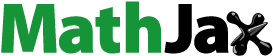
OCCUPATIONAL APPLICATIONS
A long-standing debate has surrounded the factors that lead to drone mishaps. The results of our study indicate that, from the perspective of drone pilots, situational awareness, decision-based, and skill-based errors are the primary human-factors relevant causes of drone mishaps. Additionally, deficiencies in drone interfaces should be addressed comprehensively to ensure humans can more precisely control drones. Our findings suggest that following safety procedures, receiving technical training, and flying outdoors were associated with a reduced risk of drone-related mishaps at work.
TECHNICAL ABSTRACT
Background: Commercial drones are rapidly transforming business operations, however there is a paucity of research evaluating occupational hazards and risks associated with drone deployment in the workplace.Purpose: We aimed to identify challenges of human-drone collaborations and assess drone pilot perceptions of workplace safety.Methods: An online questionnaire was generated and sent to 308 drone pilots working in different industries. A total of 75 of responses were included for data analysis. Descriptive statistics, principal component analysis, and association rule mining were employed to extract knowledge from the obtained data.Results: Our results indicate that human factors are the main contributors to workplace drone mishaps. Poor communication, information display, and control modes were found to be chief obstacles to effective human-drone collaboration. Drone pilots indicated a propensity for complying with and participating in safety practices. Following safety procedures, receiving technical training, and flying outdoors may all be associated with a lower risk of drone mishaps.Conclusions: Offering professional training to pilots and following safety procedures could decrease the risks associated with occupational drones.
1. Introduction
Unmanned aerial vehicles (UAVs), colloquially known as “drones,” have risen in popularity in recent years. Drones are manufactured in a wide variety of types, sizes, and capabilities to accommodate civil, commercial, and industrial needs while also enhancing productivity and efficiency (Alwateer et al., Citation2019; Howard et al., Citation2018; Mukhamediev et al., Citation2021; Rahmani, Citation2019; Zhen et al., Citation2023). UAVs can enhance workplace safety by assisting in emergencies and undertaking tasks that would be hazardous for humans, such as performing oil and gas rig safety inspections. Furthermore, drones can enter confined and inaccessible spaces, as well as ignitable and toxic environments (Irimia et al., Citation2019; Occupational Safety and Health Administration (OSHA), Citation2018).
The growing demand for drone technology has challenged the basic tenets of privacy and safety (Bernauw, Citation2016; Luppicini & So, Citation2016; Smith et al., Citation2022; Vacek, Citation2018; Xu & Turkan, Citation2022; Zwickle et al., Citation2019). Well-founded concerns about the erosion of privacy via the presence of drones reinforces the importance of the public’s right to privacy under the Fourth Amendment (Koerner, Citation2015). Similarly, several disturbing implications arise from employing drones, such as personal injuries and property damage. The introduction of UAVs into the workplace may also carry safety risks for pilots due to their close proximity to UAVs, putting them at a higher risk of injury. These concerns should be carefully assessed to ensure that the risk-benefit balance of this robotic innovation is favorable (Choi, Citation2021). Despite growing commercial use of drones across various industries, areas requiring special attention that appear to be ignored in drone safety research thus far are occupational hazards and the risks of drone use to pilot well-being and health in the workplace.
The causes of drone mishaps have been a subject of debate (Fernando, Citation2017; Kasprzyk & Konert, Citation2021). Wild et al. (Citation2016) argued that the majority of drone mishaps stem from UAV technical issues. Likewise, Williams (Citation2004) claimed that electromechanical malfunctions play a more pronounced role in drone mishaps in U.S. military UAVs than human factors. It has been demonstrated that software failures, poor navigation systems, and deficiencies in ground control systems are among the primary causes of drone mishaps related to UAV technical issues (Foreman et al., Citation2015; Hoem et al., Citation2021). In contrast, other research has identified that negative organizational influences, lack of pilot vigilance, operator overconfidence and inattentiveness, fatigue, heavy workloads, as well as low levels of situational awareness and poor crew coordination are among the main causes of drone mishaps attributed to human factors (Arrabito et al., Citation2010; Citation2021; Cardosi & Lennerts, Citation2017; Tvaryanas et al., Citation2005). According to Arrabito et al. (Citation2021), providing effective training and enhancing human-machine interfaces via multimodal displays with auditory and tactile cues can minimize the risk of mishaps due to human factors. It is also important to consider environmental differences and weather conditions, as these aspects have a significant impact on drone flyability and reliability (Gao et al., Citation2021; Khosiawan & Nielsen, Citation2016). While indoor flights can avoid extreme weather conditions, flying UAVs outdoors with enough room to land and take-off is simpler when compared to indoor spaces cluttered with obstructions. Under certain circumstances, such as on sunny days, outdoor spaces also provide adequate lighting and illumination from the sky, making obstacles more visible to drone sensors. Conversely, walls with repetitive textures in indoor environments provide fewer visual cues for drones to detect and avoid other airborne objects (De Croon & De Wagter, Citation2018).
