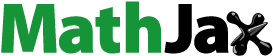
Abstract
Earned value analysis is an important tool to measure the performance of a project to indicate what will happen to the work in the future. This study focuses on developing a fuzzy-based earned value analysis model for estimating the performance of projects. The model provides to make estimations getting closer and reduces the errors in calculations through the fuzzy linguistic terms. The data for this study were taken from projects which were done between 2020 and 2023 for the six sets of building projects. The data has been analyzed through a fuzzy-based earned value analysis model by using Microsoft Excel as a basic tool. It was found that the fuzzy-based SPI value for all projects was in Scenario 1, which implies that T(()≥1) = 0 and T((
) ≤ 1) = 1, So the work package is behind the schedule. Similarly, the value of fuzzy-based CPI for all projects except one were in Scenario 1, implies that T((
) ≥1) = 0 and T((
) ≤ 1) = 1, so the work package is behind the budget. Finally, it can be recommended that it needs to properly monitor, and control the project time, and cost to recover deviation in the remaining periods.
Reviewing Editor:
1. Introduction
The construction industry contributes to the growth of our economy to a large extent. One of the main problems that the industry faces is project delay. This can be caused due to various reasons like deviation from the initial plan, scarcity of resources, poor planning, poor execution and natural calamities (Suresh & Ramasamy, Citation2017). This causes the project to go over the expected budget, fail to be completed within the scheduled time and become costlier. Hence it is important that there is a systematic and scientific approach to project management to ensure that the project is completed within the constraints of time and resources.
EV analysis is an important tool to measure the performance of a project. It is a program management technique that uses ‘work in progress’ to indicate what will happen to the work in the future.
It compares the actual work performed against a baseline plan. In this way, the analysis helps in setting a standard for performance evaluation and controls the time and cost constraints.
The earned value management system assists in a simultaneous time and cost control system to be conducted in accordance with the project scope. As is common in the project management context, the earned value method is applied to accomplish the project on time and on budget with an acceptable level of quality (Noori et al., Citation2008).
The PMBOK Guide initially defines EVM as ‘a management methodology for integrating scope, schedule and resources for objectively measuring project performance and progress’. Performance is measured by determining the budgeted cost of the work performed (i.e. earned value) and comparing it to the actual cost of the work performed (i.e. actual cost). Progress is measured by comparing the earned value to the planned value. Earned Value Management has any modifications as time proceeds (Acebes et al., Citation2022).
EVA calculates the cost and time performance indices of each project, estimates the completion cost and the completion time of each project and finally measures the performance and the progress of each project by comparing the planned value and actual costs of activities to their corresponding earned values (Moradi et al., Citation2020). As is common in the project management context, the earned value method is applied in order to accomplish the project on time and on budget with an acceptable level of quality (Noori et al., Citation2008).
Every project undergoes the triple constraints of time, money and quality; the balancing of which results in a satisfactory project output. While a project is being executed monitoring and controlling is very essential. Performance reporting is a part of the project monitoring and control process. The earned value method is a widely accepted performance measurement technique. This methodology is useful for reporting the status of the project as well as for predicting future performance based on past performance (Araszkiewicz & Bochenek, Citation2019).
Nowadays, it is accepted that using EV techniques has many advantages and would enhance the schedule and cost performances of a project, even though the studies on EVs are very limited (Asgari Dehabadi et al., Citation2014). studied the estimation of project performance using earned value management and fuzzy regression (Narbaev, Citation2011). concluded that the best and the most reliable method to estimate cost at completion was the ES-based method. He also introduced the Earned Schedule (ES) concept to resolve the limitations inherent in the use of the historical EV Schedule Variance (SV) and Schedule Performance Index (SPI). Obianyo et al. (Citation2023) stated artificial neural network models were used to model the process of estimating earned value indexes (Ponz-Tienda et al., Citation2012). investigated earned value management versus earned schedule-based regression models (Suresh & Ramasamy, Citation2017). studied the analysis of project performance using earned value analysis (Iranmanesh & Zarezadeh, Citation2008). studied the application of artificial neural networks to forecast the actual cost of a project to improve the earned value management system. G. G. Ayalew et al. (Citation2023) conducted a study on Integrating exploratory factor analysis and fuzzy AHP models for assessing the factors affecting the performance of building construction projects. G. M. Ayalew et al. (Citation2023) studied regression modeling for the prediction of earned value indexes in public building construction projects (Moradi et al., Citation2020). proposes a new version of earned value analysis (EVA) to handle uncertainty in mega projects under interval-valued fuzzy (IVF)-environment (Moradi et al., Citation2020). presented a fuzzy earned value model and provided an estimation of medical research projects and drug development projects of the total cost under uncertain conditions (Birhanu Belete et al., Citation2024). evaluated the accuracy of the time and cost forecasting of the projects.
To handle vagueness and uncertainty in engineering and management systems, fuzzy sets theory is presented separately by Zadeh (Kabir & Hasin, Citation2011). Thus the vague data may be represented using fuzzy numbers, which can be further subjected to mathematical operation in the fuzzy domain. A fuzzy set can be defined mathematically by a membership function, which assigns each element x in the universe of discourse X a real number in the interval [0, 1] (Russo & Camanho, Citation2015).
In this paper, we introduce the uncertainty concepts with the fuzzy point of view. Considering most of the activities in real-world applications for building projects have a high degree of uncertainty in the EVA calculations.
In reality, the data regarding the activities progress involved in the building project come from people’s judgments; hence they carry some degree of uncertainty.
Considering this uncertainty in interpretations and calculations helps not only in measuring better the performance and the progress of a project but also in extending the applicability of the EV techniques under real-life and uncertain conditions. This study provides a unique and extended contribution to previous research on the development of a new fuzzy-based EV technique to measure and evaluate the performance and progress of building construction projects and their activities under uncertainty.
The developed model provides as to make EVA calculations and final estimations of each building project getting closer and reduces the errors in calculations from the linguistic terms and the fuzzy theory.
Moreover, this study incorporating the uncertainty concepts is a new application in the area of earned value management in the building construction projects of Ethiopia.
Furthermore, considering the proposed model in earned value analysis provides to overcome the problems related to errors in calculations by using the fuzzy principles on the linguistic terms, and the fuzzy linguistic terms could be applied to transform the EVA’s mathematics to adjust and reflect the new values.
