Abstract
The reliance on vision to control a myoelectric prosthesis is cognitively burdensome and contributes to device abandonment. The feeling of uncertainty when gripping an object is thought to be the cause of this overreliance on vision in hand-related actions. We explored if experimentally reducing grip uncertainty alters the visuomotor control and mental workload experienced during initial prosthesis use. In a repeated measures design, twenty-one able-bodied participants took part in a pouring task across three conditions: (a) using their anatomical hand, (b) using a myoelectric prosthetic hand simulator, and (c) using a myoelectric prosthetic hand simulator with Velcro attached to reduce grip uncertainty. Performance, gaze behaviour (using mobile eye-tracking) and self-reported mental workload, was measured. Results showed that using a prosthesis (with or without Velcro) slowed task performance, impaired typical eye-hand coordination and increased mental workload compared to anatomic hand control. However, when using the prosthesis with Velcro, participants displayed better prosthesis control, more effective eye-hand coordination and reduced mental workload compared to when using the prosthesis without Velcro. These positive results indicate that reducing grip uncertainty could be a useful tool for encouraging more effective prosthesis control strategies in the early stages of prosthetic hand learning.
Introduction
Myoelectric prosthetic hands are controlled by surface electromyography (EMG) and are often provided to individuals with upper-limb difference to increase functionality. The electrodes placed on the skin detect EMG signals from muscle contractions, driving the opening and closing of the prosthetic hand to grasp objects. Due to the challenges in emulating the functionality of an anatomic hand, abandonment of these devices can be high (Biddiss & Chau, Citation2007; Salminger et al., Citation2022). Factors contributing to device abandonment are numerous, but common complaints include the dissatisfaction with appearance, comfort, fit, and weight of the device (Smail et al., Citation2021).
Another commonly cited reason for prosthesis abandonment is the cognitive burden imposed on users to visually monitor their prosthesis actions (Biddiss & Chau, Citation2007). When able-bodied individuals perform reaching and grasping actions they typically adopt a “target-focused” visual strategy where visual attention is used in a feedforward manner with vision typically located ahead of the hands (Land, Citation2009). Such a strategy allows the visual system to provide information to the motor systems ahead of time so that actions can be planned and executed in an efficient and effective manner. When using a prosthetic hand this eye-hand coordination breaks down and prosthesis users tend to adopt a “hand-focused” strategy where visual attention is used in a feedback manner. For example, prosthesis users watch the hand when reaching and grasping for objects and display a tendency to monitor the grasped object when transporting it to a target location (Bouwsema et al., Citation2012; Parr et al., Citation2018; Sobuh et al., Citation2014). The cognitive burden imposed by adopting this hand-focused visual strategy is corroborated by evidence that those who spend more time fixating their device self-report higher levels of mental workload (Parr et al., Citation2023) and display a global decrease in electroencephalography alpha power when using the device, indicative of less efficient brain activity (Parr et al., Citation2019). Understanding why users spend so much time watching their prosthesis is therefore critical for understanding this cognitive burden and designing interventions to alleviate it.
One possible explanation is that the reliance on vision to monitor the prosthesis represents a fundamental stage in the development of effective eye-hand coordination. By monitoring the hand, users may close the visual-manual loop to develop “sensorimotor mapping rules” between intended actions and movement outcomes, and between vision and proprioception in a trial-and-error fashion (Sailer et al., Citation2005, p. 8833). With practice individuals should be able to better-predict the consequences of their actions based on proprioceptive feedback, reducing the need to monitor hand-related actions. This then allows the visual system to function in a more feedforward manner. Indeed, the transition from “hand-focused” to “target-focused” visual strategies in response to practice has been observed in other human-tool interactions such as when using chopsticks (Bosch et al., Citation2018) and laparoscopic surgical tools (Vine et al., Citation2012). Prosthesis users, however, do not seem to relinquish their reliance on vision as would be expected (Bouwsema et al., Citation2012; Chadwell et al., Citation2018), unless explicitly trained to do so (Parr et al., Citation2019). Consequently, the view that this reliance on vision always reflects a functional process to develop sensorimotor mapping rules appears lacking.
An alternative suggestion is that the reliance on vision to monitor prosthesis action reflects a more passive and compensatory strategy in the face of prosthesis “uncertainty” (Parr et al., Citation2022). Unlike rigid tools that have fixed intrinsic properties (e.g., chopsticks and surgical tools), the reliability of prosthesis responsiveness can fluctuate due to EMG signal artefact arising from sweating, poor fitting, or fatigue. Chadwell et al. (Citation2016, Citation2021) showed that prosthesis users who experience a greater frequency of undesired prosthesis activations (i.e., the hand opening/closing accidentally) spent more time watching their device during a multistage functional task, suggesting that the uncertainty surrounding prosthesis responsiveness may drive the overreliance on vision to supervise the device and safeguard against task failures (e.g., dropping a held object). In support of this, a recent survey- and interview-based study identified that prosthesis users are frequently worried they cannot trust their prosthesis to do what they expect it to do (Jabban et al., Citation2022). As a result, many users (∼40% of participants) reported concern about being able to hold objects securely without dropping them, leading to a sense of nervousness around object interaction and the overreliance on vision. It could, therefore, be argued that reducing the feeling of “uncertainty” and/or lowering concerns about dropping objects during prosthesis control might be the key to developing more effective visuomotor control strategies and reducing the cognitive burden experienced by users.
