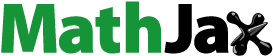
Abstract
In this subsection, we presented a mathematical model of Ebola virus (EBOV) proposed by susceptible exposed infected recovered (SEIR) model. In our model, the population is affected by animals. EBOV is an infectious agent causing haemorraghic fever, a severe infectious disease characterised by high fever and bleeding, in humans and some monkeys. Here, we assessed the transmissibility associated with the infection stages of EBOV that generated an epidemic model. In order to do this, in the first step, we formulate the model, and the basic properties of the proposed model are presented. The basic reproductive number is obtained by using the next generation matrix approach. Then, all the endemic equilibrium points related to the disease are derived. We also find the conditions to investigate all possible equilibria of the model in terms of the basic reproduction number (local and global stability). In last, numerical simulation is presented with and without vaccination or control for the proposed model.
PUBLIC INTEREST STATEMENT
Viruses are considered as a big threat all over the world. All viruses are harmful but now a days Ebola virus is baneful disease either in urban and some parts of rural areas, which become epidemic slowly. Here, we assessed Ebola SEIR mathematical model, and the transmissibility agent of the virus is animals, which spread chronic disease both in human and monkey. This study will help the people to care about animals because no corner of the world is without animals. Ebola virus is so harmful and transmissible disease even if an individual gets infection from Ebola virus, it will spread to other individual or family member. Thus, to avoid from Ebola virus and for good environment of human health, this study provides a range of safety in life to treat the animals in a safety way.
Competing Interests
The authors declares no competing interests.
1. Introduction
The recent Ebola virus (EBOV) epidemic in West Africa emerged around the end of 2013 in the prefecture of Guckdou in Guinea (Baize et al., Citation2014) and caused at least 11,310 deaths among 28,616 recorded cases in Guinea, Sierra Leone, and Liberia (World Health Organization, Citation2016c). It has been argued that the West African EBOV epidemic-illustrated problems in the early detection of, and rapid response to, infectious disease outbreaks of public health importance. Various reasons may explain the slow initial response to the West African EBOV epidemic, including poor public health infrastructure and local unfamiliarity with EBOV disease, as well as a lack of preparedness by the international community. Because efforts to control the epidemic could not rely on vaccination or effective antiviral drugs, the outbreak response focused on standard medical practices (e.g. case identification and isolation), as well as community practices (e.g. sanitary funeral practices) (Blackwood & Childs, Citation2016).
Mathematical models have been used extensively to study the dynamics of EBOV transmission e.g. superspreading events (Lau et al., Citation2017), also M.Tahir. et al. also presented mathematical model for EBOV (Tahir, Inayat Ali Shah, Zaman, & Muhammad, Citation2018). EBOV is one of the four EBOVs known to cause disease in humans. It has the highest case-fatality rate of these EBOVs, averaging 83% since the first outbreaks in 1976, although fatality rates up to 90% have been recorded in one outbreak (200,203). There have also been more outbreaks of EBOV than of any other EBOV. In 1976, the first EBOV was found of Marburg virus (Bowen et al., Citation1977; Pattyn, Jacob, van der Groen, Piot, & Courteille, Citation1977). In the mean while, other team introduced EBOV (Bowen et al., Citation1977; Kuhn et al., Citation2010; Pattyn et al., Citation1977). The name Ebola virus is derived from the Ebola River, a river that was at first thought to be in close proximity to the area in Democratic Republic of Congo. To avoid from the confusion they renamed in 2010 that Ebola virus.