However, the researchers did not find any study that is the same as this study while searching in international libraries and discreet periodicals such as Scopus, Springer, Taylor France and others, as this study is almost unique of its kind that deals with fuzzy based earned value analysis model.
Therefore, due to the uniqueness of the proposed model incorporating fuzzy set theory in earned value analysis was recognized as the most significant problem to be investigated in the building projects. Thus, the main objective of this study is to focus on developing an earned value analysis model. The study provides an important secondary source of data for future researchers and academicians to get knowledge and skills on the proposed model and also provides a reference guide for the building construction projects for evaluation of the project performance.
The remainder of this paper is organized as follows. Section 2 introduces earned value analysis and measurement techniques.
Section 3 presents the methodology it includes, the method of data collection and data processing, identification of study variables, fuzzy theory and its application to the EV is comprehensively described. It is dedicated to explaining how the fuzzy approaches can be applied as a powerful tool to predict the project performance in the future. The question related to the interpretation of fuzzy-obtained values is also responded to in section 3. Section 4 employed a case study to show how the proposed model can be utilized for real case projects. Section 5 presents a discussion of results, managerial implications and limitations. Eventually, general conclusions and remarks are then presented in Section 6.
2. Literature review
The construction industry is one of the broad and important sectors of any country’s economy, and it is one of the main engines that governments resort to moving the economy to create jobs and reduce unemployment.
The construction industry has unique characteristics, which make it an industry that is distinguished from the rest of the industries by its many risks, and its projects always suffer from the problems of delay in implementation and increases in cost in most countries of the world.
Building construction projects are one of the physical infrastructures included in civil works and they need appropriate planning organizing, monitoring and controlling the whole process of the construction (G. M. Ayalew et al., Citation2023).
2.1. Basic definition of earned value analysis and measurement techniques
In most cases, the management of building construction projects is not a simple matter, as success in such projects requires good coordination as well as a comprehensive integrated operating system for the project so that all project participants can understand their roles and agree on key performance indicators.
Earned value management system is a useful management technique available for building projects managers to monitor and control projects; a technique that combines the work scope, schedule and cost elements of a project and facilitates the integrated reporting of a project’s progress and the cost status. Earned value management system in effect integrates time management and cost management which are essential elements of buildings projects management.
According to Vyas and Birajdar (Citation2016), earned value analysis is a method of performance measurement in evaluating and forecasting construction projects. Earned value is a project control technique that provides cost and schedule performance measurements. It compares the actual accomplishment of scheduled work and associated costs against an integrated schedule and budget plan (Prasanth & Thirumalai Raja, Citation2007).
According to Salari et al. (Citation2014), earned value management is a powerful technique that allows program managers, project managers and other top-level stakeholders to visualize the status of the project during the project life cycle (Moslemi Naeni et al., Citation2014). stated that it is a crucial technique in analyzing and controlling the performance of a project which allows a more accurate measurement of both the performance and the progress of a project.
2.1.1. Terminologies
Earned value analysis requires the following three key parameters to measure project performance (Chandrasekaran & Kumar, Citation2012):
Planned Value: is that portion of the approved total cost estimate that is planned to be spent on an activity during a given period (Salari et al., Citation2014). PV = Physical Work + Approved Budget
Actual cost: the total of direct and indirect costs incurred in accomplishing work on an activity during a given period. It is also called the actual cost of work performed (ACWP).
Earned Value: This also called the budgeted cost of work performed (BCWP), is an approximation of the value of the physical work completed. It relates to the original planned costs for the project or activity and the rate at which the team is completing work on the project or activity to date.
Once the above three parameters are determined then they can be used to identify schedule and cost variances and to calculate overall project performance using the formulas given below (Suresh & Ramasamy, Citation2017).
Schedule Variance (SV) = EV – PV. Schedule Variance is a competence indicator, which reflects the schedule performance of the project. It measures the difference between what was initially planned to complete the work and what has been completed.
Cost Variance (CV) = EV – AC. Cost Variance is an efficiency indicator that reflects the cost performance of the project. It measures the difference between the budget and the actual amount spent to complete the task.
Schedule Performance Index (SPI) = EV/PV. The Schedule Performance Index is used to predict the project’s completion time and forecast the project’s estimate at completion (EAC). SPI is defined as the ratio of the EV to PV. If the value of SPI is below 1.0, it indicates that the project is behind schedule. If SPI is equal to 1.0 then the project would be completed on time. When SPI is above 1.0, it shows that the project is ahead of schedule.
Cost Performance Index (CPI) = EV/AC. The Cost Performance Index is used to track the project cost and predict cost overruns. It is commonly used to track cost–efficiency. CPI is simply the ratio of the EV to AV. The value of CPI below 1.0 indicates that the project’s cost is over the planned cost for the work performed. If CPI is equal to 1, then the project would be completed at the planned cost. When CPI is above 1.0, the project’s cost is the under-planned cost for the work performed.
Estimate at Completion (EAC) = Budget at Complete (BAC)/CPI. Estimate at completion is the projected total cost of the planned work at completion. EAC = Actual Cost (AC) + Estimate to Complete (ETC).
Estimate to Complete (ETC) = EAC – AC. Estimate to Complete is the expected cost needed to complete the remaining planned work.
Budget at Completion (BAC): is the baseline cost that shows the planned cost for a task, a resource for all assigned tasks, or for work to be performed by a resource on a task (Araszkiewicz & Bochenek, Citation2019).
2.1.2. Earned value measurement techniques
The EV could be composed of some techniques to help project managers evaluate and control the performance and progress of building construction projects by estimating their completion cost and completion time based on their actual cost and actual time up to any given point in the projects (Narbaev, Citation2011). The methods for measuring earned value depend on the type of work being performed (Asgari Dehabadi et al., Citation2014).
Some of these techniques used for measuring earned value are defined as follows (Simion & Marin, Citation2018).
Discrete Effort: Discrete effort project tasks have a definable scope and objectives that can be scheduled and have progress measured against. It includes the fixed ratio formula method, weighted milestone method, percent complete method, percent complete with milestone gates method, earned standards and equivalent unit method.
Apportioned Effort: is useful for tasks whose planning and performance have a direct relationship to another task. The earned value for the apportioned task is driven by the status of the linked task. Quality control and inspections are examples of apportioned effort.