Although UAVs can protect employees by venturing into dangerous environments where there exist threats to human health and safety, sharing the workplace with drones can heighten the risk of injury (Smith, Citation2019). Arterburn et al. (Citation2017) evaluated the severity of UAV collisions with a person on the ground by conducting several simulations. They claimed that among both pilots and members of the public potentially in harm’s way, blunt force trauma, penetration, and laceration injuries may be the most common and could result in death or lifelong disability (Arterburn et al., Citation2017). Notably, Campolettano et al. (Citation2017) suggested that the risk of developing a serious neck injury (AIS 3+) and a concussion as a result of falling impacts from UAVs can be as high as 70 and 100%, respectively. Moreover, the technology-related distraction from flying drones at work can present occupational hazards to construction workers (Namian et al., Citation2021), who are at greater risk of falling from heights. This evidence, thereby, can underscore the importance of using personal protective equipment (PPE), including hard hats, gloves, safety glasses, steel-toe boots, earplugs, vests, and full body suits, which can reduce exposure to hazards if used properly.
UAV safety laws, regulations, and standards have not kept pace with the rapid expansion of the drone industry (Tran & Nguyen, Citation2022). By default, the commercial use of drones in the United States is subject to Federal Aviation Administration (FAA) regulations, which mainly voice concerns about the safety of the general public who are not directly participating in operations, rather than pilots (ECFR, Citation2016). To adopt a holistic view of workplace safety, the National Institute for Occupational Safety and Health (NIOSH) has established a Center for Occupational Robotics Research, wherein drones are classified under the umbrella term of occupational robotics (NIOSH, n.d). Similarly, the American National Standards Institute (ANSI) has published a roadmap to identify existing standards and discuss areas of opportunities for the future standardization landscape of UAVs (ANSI, Citation2018). These efforts highlight the ongoing need for greater harmonization of UAV regulations with occupational safety requirements.
The confluence of shared values, perceptions, and attitudes by employees shapes an organization’s commitment to workplace safety (Griffin & Neal, Citation2000; Helmreich, Citation1999). It follows that management involvement in fostering robust safety practices that proactively promotes safety participation, compliance, and a blame-free working environment is critical to the safe operation of UAVs (Griffin & Hu, Citation2013; Lamb, Citation2019). Additionally, incorporating innovative knowledge transfer methods, such as simulation and virtual reality, into occupational training and development programs can establish workplace safety behaviors (Karagiannis et al., Citation2021; Li et al., Citation2022). In fact, thinking fast, acting swiftly, and the acquisition of spatial cognitive mapping and orientation skills are prerequisites to mastering flying (Arnold et al., Citation2013). Humans can naturally maintain orientation in a two-dimensional environment, on the ground. However, this innate ability must be learned and acquired through technical training, since navigating in three-dimensional space can cause spatial disorientation, which is responsible for five to 10% of aviation accidents (Antunano, Citation2005).
In this study, we examined human-drone collaboration challenges and investigated workplace safety practices among UAV pilots through a survey. This research is unique in several ways. This study is among the first to assess occupational risks and hazards of deploying drones in the workplace. Drone operators, as human workers in the gig economy, have been largely overlooked regarding occupational safety and health considerations. In addition, we sought opinions from drone pilots working in diverse occupational settings—ranging from construction to public safety—on the challenges of human-drone collaborations, which may inspire the design of enhanced UAV control interfaces. The findings of this study may inform occupational safety stakeholders of workplace drone mishaps to seek data-driven and evidence-based policy reform to create safer work environments.
2. Methods
2.1. Participants
The primary inclusion criteria for the participants were that they were at least 18 years of age and were using UAVs at work. A publicly available list of commercial drone operators provided by State of Minnesota Department of Transportation, Office of Aeronautics (Citation2023) was used. A total of 308 drone pilots were contacted directly by email. The invitation to participate in the study was also announced on the American Society of Safety Professionals’ website and LinkedIn pages, as well as on the Skydio Drone Community forum and Commercial Drone Pilots Forum (American Society of Safety Professionals, n.d.; CommercialDronePilots, n.d.; SkydioPilots, n.d.). A total of 153 drone pilots completed and returned the questionnaire (49.7% response rate). Data cleaning was performed by examining the collected data, survey completion times, and participant metadata. It should be emphasized that the estimated time for completing the survey was 15–20 minutes, as stated in the survey consent form, therefore cases in which completion time was less five minutes were identified as careless responders and excluded (Bais et al., Citation2020; Meade & Craig, Citation2012). To ensure data integrity, incomplete responses and fraudulent responses, known as alias fraud, which involves an individual disguising their identity and submitting multiple responses in order to receive monetary incentives, were also discovered and eliminated accordingly (Lawlor et al., Citation2021). As a consequence, 75 total responses were used for subsequent data analysis.
2.2. Survey Development
The initial form of the survey was designed as a self-completion questionnaire, deriving inspiration from National Aeronautics and Space Administration (NASA)’s Unmanned Aircraft Systems Integration in the National Airspace System Project and Indoor Safety Guidelines developed by Princeton University (Hobbs & Lyall, Citation2016; Princeton University, n.d.). A consent form was created using the Ohio University Consent Form template to relay key information about the study to participants. The study procedures were approved by the Ohio University Institutional Review Board (IRB # 21-X-110). An online questionnaire was crafted using Qualtrics and submitted to a panel of four safety professionals to identify potential safety risks to drone operators, as well as the content validity of the questionnaire (Qualtrics, n.d.). Moreover, an FAA-authorized commercial drone pilot operating under 14 CFR Part 107 was included in the research team as a collaborator to fine-tune the questionnaire and data to be captured. presents the survey questions, comprising three sections (A through C; see online Supplemental Materials for the complete study questionnaire). To gain a better understanding of the target population, the first section of the questionnaire (A) consisted of 11 questions (Q1-Q11) aimed at identifying the distribution of research participants by age, work experience, training received, FAA certification, industry segment, drone application, work environment, number of drone-related mishaps witnessed, and types of PPE used when operating a drone. Section B encompassed three questions (Q12-Q14) to obtain insight into the challenges of human-drone interactions. Section C (Q15-Q20) solicited data on the likelihood of occurrence of different situations, to measure opinions on compliance with occupational safety practices using a 5-point Likert scales.
