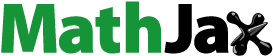
OCCUPATIONAL APPLICATIONS
Fatigue, and many other human performance factors, impact worker wellbeing, and thus production quality and efficiency. Adopting the Industry 5.0 perspective, we propose that integrating human performance models into wider industrial system models can improve modeling accuracy and lead to superior outcomes. Integrating our Worker Fatigue Model as part of their industrial system architect model allowed Airbus, a leading aircraft manufacturer, to more accurately predict system performance as a function of the workforce makeup, which could be a combination of human workers and robots, or a combination of highly experienced and less experienced workers. Our approach demonstrates the importance and value of including human performance models in trade studies for introducing robots on the shop floor, and can be used to include various aspects of human performance in industrial system models to address specific task requirements or different levels of automation.
TECHNICAL ABSTRACT
Rationale
The advent of Industry 5.0 places a heightened focus on enhancing worker wellbeing during the digital transformation of factories. System models that ignore human workers yield suboptimal results in product design and system improvement.
Purpose
In the aircraft industry, worker workload is of primary concern as most tasks are performed manually, leading to general fatigue and musculoskeletal disorders. Robot assistance could improve quality, efficiency and relieve workers from fatigue. To demonstrate the feasibility and value of integrating human performance models in system design at Airbus, a Worker Fatigue Model was developed, focusing on the effects of (1) automation (manual vs semi-automated), and (2) workforce makeup (various ratios of high-skilled to low-skilled workers). Our ultimate goal was to inform the development of effective policies and strategies for human-technology integration in Industry 5.0.
Methods
We developed the Worker Fatigue Model by adapting existing fatigue models for workers in industrial environments and by considering worker characteristics, tasks, and the presence or absence of robot-assistance. Two different scenarios were simulated (fully manual and semi-automated), with input variables such as worker skill, age, and motivation, and output variables including overall fatigue and error probabilities were evaluated. The Worker Fatigue Model was integrated into the Airbus system model to conduct trade studies based on workforce characteristics.
Results
Our findings revealed that the composition of the workforce (i.e., various ratios of high-skilled to low-skilled workers), alongside specific manufacturing technologies, significantly reduced worker fatigue, especially with higher ratios of high-skilled workers, and improved overall industrial system performance.
Conclusions
Although applying our Worker Fatigue Model effectively demonstrated the feasibility and value of integrating human factors into early industrial system design, it remains a work in progress. Future work will aim to accurately represent the workload of human workers, including operational costs, when implementing robot assistance.
1. Introduction
1.1. Background
In the current industrial digital transformation, also known as Industry 4.0, the integration of advanced technologies, such as automation, artificial intelligence, and the internet of things, is promising for enhancing product quality, system efficiency, and safety. The transformation of manufacturing and production processes alters the nature of the work. Workers find themselves at a crossroads—either adapt to the new technological environment by acquiring new skills or face displacement. The repercussions of this shift on workers’ roles, task structures, and overall wellbeing remain unknown. As highlighted by the European Commission (Breque et al., Citation2021), public discourse indicates a growing sense of redundancy, incompetence, and isolation among human workers in Industry 4.0.
Complementing Industry 4.0, the emerging framework of Industry 5.0 is directing focus on the symbiotic relationship between human workers and technological advancements, aiming for a more sustainable, resilient, and human-centric industry. For companies such as Airbus, efficiency, resilience, and adherence to the highest safety and sustainability standards are non-negotiable. Airbus has long recognized the significance of human factors in achieving optimal performance levels in its production facilities, and in the seamless integration of new manufacturing technologies, notably higher degrees of automation. Recent research activities (e.g., Helle et al., Citation2022) have included human performance modeling and simulation, with an emphasis on issues related to worker fatigue, and errors or accidents that can impact production quality and efficiency.
Model-driven design of processes or systems (i.e., model-based system engineering) has become a major trend in the manufacturing industry (e.g., Arista et al., Citation2023; Helle et al., Citation2019). In designing new production lines, incorporating system models that encompass human operator characteristics is anticipated to yield more accurate predictions of non-conformities (i.e., number of defects from a production step) and outstanding work (i.e., additional work due to correction of defects) for a production system operated by a specific workforce (Nardo et al., Citation2020). This approach also facilitates the evaluation of a production system’s adaptability to changes or disruptions, by assessing the impact of workforce variations (ratio of experienced-to-inexperienced workers) and the introduction of new manufacturing technologies on system performance (e.g., automation). Studies on demographic change predict a significant increase in the age and related attributes of the aircraft manufacturing worker population (e.g., Fuchs et al., Citation2016), emphasizing the growing need to consider worker characteristics in Industry 5.0.
