A-3)
Balancing the nonlinear term with the highest-order linear term in EquationEq. (A-2), the integer M can be determined. Additionally, is the solution of the next Reccati differential equation
(A-4)
(A-4)
where
is a constant to be evaluated.
Depending on the value of EquationEq. (A-4) has the following solutions:
(A-5)
(A-5)
(A-6)
(A-6)
and
(A-7)
(A-7)
Inserting Eqs. Equation(A-3) and Equation(A-4) into the ODE (A-2) leads to a system of algebraic equations in the unknowns and
By solving this system, the solutions of EquationEq. (A-1)
(1)
(1) can be obtained.
A.2 The deterministic solution of the GSWW equation
The METF method is applied to obtain a traveling wave solution of EquationEq. (1)(1)
(1) as follows:
Under the mapping, is the wave speed, EquationEq. (1)
(1)
(1) is transformed to the next ODE:
(A-8)
(A-8)
Balancing with
leads to
Then, according to EquationEq. (A-3),
(A-9)
(A-9)
Merging EquationEqs. (A-4) and Equation(A-9) into EquationEq. (A-8) gives an algebraic equation in powers of Equating the coefficients of
and
to zero leads to the next system of equations in the parameters
and
(A-10)
(A-10)
(A-11)
(A-11)
(A-12)
(A-12)
(A-13)
(A-13)
(A-14)
(A-14)
(A-15)
(A-15)
Using Mathematica package, the system of algebraic equations is solved, and the next sets of solutions are obtained.
(A-16)
(A-16)
(A-17)
(A-17)
(A-18)
(A-18)
(A-19)
(A-19)
Depending on Eqs. (A-16), the deterministic solution of EquationEq. (1)(1)
(1) is the next rational form:
(A-20)
(A-20)
where
and
is an arbitrary constant.
In view of EquationEqs. (A-17), and for (or
), the solution of EquationEq. (1)
(1)
(1) will take the subsequent solitary wave forms:
(A-21)
(A-21)
(A-22)
(A-22)
where
For (or
), one can get the following periodic solutions:
(A-23)
(A-23)
(A-24)
(A-24)
where
Using EquationEq. (A-18), identical solution to that is given by EquationEq. (A-21) will be obtained. Utilizing EquationEq. (A-19), the solution corresponding to (or
) is:
Or equivalently,
(A-25)
(A-25)
which is identical to
Again, depending on EquationEq. (A-19), the solution of EquationEq. (1)(1)
(1) corresponding to
(or
) takes the form:
Or equivalently,
(A-26)
(A-26)
which is identical to
Therefore, only EquationEqs. (A-20)–(A-24) represent the independent deterministic solutions of EquationEq. (1)(1)
(1) . All solutions are verified by substituting them back into EquationEq. (1)
(1)
(1) .