We explored whether experimentally reducing grip uncertainty alters the performance, visuomotor control, and mental workload experienced by prosthesis users. In a counterbalanced repeated measures design, able-bodied participants performed a pouring task using (a) their anatomical hand, (b) a myoelectric prosthetic hand simulator, and (c) a myoelectric prosthetic hand simulator with Velcro attached to the prosthesis and grasped objects to reduce grip uncertainty. Although the addition of Velcro was not expected to eliminate the inherent uncertainty surrounding prosthesis responsiveness (as caused by irregularities in surface EMG), we confirmed in a pilot study that the addition of Velcro increases confidence in grip security (∼56% more confident) and lowers concerns about dropping objects (∼63% less worried) when first using a prosthesis simulator (Supplementary file 1). In line with previous research, we expected participants to perform quicker, spend less time fixating on their hand, shift their gaze towards the target more quickly, and report lower mental workload when using their anatomical hand compared to the two prosthesis conditions. We also hypothesised that reducing grip uncertainty by attaching the Velcro to the prosthesis, would result in participants spending less time fixating on the prosthetic hand, shifting their gaze towards the target more quickly, and reporting lower levels of mental workload compared to the prosthesis condition without Velcro.
Method
Participants
Twenty-one able-bodied participants (10 males and 11 females) aged between 18 to 42 years (M = 23.95, SD = 6.71) took part in this study. Participants were predominantly right-handed (Mdn = 86.67, IQR = 25.4) as indexed by the Edinburgh Handedness Inventory (Oldfield, Citation1971). An a priori sample size was calculated using G*Power software (version 3.1.9.7; Heinrich Heine University Dusseldorf, Dusseldorf, Germany; Faul et al., Citation2007). To detect an effect size of η2p at 0.21 with an alpha level at p < .05, a sample size of 21 was required to yield 80% power level. The effect size was obtained from Parr et al. (Citation2019) due to close similarity with the current study in the repeated measures design, statistical analysis, recruitment of able-bodied participants, and the type of myoelectric prosthesis. Ethical approval was granted by the institutional ethics committee (EthOS ID: 40689) and written consent form was obtained from all participants. The study was performed in accordance with the Declaration of Helsinki.
Apparatus
Prosthetic Hand Simulator
The prosthesis used in this study was the right-handed Bebionic™ (Otto Bock HealthCare, Duderstadt, Germany) fully articulating myoelectric prosthetic hand (). The specification of the prosthetic hand is identical to that used by Parr et al. (Citation2018). The hand was programmed for a lateral grip, otherwise known as the key grip (Light et al., Citation2002), as this set-up was most appropriate for completing the behavioural task used in this study.
Eye Tracking Glasses
Participants wore an ETG 2 w mobile eye tracker using iView ETG 2.7 software (SMI, Teltow, Germany) connected to a Dell Precision M4800 laptop running Windows 8.1. The system comprises a pair of lightweight glasses that track binocular eye movements at a sampling rate of 60 Hz with a gaze position accuracy of 0.5°, and 24 video frames per second. The eye tracking system was calibrated for each participant using a one-point calibration prior to each condition by instructing them to look at a fixation cross, given its efficiency and equivalent measurement accuracy to a three-point calibration process (Ambrose et al., Citation2021; SensoMotoric Instruments (SMI), Citation2016). Calibration was checked after every trial by asking participants to look at task-relevant objects before looking back at the fixation cross in preparation for the next trial. If calibration was not accurate, the eye-tracker was quickly recalibrated.
Procedure
Participants were invited to the laboratory for a single testing session lasting approximately 1.5 h and were sent a participant information sheet via email. Upon arrival, participants were seated comfortably at a table before providing their written informed consent and being fitted with the eye-tracker. Participants then performed five experimental trials of a rice pouring task using their anatomic right hand (Anatomic), the myoelectric prosthetic hand simulator (Prosthesis), and the myoelectric prosthetic hand simulator with Velcro attached to the fingertips and mug handle to reduce grip uncertainty (Velcro). All participants first completed five trials (Frykberg et al., Citation2021) using their anatomic hand, allowing participants to become fully familiarised with the task components. Participants then performed five trials under counterbalanced Prosthesis and Prosthesis + Velcro conditions. The task required participants to pour a glass mug full of uncooked rice, weighing approximately 320 g (rice = 110 g), into two cups placed on the work surface without spilling any rice or dropping the mug. A similar task has been shown to place high demands on compensatory visual fixations, and prolonged grasp and release movements when using a prosthesis (Hebert et al., Citation2019). The use of rice as the pourable content was chosen as it was deemed safer than pouring liquids that could potentially damage the electrical equipment in the myoelectric prosthesis and eye-tracker in the event of spillages. Participants started each trial with their right hand rested on the table between the mug and the first cup, and their gaze fixated upon a cross located on the wall in front of them (). Once the experimenter verbally indicated the start of the trial, participants grasped the mug and sequentially filled each cup (from right to left) to a “fill-line” marked on each cup. Participants then returned the mug to its starting position and returned their gaze to the fixation cross, marking the completion of the trial.