Level of Effort: is work scope of a general or supportive nature for which performance cannot be measured or is impractical to measure. Because these tasks are more time-driven than task-driven, the earned value always equals the planned value, regardless of the work done. Therefore, level of effort scopes of work do not have a schedule variance and produce very misleading cost variances. For this reason, this method is generally not recommended to track earned value. To keep the paper short, we avoid more explanation on the sub-techniques of each earned value measuring technique.
The technique that is being used in this paper is the percent complete technique. The percent complete technique method is a subjective judgment of the EV measurement techniques, if there are no objective indicators based on which the estimates should be made. This greatly incorporates errors and uncertainty which cause biased judgments. An idea to overcome this problem is to use linguistic terms in estimating the completion percent of each activity, as imprecise and uncertain data on activity performance and activity progress commonly arise. This forms the basis of our novel idea.
There are many situations in real-life mega projects where the amount of work (or the quantity of work) for an activity is unknown or imprecise and is out of control.
For example, during the excavation of construction projects, the ground should be excavated until a hard layer of rock is reached. Before reaching this layer, the exact amount of the operations and the required work are unknown and also this is out of our control, so the percent complete of excavation activity cannot precisely be evaluated. The exact amount of work needed to derive scientific conclusions is unknown in advance. In these cases and many other similar cases, it would be better and easier to measure the percentage of the activity completed under uncertain values by linguistic terms with the new application of fuzzy sets theory rather than to measure it exactly and deterministically. Some researchers believe the developed techniques and models reflect better the uncertain nature of building projects.
3. Methodology
This section provides the methodology required for solving the fuzzy-based earned value model. This study can employ a case study research approach on a fuzzy-based earned value model for estimating the performance of building construction projects. The study was made on some projects randomly selected based on the standard data set, and it collects the schedule and cost information from the building construction projects for estimating the performance of the building construction projects. Furthermore, this study provides a novel approach to a fuzzy-based earned value model to measure the project performance while the previous study was only focused on simple crisp numerical analysis and no attention to intermediate linguistic terms had been carried out. Thus, the fuzzy-based earned value model is taken and modified for this evaluation. Thereafter, discussion and conclusions can be made regarding the status of the projects. The steps and procedures for conducting the current study are made based on the research objective, and the technique for conducting this study is presented in the .
3.1. Method of data collection and data processing
The method of data collection used in conducting this study was taken from the historical data of six sets of building construction projects that were done between 2020 and 2023 from cities, project owners, consultants and contractors. In conducting this study the collected data has been analyzed through a fuzzy-based earned value analysis model. Microsoft Excel was used as the basic tool for all sorts of calculations.
3.2. Identification of study variables
The two types of variables that are adopted in developing a fuzzy-based earned value analysis model are Dependent variables and Independent variables.
Independent variables: The variable that is manipulated or controlled by the researcher. It is the variable that is hypothesized to have an effect on the dependent variable; these variables are also called predictor or explanatory variables used to predict or explain the behavior of the dependent variable (Iranmanesh & Zarezadeh, Citation2008). In this study, the actual cost, earned value, planned value, budget at completion and duration of the project were identified as the independent variables.
Dependent variables: is the variable that is being measured or observed in the study. The dependent variable is the variable that is expected to change as a result of the independent variable manipulation. In this study, the dependent variables were fuzzy-based
3.3. Fuzzy theory to measure the earned value
In construction projects, there are many real-life situations where the amount of work (or the quantity of work) for an activity is unknown or imprecise and out of our control, so the percent complete of excavation activity cannot precisely be evaluated (Asgari Dehabadi et al., Citation2014). Thus, it can incorporate fuzzy linguistic terms in the progress of the project activity to overcome uncertainty, so the progress of the project activity can be defined by fuzzy linguistic terms may be stated as ‘Very Low, Low, Less than Half, Half, More than Half, High, Very High’ etc.
These linguistic terms cannot be applied to the earned value technique before converting it to a number. In carrying out the terms into the EV technique first the fuzzy principles on the linguistic terms to convert them into fuzzy numbers. Secondly, the EVA’s mathematics will be adjusted to reflect the new values (fuzzy numbers). Typically, the project experts implement this transformation in accordance with their knowledge and their experience about the project and with activity attributes.
The application of the proposed method arises in situations where the total amount of the work required to perform the activities is unknown or uncertain and is out of control. The exact amount of work required to derive scientific conclusions is unknown in advance. In these cases and many other similar cases to include this uncertainty in our results, it would be better and easier to evaluate the percentage of the activity completed by linguistic terms rather than to evaluate it exactly and deterministically. The developed techniques and models reflect better the uncertain nature of building projects.
3.3.1. Fuzzy set theory
The fuzzy sets theory introduced by Zadeh (Citation1965) describes uncertainty and vagueness in events and systems to enhance precision (Kabir & Hasin, Citation2011). It is logical to model and consider the uncertainty by implementing linguistic terms with the fuzzy sets theory. It is logical to model and consider the uncertainty by employing linguistic terms with the fuzzy sets theory (G. G. Ayalew et al., Citation2022). A trapezoidal fuzzy number is a special class of fuzzy numbers whose membership is defined by four real numbers, expressed as
In general, the membership functions of a trapezoidal fuzzy number can be defined as follows (Huang & Ho, Citation2016):
(1)
(1)
Note that a trapezoidal fuzzy number becomes triangular when b = c.
For ease of representation and calculation, a triangular fuzzy number is also represented as a trapezoidal fuzzy number [a,b,b,d] or [a,c,c,d]. The trapezoidal fuzzy number and the triangular fuzzy number are both the simplest fuzzy numbers and their corresponding calculations can be performed easily. This enables the authors to explain their novel fuzzy-EV approach.
3.3.2. Linguistic terms
A linguistic term helps to estimate the activity progress and it can show the percentage completion of an activity. It was strongly believed the newly developed technique reflects better the uncertain nature of the project (Sun, Citation2010).
Suppose that the completion of an activity consists of uncertainty. As stated earlier the project experts can transform the uncertainty into a fuzzy number by assigning a membership function to this linguistic term, to indicate the relationship between the linguistic term and the fuzzy number as shown in . In this figure, the horizontal axis refers to the progress and is on a scale of 1. The summary of the transformation related to is reported in . The output of this transformation is a fuzzy number.