Table 1. Survey questions.
2.3. Data Analysis
We obtained descriptive statistics and used two machine learning algorithms to analyze the survey responses; principal component analysis (PCA) and association rule mining (ARM). PCA is a widely used technique in survey analysis and was applied to Section C of the questionnaire to uncover underlying trends while also reducing the number of dimensions and retaining the best possible variability in the data (Chao et al., Citation2018; Cox & Cox, Citation1991). Specifically, PCA is a dimensionality reduction technique that extracts the linear combinations of primary variables called principal components, which can summarize latent constructs in the data. If not properly applied, this method may lead to misinterpretations and distorted results (Lever et al., Citation2017). In response to the concern noted above, Bartlett’s test of sphericity and the Kaiser-Meyer-Olkin (KMO) test of sampling adequacy were employed to determine the suitability of performing PCA (Rojas-Valverde et al., Citation2020). ARM was used as a knowledge discovery technique to identify significant relationships between distinct features in the data set, which are known as association rules (Young et al., Citation2011). ARM can detect frequent items that appear together in the data satisfying minimum support and confidence thresholds. An association rule is generally displayed as A→B, in which a set of items A is called the antecedent and another set of items B is referred to as the consequent. Support measures the percentage of records that contain both A and B. Similarly, confidence calculates how often a derived association rule holds true in a data set, hence measuring the rule’s accuracy (Larose & Larose, Citation2014). We used a deep ARM to determine which items that frequently occurred together could explain drone-related mishaps at work.
There is no consensus on the optimal choices of minimum support and confidence thresholds to ensure convergence to the strongest association rules as these choices are problem-specific (Coenen et al., Citation2005; Hikmawati et al., Citation2021). Certain important variables could be excluded from the association rules if the minimum threshold for support is placed at a higher value. For instance, to uncover fraudulent records in large sets of transactions, fraud detection analysts may have to decrease the minimum support to as low as 0.01 in order to avoid discarding any suspicious transactions (Larose & Larose, Citation2014). In the case of customer shopping behavior analysis, however, the minimum support may be set at a greater number to decrease computational complexity while retaining the most useful data. We determined the minimum support and confidence by exploring different combinations of thresholds for support and confidence, with the aim of identifying possible correlations between the variable under study, drone mishaps, and other variables of interest, such as following safety procedures and conducting hazard identification.
Next, the entire dataset was transformed into a binary matrix for use in the ARM algorithm (Lee et al., Citation2017). The number of drone-related mishaps was set as the target variable, which was defined as a close-ended question having five options, including “None,” “Between 1 & 5,” “Between 5 & 10,” “Between 10 & 15,” and “More than 15.” Independent variables were either defined as open-ended, 5-point Likert, or close-ended questions. Regarding the Likert questions, respondents could select either “Extremely unlikely,” “Unlikely,” “Unknown or neutral,” “Likely,” or “Extremely likely” (Vagias, Citation2006). Therefore, responses with the value of “Extremely unlikely,” “Unlikely,” or “Unknown or neutral” were labeled with a value of zero. Likewise, records with the value of “Likely” and “Extremely likely” were given a value of one. Regarding close-ended questions, if the participant checked the “None” option, the result was given a zero value; any other options that were selected were transformed into a value of one (Lee et al., Citation2017). Data analysis was performed in Python 3.10 using Pandas (1.4.3), NumPy (1.23.1), Sklearn (0.0), Mlxtend (0.20.0), Matplotlib (3.5.2), Plotly (5.10.0), and Scipy (1.8.1) libraries.
3. Results
3.1. Descriptive Analysis
Characteristics of study respondents are summarized in . Drone pilots, who were between 35 and 44 years of age, accounted for the largest share of participants. More than half of the participants had between one and five years of work experience as drone operators, and were FAA-certified drone pilots. Furthermore, most respondents reported receiving drone-related safety training. Services and construction constituted the two industry sectors with the largest number of participants. The main applications of drones were reported to be photography and site inspection. Regarding environmental settings in which drones are operated, most pilots utilized drones outdoors. Slightly more than half of respondents witnessed between one and five drone-related mishaps or near misses. Finally, safety vests were the most frequently used PPE when flying a drone, as reported by roughly half of the participants.
Table 2. Demographic and professional characteristics of research participants (n = 75). note that some questions asked respondents to select all that apply, so some response percentages may not add up to 100%.
Three questions were compiled in Section B of the survey to gather information regarding barriers to advances in human-drone collaboration. To this end, Q12 and Q13 were formulated to investigate the main reasons for drone-related mishaps from the perspective of the respondents, respectively caused by either human factors or drone failures. illustrates the expressed concerns by the pilots, along with their corresponding frequencies.
Figure 1. Sankey diagram of the causes of drone mishaps and their corresponding frequencies reported by the respondents, wherein the width of each link is proportional to the frequency of the cause of the reported mishap. Diagram created using SankeyMATIC.