The prosthetic hand is controlled by muscular contractions detected by two electrodes placed on the extensor (extensor carpi radialis) and flexor (flexor carpi radialis) muscles of the forearm. Contraction of the extensor muscle triggers the opening of the hand, whereas contraction of the flexor muscle triggers the closing of the hand. When first fitted with the prosthetic hand, participants were required to successfully open and close the hand ten times on demand to indicate they could sufficiently control the device (Parr et al., Citation2018, Citation2019). For the Prosthesis condition, the task was completed with no Velcro as would be typically experienced by users of these devices. The Velcro condition had Velcro attached to the thumb and index finger of the prosthetic hand and around the protruding handle of the mug. Participants were told that the addition of Velcro would prevent the mug from being dropped and that any unintended activations of the device would therefore have no negative consequence. Following the completion of each condition, perceptions of mental workload experienced were measured.
Measures
Performance Time and Unintended Grip Release
Performance time was measured offline using the video footage obtained from the mobile eye-tracker. Specifically, we recorded the elapsed time (in seconds) from the moment participants first looked away from the fixation cross to initiate the trial, to the moment they returned their gaze to the fixation cross upon completion of the task. We adopted this approach to minimise human error that could occur when attempting to manually record these times online. We also recorded the total number of unintended grip releases in each condition, defined as any non-task-related hand opening activations while holding the mug. Unintended device activations has been shown to impair performance (Chadwell et al., Citation2021), so we wanted to understand if reducing grip uncertainty would consequently affect this.
Target Locking Strategy
To describe the extent to which participants visually monitored the hand across each condition we utilised the Target Locking Strategy (TLS) as used in previous research (Parr et al., Citation2018, Citation2019). The TLS metric is computed by subtracting the percentage of time fixating the hand from the percentage of time fixating target locations. A positive TLS reflects more time spent fixating targets, hence, “target-focused” strategy. A negative score reflects more time spent fixating the hand, hence, “hand-focused” strategy. A TLS of zero would indicate an equal amount of time fixating the hand and targets. Any fixations made towards the hand (or prosthesis) or objects that were being manipulated by the hand were deemed to be hand-focused, whereas fixations towards the target object or location during a given phase of movement were considered target-focused. For example, fixations towards the mug would be considered target-focused whilst reaching for it but considered hand-focused once grasped (Parr et al., Citation2018, Citation2019).
The recorded fixations were initially processed using the BeGaze analysis software (SMI, Teltow, Germany). A fixation was defined automatically by the software as any static gaze fixation over 80 ms in duration. provides the categorisation of areas of interests (AOIs) and visual representation of each task phase, defining their given onset and offset. The data were then exported to MATLAB R2021a (MathWorks Inc, Massachusetts, United States) to process the TLS. AOIs were coded as either hand-focused or target-focused categories (Parr et al., Citation2018).
Gaze Shift
The temporal sequencing of gaze behaviour was measured to understand participants’ ability to look towards the next phase of the task before the completion of the current phase, a measure of feedforward visual control (Parr et al., Citation2018, Citation2019). This was calculated as the time taken in milliseconds for participants to shift their attention from looking at their hand, towards looking at the target object. Therefore, if participants shifted their attention ahead of the hand between phases, a negative time was recorded. If the participant shifted their attention behind the hand, a positive time was recorded. Thus, a negative time could be indicated as a feedforward control, while a positive time indicated a feedback control. This measure was quantified in between the phases of lifting to pour rice in the first cup, transporting between the first cup to the second cup, and transporting the mug to the end position.
Mental Workload
The Prosthesis Task Load Index (PROS-TLX) was used to measure perceptions of mental workload (Parr et al., Citation2023). Participants were asked to report their perception of workload related to eight constructs (i.e., mental demands, physical demands, visual attention, conscious processing, frustration, situational stress, time pressure, and uncertainty) after each condition on a 21-point Likert scale ranging from 0 ‘very low’ to 20 ‘very high’; a lower score would therefore indicate lower workload. The weighting of each construct was determined by asking participants to make a series of 28 pairwise comparisons. The workload of each score was multiplied by the weighted score. The total workload was calculated by summing the workload score and dividing by the eight constructs.