Figure 1. Steps to conduct the study (Jie & Wei, Citation2022).

Figure 2. Fuzzy membership including trapezoidal and triangular fuzzy numbers and the corresponding linguistic terms.

Table 1. The assigned fuzzy numbers to each linguistic term of (Moradi et al., Citation2020).
According to and , the linguistic term ‘high’ equals to the fuzzy number [0.7, 0.8, 0.8, 0.9].
To deal with the basic operation of fuzzy numbers, let as suppose r ≥ 0 is a real number, and and
are two trapezoidal fuzzy numbers each with four members, i.e.
= (a1, a2, a3, a4) and
= (b1, b2, b3, b4).
The arithmetic operations of fuzzy numbers are defined as follows (Sun, Citation2010):
Fuzzy number addition ⊕
(2)
(2)
Fuzzy number subtraction Ɵ
(3)
(3)
Fuzzy number multiplication ⊗
(4)
(4)
Fuzzy number division ∅
(5)
(5)
In expressing the completion percent of each activity based on a fuzzy number, the fuzzy EV of activity i i can be calculated using the EquationEquation (6)
(6)
(6) .
(6)
(6)
Where is the fuzzy completion percent of the activity i, i.e.
(7)
(7)
is the Budget at Completion of activity i which denotes the planned budget to complete the activity i. The total fuzzy EV in each measurement period,
is calculated by summing up all the
for i = 1, …, n where n is the total number of the project activities:
(8)
(8)
In the EquationEquation (8)(8)
(8) some of the
can be fuzzy numbers while some of them can be deterministic numbers.
With the EquationEqs. (1) (6) (7)(7)
(7) and Equation(8)
(8)
(8) , other fuzzy EV indices can be developed similarly and it will discuss them thoroughly in Section 4.
To conclude this section we should add that the new fuzzy-based EV technique would result in a more practical technique in comparison to the traditional EV technique by incorporating greatly into a more realistic implementation and interpretation of the EV indices and estimates where measuring and evaluating the activity progress under uncertainty can be dealt very effectively.
3.4. Fuzzy-based earned value indices and estimates
The Percent completion of an activity can be expressed as a fuzzy number. The fuzzy percent complete and a fuzzy activity EV, all related indices and estimates have to be expressed as fuzzy numbers. This section is devoted to the development of these indices and estimates (Moradi et al., Citation2020).
3.4.1. Fuzzy performance indices
SPI and CPI are the two widely used indices in the EV technique to evaluate the time progress and cost performance of a project.
SPI is a measure of the conformance of the actual progress to schedule. The schedule performance index is calculated as the earned value divided by the planned value.i.e. = EV/PV, where PV known also as the budgeted cost of work scheduled (BCWS) is scheduled to be used during the project. The
is calculated as follows:
(9)
(9)
The CPI is a measure of the budgetary conformance of the actual cost of work performed. In other words, the CPI is
the most useful index indicating the cumulative cost efficiency of a project. The CPI is the ratio of the EV to the Actual Cost (AC), i.e. The
is calculated as follows:
(10)
(10)
The AC is also known as the Actual Cost of Work Performed (ACWP) and is an indication of the resources that have been used to achieve the actual work performed. The Critical Ratio (CR) is a multiplication of the SPI by the CPI and shows the project’s health. It is also called Schedule Cost Index (SCI) which is calculated as follows:
(11)
(11)
3.4.2. Fuzzy cost estimate at completion
EVA can also be used to forecast cost and time at project completion. For both cost and duration forecasting, several formulas have been introduced in the related literature (Moradi et al., Citation2020). Among several formulas available to calculate cost estimate at completion (EAC), a common formula presumes the future trend of the project cost performance remains untouched. In this formula, EAC is calculated by dividing the budget at completion (BAC) by CPI. In other words, this formula assumes that the CPI would be fixed during the rest of the project. As in the proposed method, the CPI is a fuzzy number, and the EAC becomes a fuzzy number too, thus:
(12)
(12)
In the other formula to calculate the EAC, the EAC will be influenced both by current cost performance and current schedule performance indices. The is calculated as follows:
(13)
(13)
3.4.3. Fuzzy time estimate at completion
Even though the EV technique has been designed to manage both time and cost, most of the research has been dedicated to the cost aspects (Moradi et al., Citation2020). Thus, the EV technique has not been widely used to estimate the total time at completion or total project duration based on the actual performance up to a given point in the project. However, there are three different techniques for estimating the completion time (Moradi et al., Citation2020): These are the Planned Value (PV) technique, the Earned Duration (ED) technique and the Earned Schedule (ES) technique.
According to Moradi et al. (Citation2020), among these three techniques, the third technique developed by Asgari Dehabadi et al. (Citation2014) and later extended by Chandrasekaran and Kumar (Citation2012) is the most reliable and the most practical. The first two techniques use the SPI. As the SPI tends to be 1 at the end of the project and does not reflect the real performance of the project, these two techniques are not reliable. Thus it can use the ES technique to develop the fuzzy-based time estimate at completion. Since the EAC reflects the estimate of the completion cost, we follow the notation with index t to reflect the time estimate at completion.
According to Chandrasekaran and Kumar (Citation2012), the ES is the time equivalent of the EV which is the result of projecting the EV on the baseline. Thus it measures schedule performance using time. Calculation of the ES is determined by comparing the EV against the PV presented in EquationEquation (14)(14)
(14) . explains the ES and the EV graphically.
(14)
(14)
Where N is the longest time interval in which the PVN is less than the EV, the PVN is the planned value at time N and the is the planned value at the next time interval, i.e. time N + 1 (Narbaev, Citation2011).
Generally, EquationEquation (14)(14)
(14) is an interpolation formula.
As in the fuzzy-based EV, the EV is a fuzzy number (EquationEquation (8)(8)
(8) ), then the ES will be a fuzzy number too.
Thus each of the four members of the trapezoidal fuzzy number EV is projected on the baseline and forms a member of the trapezoidal fuzzy number ES. The mathematical calculations for the ES are as follows (Moradi et al., Citation2020):
(15)
(15)
In contrast to the SPI, the is expressed in time units and is the ratio of the ES to the actual duration (AD), i.e.