It is apparent from that situational awareness, decision-based, and skill-based errors contribute to the majority of drone-related mishaps caused by human factors. Similarly, loss of GPS signal, mechanical failures, and battery failures are the top three root causes of mishaps reported by the participants that were classified as drone failures. By the same token, Q14 asks the respondents to indicate possible deficiencies in drone interface design that may cause distress and/or confusion. According to the pilots, non-intuitive menus (52%), poor visualization (48%), and lack of system feedback (47%) are the main design deficiencies of the human-drone interface. Participants’ responses to Q14 were classified into several categories, along with their most pertinent answers provided by the pilots (). These categories suggest three primary mechanisms through which the barriers against successful human-drone collaboration may operate, including communication, visibility and information presentation, and control modes.
Table 3. Human-drone interface issues as expressed by the pilots.
Section C in the survey was designed to elicit opinions regarding practices for workplace safety, and results are summarized in . Most respondents indicated a marked preference for outdoor flying with drones that are specifically designed to be flown in those areas (Q15). In response to Q17, concerning the use of protective indoor hulls, most respondents selected “Unknown or neutral,” suggesting their unfamiliarity with this notion. In fact, propeller guards and protective indoor hulls can be placed on the rotor blades of drones to minimize the risk of injury or damage in case of collision. Moreover, following safety procedures (Q18) as well as conducting hazard identification and risk assessment (Q20) were endorsed as “Extremely likely” by most respondents. Conversely, in response to Q22 most participants indicated that it would be “Unlikely” for them to be distracted by a nearby flying drone.
3.2. Principal Component Analysis
Regarding the suitability of PCA for its intended purpose, the Bartlett test yielded 46.37 with a p-value of . Since the p-value is less than alpha (0.05), there is sufficient evidence to conclude that a significant correlation is present among the variables with a 95% confidence level (Bartlett, Citation1950). Similarly, the KMO test returned a value of 0.63, which indicates that sampling was adequate, since the value was greater than 0.5 (Kaiser, Citation1974). Hence, these findings confirm the appropriateness of deploying PCA. The proper number of principal components should be calibrated by calculating the percentage of the variance explained by each component. The results of the explained variance ratio demonstrated that the first two components are indicative of 50% of the variations in the data. Thus, the first two components were adopted for further analysis.
depicts the PCA loadings of the first two components, in which each loading demonstrates the contribution of each primary variable to its principal component (Abdi & Williams, Citation2010). According to Guadagnoli and Velicer (Citation1988), a loading cutoff of 0.4 was applied, since factor loadings of over 0.4 are considered satisfactory and stable.
Figure 3. PCA loadings for corresponding primary variables. A: the first principal component. B: the second principal component. Dashed lines indicate loadings of 0.4.

From , it can be inferred that Q18 (likelihood of following safety procedures) and Q20 (likelihood of performing hazard identification and risk assessment before each flight) have the strongest effects on the first principal component. These two item loadings capture a recurring theme in respondents’ perceptions of safety, which can form a latent variable of safety compliance. suggests that Q16 (likelihood of using drones specifically designed to be flown indoors for indoor flights), Q19 (likelihood of reading any guidelines in the drone’s manual before each flight), and Q21 (likelihood of gaining management support when a safety concern is raised) are highly correlated with the second principal component. These item loadings can mirror a common behavioral construct in respondents’ attitude to safety, which can be classified as safety participation. These three items underline the willingness of the respondents to improve workplace safety practices. The first two components reduce the overall dimensionality of data by transforming it from a high dimensional feature space (eight questions) to a low dimensional feature space with only two factors (safety compliance and safety participation). Caution is required in interpreting these results, though, since only half of the variance is observed in the first two components.
3.3. Association Rule Mining
ARM was employed to find the critical variables that most influence the likelihood of drone-related mishaps occurring. Configuring the minimum support at 0.2 and confidence at 0.5 yielded 1,328 association rules. displays some of the possible results of ARM.
Table 4. Association rule mining results.
Rule 1 states that drone operators who answered “Extremely likely” on questions regarding the likelihood of following safety procedures and conducting risk assessment, as well as the likelihood of flying drones outdoors, would have between one and five drone-related mishaps at work. In addition, people who received training, flying drones outdoors, and answered “Extremely likely” on the question about the likelihood of conducting risk assessment would witness between one and five drone mishaps, as explained by Rule 2. In the similar way, according to Rule 3, people who answered “Extremely likely” on questions about the likelihood of following safety procedures and using drones specifically designed to be flown outdoors, as well as flying those drones outside, would report having one to five drone-related mishaps. Rule 4 shows that pilots who answered “Extremely likely” on questions about the likelihood of following safety procedures and using drones specifically designed to be flown outdoors, as well as having management support when safety concerns arise, would answer “Extremely likely” on the question about the likelihood of conducting risk assessment before each flight. Similarly, participants who selected “Extremely likely” for the question about the likelihood of conducting risk assessment, having management support, and flying drones outdoors, would select “Extremely likely” for the question about the likelihood of following safety procedures, as shown in Rule 5. Rules 6 -8 explain that drone pilots who reported not having any drone-related mishaps at their workplaces were associated with answering “Extremely likely” on the question about the likelihood of following safety procedures, flying drones outdoors, and reporting that they had received commercial drone training. From Rules 9 and 10, we can conclude that drone pilots who were between the ages of 25 and 34, received training, and had between one and five years of work experience would report witnessing between one and five drone-related mishaps at work.
4. Discussion
Despite the obvious benefits of using UAVs, the rapid proliferation of drones in diverse work environments poses social and ethical dilemmas centered around invasion of individual privacy and endangering public safety. Moreover, drones can present a number of safety risks to pilots, since they work in the vicinity of UAVs. Several studies have addressed technoethics challenges as a result of the widespread use of UAVs. Nonetheless, the occupational safety hazards to drone pilots caused by UAV operations remain unclear in the literature and merit special attention.