Statistical Analysis
A single trial of the performance time for one participant was removed due to the prosthesis being unresponsive when attempting to pick up the mug. A Shapiro-Wilk’s test revealed that data for performance, unintended grip releases, and mental workload were not normally distributed. Consequently, a Friedman’s ANOVA was used to test the main effect of condition (Anatomic, Prosthesis, Velcro), with post-hoc tests performed using Wilcoxon Signed-Rank Test to investigate significant Friedman’s ANOVA test outcomes. For TLS, a 3 (Condition) × 6 (Phase) repeated measures ANOVA was performed, whilst for gaze-shifting a 3 (Condition) × 3 (Phase) repeated measures ANOVA was performed. Where sphericity was violated, the Greenhouse-Geisser corrections were applied. The effect size for the ANOVA tests were reported as ηp2, and the Wilcoxon Signed-Rank Tests were reported as r = Z/√N. Pairwise comparisons were adjusted via Bonferroni corrections (i.e., p values inflated but α remained < .05) to counteract the problem of multiple comparisons. The parametric data are reported descriptively as the mean (M) and standard deviation (SD), whereas non-parametric data are reported descriptively as the median (Mdn) and interquartile range (IQR). All analysis was conducted using IBM SPSS Statistics version 28.
Results
Performance Time and Unintended Grip Release
The Friedman Test showed a significant difference in performance time (), χ2(2) = 38.38, p < .001. The Wilcoxon Signed-Rank Test showed that participants performed significantly faster in the Anatomic condition (Mdn = 12.11, IQR = 4.05) compared to the Prosthesis (Mdn = 49.57, IQR = 30.69; Z = −4.01, p < .001, r = .51) and Velcro (Mdn = 32.32, IQR = 22.87; Z = −4.01, p < .001, r = 0.51) conditions. Participants also performed significantly faster in the Velcro condition compared to the Prosthesis condition (Z = −3.52, p = .001, r = .44).
FIGURE 3. Graphs of the individual data points (coloured circles), boxplot, and violin plot showing the distribution between the conditions for performance time (left) and the number of unintended grip release (right). The horizontal black line in the boxplot represents the median score, while the brackets extending off the top and bottom of the coloured boxes represent the interquartile range. The violin plot indicates the density of the data across the distribution. The Anatomic condition is not shown on the right graph because there were no unintended grip releases in that condition. Asterisks indicate a significant difference between the conditions (*p < 0.05, **p < 0.01, ***p < 0.001).
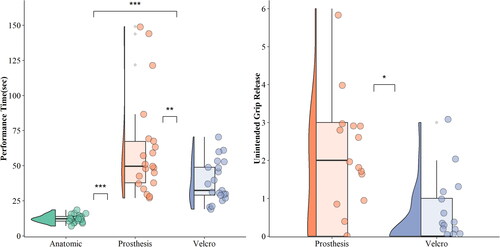
The Friedman Test also showed a significant difference in the number of unintended grip releases, χ2(2) = 17.08, p < .001. The Wilcoxon Signed-Rank Test showed the Anatomic condition had significantly fewer unintended grip releases than the Prosthesis condition (Z = −3.21, p = .004, r = .40), but not the Velcro condition (Z = −2.26, p = .07, r = .29). There was a significant difference in the number of unintended grip releases between the Velcro and Prosthesis conditions (Z = −2.63, p = .03, r = .33).
Target Locking Strategy
A 6 (phase) x 3 (condition) repeated measures ANOVA revealed significant main effects of Phase, F(2.36, 47.32) = 92.35 p < .001, ηp2 = .82, and Condition, F(2, 40) = 369.69, p < .001, ηp2 = .94, which were both superseded by a significant interaction between Phase and Condition, F(5.43, 108.73) = 13.92, p < .001, ηp2 = .409 (). Pairwise comparisons showed the Anatomic condition was significantly less hand-focused than the Prosthesis and Velcro conditions across all task phases (ps < .001). The Velcro condition was significantly less hand-focused than the Prosthesis condition in the first and second pour phases (ps < .001).
FIGURE 4. A line graph showing the percentages of the target locking strategy. The lines represent the percentage of hand-focus against the target-focus for each condition across the phases, while the error bars represent the standard error. Asterisks indicate a significant difference between the Prosthesis and Velcro conditions in the first and second pour phase (***p < 0.001).