In this paper, we describe a project conducted at Airbus to demonstrate the feasibility and efficacy of integrating human factors into the design of new production processes, particularly those involving automation or robots. Preliminary results were previously reported in a case study (Allemang–Trivalle et al., Citation2023). The current paper substantively expands on this earlier report by detailing the fatigue model, the simulation results comparing manual and robot-assisted scenarios in the manufacturing process, and the implementation by the industry partner (Airbus).
Our overarching objective was to advocate for the inclusion of human factors and ergonomics as a cornerstone in the company’s future system and product design endeavors, positioning Airbus as a pioneer in the Industry 5.0 revolution. The insights gleaned from Airbus’s approach were expected to provide a valuable framework for industries across the spectrum, aiding in the development of systems and processes that are not only technologically advanced but also human-centric.
1.2. Relevant Literature
A plethora of studies has delved into understanding and modeling the impact of various shop floor parameters on worker performance (e.g., Katiraee et al., Citation2021; Kolus et al., Citation2018). The literature identifies a multitude of risk factors that affect workers’ health and wellbeing. These factors are broadly categorized into four main domains: Individual, Task-related, Process-related, and Environmental. A comprehensive review by Kolus et al. (Citation2018) examined the intricate relationships between these risk factors and their subsequent effects on variables such as worker fatigue, occurrence of errors, and quality of production. Notably, their review sheds light on the pivotal role of process design, which constitutes 37% of the impact, followed by task design, which accounts for 27%, in influencing the overall production quality. Additionally, using fuzzy extent analysis, Abubakar and Wang (Citation2019) offers a nuanced perspective by ranking various individual factors based on their contribution to human-centered performance. Their analysis revealed that experience is the most significant factor, contributing 34% of the effect, followed by age (26%), and general cognitive abilities (18%). Furthermore, physical work capacity, learning, and forgetting each contributed 7% of the impact, whereas reaction time and job satisfaction were each identified as having a 4% influence. This comprehensive exploration of risk factors and their impacts forms a foundation for understanding the multifaceted dynamics that influence worker performance and wellbeing.
Several methodologies have been employed to model worker performance within the industrial assembly process, such as digital human modeling (Alexander & Fromm, Citation2019), human performance modeling (Fritzsche et al., Citation2011), human error modeling (Elmaraghy et al., Citation2008), and fuzzy logic application (Guiffrida & Nagi, Citation1998). Digital human modeling focuses on the anthropometric and biomechanical characteristics of the worker, creating a detailed virtual body model to evaluate risks such as musculoskeletal disorders associated with tasks. However, a significant limitation of this approach is its failure to provide a complete model of the entire factory, particularly regarding the diverse effects beyond biomechanics. Similarly, human performance modeling, focusing on cognitive aspects such as perception, attention, and memory, offers insights into error probabilities and task completion times, but often necessitates extensive psychological analysis, rendering it less adaptable to holistic factory settings.
In response to such limitations, simplified human effect models have emerged, focusing on learning-forgetting and fatigue-recovery based on various tasks (e.g., repetitiveness, complexity, expected duration) and worker characteristics (e.g., experience, age), which are instrumental in optimizing job rotation (Jaber et al., Citation2013). Fuzzy logic has also been proposed to address the complexity and imprecision inherent in human decision-making. However, it requires abundant data (Abubakar & Wang, Citation2019; van Laarhoven & Pedrycz, Citation1983).
1.3. Objectives
While the existing literature provides a foundational understanding of the diverse factors impacting worker performance and how to model them, it also unveils gaps in offering a holistic and integrated model that is adaptable to entire factory settings. Our project aimed to narrow this gap by: (1) developing a model that balances the physical and cognitive dimensions of worker performance within industrial settings; and (2) demonstrating the feasibility and added value of integrating human fatigue models into the existing Airbus system modeling framework. The innovation of our approach is to formulate an adapted fatigue model based on previously validated models for a real-world scenario in an industrial environment. Our demonstration of the feasibility and value of this approach at Airbus provides evidence to promote the implementation of human factors at scale in future system and product design.
2. Methods
For this study, the Orbital Joint Assembly process was selected as a use case to demonstrate the added value of human factors in the design of assembly processes. This process involves the connection of two aircraft sections and comprises approximately 1,200 riveted joints along a circular connecting line. Designed in the 1980s, these aircraft structures were assembled manually, requiring workers to adopt ergonomically poor postures while riveting the circular Orbital Joint. However, this process cannot be easily automated. Airbus has studied partial automation concepts using industrial robots (Kuka, Light Flextrack robots) at various production sites. These automation solutions, though, still require manual intervention for setup and in-process monitoring. Notably, approximately 10% of the assembly tasks can only be performed manually, due to the presence of obstacles that prevent the use of robots.