The incubation period, that is, the time interval from infection with the virus symptoms is 2 to 21 days. Humans are not infectious until they develop symptoms. The family of the related virus include (1) Cuevavirus,(2) Marburgvirus, and (3) Ebolavirus. Majority of human death occurred by EBOV, and in West Africa, it becomes epidemic in 2013 to 2015 (World Health Organisation, Citation2016a). Some cases reported out from West Africa reveal that all infected are foreign travellers who exposed to affected regions while later they showed Ebola fever symptoms when reached to destinations (Khan, Islam, Arif, & Ul Haq, Citation2013). In this period, the virus caused nearly about 28,616 are suspected while exactly 11,310 confirmed deaths cases (Reardan, Citation2014; Bausch & Schwarz, Citation2016b). The EBOV spread in many countries, which started in Guinea and moved across Liberia and Sierra Leone. The EBOV also spreads by human to human contacts such as secretions, blood, body fluids of the infected individuals, surfaces, and the materials of infected like cloth and bedding.
The virus causes serious acute illness and becomes fatal if the patient takes no treatment. The EBOV causes an acute, serious illness, which is often fatal if untreated. Pathogen genome sequencing is also being used to assist with the identification of unknown infection sources and transmission chains, as pathogen genomes contain valuable information that complements contact tracing efforts. In the case of EBOV, Arias et al. (Citation2016) demonstrated that rapid outbreak sequencing in locally established sequencing facilities can identify transmission chains linked to sporadic cases. In addition to identifying specific transmission pathways, pathogen genome analyses can also shed light on the origins, evolution and transmission dynamics of a pathogen during an epidemic (Holmes, Dudas, Rambaut, & Andersen, Citation2016). Early in the EBOV epidemic, analyses such as those by Gire et al. (Citation2014) demonstrated that the virus entered the human population in late 2013 and crossed from Guinea to Sierra Leone in May 2014 through sustained human-to-human transmission.
In this article, we processed as fallows: First, the disease according to their infection has been formulated, then the key value reproductive number is derived. After that endemic equilibrium points are obtained. Then, the local stability analysis is shown stable at disease free, as well as, at endemic equilibrium. Further, we derived the global stability of the model with the help of Lyapunov function has been discussed at disease free, and at endemic equilibrium. Finally, we show numerically the result with and without vaccination or control which supports the model.
2. Model formulation and method
In this subsection, we presented Ebola SEIR mathematical model (Anderson & May, Citation1991; Keeling & Rohani, Citation2007). In this model, virus is transmitted from wild and domestic animals. The proposed model is defined in the following four classes: first class is about susceptible individuals and represented by whose are not still infected but have a chance to be suffer in the said disease in any time t . Second class is about
that is, exposed individuals showing no symptoms of virus. The third class is about infected individuals and represented by
who infected by EBOV in any time t where the fourth class is the class of
that is, the people recovered from EBOV. Then the differential equations of the mathematical SEIR model of the EBOV are represented as:
With the initial conditions,
Certain assumptions have been used in model (1), which are classified as, represents susceptible individuals,
shows exposed individuals,
represents infected individuals,
represents recovered individuals,
represents new birth rate inter in the susceptible individuals,
shows natural death rate of susceptible individuals,
shows transmission rate from susceptible to infected individuals through wild animals,
represents the transmission rate from susceptible to infected individuals through domestic animals,
shows the transmission rate from susceptible to exposed individuals,
represents transmission rate from exposed to infected individuals,
represents the rate of infected to recovered individuals,
and
show natural death rate and infectious death rate of exposed individuals,
and
represent natural and infectious death rate of infected individuals, respectively.
The total population of the model is represented by,
Which will be written as,
Using values from model (1), we get the following result.
While from EquationEquation (2)(2)
(2) we write that,
Clearly
Now, the feasible region, which is sufficient for the study of the model (1), that is, R is., as.
3. 
the reproduction number of model
Reproductive number is considered as one of the important key values in many epidemiological work represented by , which predicts that where the infectious disease will be spread into a population class or not. We define the basic reproduction number as it shows average rate of a secondary infectious cases when one of the infectious individuals introduced in a susceptible population (Keeling & Rohani, Citation2007). In many epidemiological models, the basic reproduction number is one of the key values that can predict whether the infectious disease will spread into a population or die out. The basic reproduction number is the average rate of secondary infectious cases when one infectious individual is introduced in a susceptible population. In this section, we used the concept of next generation matrix method, which was developed by Driessche et al., (Anderson & May, Citation1991). For this, we divide the system as follows,
We have also,
Now to find
and
we processed as.