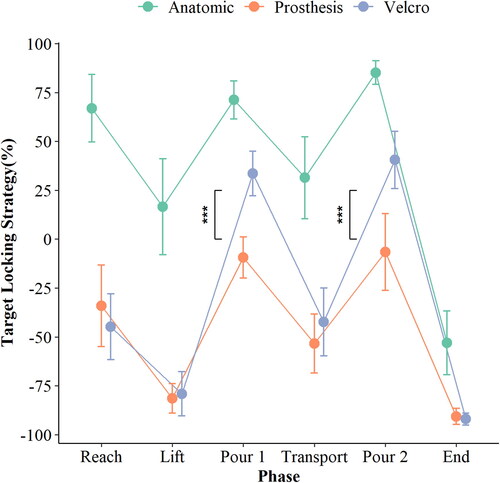
Gaze Shift
A 3 (phase) × 3 (condition) repeated measures ANOVA revealed significant main effects of Phase, F(2, 40) = 75.53, p < .001, ηp2 = .791, and Condition, F(2, 40) = 134.19, p < .001, ηp2 = .871, which were both superseded by a significant Phase × Condition interaction, F(2.78, 55.71) = 7.43, p < .001, ηp2 = .271 (). Pairwise comparisons showed that participants made significantly faster gaze shifts towards target locations during the Anatomic condition than they did during the Prosthesis and Velcro conditions across all task phases (ps < .001). Participants also displayed a significantly faster gaze shifts towards target locations in the Velcro condition compared to the Prosthesis condition across all task phases (ps ≤ .02).
FIGURE 5. A line graph showing the moment participants disengage visual fixations from the hand in between phases, while the error bars represent the standard error. A negative time represents participant shifting attention ahead of the hand, while a positive time represents participants shifting attention behind the hand. Asterisks indicate a significant difference between the Prosthesis and Velcro conditions (*p < 0.05, **p < 0.01, ***p < 0.001).
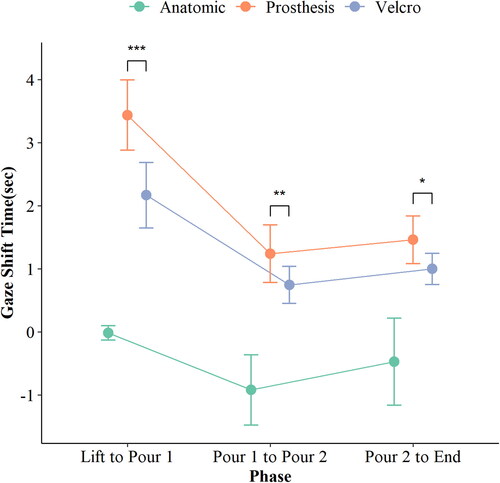
Mental Workload
and show the results of statistical analyses of the PROS-TLX data. Except for Time Pressure, the Anatomic condition had significantly lower scores across all constructs compared to the Prosthesis and Velcro conditions. The Velcro condition had significantly lower scores compared to the Prosthesis condition for Mental Demands, Uncertainty, and Total Workload.
FIGURE 6. A boxplot of the PROS-TLX showing the distribution between the conditions across all PROS-TLX constructs. A higher score represents an increased level of demand within the construct. Asterisks indicate a significant difference between the Prosthesis and Velcro conditions (*p < 0.05).
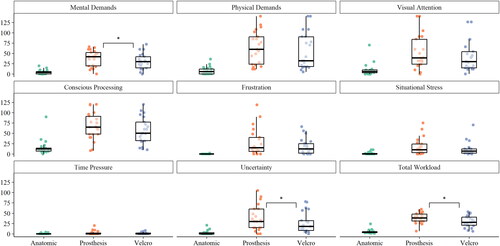
TABLE 1. Showing the statistics for PROS-TLX scores for each construct, the Bonferroni corrected pairwise comparisons, Wilcoxon Signed Rank Test, and r effect size between each group.
Discussion
We explored whether experimentally reducing grip uncertainty during initial prosthetic hand use altered performance, eye-hand coordination, and the mental workload experienced. Consistent with previous research (Chadwell et al., Citation2016; Parr et al., Citation2018, Citation2019), using the prosthesis (with or without Velcro) resulted in slower performance times, more hand-focused fixations, slower shifts of gaze towards target locations, and heightened mental workload compared to when using an anatomic hand. However, when the Velcro was attached to the prosthesis, participants reported lower levels of uncertainty (as indexed by the PROS-TLX), performed more quickly, exhibited fewer unintended grip releases, displayed faster shifts of gaze towards target locations, reduced their hand-focused fixations during the pouring phases, and reported lower levels of mental workload compared to when the Velcro was not attached to the prosthesis. Our findings therefore support the view that the reliance on vision to monitor prosthesis actions is related to grip uncertainty, and that reducing uncertainty might promote more effective eye-hand coordination and reduce cognitive effort.
These findings highlight that grip uncertainty plays an important role in the spatiotemporal allocation of gaze when first using a prosthesis. From a temporal perspective, reducing uncertainty decreased the time participants spent watching a grasped object when first transporting it (i.e., earlier gaze shifts towards target locations), and encouraged faster and less errorful task performance. Previous research has shown that faster shifts of gaze towards target locations are associated with better performance when first learning to use a prosthesis (Parr et al., Citation2018), and typically develop with increasing skill in other human-tool interactions (Bosch et al., Citation2018; Vine et al., Citation2012). It is proposed that adopting earlier “look ahead fixations” reflects an improved ability to predict the sensorimotor outcomes of intended actions, and serves effective eye-hand coordination by providing target-related visual information to the motor system, so that actions can be planned and executed in a timely and coordinated manner (Land, Citation2009). As such, our findings indicate that the tendency to visually guide grasped objects with a prosthesis reflects a compensatory strategy to overcome sensorimotor uncertainty in a manner that slows performance and elevates cognitive demands.