2.1. Observations and Domain Expert Interviews
Field observations and interviews were conducted at three different Airbus plants: Nantes, France; Saint-Nazaire, France; and Hamburg, Germany. Adhering to the stipulations of law n°2012–300 (dated March 5, 2012) and its implementing decree n°2017–884 (dated May 9, 2017) that govern non-interventional clinical research in France, this study was conducted without requiring written informed consent from the participants or authorization from an ethics committee; however, it was still reviewed and authorized by Airbus management.
Semi-structured interviews were conducted at each Airbus location with key stakeholders:
Mechanical Engineers: Two engineers responsible for enhancing the existing manufacturing processes, which encompass tasks such as tool development and process modifications.
Manufacturing Engineers: One engineer in charge of automating current processes, including the integration of robots and sensors.
Ergonomists: Two ergonomists responsible for task assessment and ensuring operator safety.
Health and Safety Physicians: Two physicians responsible for treating worker injuries and ensuring wellbeing.
In addition, three all-day workshops with stakeholders and production plant tours provided a comprehensive understanding of the various perspectives. The interviews were designed to gain insights into the various facets of the production process and workforce training. At least two research team members were present during each interview: one was responsible for taking notes, while the other conducted the interview.
Additionally, line managers furnished activity distribution programs that included detailed work procedures, task descriptions, time and tools required, worker-task assignments, team compositions, and shift durations for specific production lines. Each task was evaluated through the Ergonomie Merkmal Methode Airbus (EMMA), an internal ergonomic assessment tool at Airbus (Brombach et al., Citation2019). These assessments provided data on the risk of injuries based on the postures, physical loads, and environmental conditions encountered by a worker during task execution. Furthermore, company ergonomists and physicians supplied samples of de-identified worker ergonomic assessment records from one production line in the Orbital Joint Assembly Process.
2.2. Worker Fatigue Model – Development
During the interviews a consensus emerged among the stakeholders that worker fatigue represented a major concern. From the knowledge obtained in the observations, interviews, and process descriptions, and by leveraging of the relevant literature on fatigue generally and in the industrial context, a model of fatigue for the application (Orbital Joint Assembly Process) was defined. This new model of worker fatigue was developed by integrating and customizing components of existing models to fit our real-world application.
Data related to worker performance (time to complete a task) and quality of work (number and type of defects) are considered very sensitive and highly protected in Germany. Increased production demands during the ramp-up following the COVID slowdown also made it difficult to recruit workers to participate in studies. The availability of Airbus facilities for experiments, or the collection of physiological data from workers to analyze the stress induced by a specific task, was severely limited. Therefore, the model and simulations were built on a small set of work orders and the sample worker/task ergonomic rating data provided by Airbus.
Numerous tasks and worker characteristics have been shown in the literature to impact the overall workforce workload/fatigue and system output (Kolus et al., Citation2018). For the purpose of demonstration, and to allow for comparison of manual work versus robotic assistance, three classes of factors were included in our Worker Fatigue Model to provide a global assessment of fatigue: physical, cognitive, and perceptual factors. Each task within the Orbital Joint Assembly Process was characterized by these three factors. Some tasks are primarily physical whereas others are more cognitive or perceptual.
Despite the many fatigue models in the literature, it was necessary to adapt selected components of existing models to fit a specific application. Existing models are either too focused on specific aspects of a task or not transferable from one system to another, and therefore are not suitable for modeling fatigue in the Airbus industrial system, where many different tasks and workers are involved in a particular production line at any given time. lists the formulae adapted for the Worker Fatigue Model. Workers are modeled as having different workloads based on nine individual characteristics. This approach aligns with expected demographic changes, such as an increase in the average age of the aircraft manufacturing workforce (Fuchs et al., Citation2016).
Table 1. Formulae for Worker Fatigue Model.
The human fatigue and recovery model by Jaber et al. (Citation2013) was adapted to allow the possibility of adding robots to the work process as well as additional parameters related to workers and task design, as determined based on subject matter expert interviews (see Section 3.1). Specifically, the constant fatigue exponent of the original formula, , was replaced with the parametric formula in Equation (2). This parametric formula includes the physical (
), cognitive (
), and perceptual (
) dimensions of the tasks, and the associated resilience of the workers:
,
and
. For a given task, the rate of fatigue increase is slower among workers who have higher resilience levels, as opposed to those with lesser resilience. In parallel, tasks that are less demanding will cause a slower buildup of fatigue in comparison to more demanding ones.
In this context, resilience represents the capacity of a worker to navigate and adapt to different constraints related to the three dimensions of tasks. This deliberate alignment and comparison between task and worker resilience dimensions serves as the foundational structure of our model, aiming to provide a comprehensive representation of worker performance amidst varying constraints. Resilience is a composite measure derived from several worker characteristics, such as skill level, experience, and motivation (see Equations (3)–(6)). These equations are designed to be generic, to be easily adapted to different contexts. The model distinguishes between resources associated with the physical (strength and endurance), cognitive (decision-making and information processing), and perceptual (perception and detection) aspects of tasks (see ). In Equation (4), distinct pairs of skills are delineated for each resilience aspect; for instance, within the physical aspect, ‘SkillA’ represents Strength and ‘SkillB’ represents Endurance. In this instance, the two skills are combined linearly (averaged), illustrating the potential for incorporating an expanded set of skills into the model. Adopting more complex mathematical models remains a viable option for further refinement and customization of the framework to suit specific scenarios and requirements.