So (Reproductive number) of our model (1) is given below,
4. Endemic equilibrium points of model
In this section, we find the endemic equilibrium points, which also play important role in any epidemiological model. Following are the endemic equilibrium points of the concerned model.
Where the value of the term is given below,
5. Local stability analysis of proposed model
In this area of the model, we work on the model local stability analysis. The local stability analysis needs to be found on disease-free equilibrium and endemic equilibrium.
6. Local stability analysis at disease-free equilibrium
Now the local stability analysis at disease-free equilibrium of the model (1), is, which implies in disease-free form as
thus we processed by the following Jacobian matrix at
, under,
Thus, for disease-free equilibria at local stability analysis, we have the following theorem.
Theorem 8.1. At disease-free equilibrium if
, then the concern model
is locally asymptotically stable, while if
we said the model is unstable.
Proof: We have the following eigenvalues from Jacobian matrix in EquationEquation (4)
(4)
(4) ,
Now, we discuss the local stability analysis at disease-free equilibrium as it is clear from EquationEquation (5)(5)
(5) , that is
Taking EquationEquation (6)
(6)
(6)
, it implies that
if and only if
which is clear from EquationEquation (7)
(7)
(7) , that is,
So it clearly shows
. So
iff
From EquationEquation (8)
(8)
(8)
iff
which completes the proof. Hence, we say that local stability analysis at disease-free equilibrium of the model (1) is asymptotically stable.
7. Local stability analysis at endemic equilibrium
Now, in this subsection of the article, we find stability analysis of the model (1) at endemic equilibrium. For local stability analysis at endemic equilibrium, we have the following result.
Theorem 9.1. Local asymptotical stability at endemic equilibrium, will hold if for model
that is, at
and unstable if
.
Proof: For stability analysis at endemic equilibrium, consider the matrix, thus
The above terms are specified below,
Thus for endemic equilibrium, we get,
Now, we discuss the endemic equilibrium points, from EquationEquation (9)(9)
(9)
so
iff
and
. Now using EquationEquation (10)
(10)
(10)
if and only if
and
. Now, we check the value of
from EquationEquation (11)
(11)
(11) we observed that
iff
by performing some calculation we observed that
Taking EquationEquation (12)
(12)
(12) and performing some calculation then
if and only if
Clearly, local stability analysis at endemic equilibrium is asymptotically stable for system (1), which completed the proof.
8. Global stability analysis of the proposed model
In this section, we discuss the global stability analysis of the problem because the global stability analysis is considered one of the fundamental. There is a power full tool Lyapunov function is used for the global stability analysis of the model. To check the global stability analysis of our model (1), we construct a Lyapunov function, which used many authors also in their models (Reardan, Citation2014; Van Den Driessche & Watmough, Citation2002). For the global stability analysis of model (1) at disease-free equilibrium, considered the following Lyapunov function. Now we have the following known stability results which are stated below,
9. Global stability analysis at disease free equilibrium
To prove global stability analysis at disease-free equilibria, we construct Lyapunov function for which the following result states as under,
Theorem 11.1. For system if
, then Globally asymptotically stability, well hold for disease-free equilibrium if
and unstable for
Proof: To show global stability at disease-free equilibrium of the model (1), considered the following Lyapunov function,
Obviously, the ‘above function is greater then zero at disease-free equilibrium and equal to zero at , and
. Differentiating
with respect to
, we obtain the following result using model (1),
After some simplification we get,
Clearly Equation (13) is less then zero if and only if , where
And
Here, we see that if and only if
,
,
, and
while
iff
. Then the disease-free equilibrium is globally asymptotically stable.