Researchers have a divided view on what causes drone mishaps in the nascent literature. Research has confirmed that UAV technical issues, such as mechanical and electrical component failures, as well as software and flight control system malfunctions, play a major role in causing drone mishaps in the workplace. On the other end of the spectrum, there is speculation that human factors, such as inadequate organizational training of drone operators, overconfidence of pilots, high workloads, and low attentiveness, may contribute to drone mishaps. Moreover, to improve workplace safety, it is crucial to take environmental and weather factors into account. In order to fully unlock the potential of UAVs in various work settings, existing safety rules and standards should be comprehensively revisited. In fact, the regulatory landscape surrounding the use of drones requires a constant state of evolution to keep step with rapid advancements in industrial automation and robotics. Remarkably, pilot training to address skills mismatches can lead to a lower risk of mishap involving drones.
This study was undertaken to elucidate possible challenges that hinder effective human-drone collaboration and analyze whether drone pilots demonstrate a commitment to workplace safety via data collection. Our findings indicate that human factors, rather than flaws in technology, were considered by pilots as the source for a majority of drone mishaps. Particularly, situational awareness, decision-based, and skill-based errors were the leading causes of human factors-related mishaps, whereas GPS signal loss, mechanical malfunction, and battery failures accounted for the three most common causes of drone mishaps attributed to technical issues. Furthermore, poor communication, information displays, and control modes were found to be the three primary drone interface deficiencies that impede effective human-drone collaborations. Two key themes—safety compliance and safety participation—emerged from the findings of the PCA, implying the participants’ inclination to adopt safety practices in the workplace. Additionally, the results of ARM demonstrate that following safety procedures, receiving technical training, and flying outdoors could be linked to a reduced likelihood of mishaps occurring. These results broadly corroborate the findings of earlier studies in this area that relate organizational factors, safety compliance, safety participation, and training with drone mishaps (Arrabito et al., Citation2021; Lamb, Citation2017).
The generalizability and transferability of our findings are bound by certain limitations. The major limitation of this study is the nature of the self-administered questionnaire, which makes it prone to bias and could have negatively affected the quality of the obtained data. Moreover, our response rate and acceptance of valid responses were low, which unfortunately may have introduced bias and limited the representativeness and generalizability of our results. Underreporting workplace mishaps could be another source of weakness in this research. According to data available, a substantial percentage of UAV mishaps go unreported (Kasprzyk & Konert, Citation2021). Hence, it is critical to raise awareness among drone pilots about the necessity of reporting mishaps to the regulatory authorities. Although the current study is based on a small sample of participants, our findings can be used to inform the development of safety protocols, guidelines, and training for drone operations, as well as safety-oriented drone interface designs.
Whether self-inflicted or caused by others, drone-related mishaps have become common occurrences in modern industry. These mishaps can erode public trust in drones, which is essential for effective human-drone collaboration. There is a consensus that a higher level of performance and safety could be achieved with trust in automation established by human workers, which in turn increases the willingness to adopt emerging technologies (Andrews et al., Citation2023; French et al., Citation2018). Equally important is readjusting human trust in automation, since overtrust and overreliance may endanger individuals and obscure risks associated with drone use.
Supplemental Material
Download PDF (82.4 KB)Acknowledgments
The authors thank Darrell Binnion, the collaborator of this study, for his scholarly contributions. Additionally, the authors recognize Dr. Tao Yuan from the Ohio University Department of Industrial and Systems Engineering for his kind assistance in IRB preparation and approval. Moreover, the authors thank Brad Weckman for his insightful comments. Finally, the authors thank Dr. Dale Masel from the Ohio State University Department of Engineering Education for his help with funding.
Disclosure statement
No potential conflict of interest was reported by the author(s).
Correction Statement
This article has been corrected with minor changes. These changes do not impact the academic content of the article.