Figure 1. Polar star graphs showing characteristics associated with (a) workers, (b) tasks, and (c) robots.
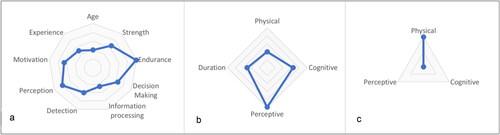
Age and overall experience were also selected as key variables, as they are among the most impactful and well-studied characteristics (Katiraee et al., Citation2021). We adapted a formula from Boenzi et al. (Citation2015) to include the effect of aging on physical fatigue. This formula is based on the phenomenon that physical capacity reaches its maximum at the age of 20 years, followed by a reduction, leading to an estimated 30% decline at the age of 60. For this study, cognitive and perceptual effects of age were not included. As fatigue tends to decrease when workers take rest breaks, we included the recovery formula from Jaber et al. (Citation2013), as shown in Equation (7).
A motivation factor was included to reflect a general and linear decrease in motivation over time in a shift. The linear function is a first approximation and ignores the potential boost in energy (motivation) immediately before the shift ends. Motivation is therefore modeled as a regular decay from an initial value of motivation (0: no motivation at all = no work, 1: normal motivation = normal work, 2: high motivation = highly productive work) and is computed for each category of workers (see Equation (5)). This approach acknowledges unmeasurable yet varying levels of worker motivation. For workers of the same age and skill level, if one is initially more motivated than the other, then his/her fatigue will increase more slowly during the shift. It is important to note that the initial value for motivation is not based on (un-) motivating circumstances: type of task, shift, day of the week, break duration, etc., as psychological factors are difficult to obtain and model. Motivation based on intrinsic or extrinsic rewards, such as worker personality or higher hourly rates paid for weekends or holidays, was not considered, although the model could be extended to include such elements as desired.
The robots were modeled simply as either physical (e.g., moving heavy items from area A to area B), perceptual (e.g., scanning an object to display specific alignment markers), or cognitive/processing (e.g., sorting items automatically) resources. The operational costs for the inclusion of robots in the production process were ignored in this modeling effort.
2.3. Worker Fatigue Model – Implementation
Figure 2 illustrates the conceptual framework for the Orbital Joint Assembly Process at Airbus. The Worker Fatigue Model is designed to simulate multiple workers and robots, with different characteristics, performing different tasks during work shifts. The macro level considers the entire assembly process. Information is provided on the composition of human and robot teams to maximize performance while minimizing human operator fatigue. The micro level considers the fatigue level of each individual worker in the team. Worker fatigue can be predicted depending on the schedule of the assigned tasks for any given worker. The fatigue model was developed using AnyLogic, a process modeling software based on the Java language that is designed to support agent-based and discrete event simulations.
To verify the Worker Fatigue Model for Airbus Orbital Joint Assembly Process, two scenarios were simulated and compared. One scenario represented a fully manual process whereas the other represented a robot-assisted process. To reflect real-life conditions in the plant, worker characteristics were manipulated for each scenario to include super workers, average workers, and basic workers. Super workers are characterized in the Airbus workforce as expert workers who are able to work at a high skill level on multiple tasks in the process; average workers are well trained for specific tasks within the process; and basic workers are low-skilled workers and limited to one or two assigned tasks. The input variables and their ranges of values are listed in . The verification of the model was based on model sensitivity from the output variables.
Table 2. Input variables for worker and task characteristics. Note that the robot is associated with a specific task and does not appear as an input variable.
A graphical user interface was designed to provide an easy configuration of the model input variables and a visualization of the output variables (). Results of the model cover both the macro and micro levels. At the “macro” level, the results show the overall effect of the average fatigue among workers. This effect is represented by three percentages, each reflecting a different dimension of industrial performance: quality, duration, and safety. Percentages represent the probability that an error on the assembly line affects either the quality of work, as in the case of non-conformities, the duration due to outstanding work, or the safety as an increased accident risk. The “micro” level focuses on either the team or a specific worker. It shows the evolution of the fatigue level for each worker as different tasks are performed. The quality and safety metrics are arithmetically derived from fatigue without considering other parameters. There is a (simplified) direct computation of the quality and safety depending on the (only) fatigue variable. For each time step, the percentage of an error/increase in duration/accident, is the error/duration/accident constant (arbitrary), multiplied by the average fatigue of the team of workers. As fatigue increases over time, the risks of errors, delays in task execution, and accidents also increase.