From a spatial perspective, reducing grip uncertainty resulted in significantly fewer hand-focused fixations (i.e., increased TLS) during the two pouring phases. Previous research has shown that the volatility of unintended prosthesis openings is magnified when participants are required to rotate their device during goal-directed tasks (like when pouring), because these actions increase the tendency for the electrodes being activated erroneously (Chadwell et al., Citation2021). It is therefore possible that reducing grip uncertainty was particularly effective for the pouring phases as it decreased the precarity of unintended prosthesis activations that might have led to a greater risk of spillages or breakages. That said, participants still displayed greater hand-focused gaze during the Velcro condition compared to the anatomic condition, and the spatial allocation of gaze was not different between the Velcro and non-Velcro prosthesis conditions during the reaching and transport phases. Consequently, our findings suggest that grip uncertainty cannot fully explain the tendency to visually monitor the prosthesis. Indeed, the PROS-TLX scores reveal that while the sense of uncertainty was significantly lower with the attachment of Velcro (PROS-TLX = ∼27) compared to without it (PROS-TLX = ∼38), it remained significantly higher compared to the anatomic hand (PROS-TLX = ∼2). As such, the hand-focused gaze observed during initial prosthetic hand control is likely to encompass the broader inherent uncertainty associated with controlling and moving the prosthesis. For instance, Velcro does not directly tackle concerns related to the uncertainty of prosthesis responsiveness, a pivotal factor influencing prosthesis-focused gaze that is caused by irregularities in surface EMG (Chadwell et al., Citation2021; Jabban et al., Citation2022). That said, the introduction of Velcro decreased the frequency of unintended grip releases (and improved performance speed), implying that reducing concerns about dropping objects indirectly improves prosthesis control. Whilst speculative, it is possible that these effects are driven by the observed differences in total workload when using the prosthesis with Velcro. In essence, reducing worry may free up attentional resources that can be utilised to meet the demands of the task (Eysenck & Calvo, Citation1992).
Our findings raise the possibility that training with reduced grip uncertainty could benefit rehabilitation by implicitly training users to adopt more effective gaze strategies. To elaborate, reducing grip uncertainty will inherently reduce the frequency of performance errors and could therefore promote “errorless” motor learning—a form of implicit motor learning shown to support motor skill learning across sport and rehabilitation (Maxwell et al., Citation2001). Errorless learning involves minimising errors during the learning process, typically by starting with an easily achievable task that is incrementally made more difficult throughout practice. By minimising errors, the demands on working memory to detect and correct for performance errors can be reduced, promoting the accrual of implicitly learned motor skills that are less cognitively demanding (Poolton et al., Citation2005) and more robust when multitasking (Maxwell et al., Citation2001). We suggest that the addition of the Velcro produced an errorless learning environment where reduced prosthesis uncertainty decreased mental demands and the overall workload experienced (). Previously, researchers have established a relationship between mental demands, conscious processing, total workload and the amount of visual attention directed towards the prosthesis (Parr et al., Citation2023), therefore it is likely that these reductions in cognitive workload allowed vision to be used in a more efficient and effective manner. In future work, we will test the utility of training with decreased device uncertainty.
There are several limitations to consider for this study. For example, we have only explored the impact of reduced uncertainty in the context of initial prosthesis use. It is therefore unclear how training with reduced uncertainty would impact long-term prosthesis skill acquisition and the transfer of these skills to real-word scenarios when typical levels of uncertainty are reintroduced. It is plausible that long-term practice under reduced uncertainty conditions may lead to a dependency on Velcro attachments. This dependence, in turn, could potentially hinder learning and skill development when uncertainty is reintroduced. To better understand the long-term implications of reduced uncertainty and its subsequent removal, further research is needed. A further limitation is that participants were limb-intact users of a prosthesis simulator rather than individuals with limb loss/difference. However, it is important to note that both prosthesis users and participants using a prosthesis simulator display similarities in kinematic profiles (Bouwsema et al., Citation2014), visuomotor behaviours (Sobuh et al., Citation2014), and perceptual experiences (Buckingham et al., Citation2018). This suggests that recruiting limb-intact participants for a study using a prosthesis simulator can provide some useful insight for sensory-motor deficits faced by actual prosthesis users when learning and using the device (Sinke et al., Citation2022).