The is calculated as follows: EquationEquation (16)
(16)
(16) represents this.
(16)
(16)
The generic ES-based equation to estimate the time at the completion of a project, or the project duration is:
(17)
(17)
Where the PD is the planned duration to complete the project and the PF is the performance factor which depends on the project status. Usually, the following three cases are considered for the PF (Moradi et al., Citation2020):
PF = 1: The duration of the remained activities is as planned (EquationEquation (18)
(18)
(18) ).
(18)
(18)
PF =
The duration of remaining activities changes with the current
trend (EquationEquation (19)
(19)
(19) ).
(19)
(19)
PF =
t: The duration of remaining activities changes with the
trend.
is the multiplication of the
by the
i.e.
=
3.4.4. Interpretation of fuzzy performance indices
After developing the fuzzy-based EV indices, we should interpret them to have an inference regarding the project’s progress and its status (Chandrasekaran & Kumar, Citation2012). Similar to the traditional EV indices where a comparison is made against the value 1which shows the EV against planned value and actual cost, the same story goes here, i.e. the new fuzzy indices must be compared against value 1. However as the new indices are fuzzy numbers, we should compare them using the methods proposed for comparing the fuzzy numbers. Out of different methods proposed to compare fuzzy numbers in the literature (Asgari Dehabadi et al., Citation2014), a well-known fuzzy ranking method proposed by Moradi et al. (Citation2020) evaluates the degree of possibility by which a fuzzy number is either greater or less than another fuzzy number.
According to Moradi et al. (Citation2020) T() is the degree of possibility of fuzzy number
thus T(
) = µ
(x) where x ∈
Given two fuzzy numbers
the degree of possibility that
≥
is:
(21)
(21)
The proposed method leads to deterministic conclusions almost in all cases; however, in several cases, the results are not deterministic which might be of interest as it is much closer to the nature of the project and the proposed new uncertain model in this paper.
To apply the EquationEquation (21)(21)
(21) to EquationEquation (9)
(9)
(9)
=
=
and to EquationEquation (10)
(10)
(10)
=
=
however the comparison is made against 1, thus
(22)
(22)
(23)
(23)
Assuming trapezoidal fuzzy numbers, thus both T (≥1), and T (
≥1) results in five scenarios as and show, respectively.
Table 2. The degree of possibility of ≥1 and
≤ 1.
Table 3. The degree of possibility of ≥1 and
≤ 1.
In these tables, the vertical line depicts the position of value 1, as we would like to compare ≥1, and
≥1, to this value. According to the tables, the degree of possibility of
≥1, and
≥1, i.e. T (
≥1), and T (
≥1) are between 0 and 1.
The proximity of T (≥1) to 1, for instance, shows the project is ahead of budget and its proximity to 0 shows the project is behind the budget. As mentioned earlier, this comparison does not always give crisp decisions, which is more appropriate and consistent with the basis of the developed model. For Scenarios 2 and 4 in and , the values of T (
≥1), and T (
≥1) are depicted in the graphical description by black bold lines (as a part of the original red line). Thus, for illustration in Scenario 2 of , the length of the black bold line inside the trapezoidal is equal to
(24)
(24)
Note that according to the fuzzy principles we cannot conclude T (≤ 1) based on T (
≥1), thus we calculated T (
≥1) separately (see ). This rule applies also to T (
≤ 1) ().
4. Case study: numerical data analysis
In this section, a case study from the construction industry is applied to exhibit how the proposed model can be employed for real case projects. This study focused on estimating the performance of building construction projects. The projects can be broken down into manageable work elements allowing these elements to be used throughout the project for planning, scheduling, budgeting, performance evaluation and most importantly, earned value analysis.
A Work Breakdown Structure is a tool that helps you plan, organize and control any project. Among the elements of the WBS, the project work can be divided into two work packages ‘sub-structure’ and ‘super-structure’. Each of these work packages consisted of 10 activities ‘Excavation, and Earth Work, ‘Masonry Work’, ‘Concrete Work’, ‘Block Work’, ‘Roofing’, ‘Metal Work and Steel Structure’, ‘Finishing’, ‘Glazing’, ‘Painting’, ‘Sanitary and Electrical Work’.
In this project, it was required to collect the cost and time information of each project activity from six real case-building projects. In carrying out this study, the objective of the study was more focused on analyzing and answering the value of the dependent variables of The planned duration for carrying out the project activities was scheduled for 30 months for Project 1, 40 months for Project 2, 20 months for Project 3, 30 months for Project 4, 18 months for Project 5 and 17 months for Project 6. However, the analysis was made based on the planned values (PV) and actual costs (AC) up to the data date of month 12 as observed in .
Table 4. The PV and the AC of the building projects.
The information regarding activity progress and activity budget at completion (BAC) are brought in . As shows, the progress of all activities is stated using linguistic terms. These linguistic terms are transformed into fuzzy numbers using and have brought in to .
Table 5. The information on the work package of the building projects.
Table 6. The percentage complete and the EV of activities for Project 1 (P1).
Table 7. The percentage complete and the EV of activities for Project 2 (P2).
Table 8. The percentage complete and the EV of activities for Project 3 (P3).
Table 9. The percentage complete and the EV of activities for Project 4 (P4).
Table 10. The percentage complete and the EV of activities for Project 5 (P5).
According to the results of Tables (6) to (11) BAC of each activity, the of each activity is calculated using EquationEquation (6)
(6)
(6) . Using EquationEquation (8)
(8)
(8) we can find the total EV of the work package, i.e. the total EV for all of the four activities of the projects.
As shows, the total AC for the work package at the data date of month 12 for the six projects are 152,205,544.90, 622,507,920.37, 396,592,035.81, 798,555,515.94, 131,685,896.73 and 204,593,727.00, respectively.
As illustrates, the total PV for the work package at the data date of month 12 for the six projects are 252,441,378.62, 612,011,954.80, 350,381,026.70, 649,719,548.52, 122,628,103.81, and 217,125,101.85, respectively.
The CPI and SPI of this work package are interpreted as follows.
According to (see Scenario 5), T (≥1) = 1 and T (
≤ 1) = 0. Thus the work package is ahead of the budget.
Scenario 1 in implies that T (≥1) = 0 and T (
≤ 1) = 1, so the work package is Behind the schedule. These results imply that this work package is ahead of the budget and behind schedule.