Additional information
Funding
References
- Abdi, H., & Williams, L. J. (2010). Principal component analysis. Wiley Interdisciplinary Reviews: Computational Statistics, 2(4), 433–459.https://doi.org/10.1002/wics.101
- Alwateer, M., Loke, S. W., & Zuchowicz, A. M. (2019). Drone services: Issues in drones for location-based services from human-drone interaction to information processing. Journal of Location Based Services, 13(2), 94–127.https://doi.org/10.1080/17489725.2018.1564845
- American Society of Safety Professionals. (n.d). The ASSP Community. https://community.assp.org/home
- Andrews, R. W., Lilly, J. M., Srivastava, D., & Feigh, K. M. (2023). The role of shared mental models in human-AI teams: A theoretical review. Theoretical Issues in Ergonomics Science, 24(2), 129–175.https://doi.org/10.1080/1463922X.2022.2061080
- Antunano, M. J. (2005). Spatial disorientation (Report No. AM-400 03/1). Federal Aviation Administration. https://rosap.ntl.bts.gov/view/dot/39656
- Arnold, A. E. G. F., Burles, F., Krivoruchko, T., Liu, I., Rey, C. D., Levy, R. M., & Iaria, G. (2013). Cognitive mapping in humans and its relationship to other orientation skills. Experimental Brain Research, 224(3), 359–372. https://doi.org/10.1007/s00221-012-3316-0
- Arrabito, G. R., Ho, G., Lambert, A., Rutley, M., Keillor, J., Chiu, A., Au, H., & Hou, M. (2010). Human factors issues for controlling uninhabited aerial vehicles: Preliminary findings in support of the Canadian forces joint unmanned aerial vehicle surveillance target acquisition system (Tech. Rep. No. TR-2009-043). Defense Research and Development Canada. https://apps.dtic.mil/sti/pdfs/ADA543186.pdf
- Arrabito, G. R., Hou, M., Banbury, S., Martin, B. C. W., Ahmad, F., & Fang, S. (2021). A review of human factors research performed from 2014 to 2017 in support of the Royal Canadian Air Force remotely piloted aircraft system project. Journal of Unmanned Vehicle Systems, 9(1), 1–20.https://doi.org/10.1139/juvs-2020-0012
- Arterburn, D. R., Duling, C. T., & Goli, N. R. (2017). Ground collision severity standards for UAS operating in the National Airspace System (NAS) [Paper presentation]. 17th AIAA Aviation Technology, Integration, and Operations Conference, Denver, CO, United States. https://doi.org/10.2514/6.2017-3778
- Bais, F., Schouten, B., & Toepoel, V. (2020). Investigating response patterns across surveys: Do respondents show consistency in undesirable answer behaviour over multiple surveys? Bulletin of Sociological Methodology/Bulletin de Méthodologie Sociologique, 147-148(1-2), 150–168.https://doi.org/10.1177/0759106320939891
- Bartlett, M. S. (1950). Tests of significance in factor analysis. British Journal of Statistical Psychology, 3(2), 77–85. https://doi.org/10.1111/j.2044-8317.1950.tb00285.x
- Bernauw, K. (2016). Drones: The emerging era of unmanned civil aviation. Zbornik Pravnog Fakulteta u Zagrebu, 66(2-3), 223–248. https://hrcak.srce.hr/157605
- Campolettano, E. T., Bland, M. L., Gellner, R. A., Sproule, D. W., Rowson, B., Tyson, A. M., Duma, S. M., & Rowson, S. (2017). Ranges of injury risk associated with impact from unmanned aircraft systems. Annals of Biomedical Engineering, 45(12), 2733–2741. https://doi.org/10.1007/s10439-017-1921-6
- Cardosi, K., & Lennerts, T. (2017). Human factors considerations for the integration of unmanned aerial vehicles in the National Airspace System: An analysis of reports submitted to the Aviation Safety Reporting System (ASRS). (Report No. DOT/FAA/TC-17/25). U.S. Department of Transportation. https://rosap.ntl.bts.gov/view/dot/12500
- Chao, Y.-S., Wu, H.-C., Wu, C.-J., & Chen, W.-C. (2018). Principal component approximation and interpretation in health survey and biobank data. Frontiers in Digital Humanities, 5, 11.https://doi.org/10.3389/fdigh.2018.00011
- Choi, H. H. (2021). Delivery drones: Inapt for application of current negligence theory. Journal of Air Law and Commerce, 86(3), 435–466. https://scholar.smu.edu/jalc/vol86/iss3/11
- Coenen, F., Leng, P., & Zhang, L. (2005). Threshold tuning for improved classification association rule mining. In T. B. Ho, D. Cheung, & H. Liu (Eds.), Advances in knowledge discovery and data mining (pp. 216–225). Springer. https://doi.org/10.1007/11430919_27
- CommercialDronePilots. (n.d). Commercial drone forums. https://commercialdronepilots.com/
- Cox, S., & Cox, T. (1991). The structure of employee attitudes to safety: A European example. Work & Stress, 5(2), 93–106.https://doi.org/10.1080/02678379108257007
- De Croon, G., & De Wagter, C. (2018). Challenges of autonomous flight in indoor environments [Paper presentation]. 2018 IEEE/RSJ International Conference on Intelligent Robots and Systems, Madrid, Spain. https://ieeexplore.ieee.org/document/8593704
- Fernando, A. V. (2017). Survey of sUAS unintended flight termination as depicted in internet video. Journal of Unmanned Vehicle Systems, 5(3), 109–114.https://doi.org/10.1139/juvs-2017-0007
- French, B., Duenser, A., & Heathcote, A. (2018). Trust in automation: A literature review. (Report No. EP184082). Commonwealth Scientific and Industrial Research Organisation (CSIRO).
- Gao, M., Hugenholtz, C. H., Fox, T. A., Kucharczyk, M., Barchyn, T. E., & Nesbit, P. R. (2021). Weather constraints on global drone flyability. Scientific Reports, 11(1), 12092.https://doi.org/10.1038/s41598-021-91325-w
- Griffin, M. A., & Hu, X. (2013). How leaders differentially motivate safety compliance and safety participation: The role of monitoring, inspiring, and learning. Safety Science, 60, 196–202.https://doi.org/10.1016/j.ssci.2013.07.019
- Griffin, M. A., & Neal, A. (2000). Perceptions of safety at work: A framework for linking safety climate to safety performance, knowledge, and motivation. Journal of Occupational Health Psychology, 5(3), 347–358. https://doi.org/10.1037/1076-8998.5.3.347
- Guadagnoli, E., & Velicer, W. F. (1988). Relation of sample size to the stability of component patterns. Psychological Bulletin, 103(2), 265–275. https://doi.org/10.1037/0033-2909.103.2.265
- Helmreich, R. L. (1999). Building safety on the three cultures of aviation. In Proceedings of the IATA Human Factors Seminar (pp. 39–43).