In conclusion, our findings provide evidence that reducing grip uncertainty when first learning to use a myoelectric prosthetic hand can encourage more effective visuomotor control and lower mental workload. However, even with the addition of Velcro, participants remained highly dependent on vision to guide their actions, implying that grip uncertainty alone cannot fully explain the tendency to watch the prosthesis. Prosthesis-focused gaze is therefore likely to additionally reflect the functional development of sensorimotor mapping rules to support hand-eye coordination, and/or a compensatory strategy to overcome more general aspects of prosthesis uncertainty (such as responsiveness). Future work is needed to determine whether training with reduced grip uncertainty could improve the early stages of clinical rehabilitation by decreasing the heavy reliance on vision. Adopting such an approach may encourage users to learn a more target-focused and feed-forward gaze behaviour and alleviate the mental workload.
Supplemental Material
Download MS Word (186 KB)Disclosure statement
The authors do not report any financial or business interest in the publishing of this article. No potential conflict of interest was reported by the authors.
Additional information
Funding
References
- Ambrose, D., MacKenzie, D. E., Ghanouni, P., & Neyedli, H. F. (2021). Investigating joint attention in a guided interaction between a child with ASD and therapists: A pilot eye-tracking study. British Journal of Occupational Therapy, 84(10), 637–646. https://doi.org/10.1177/0308022620963727
- Biddiss, E. A., & Chau, T. T. (2007). Upper limb prosthesis use and abandonment: A survey of the last 25 years. Prosthetics and Orthotics International, 31(3), 236–257. https://doi.org/10.1080/03093640600994581
- Bosch, T. J., Hanna, T., Fercho, K. A., & Baugh, L. A. (2018). Behavioral performance and visual strategies during skill acquisition using a novel tool use motor learning task. Scientific Reports, 8(1), 13755. https://doi.org/10.1038/s41598-018-32001-4
- Bouwsema, H., Kyberd, P. J., Hill, W., van der Sluis, C. K., & Bongers, R. M. (2012). Determining skill level in myoelectric prosthesis use with multiple outcome measures. Journal of Rehabilitation Research and Development, 49(9), 1331–1348. https://doi.org/10.1682/JRRD.2011.09.0179
- Bouwsema, H., van der Sluis, C. K., & Bongers, R. M. (2014). Changes in performance over time while learning to use a myoelectric prosthesis. Journal of Neuroengineering and Rehabilitation, 11(1), 16. (https://doi.org/10.1186/1743-0003-11-16
- Buckingham, G., Parr, J., Wood, G., Vine, S., Dimitriou, P., & Day, S. (2018). The impact of using an upper-limb prosthesis on the perception of real and illusory weight differences. Psychonomic Bulletin & Review, 25(4), 1507–1516. https://doi.org/10.3758/s13423-017-1425-2
- Chadwell, A., Kenney, L., Granat, M. H., Thies, S., Head, J., Galpin, A., Baker, R., & Kulkarni, J. (2018). Upper limb activity in myoelectric prosthesis users is biased towards the intact limb and appears unrelated to goal-directed task performance. Scientific Reports, 8(1), 11084. https://doi.org/10.1038/s41598-018-29503-6
- Chadwell, A., Kenney, L., Thies, S., Galpin, A., & Head, J. (2016). The reality of myoelectric prostheses: Understanding what makes these devices difficult for some users to control. Frontiers in Neurorobotics, 10, 7. https://doi.org/10.3389/fnbot.2016.00007
- Chadwell, A., Kenney, L., Thies, S., Head, J., Galpin, A., & Baker, R. (2021). Addressing unpredictability may be the key to improving performance with current clinically prescribed myoelectric prostheses. Scientific Reports, 11(1), 3300. https://doi.org/10.1038/s41598-021-82764-6
- Eysenck, M. W., & Calvo, M. G. (1992). Anxiety and performance: The processing efficiency theory. Cognition & Emotion, 6(6), 409–434. https://doi.org/10.1080/02699939208409696
- Faul, F., Erdfelder, E., Lang, A.-G., & Buchner, A. (2007). G*Power 3: A flexible statistical power analysis program for the social, behavioral, and biomedical sciences. Behavior Research Methods, 39(2), 175–191. https://doi.org/10.3758/BF03193146
- Frykberg, G. E., Grip, H., & Alt Murphy, M. (2021). How many trials are needed in kinematic analysis of reach-to-grasp?—A study of the drinking task in persons with stroke and non-disabled controls. Journal of NeuroEngineering and Rehabilitation, 18(1), 101. https://doi.org/10.1186/s12984-021-00895-3