According to (see Scenario 1), T (≥1) = 0 and T (
≤ 1) =1. Thus the work package is behind the budget.
Scenario 1 in implies that T (≥1) = 0 and T (
≤ 1) = 1, so the work package is behind the schedule. These results imply that this work package is behind the budget and behind the schedule.
According to (see Scenario 1), T (≥1) = 0 and T (
≤ 1) =1. Thus the work package is behind the budget.
Scenario 1 in implies that T (≥1) = 0 and T (
≤ 1) = 1, so the work package is behind the schedule. These results imply that this work package is behind the budget and behind the schedule.
According to (see Scenario 1), T (≥1) = 0 and T (
≤ 1) =1. Thus the work package is behind the budget.
Scenario 1 in implies that T (≥1) = 0 and T (
≤ 1) = 1, so the work package is behind the schedule. These results imply that this work package is behind the budget and behind the schedule.
According to (see Scenario 1), T (≥1) = 0 and T (
≤ 1) =1. Thus the work package is behind the budget.
Scenario 1 in implies that T (≥1) = 0 and T (
≤ 1) = 1, so the work package is behind the schedule. These results imply that this work package is behind the budget and behind the schedule.
According to (see Scenario 2), T (≥1) =
and T (
≤ 1) =1. Thus the work package is approximately behind the budget.
Scenario 1 in implies that T (≥1) = 0 and T (
≤ 1) = 1, so the work package is behind the schedule. These results imply that this work package is behind the budget and behind the schedule.
Now, we would like to estimate the completion cost of the work package. Given the trend affecting the CPI is kept fixed during the rest of the project; the completion cost of the work package is estimated as follows: (According to , the total BAC for all activities for the six projects are 368,907,074.10, 703,054,703.97, 326,528,668.9, 762,904,871.4, 115,900,279.1 and 241,681,352 respectively.
To calculate the each of the four members of the trapezoidal
is projected on the baseline and forms a member of the trapezoidal fuzzy number ES (EquationEquation (14)
(14)
(14) ). For instance, the calculations behind the
for Project 1 is calculated as follows:
Following these calculations, =
Likewise, the remaining values are obtained as follows:
also show how should be calculated in the developed model.
Table 11. The percentage complete and the EV of activities for Project 6 (P6).
Table 12. The summary of calculations for Project 1.
Table 13. The summary of calculations for Project 2.
Table 14. The summary of calculations for Project 3.
Table 15. The summary of calculations for Project 4.
Table 16. The summary of calculations for Project 5.
Table 17. The summary of calculations for Project 6.
To derive the (EquationEquation (16)
(16)
(16) ) and the
(EquationEquation (20)
(20)
(20) given PF=
) we have
5. Discussions, managerial implications and limitations
5.1. Discussion of results
Measuring the Earned Value (EV) is one of the first stages in implementing EV management. There are some different techniques to measure the EV. The Percent Complete is the simplest and the most implemented technique for measuring the
EV; however it has the disadvantage of using subjective judgments to describe the percentage of the completed work.
On the other hand, in this case study incorporating the fuzzy principles into the EV calculations for the fuzzy estimates of both the completion cost and the completion time can assist project managers in estimating the future status of the project more robustly and reliably by using the linguistic terms and fuzzy approach for measuring the EV. The robustness and flexibility of the model provide for the accurate performance analysis of the project to model their status closer to reality. Real-life applications of the proposed fuzzy EV have motivated us to continue the research on the application of the fuzzy theory in EV calculations and interpretations. As future research directions, we are working on the generalization of the proposed method and other fuzzy number ranking methods to interpret the indices and estimates. We would also be interested in developing more comprehensive EV models by using the fuzzy theory.
The study explained thoroughly how the fuzzy EV indices and estimates can be developed and interpreted. In the interpretation of the fuzzy indices, we benefited from evaluating the degree of possibility of a fuzzy number in taking different values. However, the historical data in this technique was built up on six sets of building construction projects as the data was collected from cities, the Ministry of Construction and Housing, consultants and contractors.
Based on the results of the numerical data analysis made in Section Four, the fuzzy-based SPI and CPI value of the project can be discussed as follows.
During the prediction process as it can be observed from the calculation, the SPI and CPI values are obtained as trapezoidal fuzzy numbers. The trapezoidal fuzzy numbers are divided into 5 separate zones to facilitate the interpretation process. If the value 1 is located in each of these zones, it results in a distinctive interpretation of project cost or schedule performances. According to the results of fuzzy-based SPI and CPI value, the condition of the project can be discussed as follows.
The results of the schedule performance index and cost performance index show how efficiently the project team is using its time and cost.
Based on the results of the finding the fuzzy-based performance indices for each of the building construction projects can be compared against 1. According to in Scenario 1 implies that T (≥1) = 0 and T (
≤ 1) = 1, so the work package is behind the schedule.
Based on the results of the finding the fuzzy-based SPI value in all recorded periods for all six building projects was below one indicating unfavorable schedule variance or it means that for every Birr of work scheduled to be completed below a Birr has been completed in these periods. This implies also that, the project suffers from scheduled week performance from the schedule viewpoint, and the project situation is not proper it should be taken into consideration for further preventive actions which assist managers in following up the project in the near future much better than before in order to achieve project objectives effectively. At the same time, a late response would also cost more and the problem might grow to a stage unrecoverable. Thus, it needs to properly monitor and control the project time to recover the schedule in the remaining periods.
The value of CPI measures how well the budget of the project has been spent based on the collected data. The finding of the study revealed that the value of fuzzy-based CPI for the building Project 1 is in Scenario 5 under implies that T(≥1) = 1 and T (
≤ 1) = 0, so the work package is ahead of the budget. It means that project performance from the cost performance indexes point of view is appropriate and consequently the project will be finished with less required budget sooner than expected in comparison with planned start time. Thus, the project cost is the under-planned cost for the work performed and efficiency in utilizing the resources allocated to the project is good.
Moreover, the value of fuzzy-based CPI for Projects 2, 3, 4 and 5 in Scenario 1 implies that T (≥1) = 0 and T (
≤ 1) = 1, so the work package is behind the budget. This indicates that the project’s cost is over the planned cost for the work performed. Thus, the status was not good until the last date of the data recorded period and the project could not recover in line with the specified budget. This indicates that the project has consumed more money than planned and efficiency in utilizing the resources allocated to the project is poor. So, it needs close cost monitoring and controlling of the project to recover deviation.