2.4. Simulation and Verification Results
A simulation of the two scenarios of the Orbital Joint Assembly process (fully manual, and semi-automated) was performed with the following specifications: each process was composed of 240 tasks performed by five teams of workers working simultaneously on the assembly line. Each worker worked a 7-h shift. Super workers were characterized by (μ = 0.8, σ = 0.2); average workers by (μ = 0.5, σ = 0.15); and basic workers by (μ = 0.3, σ = 0.15). A higher μ value is indicative of greater average resilience in workers.
Fully manual scenario: Each of the five teams consisted of four workers. The workforce was composed of two super workers and two basic workers in Team 1. Team 2 comprised four basic workers. Team 3 was composed of two basic workers and two average workers, whereas Teams 4 and 5 were composed of four average workers. The simulation results () show a steady increase in the fatigue level for workers during each shift and a slow decrease at the end of the shift. Super workers experienced fatigue more slowly than average workers, who in turn experienced fatigue more slowly than basic workers did. Gray areas on the Gantt chart represent rest breaks for the workers.
Figure 4. Simulation results showing fatigue levels for a fully manual production line (left) and a robot-assisted production line (right) of the Orbital Joint Assembly process (top), and error prediction due to fatigue (bottom). There is a steady increase of fatigue level for workers during each shift, and a slow decrease at the end of the shift. Super workers experienced fatigue more slowly than the average workers, who in turn experienced fatigue more slowly than basic workers. Gray areas on the Gantt chart represent rest breaks for workers.
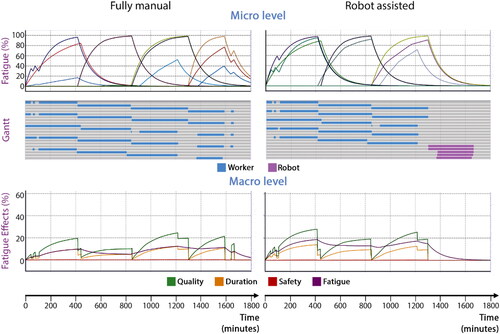
Semi-automated scenario: Each team consisted of a robot and three human workers. In Team 1, the human workers consisted of two super workers and one basic worker. Teams 2 and 3 were composed of three basic workers. Team 4 was composed of one basic worker and two average workers, whereas Team 5 was composed of three average workers. Thus, the workforce for this assembly line consisted of a total of 15 (two super, eight basic and five average) human workers and five robots (). The overall workload and fatigue of the workers were shown to decrease with the addition of robots compared to the fully manual scenario.
2.5. Stakeholder Feedback
In addition to the fatigue model development in the previous section, interviews and workshop discussions with various Airbus stakeholders provided valuable feedback regarding workers’ concerns about automation in a traditionally manual production industry. There was a consensus on the major benefits of automation in the work process:
Higher Product Quality: The implementation of automation was expected to yield products of superior quality, characterized by a reduced incidence of defects and non-conformities. This improvement in product quality could, in turn, contribute to a more efficient and reliable manufacturing process.
Enhanced Efficiency: Increased efficiency was seen as a significant benefit of automation. This efficiency gain could potentially be translated into shortened time needed for training operators and reduced time needed for quality corrections. This could thereby lead to maximized production output.
However, the adoption of automation has also been perceived as introducing certain drawbacks and misunderstandings:
Reduced Flexibility: As automation is integrated into various aspects of production, it could lead to a loss of flexibility in scheduling, line balancing, and human resource allocation. The adaptive coordination of tasks provided by human workers may be constrained by the rigidity of the automated processes.
Neglected preparation: When considering the benefits of automation, it is often presumed that the product and production environment within assembly plants are already primed for robotic operation. These prerequisites include accessibility to robots and the standardization of parts and components. The benefits also presume that the enhanced rate of automated production would justify the initial investment in acquiring and implementing robotic systems.
However, many organizational costs associated with robots are neglected. Notably, the cost and lead time needed to setup robotic systems are often not considered or documented. Considering the transformative impact of automation on the workforce, the workforce must be able to support the use of automation. Human-robot interaction introduces a new skill set requirement for human workers. These skills encompass various facets, including the ability to initiate, calibrate, monitor, troubleshoot, recover from failure, and execute shutdown procedures when collaborating with automation. The acquisition and maintenance of these specialized skills would necessitate dedicated training and an ongoing commitment to skill development.
3. Worker Fatigue Model Integration at Airbus
3.1. Integration and Implementation of Worker Fatigue Model at Airbus
During the product design phases in which the introduction of robots was considered, Airbus had limited data pertaining to the utilization of robots, associated tasks, and their associated costs. The Worker Fatigue Model, although verified to be sensitive to input variables such as workforce composition, could not be implemented with real data from the plants. In light of the concerns regarding automation from Airbus workers (see Section 2.5), and despite the positive simulated performance outcomes, it was determined that Airbus would refrain from conducting design simulations involving both robots and workers at this time.