- Hikmawati, E., Maulidevi, N. U., & Surendro, K. (2021). Minimum threshold determination method based on dataset characteristics in association rule mining. Journal of Big Data, 8(1), 146. https://doi.org/10.1186/s40537-021-00538-3
- Hobbs, A., & Lyall, B. (2016). Human factors guidelines for remotely piloted aircraft system (RPAS) remote pilot stations (RPS). (Report No. ARC-E-DAA-TN34128). National Aeronautics and Space Administration. https://ntrs.nasa.gov/api/citations/20190000211/downloads/20190000211.pdf
- Hoem, Å. S., Johnsen, S. O., Fjørtoft, K., Rødseth, Ø. J., Jenssen, G., & Moen, T. (2021). Improving safety by learning from automation in transport systems with a focus on sensemaking and meaningful human control. In S. O. Johnsen & T. Porathe (Eds.), Sensemaking in safety critical and complex situations human factors and design (pp. 191–207). CRC Press. https://doi.org/10.1201/9781003003816
- Howard, J., Murashov, V., & Branche, C. M. (2018). Unmanned aerial vehicles in construction and worker safety. American Journal of Industrial Medicine, 61(1), 3–10. https://doi.org/10.1002/ajim.22782
- Irimia, A., Gaman, G. A., Pupazan, D., Ilie, C., & Nicolescu, C. (2019). Using drones in support of rescue interventions teams in toxic/flammable/explosive environments. Environmental Engineering and Management Journal, 18(4), 831–837. http://www.eemj.icpm.tuiasi.ro/pdfs/vol18/full/no4/8_285_Irimia_18.pdf https://doi.org/10.30638/eemj.2019.079
- Kaiser, H. F. (1974). An index of factorial simplicity. Psychometrika, 39(1), 31–36. https://doi.org/10.1007/BF02291575
- Karagiannis, P., Togias, T., Michalos, G., & Makris, S. (2021). Operators training using simulation and VR technology. Procedia CIRP, 96, 290–294.https://doi.org/10.1016/j.procir.2021.01.089
- Kasprzyk, P. J., & Konert, A. (2021). Reporting and investigation of Unmanned Aircraft Systems (UAS) accidents and serious incidents. Regulatory perspective. Journal of Intelligent & Robotic Systems, 103(1), 3.https://doi.org/10.1007/s10846-021-01447-6
- Khosiawan, Y., & Nielsen, I. (2016). A system of UAV application in indoor environment. Production & Manufacturing Research, 4(1), 2–22.https://doi.org/10.1080/21693277.2016.1195304
- Koerner, M. R. (2015). Drones and the Fourth Amendment: Redefining expectations of privacy. Duke Law Journal, 64(3), 1129–1172. http://scholarship.law.duke.edu/dlj/vol64/iss6/3
- Lamb, T. (2017). Developing a safety culture for remotely piloted aircraft systems operations: To boldly go where no drone has gone before [Paper presentation].SPE Health, Safety, Security, Environment, & Social Responsibility Conference - North America, New Orleans, LA, United States.https://doi.org/10.2118/184476-MS
- Lamb, T. (2019). The changing face of airmanship and safety culture operating unmanned aircraft systems. In T. Kille, P. Bates, & S. Lee (Eds.), Unmanned aerial vehicles in civilian logistics and supply chain management (pp. 243–265). IGI Global. https://doi.org/10.4018/978-1-5225-7900-7.ch009
- Larose, D. T., & Larose, C. D. (2014). Association rules. In D. T. Larose (Ed.), Discovering knowledge in data: An introduction to data mining (pp. 247–264). Wiley.
- Lawlor, J., Thomas, C., Guhin, A. T., Kenyon, K., Lerner, M. D., & Drahota, A, UCAS Consortium. (2021). Suspicious and fraudulent online survey participation: Introducing the REAL framework. Methodological Innovations, 14(3), 205979912110504.https://doi.org/10.1177/20597991211050467
- Lee, Y.-C., Huang, C.-H., Lin, Y.-C., & Wu, H.-H. (2017). Association rule mining to identify critical demographic variables influencing the degree of burnout in a regional teaching hospital. TEM Journal, 6(3), 497–502. https://www.temjournal.com/content/63/TemJournalAugust2017_497_502.pdf
- Lever, J., Krzywinski, M., & Altman, N. (2017). Principal component analysis. Nature Methods, 14(7), 641–642. https://doi.org/10.1038/nmeth.4346
- Li, S., Cummings, M. L., & Welton, B. (2022). Assessing the impact of autonomy and overconfidence in UAV first-person view training. Applied Ergonomics, 98, 103580. https://doi.org/10.1016/j.apergo.2021.103580
- Luppicini, R., & So, A. (2016). A technoethical review of commercial drone use in the context of governance, ethics, and privacy. Technology in Society, 46, 109–119.https://doi.org/10.1016/j.techsoc.2016.03.003
- Meade, A. W., & Craig, S. B. (2012). Identifying careless responses in survey data. Psychological Methods, 17(3), 437–455. https://doi.org/10.1037/a0028085
- Mukhamediev, R. I., Symagulov, A., Kuchin, Y., Zaitseva, E., Bekbotayeva, A., Yakunin, K., Assanov, I., Levashenko, V., Popova, Y., Akzhalova, A., Bastaubayeva, S., & Tabynbaeva, L. (2021). Review of some applications of unmanned aerial vehicles technology in the resource-rich country. Applied Sciences, 11(21), 10171.https://doi.org/10.3390/app112110171
- Namian, M., Khalid, M., Wang, G., & Turkan, Y. (2021). Revealing safety risks of unmanned aerial vehicles in construction. Transportation Research Record: Journal of the Transportation Research Board, 2675(11), 334–347.https://doi.org/10.1177/03611981211017134
- Occupational Safety and Health Administration (OSHA). (2018). OSHA’s use of unmanned aircraft systems in inspections. OSHA Archive, U.S. Department of Labor.