Additionally, the value of fuzzy-based CPI for Project 6 in Scenario 2 implies that T (≥1) =
and T (
≤ 1) = 1, so the work package is approximately behind the budget. This shows that the efficiency in utilizing the resources allocated to the project is relatively low. Therefore, make good decisions regarding the cost of the project to solve the problems to run back the project on cost performance.
5.2. Managerial implications
This study provides some significant implications on how young researchers to conduct a study by applying a new version of the fuzzy-based earned value analysis model and also for academicians how to demonstrate its practicality in real case projects.
The study has an important implication for policymakers, project managers and practitioners. As a result of this study, policymakers in the construction industry need to create plans and specifications for implementing and evaluating their performance accordingly. Thus, the projects can use these results to establish construction policies.
This study provides a novel and extended contribution to previous studies on developing a fuzzy-based earned value analysis model for estimating the future performance of projects regarding past relative information. Incorporating the fuzzy-based models in the estimation process helps to deal with the vagueness and impreciseness of real data for the accurate performance analysis of the project to model their status closer to reality.
The fuzzy-based earned value estimation is evaluated using linguistic terms to interpret the different possible conditions of projects. Hence, the study helps to greatly assist the project managers in assessing the prospective performance of the project and alerts them in taking necessary actions.
Moreover, this study provides project practitioners with knowledge on the future performance of projects by using the applied methodologies through using the available resources.
5.3. Limitations
In the course of conducting this study, we have an awareness that the fuzzy-based EVA model is an important estimating approach that models the project status closer to reality, and it was the most thematic area that needed to be investigated. Even several studies explored the techniques of a fuzzy approach for earned value management (Chandrasekaran & Kumar, Citation2012). However, there are some limitations concerning this study. The first deficiency of the published paper is the mathematical calculation of the fuzzy-based earned value analysis model requires high computational requirements. This makes the development more complex and time-consuming as far as the formulation of the historical database is concerned.
Second, computational results of the presented model have been conducted through the classical fuzzy method from the recent literature. Thus, it is better to conduct the study in other fuzzy set theories like, interval-valued fuzzy sets theory in real-life applications to make a comparison of the results and to check the consistency of the results.
Finally, there is no fuzzy logic approach, in the literature, aimed at an earned value analysis model for estimating the performance of building construction projects through the use of fuzzy set theory is a hot research topic.
6. Conclusions and remarks
Everyone is concerned about the future; especially project managers are very much worried about the future of the project. So, the earned value analysis has always been a subject of much research used in different projects to demonstrate extracting additional information from data about the status, trend and future project schedule performance and associated cost.
However, due to the uncertain nature of the activities’ progress involved in a project, they are considered deterministic in all available EV techniques. The aim of this study present a new fuzzy-based earned value model with the advantage of developing and analyzing the earned value indices and the time and cost estimates at completion under uncertainty.
In light of the findings and discussions the main conclusions are:
Through the six projects studied until the date of the data collection period, it is shown that the deviations in time and planned cost.
The project performance from the schedule performance indexes point of view the current situation for all six projects is not proper and it should be taken into consideration for further preventive actions to complete the project accordingly.
Likewise, the project performance from the cost performance indexes point of view the status of the projects excluding Project 1 are not in a good condition, and it consumed more money than planned and efficiency in utilizing the resources allocated to the project is poor. The project managers to follow up on the project soon much better than before to achieve project objectives effectively.
The model developed in this study is practical and provides reliable figures during the project construction period which helps stakeholders make better decisions on the project cost and project time.
This research is an important addition to the world of knowledge, which aims to improve the appreciation of project objectives and increase confidence.
The fuzzy evaluation system is designed to provide practitioners with a fuzzy point of view to the traditional performance evaluation model for dealing with imprecision.
The proposed method enables decision analysts to better understand the complete evaluation process. Furthermore, this approach provides a more accurate, effective and systematic decision-support tool.
The data collection and converting it into Fuzzy system mode reduces the chances of errors caused by units of parameters which also creates problems for mathematical calculations. To overcome these challenges the concept of the Fuzzy modeling approach is an ideal method in earned value analysis.
Moreover, to develop the project management proficiency that the current condition of project performances is according to the plan, the organizations need to look at the critical elements of EVM as pragmatic stepping stones to prioritizing which project management processes are most important for successful delivery.
The study further provides to monitor the current project performance and forecast the future performance by integrating the scope, schedule and cost of the project. It is also useful to demonstrate past performance and current performance and predict the future performance of the project by reducing a large amount of issues arising out of schedule and cost overruns problems by incorporating fuzzy set theory.
Generally adopting the proposed model in building construction projects extends the applicability of the EV techniques under real-life and uncertain conditions. Thus, using integrated time series and simulation is recommended for further development of the proposed model.
Disclosure statement
No potential conflict of interest was reported by the author(s).
Data availability statement
The source of data used to support the findings of this study is available from the corresponding author upon request.
Additional information
Funding
Notes on contributors

Girmay Getawa Ayalew
Girmay Getawa Ayalew is currently working as a lecturer at the Woldia Institute of Technology, Woldia University, P.O. Box 400, Woldia, Ethiopia. He is former a lecturer at the Gondar Institute of Technology, University of Gondar, P.O. Box 196, Gondar, Ethiopia. He received his BSc degree in Construction Technology and Management from Woldia University and his MSc degree in Construction Engineering and Management from the University of Gondar. His research interests include earned value analysis, system analysis, fuzzy logic regression modeling, emerging technologies in construction and project management. Email: [email protected]. Institutional Email: [email protected].
Genet Melkamu Ayalew
Genet Melkamu Ayalew is currently working as a lecturer at the Woldia Institute of Technology, Woldia University, P.O. Box 400, Woldia, Ethiopia. She is former a lecturer at the Gondar Institute of Technology, University of Gondar, P.O. Box 196, Gondar, Ethiopia. She received her BSc degree in Construction Technology and Management from Debre Berhan University and her MSc degree in Construction Engineering and Management from the University of Gondar. Her research interests include earned value analysis, performance management, project cost estimation and regression modeling. Email: [email protected]. Institutional Email: [email protected].