10. Global stability analysis at endemic equilibrium
In this subsection, we construct the following Lyapunov function to verify the endemic equilibrium, as
Theorem 12.1. For globally asymptotically stability for , then the endemic equilibrium of model
is stable, if
,
,
and unstable, if
.
Proof: For global stability analysis at endemic equilibrium, we define the following Lyapunov for model ,
the define function and it is equal to zero at
,
,
Differentiating
with respect to ‘t’ we get,
Putting values from model (1) in above then we obtain,
Hence we have if and only if
and
and
,
also
, iff
from above it is clear that endemic equilibria is globally asymptotically stable for model
. So the proof is completed.
11. Numerical simulation and discussion
Work in this subsection contains numerical interpretation of the proposed model by the use of Runge-kutta method, that is, . We use different parameters. Its values and numerical result are stated in Figures –. In this section, the numerical simulations of the proposed model (1) are presented for the verification of analytical results. The numerical results are obtained by using the Runge-Kutta method of order four. The parameter values used in the simulation are given in Table , which are biologically feasible. By using the parameter values, non-negative initial population sizes and from the time interval, we obtain the simulation (Figures –), which represents that there are always susceptible S(t) and recovered R(t) population which quickly recovered with vaccination, while the remaining individuals i.e., exposed E(t), and infected I(t) individuals, respectively, show that exposed also need vaccination, while without vaccination exposed class getting health but very slowly, while vaccine provides a rapid health recovery. Similarly, if we do not provide vaccine to the infected class, we see from the graph, simulation goes high but vaccination rapidly covers their problem. All values taken are fixed in the table above. In Figures –, the simulation is presented with vaccination to the population (Khan et al., Citation2013).
Table 1. Description of parameter and its values
12. Conclusion of the proposed model
A compartmental (SEIR) mathematical epidemic model of the EBOV is considered. In our proposed model, the transmissibility agent is considered animal at any time t in the population. First, we formulated the model, and obtained key number reproductive number, that is, R0. After that, we discussed endemic equilibrium points of the model. Then, according to the reproductive number, we discussed the local stability and global stability at disease-free equilibrium and at endemic equilibrium and shown stable. Finally, we obtained numerical solution of compartmental mathematical model by the use of Runge-Kutta method RK4 tool and presented the results from Figures – with vaccination which support the model.
Conflict of Interest
There is no conflict of interest regarding this paper.
Authors contribution
All authors equally contributed this paper.
Acknowledgements
All authors read and approved the final version.
Additional information
Funding
Notes on contributors
Muhammad Tahir
Muhammad Tahir is the corresponding author and his research areas are Mathematical Biology, and fluid dynamics. He is a Ph.D research scholar in Mathematics, Islamia College Peshawar, 25,000, Pakistan. E-mail addresses: [email protected]; Tel.: +92-345-9063508
Nousheen Anwar
Nousheen Anwar student in MS at Peshawar University, 25000, Department of Bio Chemistry, Email addressses:[email protected]
Syed Inayat Ali Shah
Syed Inayat Ali Shah is currently working as a professor and dean in mathematics at Islamia College Peshawar, 25000. He is Ph.D. from Saga University Japan in 2002. E-mail addresses:[email protected]
Tahir Khan
Tahir Khan Ph.D is research scholar and lecturer in Mathematics at the University of Malakand lower ir.E-mail addresses:[email protected]
References
- Anderson, R. M., & May, R. M. (1991). Infectious diseases of humans: Dyn and Cont. Oxford, UK: Oxford University Press.
- Arias, A., Watson, S. J., Asogun, D., Tobin, E. A., Lu, J., Phan, M. V. T., ... Cotten, M. (2016). Rapid outbreak sequencing of Ebola virus in Sierra Leone identifies transmission chains linked to sporadic cases. Virus Evolution, 2, vew016.