Instead, for a final demonstration of the utility of the Worker Fatigue Model, the model was integrated with Airbus system models. At Airbus Central Research and Technology (R&T), a platform for experimental tool integration (DISM) is used for multidisciplinary analysis and optimization (Helle et al., Citation2022) ().
Figure 5. Conceptual overview of DISM platform for multidisciplinary analysis and optimization (Helle et al., Citation2022).

The DISM platform follows microservice paradigms to build applications by orchestrating small, independent services. The fundamental blocks of the DISM platform are a graph database to store data and ensure data consistency, a front-end to initiate individual simulations as well as design of experiments, and an analysis execution solution based on a message broker to connect domain models and calculation services in an efficient manner.
To support the analysis of the impact of different workforce compositions on the performance of the Orbital Joint process, the Worker Fatigue Model was integrated with a Workforce Generator application that generates sample sets of worker groups with different compositions of low and highly experienced and motivated workers (). Using the DISM web front-end, a Design of Experiment (DoE)—specifically repeated execution of one PAM [Parametric Analysis Model] with different inputs from the Worker Generator—was then launched to simulate the effect of different worker groups on the number of non-conformities and on the outstanding work for a specific orbital joint process.
Figure 7. Parametric analysis model. Parameter flow from workforce generator to Worker Fatigue Model.

provides an overview of the simulation workflow (i.e., Parametric Analysis Model) used for the integration of the Worker Fatigue Model in the Airbus Central R&T DISM platform. The Worker Fatigue Model (Fatigue Simulator) shown in the center of the figure receives information on the Orbital Joint process tasks from a JSON file (“LoadDB from JSON” function). It also receives worker profiles generated by a Workforce Generator application via another JSON file. The Worker Fatigue Model provides time-series information on the quality, duration, and safety of the Orbital Joint process as an outcome. An additional time-series analyzer function was implemented to provide mean, maximum, and integral values for the expected impact on the quality of the process (expected non-conformities).
3.2. Demonstration of Worker Fatigue Model
For this implementation demonstration, the Workforce Generator application utilized two Worker Group profiles: “Early Career” and “Mid-Career” workers. “Mid-Career” workers are deemed to have a higher level of experience and comprehension but slightly lower level of endurance. They represent workers that are considered “average skilled workers” (from Section 2.4) who are also older and tend to have lower endurance. Results from a DoE with 50 sampling sets of two worker ratios are shown in . In general, a higher ratio of “Early Career” workers to “Mid-Career” workers led to a greater reduction in quality, both in terms of average quality and maximum quality (). On the other hand, a higher ratio of “Mid-Career” to “Early Career” workers leads to lower negative impacts on quality ().
Figure 8. Parallel Coordinate Plot for visualization of the results of several simulation runs with different ratios of "Early Career" and "Mid-Career" workforces as inputs, and quality reduction as output (integral quality, max quality, average quality).
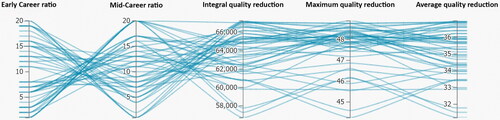
Figure 9. Example of a simulation run with high ratio of Early-Career to Mid-Career Workers (20:1). With this group of workers, reduction of quality is large, as seen on the integral quality scale, max quality scale, and average quality scale.
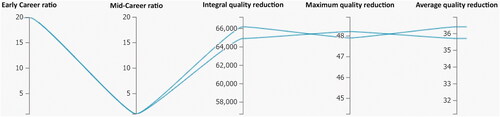
Figure 10. Example of a simulation run with a high ratio of Mid-Career to Early Career Workers (20:1). With this group of workers, the Mid-Career workers help to minimize the quality reduction, as seen on the integral quality scale, max quality scale and average quality scale. With a skill distribution from 0.3 to 0.7 for Mid-Career workers, impact on average quality reduction ranges from 31% to 35.5%.
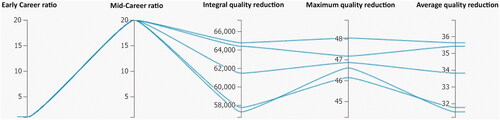
4. Discussion
This study was conducted to demonstrate the value of including human factors in industrial system models for designing new processes and products. Specifically, including a human performance model based on worker fatigue during a critical assembly task where robots are being introduced allows the company to visualize and better understand the impacts of manual versus robotic assistance on worker wellbeing (fatigue level and propensity for error) and system performance (i.e., time and costs). A Worker Fatigue Model was developed by adapting an existing human fatigue model designed for discrete-event simulations (Jaber et al., Citation2013) by incorporating age (Boenzi et al., Citation2015) and motivation factors to fit the Orbital Joint Assembly process at Airbus.