References
- Acebes, F., Poza, D., González-Varona, J. M., & López-Paredes, A. (2022). Stochastic earned duration analysis for project schedule management. Engineering, 9, 148–161. https://doi.org/10.1016/j.eng.2021.07.019
- Araszkiewicz, K., & Bochenek, M. (2019). Control of construction projects using the earned value method - Case study. Open Engineering, 9(1), 186–195. https://doi.org/10.1515/eng-2019-0020
- Asgari Dehabadi, M. M., Salari, M., & Mirzaei, A. R. (2014). Estimation of project performance using earned value management and fuzzy regression. Shiraz Journal of System Management, 2(1), 105–122.
- Ayalew, G. G., Ayalew, G. M., & Meharie, M. G. (2023). Integrating exploratory factor analysis and fuzzy AHP models for assessing the factors affecting the performance of building construction projects : The case of Ethiopia. Cogent Engineering, 10(1), 1–38. https://doi.org/10.1080/23311916.2023.2243724
- Ayalew, G. G., Meharie, M. G., & Worku, B. (2022). A road maintenance management strategy evaluation and selection model by integrating Fuzzy AHP and Fuzzy TOPSIS methods : The case of Ethiopian Roads Authority. Cogent Engineering, 9(1), 1–35. https://doi.org/10.1080/23311916.2022.2146628
- Ayalew, G. M., Meharie, M. G., & Ayalew, G. G. (2023). Regression modeling for prediction of earned value indexes in public building construction projects : The case of Ethiopia. Cogent Engineering, 10(1), 1–30. https://doi.org/10.1080/23311916.2023.2220497
- Birhanu Belete, S., Getnet Meharie, M., & Getawa Ayalew, G. (2024). Development of a mathematical model to predict the cost of Ethiopian Roads Authority road projects: The case of Ethiopia. Cogent Engineering, 11(1), 1–21. https://doi.org/10.1080/23311916.2023.2297492
- Chandrasekaran, R., & Kumar, R. (2012). Application of logistic regression to predict over target baseline of software projects. International Journal of Computer Applications, 44(10), 1–6. https://doi.org/10.5120/6296-8493
- Huang, H., & Ho, C. (2016). Applying the fuzzy analytic hierarchy process to consumer decision-making regarding home stays. 5(February 2013). 981–990. https://doi.org/10.4156/ijact.vol5.issue4.119
- Iranmanesh, S. H., & Zarezadeh, M. (2008). Application of artificial neural network to forecast actual cost of a project to improve earned value management system. In Seyed Hossein Iranmanesh, and Mansoureh Zarezadeh (pp. 210–213). World Academy of Science, Engineering and Technology.
- Jie, D., & Wei, J. (2022). Estimating construction project duration and costs upon completion using Monte Carlo simulations and improved earned value management. Buildings, 12(12), 2–23. https://doi.org/10.3390/buildings12122173
- Kabir, G., & Hasin, M. A. A. (2011). Evaluation of customer oriented success factors in mobile commerce using fuzzy AHP. Journal of Industrial Engineering and Management, 4(2), 361–386. https://doi.org/10.3926/jiem.2011.v4n2.p361-386
- Moradi, N., Mousavi, S. M., & Vahdani, B. (2020). A new version of earned value analysis for mega projects under interval-valued fuzzy environment, 13(2), 57–72. https://doi.org/10.22094/JOIE.2016.267
- Moslemi Naeni, L., Shadrokh, S., & Salehipour, A. (2014). Erratum to A fuzzy approach for the earned value management [International Journal of Project Management, 32, (2014), 709-716]. International Journal of Project Management, 32(4), 709–716. https://doi.org/10.1016/j.ijproman.2013.02.002
- Narbaev, T. (2011). Cost estimate at completion methods in construction projects. 10, 1–6. https://doi.org/10.13140/2.1.1898.3369
- Noori, S., Bagherpour, M., & Zareei, A. (2008). Applying fuzzy control chart in earned value analysis: A new application. Complete Fuzzy Scheduling and Fuzzy Earned Value Management in Construction Projects, 3(4), 684–690.
- Obianyo, J. I., Udeala, R. C., & Alaneme, G. U. (2023). Application of neural networks and neuro-fuzzy models in construction scheduling. Scientific Reports, 13(1), 8199. https://doi.org/10.1038/s41598-023-35445-5
- Ponz-Tienda, J. L., Pellicer, E., & Yepes, V. (2012). Complete fuzzy scheduling and fuzzy earned value management in construction projects. Journal of Zhejiang University Science A, 13(1), 56–68. https://doi.org/10.1631/jzus.A1100160
- Prasanth, A., & Thirumalai Raja, K. (2007). Project performance evaluation by earned value method. International Journal of Innovative Research in Science, Engineering and Technology, 3297(1), 73–81.
- Russo, R. D. F. S. M., & Camanho, R. (2015). Criteria in AHP: A systematic review of literature. Procedia Computer Science, 55(Itqm), 1123–1132. https://doi.org/10.1016/j.procs.2015.07.081
- Salari, M., Bagherpour, M., & Wang, J. (2014). A novel earned value management model using Z-number. International Journal of Applied Decision Sciences, 7(1), 97–119. https://doi.org/10.1504/IJADS.2014.058037
- Simion, C. P., & Marin, I. (2018). Project cost estimate at completion: Earned value management versus earned schedule-based regression models. A comparative analysis of the models application in the construction projects in Romania. Economic Computation & Economic Cybernetics Studies & Research, 52(3), 205–216. https://doi.org/10.24818/18423264/52.3.18.14
- Sun, C. C. (2010). A performance evaluation model by integrating fuzzy AHP and fuzzy TOPSIS methods. Expert Systems with Applications, 37(12), 7745–7754. https://doi.org/10.1016/j.eswa.2010.04.066
- Suresh, S., & Ramasamy, G. (2017). Analysis of project performance using earned value analysis, 4, 1080–1085.
- Vyas, A. B., & Birajdar, B. V. (2016). Tracking of construction projects by earned value management. International Journal of Engineering Research, V5(03), 829–831. https://doi.org/10.17577/ijertv5is031318
- Zadeh, L. A. (1965). Fuzzy sets. Information and control, 8(1965), 338–353.