- Hebert, J. S., Boser, Q. A., Valevicius, A. M., Tanikawa, H., Lavoie, E. B., Vette, A. H., Pilarski, P. M., & Chapman, C. S. (2019). Quantitative eye gaze and movement differences in visuomotor adaptations to varying task demands among upper-extremity prosthesis users. JAMA Network Open, 2(9), e1911197. https://doi.org/10.1001/jamanetworkopen.2019.11197
- Jabban, L., Metcalfe, B. W., Raines, J., Zhang, D., & Ainsworth, B. (2022). Experience of adults with upper-limb difference and their views on sensory feedback for prostheses: A mixed methods study. Journal of NeuroEngineering and Rehabilitation, 19(1), 80. https://doi.org/10.1186/s12984-022-01054-y
- Land, M. F. (2009). Vision, eye movements, and natural behavior. Visual Neuroscience, 26(1), 51–62. https://doi.org/10.1017/S0952523808080899
- Light, C. M., Chappell, P. H., & Kyberd, P. J. (2002). Establishing a standardized clinical assessment tool of pathologic and prosthetic hand function: Normative data, reliability, and validity. Archives of Physical Medicine and Rehabilitation, 83(6), 776–783. https://doi.org/10.1053/apmr.2002.32737
- Maxwell, J. P., Masters, R. S. W., Kerr, E., & Weedon, E. (2001). The implicit benefit of learning without errors. The Quarterly Journal of Experimental Psychology. A, Human Experimental Psychology, 54(4), 1049–1068. https://doi.org/10.1080/713756014
- Oldfield, R. C. (1971). The assessment and analysis of handedness: The Edinburgh inventory. Neuropsychologia, 9(1), 97–113. https://doi.org/10.1016/0028-3932(71)90067-4
- Parr, J. V. V., Galpin, A., Uiga, L., Marshall, B., Wright, D. J., Franklin, Z. C., & Wood, G. (2023). A tool for measuring mental workload during prosthesis use: The Prosthesis Task Load Index (PROS-TLX). PLoS One, 18(5), e0285382. https://doi.org/10.1371/journal.pone.0285382
- Parr, J. V. V., Vine, S. J., Harrison, N. R., & Wood, G. (2018). Examining the spatiotemporal disruption to gaze when using a myoelectric prosthetic hand. Journal of Motor Behavior, 50(4), 416–425. https://doi.org/10.1080/00222895.2017.1363703
- Parr, J. V. V., Vine, S. J., Wilson, M. R., Harrison, N. R., & Wood, G. (2019). Visual attention, EEG alpha power and T7-Fz connectivity are implicated in prosthetic hand control and can be optimized through gaze training. Journal of NeuroEngineering and Rehabilitation, 16(1), 52. https://doi.org/10.1186/s12984-019-0524-x
- Parr, J. V. V., Wright, D. J., Uiga, L., Marshall, B., Mohamed, M. O., & Wood, G. (2022). A scoping review of the application of motor learning principles to optimize myoelectric prosthetic hand control. Prosthetics and Orthotics International, 46(3), 274–281. https://doi.org/10.1097/PXR.0000000000000083
- Poolton, J. M., Masters, R. S. W., & Maxwell, J. P. (2005). The relationship between initial errorless learning conditions and subsequent performance. Human Movement Science, 24(3), 362–378. https://doi.org/10.1016/j.humov.2005.06.006
- Sailer, U., Flanagan, J. R., & Johansson, R. S. (2005). Eye–hand coordination during learning of a novel visuomotor task. The Journal of Neuroscience: The Official Journal of the Society for Neuroscience, 25(39), 8833–8842. https://doi.org/10.1523/JNEUROSCI.2658-05.2005
- Salminger, S., Stino, H., Pichler, L. H., Gstoettner, C., Sturma, A., Mayer, J. A., Szivak, M., & Aszmann, O. C. (2022). Current rates of prosthetic usage in upper-limb amputees – have innovations had an impact on device acceptance? Disability and Rehabilitation, 44(14), 3708–3713. https://doi.org/10.1080/09638288.2020.1866684
- SensoMotoric Instruments (SMI) (2016). IViewETG User Guide Version 2.7.
- Sinke, M., Chadwell, A., & Smit, G. (2022). State of the art of prosthesis simulators for the upper limb: A narrative review. Annals of Physical and Rehabilitation Medicine, 65(6), 101635. https://doi.org/10.1016/j.rehab.2022.101635
- Smail, L. C., Neal, C., Wilkins, C., & Packham, T. L. (2021). Comfort and function remain key factors in upper limb prosthetic abandonment: Findings of a scoping review. Disability and Rehabilitation. Assistive Technology, 16(8), 821–830. https://doi.org/10.1080/17483107.2020.1738567
- Sobuh, M. M. D., Kenney, L. P. J., Galpin, A. J., Thies, S. B., McLaughlin, J., Kulkarni, J., & Kyberd, P. (2014). Visuomotor behaviours when using a myoelectric prosthesis. Journal of NeuroEngineering and Rehabilitation, 11(1), 72. https://doi.org/10.1186/1743-0003-11-72
- Vine, S. J., Masters, R. S. W., McGrath, J. S., Bright, E., & Wilson, M. R. (2012). Cheating experience: Guiding novices to adopt the gaze strategies of experts expedites the learning of technical laparoscopic skills. Surgery, 152(1), 32–40. https://doi.org/10.1016/j.surg.2012.02.002