In simulation studies for model verification, the Worker Fatigue Model was sensitive to variations in the type of workers (super worker, average worker, and basic worker), team composition (different type of human workers and robots), and task demands (manual vs. robot-assisted). The amount of fatigue computed per worker was positively correlated with task difficulty, and the probability of an error occurring, which in turn impacts quality, duration, and safety. However, feedback from workers at Airbus assembly plants highlighted real concerns about automation that were not included in the model. For example, the model does not consider operational costs for workers while robots are active and engaged, including the human work necessary to set up, calibrate, operate, monitor, and recover in case of failure, which are tasks that did not exist before the introduction of robots.
Airbus workers reported a higher level of cognitive fatigue when tasked with monitoring robots during the Orbital Joint Assembly Process. One surmised that the manual process provided a diverse set of tasks, which resulted in a lower level of fatigue. Additionally, as the robotic systems were a new addition to the work process, a negative learning curve was observed when workers resumed work after the COVID shutdown. Even experienced workers took a long time to re-learn the task.
Finally, even though automation provides measurable benefits for productivity and efficiency in certain tasks and environments that are robot-compatible, for a company such as Airbus, whose business competitiveness is partly based on providing customization of aircrafts to different airlines, automation may present more challenges in implementation. Airbus experts expect automation to become more widespread, with limited productivity gains, but important improvements in product quality (consistently good, fewer errors) and a significant reduction in the burden on workers from non-ergonomic tasks. Downsides of automation are the perceived lower flexibility in comparison to human workers, and the safety restrictions around the automation area for humans running other tasks in parallel. Finally, the effects of transitioning workers to higher cognitive and perceptual workloads for in-process control of the automation in exchange for a reduced physical workload are unknown.
4.1. Limitations & Future Work
Our goal was to demonstrate the feasibility and potential of integrating human performance models into industrial system design. Thus, the Worker Fatigue Model is not meant to be a complete model. In this demonstration project, several aspects related to worker fatigue were not addressed, such as perceptual and cognitive fatigue due to the need to monitor robots during operation, accidents due to working in close proximity to the robots, and effects of boredom from lack of engagement when robots take over workers’ tasks. Further, due to limited data (from lack of access and a short history of automation uses at Airbus), model verification and implementation relied on simulated data, and many assumptions were made, such as configurations of worker profiles. Furthermore, we did not consider other important factors in worker wellbeing, such as a sense of autonomy, trust, and work satisfaction. Depending on the workforce and industrial sector, gender (Efe et al., Citation2018), age, motivation, work-rest schedule, skill level, incentives, and rewards, among others, may be more important.
Our study provides a practical methodology for incorporating human factors into industrial system models to improve the design of work processes and products. The Worker Fatigue Model allows Airbus to perform trade studies comparing different types of workers and their impact on different industrial system designs, such as the cost and savings associated with outstanding work and non-conformities. Our demonstration project illustrates a way for Airbus to engage in sustainable, human-centric approaches to manufacturing and production services at scale, and to support worker wellbeing in Industry 5.0.
4.2. Considerations/Recommendations
We recommend that companies interested in applying similar methodologies consider discussions with all stakeholders, including workers unions and production line experts. Collecting worker performance data through monitoring, even for the purpose of improving worker wellbeing and transitioning to Industry 5.0, is a highly difficult and sensitive undertaking and requires that we respect worker privacy and adhere to ethical and legal guidelines for data handling. Framed as a way to ensure worker wellbeing, a human-centered, a participatory approach is likely to lead to higher acceptance and buy-in from all stakeholders.
Acknowledgments
We thank the production line workers and managers at Airbus Hamburg for their expertise, the Region Bretagne, FEDER and the University of South Australia for funding the Chair for Industry of the Future (Prof. Cao), the International Max Planck Research School for Intelligent Systems (IMPRS-IS) and the AI@IMT program of the ANR (French National Research Agency) for supporting Arnaud Allemang--Trivalle.
Conflict of Interest
The authors declare no conflict of interest. Contents of this paper are solely the responsibility of the authors and do not necessarily represent the official views of Airbus.
Correction Statement
This article has been corrected with minor changes. These changes do not impact the academic content of the article.
Additional information
Funding
References
- Abubakar, M. I., & Wang, Q. (2019). Key human factors and their effects on human centered assembly performance. International Journal of Industrial Ergonomics, 69, 48–57. https://doi.org/10.1016/j.ergon.2018.09.009
- Alexander, T., & Fromm, L. (2019). Beyond anthropometry and biomechanics: Digital human models for modeling realistic behaviors of virtual humans. In D. N. Cassenti (Ed.), Advances in human factors in simulation and modeling (pp. 26–33). Springer International Publishing. https://doi.org/10.1007/978-3-319-94223-0_3
- Allemang-Trivalle, A., Donjat, J., Bechu, G., Coppin, G., Klaproth, O. W., Mitschke, A., Schirrmann, A., Chollet, M., & Cao, C. G. L. (2023). Human model for industrial system and product design in Industry 5.0: A case study. IISE Annual Conference Proceedings, 1–2.