- Baize, S., Pannetier, D., Pharm, D., Oestereich, L., Rieger, T., & Koivogui, L. (2014). Emergence of Zaire Ebola virus disease in Guinea. The New England Journal of Medicine, 371, 1418–11. doi:10.1056/NEJMoa1410490
- Bausch, D. G., & Schwarz, L. (2016b). Outbreak of Ebola virus disease in Guinea: Where ecology meets economy. Retrieved from https://doi.org/10.1371/journal.pntd.0003056
- Blackwood, J. C., & Childs, L. M. (2016). The role of interconnectivity in control of an Ebola epidemic. Scientific Reports, 6, 29262. doi:10.1038/srep29262
- Bowen, E. T. W., Lloyd, G., Harris, W. J., Platt, G. S., Baskerville, A., & Vella, E. E. (1977). Viral haemorrhagic fever in southern Sudan and northern Zaire. Preliminary studies on the aetiological agent. Lancet, 309(8011), 5713. PMID 65662. doi:10.1016/s0140-6736(77)92001-3
- Gire, S. K., Goba, A., Andersen, K. G., Sealfon, R. S. G., Park, D. J., Kanneh, L., … Sabeti, P. C. (2014). Genomic surveillance elucidates Ebola virus origin and transmission during the 2014 outbreak. Science, 345, 1369–1372. doi:10.1126/science.1259657
- Holmes, E. C., Dudas, G., Rambaut, A., & Andersen, K. G. (2016). The evolution of Ebola virus: Insights from the 20132016 epidemic. Nature, 538, 193200.
- Keeling, M., & Rohani, P. (2007). Modelling infectious diseases in humans and animals. Princeton, NJ: Princeton University Press.
- Khan, M. A., Islam, S., Arif, M., & Ul Haq, Z. (2013). Transmission model of hepatitis B virus with the migration effect. Hindawi Publishing Corporation BioMed Research International, 150681, 10.
- Kuhn, J. H., Becker, S., Ebihara, H., Geisbert, T. W., Johnson, K. M., Kawaoka, Y., … Jahrling, P. B. (2010). Proposal for a revised taxonomy of the family filoviridae: Classification, names of taxa and viruses, and virus abbreviations. Archives of Virology, 155(12), 2083103. PMC 3074192?Freely accessible. PMID 21046175.. doi:10.1007/s00705-010-0814-x
- Lau, M. S. Y., Dalziel, B. D., Funk, S., McClelland, A., Tiffany, A., Riley, S., … Grenfell, B. T. (2017). Spatial and temporal dynamics of superspreading events in the 2014–2015 West Africa Ebola epidemic. Proceedings of the National Academy of Sciences, 114, 2337–2342. doi:10.1073/pnas.1614595114
- Pattyn, S., Jacob, W., van der Groen, G., Piot, P., & Courteille, G. (1977). Isolation of Marburg-like virus from a case of haemorrhagic fever in Zaire. Lancet, 309(8011), 5734. PMID 65663. doi:10.1016/s0140-6736(77)92002-5
- Reardan, S. (2014). The first nine months of the epidemic and projection, Ebola virus disease in west Africa. archive of Ebola Response Team. New England Journal of Medicine, 511(75.11), 520.
- Tahir, M., Inayat Ali Shah, S., Zaman, G., & Muhammad, S. (2018). Ebola virus epidemic disease its modeling and stability analysis required abstain strategies. Cogent Biology, 4(1), 1488511. doi:10.1080/23312025.2018.1488511
- Van Den Driessche, P., & Watmough, J. (2002). Reproduction numbers and sub threshold endemic equilibria for compartmental models of disease transmission. Mathematical Biosciences, 180, 29–48. doi:10.1016/S0025-5564(02)00108-6
- World Health Organisation. (2016a). Ebola data and statistics.
- World Health Organization. (2016c). Ebola situation report. Retrieved from apps.who.int/iris/bitstream/10665/208883/1/ebolasitrep-10Jun2016-eng.pdf