- ECFR (2016). Part 107- Small Unmanned Aircraft Systems, 14 C.F.R. § 107. https://www.ecfr.gov/current/title-14/chapter-I/subchapter-F/part-107
- Princeton University. (n.d). Indoor Safety Guidelines. https://drones.princeton.edu/sites/g/files/toruqf1701/files/indoor_safety_guidelines.pdf
- Qualtrics. (n.d). Qualtrics XM: The leading experience management software. https://www.qualtrics.com/
- Rahmani, H. (2019). Traveling salesman problem with single truck and multiple drones for delivery purposes. [Master’s thesis]. Ohio University. OhioLINK Electronic Theses and Dissertations Center. http://rave.ohiolink.edu/etdc/view?acc_num=ohiou1563894245160348
- Rojas-Valverde, D., Pino-Ortega, J., Gómez-Carmona, C. D., & Rico-González, M. (2020). A systematic review of methods and criteria standard proposal for the use of principal component analysis in team’s sports science. International Journal of Environmental Research and Public Health, 17(23), 8712. https://doi.org/10.3390/ijerph17238712
- SkydioPilots. (n.d). General Forums. https://skydiopilots.com/
- Smith, A. N. (2019). Finite element analysis of traumatic brain injury due to small unmanned aircraft system impacts on the human head. [Master’s thesis]. Mississippi State University. Scholars Junction. https://scholarsjunction.msstate.edu/td/2286
- Smith, A., Dickinson, J. E., Marsden, G., Cherrett, T., Oakey, A., & Grote, M. (2022). Public acceptance of the use of drones for logistics: The state of play and moving towards more informed debate. Technology in Society, 68, 101883.https://doi.org/10.1016/j.techsoc.2022.101883
- State of Minnesota Department of Transportation, Office of Aeronautics. (2023). Commercial operators listing [Data set]. Minnesota Department of Transportation. https://www.dot.state.mn.us/aero/documents/aviationbusinesses/unmannedaerialvehicle.pdf
- The American National Standards Institute (ANSI). (2018). ANSI standardization roadmap for unmanned aircraft systems published. https://www.ansi.org/news/standards-news/all-news/2018/12/ansi-standardization-roadmap-for-unmanned-aircraft-systems-published-20
- The National Institute for Occupational Safety and Health (NIOSH). (n.d). Robotics. https://www.cdc.gov/niosh/topics/robotics/aboutthecenter.html
- Tran, T.-H., & Nguyen, D.-D. (2022). Management and regulation of drone operation in urban environment: A case study. Social Sciences, 11(10), 474. https://doi.org/10.3390/socsci11100474
- Tvaryanas, A. P., Thompson, W. T., & Constable, S. H. (2005). U.S. military unmanned aerial vehicle mishaps: Assessment of the role of human factors using Human Factors Analysis and Classification System (HFACS). (Report No. HSW-PE-BR-TR-2005-0001). United States Air Force 311th Human Systems Wing.
- Vacek, J. (2018). Threatening drones: How to answer when your client asks: "Can i shoot down that drone? Aviation Faculty Publications. https://commons.und.edu/avi-fac/51
- Vagias, W. M. (2006). Likert-type scale response anchors. Clemson International Institute for Tourism & Research Development, Department of Parks, Recreation and Tourism Management. Clemson University.
- Foreman, V. L., Favaró, F. M., Saleh, J. H., & Johnson, C. W. (2015). Software in military aviation and drone mishaps: Analysis and recommendations for the investigation process. Reliability Engineering & System Safety, 137, 101–111.https://doi.org/10.1016/j.ress.2015.01.006
- Wild, G., Murray, J., & Baxter, G. (2016). Exploring civil drone accidents and incidents to help prevent potential air disasters. Aerospace, 3(3), 22.https://doi.org/10.3390/aerospace3030022
- Williams, K. W. (2004). A summary of unmanned aircraft accident/incident data: Human factors implications. (Report No. DOT/FAA/AM-04/24). Federal Aviation Administration. https://apps.dtic.mil/sti/pdfs/ADA460102.pdf
- Xu, Y., & Turkan, Y. (2022). The development of a safety assessment model for using unmanned aerial systems (UAS) in construction. Safety Science, 155, 105893.https://doi.org/10.1016/j.ssci.2022.105893
- Young, W., Weckman, G., & Holland, W. (2011). A survey of methodologies for the treatment of missing values within datasets: Limitations and benefits. Theoretical Issues in Ergonomics Science, 12(1), 15–43.https://doi.org/10.1080/14639220903470205
- Zhen, L., Gao, J., Tan, Z., Wang, S., & Baldacci, R. (2023). Branch-price-and cut for trucks and drones cooperative delivery. IISE Transactions, 55(3), 271–287. https://doi.org/10.1080/24725854.2022.2060535
- Zwickle, A., Farber, H. B., & Hamm, J. A. (2019). Comparing public concern and support for drone regulation to the current legal framework. Behavioral Sciences & the Law, 37(1), 109–124. https://doi.org/10.1002/bsl.2357