- Arista, R., Zheng, X., Lu, J., & Mas, F. (2023). An ontology-based engineering system to support aircraft manufacturing system design. Journal of Manufacturing Systems, 68, 270–288. https://doi.org/10.1016/j.jmsy.2023.02.012
- Boenzi, F., Digiesi, S., Mossa, G., Mummolo, G., & Romano, V. A. (2015). Modelling workforce aging in job rotation problems. IFAC-PapersOnLine, 48(3), 604–609. https://doi.org/10.1016/j.ifacol.2015.06.148
- Breque, M., De Nul, L., & Petridis, A, European Commission, Directorate-General for Research and Innovation. (2021). Industry 5.0: Towards a sustainable, human centric and resilient European industry. Publications Office of the European Union. https://doi.org/10.2777/308407
- Brombach, J., Kämpfer, G., & Markfort, J. (2019). Von der Idee zur ergonomischen Verbesserung – Ein Projekt bei Airbus. In Landmann, N., & Schat, H.D. (Eds.), Ideen erfolgreich managen. Springer Gabler. https://doi.org/10.1007/978-3-658-26520-5_1
- Efe, B., Kremer, G., & Kurt, M. (2018). Age and gender-based workload constraint for assembly line worker assignment and balancing problem in a textile firm. International Journal of Industrial Engineering: Theory Applications and Practice, 25, 1–17. https://doi.org/10.23055/ijietap.2018.25.1.2961
- Elmaraghy, W. H., Nada, O. A., & Elmaraghy, H. A. (2008). Quality prediction for reconfigurable manufacturing systems via human error modelling. International Journal of Computer Integrated Manufacturing, 21(5), 584–598. https://doi.org/10.1080/09511920701233464
- Fritzsche, L., Jendrusch, R., Leidholdt, W., Bauer, S., Jäckel, T., & Pirger, A. (2011). Introducing EMA (editor for manual work activities) – A new tool for enhancing accuracy and efficiency of human simulations in digital production planning. In V. G. Duffy (Ed.), Digital human modeling (Vol. 6777, pp. 272–281). Springer. https://doi.org/10.1007/978-3-642-21799-9_31
- Fuchs, K., Sonntag, N., Schnalke, G., & Jacobsen, A. (2016). Die Produktionsergonomie im demografischen Wandel. In Gesellschaft für Arbeitswissenschaft e.V. Herbstkonferenz 2016: Fokus Mensch im Flugzeugbau.
- Guiffrida, A. L., & Nagi, R. (1998). Fuzzy set theory applications in production management research: A literature survey. Journal of Intelligent Manufacturing, 9(1), 39–56. https://doi.org/10.1023/A:1008847308326
- Helle, P., Feo-Arenis, S., Mitschke, A., & Schramm, G. (2019). Smart component modeling for complex system development. In International conference on complex systems design & management (pp. 117–128). Springer International Publishing. https://doi.org/10.1007/978-3-030-34843-4_10
- Helle, P., Richter, S., & Schramm, G. (2022). Implementing and deploying an execution environment for multidisciplinary-analyses in a heterogeneous tool landscape [Paper presentation]. The Eighth International Conference on Fundamentals and Advances in Software Systems Integration (FASSI 2022), Lisbon, Portugal.
- Jaber, M. Y., Givi, Z. S., & Neumann, W. P. (2013). Incorporating human fatigue and recovery into the learning–forgetting process. Applied Mathematical Modelling, 37(12–13), 7287–7299. https://doi.org/10.1016/j.apm.2013.02.028
- Katiraee, N., Calzavara, M., Finco, S., Battini, D., & Battaïa, O. (2021). Consideration of workers’ differences in production systems modelling and design: State of the art and directions for future research. International Journal of Production Research, 59(11), 3237–3268. https://doi.org/10.1080/00207543.2021.1884766
- Kolus, A., Wells, R., & Neumann, P. (2018). Production quality and human factors engineering: A systematic review and theoretical framework. Applied Ergonomics, 73, 55–89. https://doi.org/10.1016/j.apergo.2018.05.010
- Nardo, M., Forino, D., & Murino, T. (2020). The evolution of man-machine interaction: The role of human in Industry 4.0 paradigm. Production & Manufacturing Research, 8(1), 20–34. https://doi.org/10.1080/21693277.2020.1737592
- van Laarhoven, P. J. M., & Pedrycz, W. (1983). A fuzzy extension of Saaty’s priority theory. Fuzzy Sets and Systems, 11(1–3), 229–241. https://doi.org/10.1016/S0165-0114(83)80082-7